Multiregional radiomic model for breast cancer diagnosis: value of ultrasound-based peritumoral and parenchymal radiomics
Introduction
Breast cancer is the most common cancer diagnosed and the second leading cause of cancer death among women in the United States (1). Early detection and timely treatment are crucial to the prognosis of breast cancer patients (2,3). Ultrasound is a common breast screening technique to detect cancer without invasion and radiation (4,5). In clinical practice, ultrasound can be an alternative and complementary method to mammography and magnetic resonance imaging (MRI) (6). In 2013, the American College of Radiology (ACR) updated the 2nd edition of the Breast Imaging Reporting and Data System (BI-RADS) atlas, a standardized reporting system of breast pathology as seen on ultrasound (7). However, ultrasonic manifestations are generally based on the subjective interpretation of radiologists (8). Breast lesions classified as BI-RADS category 4a and higher (more than 2% malignant probability) warrant biopsy according to the guideline, which is highly sensitive while having some false positives inevitably (6,9).
Radiomics refers to the high-throughput calculation of quantitative imaging features that may not be visible to radiologists (10). Radiomics has been reported to potentially improve breast cancer diagnosis, prognosis, and treatment prediction (11). In recent years, many radiomic studies for discriminating malignant from benign lesions reported a wide range of area under the curve (AUC) values since these studies extracted features from different medical images and datasets and used various machine learning classifiers to build models (11,12). Discrimination of the ultrasound-based radiomic model achieved AUC values ranging from 0.820 to 0.825 (8,13,14). Recent studies (14-16) improved the discrimination of radiomics through multimodal ultrasound (color doppler or elastography)-based radiomics and deep learning radiomics. Considering breast cancer is a complex disease involving the interaction of tumor cells with host stromal cells, multiregional radiomic information could be a potential approach to improve the performance in diagnosing breast cancer (17,18).
The tumor microenvironment is critical for breast cancer development, progression, and treatment (19). Dynamic contrast enhanced (DCE)-MRI-based textural heterogeneity within the peritumoral region was significantly associated with the density of tumor-infiltrating lymphocytes in breast cancer, suggesting that radiomic features in the peritumoral region were associated with the tumor microenvironment (20). Parenchyma stromal cells are likewise essential in fostering tumor growth (18). Breast parenchyma could reflect the biological risk factors associated with breast cancer development (21). The radiological pattern of the parenchyma was linked to the risk of developing breast cancer (22,23). MRI- and mammography-based radiomics studies (24,25) have reported that added radiomic features beyond the lesion could improve diagnostic accuracy for breast cancer.
To our knowledge, reports on ultrasound-based radiomic features extracted from the region beyond the lesion distinguishing malignant from benign lesions have not been published (12). We hypothesized that the performance of radiomics in diagnosing breast cancer might be improved by combining the information from the lesion, peritumoral tissue, and parenchyma. Our study aimed to develop and validate a multiregional model by adding ultrasound-based peritumoral and ipsilateral parenchymal radiomic features for breast lesion classification. We present the following article in accordance with the TRIPOD reporting checklist (available at https://qims.amegroups.com/article/view/10.21037/qims-22-939/rc).
Methods
Patients
This study was conducted in accordance with the Declaration of Helsinki (as revised in 2013). The study was approved by the local medical ethics committee of the First Affiliated Hospital of Nanchang University (No. 220204013). All data used in this study were anonymized and did not involve personal privacy or commercial interests. Thus, the need for informed consent from all patients was waived.
This study continuously included patients who underwent breast lesion surgery at institution #1 from April 2018 to April 2022 and at institution #2 from October 2019 to October 2021. The inclusion criteria included: (I) lesion treated with surgical resection and clinicopathological results available; (II) ultrasound performed within 2 weeks before surgery; and (III) BI-RADS category (7) ≥3. The exclusion criteria included: (I) biopsy or anticancer treatment (surgery, radiotherapy, and chemotherapy) before ultrasound examination; (II) lesion and parenchyma unclear on ultrasound images due to artifacts; and c. lesion with diameter >40 mm excluded to ensure peritumoral tissue and parenchyma present for analysis. Only lesions with pathological results were included for multiple breast lesions, and complications of benign and malignant lesions were excluded.
A total of 591 breast lesions (median of maximum diameter 19 mm, range from 4 to 40 mm) of 526 patients (526 women; median age 46 years, range from 14 to 87 years) were included. We estimated that a sample size of a minimum of 185 lesions was required based on the following input: a type I error of 0.05, a confidence interval (CI) of 0.95, an AUC of 0.80, and a ratio of the number of benign to malignant lesions in the study sample of 1 (26). Breast lesions from institution #1 were randomly assigned to the training (n=339) and internal test cohorts (n=146) at a ratio of 7:3, and the institution #2 dataset was used as the external test cohort (n=106) (Figure 1A).
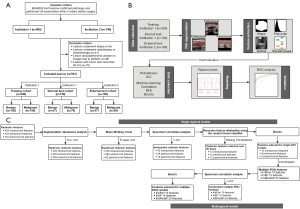
Ultrasound image analysis
Detailed image acquisition parameters in the two institutions are presented in Table S1. Two radiologists blinded to the pathological results reviewed the images using the Picture Archiving and Communication Systems (PACS). The lesion size (maximum diameter), location (quadrant and distance between the lesion and nipple), and BI-RADS category were estimated by radiologists in consensus.
Lesion segmentation
An image showing the largest lesion area and an image showing normal parenchyma 10–20 mm from the lesion were identified as the lesion section and parenchymal section of each target lesion. Radiologist #1, blinded to the pathological results, manually segmented the region of interest (ROI) using the 3D Slicer (version 5.1.0) software. The radiologist delineated the intratumoral ROI along the nodule margin. A morphologic dilation operation for the intratumoral ROI was performed to capture regions of 3-mm outside lesions (20,27). The intratumoral mask was then subtracted from the dilated mask to obtain a ring mask around the lesion. The part beyond the pre-mammary and retro-mammary fat was manually erased from each ring mask to get the peritumoral ROI. The strip-shaped breast parenchymal region (including premammary fat, fibroglandular tissue, and retro-mammary fat) was delineated as the parenchymal ROI. Segmentation robustness of radiomic features was evaluated using 30 randomly selected lesions. Radiologist #2 delineated the ROI twice within 2 weeks to evaluate the intra-observer agreement; meanwhile, the ROI was compared with that delineated by radiologist #1 to assess the inter-observer agreement. The agreement was assessed using the intraclass correlation coefficient (ICC).
Feature extraction
Radiomic features were calculated in 2-dimension because the study used a single-section ROI. All images were resampled to 2 mm × 2 m × 0 mm voxel size using linear interpolation, and voxel values were aggregated into bins 5 wide to partially reduce the heterogeneous settings in image collection (28). Pyradiomics (version 3.0.1) extracted 464 radiomic features from each intratumoral ROI, including shape-based (n=9), first-order statistics (n=18), textural (n=73), and wavelet-based features (n=364). Besides, 455 peritumoral features and 455 parenchymal features were extracted, including first-order statistics, textural, and wavelet-based features (Appendix 1). Since different radiomic features have different value ranges, which makes it difficult to compare features with variable orders of magnitude, a preprocessing step (Z-score) was used to standardize the radiomic features (29).
Feature selection and modeling
Feature selection was performed using lesions in the training cohort. To ensure the robustness of models, features with ICC values >0.80 were used for the following analysis. The Mann-Whitney U test was performed to test for differences in each radiomic feature of benign and malignant lesions, and features with P<0.05 were reserved. Features showing high pairwise Spearman correlation (r>0.80) and the highest mean correlation with all remaining features were removed. Then, the recursive feature elimination using the random forest as a based classifier and combing with a 5-fold cross-validation algorithm was trained in 100 iterations. The top 8 ranked features were selected for each iteration. Features selected 30 or more times were retained. Finally, Boruta (28) method was used to select features to train single-ROI (the intratumoral, peritumoral, and parenchymal) models. The correlation and Boruta steps were used to select integrated features of single-ROI models to train the intratumoral & peritumoral (In&Peri), intratumoral & parenchymal (In&P), and intratumoral & peritumoral & parenchymal (In&Peri&P; multiregional) models. The 6 radiomic models were developed using the random forest classifier (Figure 1B).
Model validation
Model performance was evaluated in the internal and external test cohorts. Discrimination was assessed using the receiver operating characteristic (ROC) analysis, sensitivity, specificity, and accuracy. The integrated discrimination improvement (IDI) test evaluated the performance improvement of radiomic models with added peritumoral and parenchymal features by summarizing the extent to which a new model increases risk in events and decreases risk in non-events (30). Calibration was assessed using the calibration curve and the Hosmer-Lemeshow test, which assesses whether or not the observed event rates match expected event rates in subgroups of the model population (31).
Performance of the radiomic signature score
In this study, the malignant probability predicted by the best radiomic model is called the radiomic signature score (Rad-score). The optimal cutoff value of the Rad-score was determined by maximizing the Youden index. Univariate and multivariate logistic regression analyses determined whether the Rad-score related to breast cancer discrimination beyond clinical factors. We further constructed a combined (Rad-BI-RADS) category by downgrading and upgrading the BI-RADS according to the optimal Rad-score cutoff value: the BI-RADS category was downgraded when the Rad-score below the cutoff value and the BI-RADS category was upgraded when the Rad-score higher the cutoff value. The sensitivity, specificity, and accuracy were used as performance metrics.
Statistical analysis
Statistical analysis was performed with R software (version 4.0.1). R software packages used are presented in Table S2. Continuous data are expressed as the median with interquartile range (IQR) and compared using the Mann-Whitney U and Kruskal-Wallis H tests. Categorical data are expressed as numbers with percentages and compared using the chi-square test or Fisher’s exact test. R codes are available at GitHub (https://github.com/feliciahz/multiregional). All statistical tests were two-sided, the Holm-Bonferroni method (32) was applied to adjust for multiple comparisons, and P<0.05 was considered statistically significant. The validity of radiomic studies was assessed using the radiomics quality score, which is 55.6% (20 of 36) in this study (33).
Results
Patient clinicopathological characteristics
Baseline clinicopathological characteristics are presented in Table 1. Analysis showed no significant differences in clinicopathological characteristics between different cohorts. Breast cancer accounted for 54.9% (186/339), 54.1% (79/146), and 65.1% (69/106) of the training, internal test, and external test cohorts, respectively (Kruskal-Wallis H test, P=0.143). The characteristics of patients according to benign and malignant lesions are listed in Table 2. Older age, larger size, non-inner quadrant location, and higher BI-RADS category were associated with breast cancer (Mann-Whitney U test, all P<0.01).
Table 1
Characteristics | Training cohort (patient n=301; lesion n=339) | Internal test cohort (patient n=131; lesion n=146) | External test cohort (patient n=94; lesion n=106) | P value |
---|---|---|---|---|
Age (years) | 46 [38–52] | 46 [40–53] | 47 [42–53] | 0.467 |
Conventional ultrasound | ||||
Lesion size (mm) | 19 [12–30] | 18 [13–29] | 19 [13–26] | 0.903 |
Tumor location | 0.954 | |||
Inner quadrant | 105 (31.0) | 44 (30.1) | 30 (28.3) | |
Outer quadrant | 212 (62.5) | 94 (64.4) | 68 (64.2) | |
Subareolar | 22 (6.5) | 8 (5.5) | 8 (7.5) | |
Distance from the nipple (mm) | 24 [10–35] | 25 [10–40] | 25 [15–36] | 0.414 |
BI-RADS category* | 0.902 | |||
3 | 65 (19.2) | 29 (19.9) | 19 (17.9) | |
4a | 105 (31.0) | 44 (30.1) | 28 (26.4) | |
4b | 77 (22.7) | 34 (23.3) | 31 (29.2) | |
4c | 45 (13.3) | 15 (10.3) | 12 (11.3) | |
5 | 47 (13.9) | 24 (16.4) | 16 (15.1) | |
Pathology | 0.143 | |||
Benign | 153 (45.1) | 67 (45.9) | 37 (34.9) | |
Malignant | 186 (54.9) | 79 (54.1) | 69 (65.1) |
Continuous data are presented as median [interquartile range]. Categorical data are presented as the number of lesions (percentage). *, BI-RADS category was referred for the senior radiologist. BI-RADS, Breast Imaging Reporting and Data System.
Table 2
Characteristics | Benign (n=257) | Malignant (n=334) | P value |
---|---|---|---|
Age (years) | 42 [32–48] | 48 [44–56] | <0.001 |
Conventional ultrasound | |||
Lesion size (mm) | 14 [10–23] | 23 [16–33] | <0.001 |
Tumor location | 0.003 | ||
Inner quadrant | 98 (38.1) | 89 (26.6) | |
Outer quadrant/subareolar | 159 (61.9) | 245 (73.4) | |
Distance from the nipple (mm) | 25 [12–40] | 25 [10–35] | 0.706 |
BI-RADS category* | <0.001 | ||
3 | 105 (40.9) | 8 (2.4) | |
4a | 113 (44.0) | 64 (19.2) | |
4b | 24 (9.3) | 118 (35.3) | |
4c | 10 (3.9) | 62 (18.6) | |
5 | 5 (1.9) | 82 (24.6) | |
Pathology | – | ||
Invasive ductal carcinoma | – | 263 (78.7) | |
Invasive lobular carcinoma | – | 14 (4.2) | |
Ductal carcinoma in situ | – | 34 (10.2) | |
Metaplastic carcinoma | – | 10 (3.0) | |
Fibroadenoma | 117 (45.5) | – | |
Adenosis | 77 (30.0) | – | |
Intraductal papilloma | 27 (10.5) | – | |
Benign phyllodes tumor | 6 (2.3) | – | |
Mastitis | 23 (8.9) | – | |
Other | 7 (2.7) | 13 (3.9) |
Continuous data are presented as median [interquartile range]. Categorical data are presented as the number of lesions (percentage). *, BI-RADS category was referred for the senior radiologist. BI-RADS, Breast Imaging Reporting and Data System.
Feature selection
The mean ICCs of intratumoral, peritumoral, and parenchymal radiomic features were 0.895, 0.889, and 0.961 for intra-observer agreement and 0.887, 0.882, and 0.902 for inter-observer agreement (Figure S1). Thus, 403 intratumoral, 383 peritumoral, and 393 parenchymal features with robustness in segmentation were reserved. Figure 1C shows the flowchart of feature selection. For the single-ROI models, 10, 9, and 5 features were selected to train the intratumoral, peritumoral, and parenchymal models. For the multiple-ROIs models, 19, 14, and 23 features were selected to train the In&Peri, In&P, and In&Peri&P models. Figure S2 describes the selection frequency of features in the 100 iterations of recursive feature elimination. Selected features of models were described in Table S3. Figure 2 shows representative heatmaps of radiomic features. The AUC values of models ranged from 0.689 to 0.921 in the training cohort (Table S4).
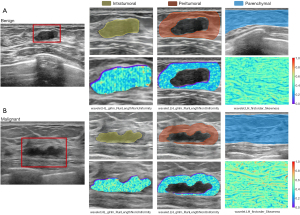
Radiomic models performance
The intratumoral, peritumoral, and parenchymal models achieved AUC values of 0.849, 0.864, and 0.670 in the internal test cohort and 0.838, 0.847, and 0.676 in the external test cohort. AUC values of the In&Peri, In&P, and In&Peri&P models were 0.892, 0.866, and 0.929 in the internal test cohort and 0.866, 0.863, and 0.911 in the external test cohort. The In&P model had the highest sensitivity (89% and 86%), the In&Peri model had the highest specificity (88% and 78%), and the In&Peri&P model had the highest accuracy (82% and 80%) in the internal and external test cohort (Table 3). The intratumoral, In&Peri, and In&Peri&P models showed proper calibrations (Hosmer-Lemeshow test, all P>0.05) (Table S5).
Table 3
Model | Internal test cohort (n=146) | External test cohort (n=106) | |||||||
---|---|---|---|---|---|---|---|---|---|
Sen (%) | Spe (%) | Acc (%) | AUC | Sen (%) | Spe (%) | Acc (%) | AUC | ||
Intratumoral | 75 [64–84] | 78 [66–87] | 76 [68–83] | 0.849 [0.787–0.911] | 75 [64–85] | 76 [59–88] | 75 [66–83] | 0.838 [0.762–0.914] | |
Peritumoral | 68 [57–78] | 88 [78–95] | 77 [70–84] | 0.864 [0.807–0.922] | 74 [62–84] | 76 [59–88] | 75 [65–82] | 0.847 [0.775–0.919] | |
Parenchymal | 73 [62–83] | 46 [34–59] | 61 [53–69] | 0.670 [0.582–0.758] | 86 [75–93] | 35 [20–53] | 68 [58–77] | 0.676 [0.570–0.783] | |
In&Peri | 65 [53–75] | 88 [78–95] | 75 [68–82] | 0.892 [0.842–0.942] | 77 [65–86] | 78 [62–90] | 77 [68–85] | 0.866 [0.800–0.933] | |
In&P | 89 [79–95] | 63 [50–74] | 77 [69–83] | 0.866 [0.810–0.923] | 86 [75–93] | 62 [45–78] | 77 [68–85] | 0.863 [0.795–0.932] | |
In&Peri&P | 82 [72–90] | 82 [71–90] | 82 [75–88] | 0.929 [0.891–0.967] | 84 [73–92] | 73 [56–86] | 80 [71–87] | 0.911 [0.860–0.963] |
Data in parentheses are 95% CIs. In&Peri = intratumoral & peritumoral model; In&P = intratumoral & parenchymal model; In&Peri&P = intratumoral & peritumoral & parenchymal model. Acc, accuracy; AUC, area under the receiver operator characteristic curve; CI, confidence interval; Sen, sensitivity; Spe, specificity.
Performance comparison
AUC of the intratumoral model was indifferent to that of the peritumoral model (IDI test, P=0.529 and 0.435) and significantly better than that of the parenchymal model (IDI test, both P<0.05) in the internal and external test cohorts. The In&Peri and In&P models significantly improved the AUC of the intratumoral model (IDI test, all P<0.05). The In&Peri&P model significantly improved AUC values (range from 0.037 to 0.080) of the intratumoral model, In&Peri, and In&P models (IDI test, all P<0.05) (Table 4). Through performance comparison, the malignant probability predicted by the In&Peri&P model is the Rad-score of this study. Figure S3 shows the important ranking of radiomic features of the In&Peri&P model.
Table 4
Model | Internal test cohort (n=146) | External test cohort (n=106) | |||||
---|---|---|---|---|---|---|---|
Improved AUC | IDI (95% CI) | IDI test P value | Improved AUC | IDI (95% CI) | IDI test P value | ||
Intratumoral vs. adding features model comparison | |||||||
Intratumoral vs. peritumoral | 0.015 | 0.029 (−0.062 to 0.120) | 0.529 | 0.009 | 0.017 (−0.026–0.056) | 0.435 | |
Intratumoral vs. parenchymal | −0.179 | −0.265 (−0.350 to −0.181) | <0.001 | −0.162 | −0.219 (−0.338 to −0.101) | 0.002 | |
Intratumoral vs. In&Peri | 0.043 | 0.080 (0.012–0.148) | 0.036 | 0.028 | 0.048 (0.011–0.086) | 0.019 | |
Intratumoral vs. In&P | 0.017 | 0.029 (0.002–0.057) | 0.047 | 0.025 | 0.030 (0.004–0.056) | 0.032 | |
Intratumoral vs. In&Peri&P | 0.080 | 0.200 (0.133–0.267) | <0.001 | 0.073 | 0.143 (0.047–0.239) | 0.009 | |
Multiregional model comparison | |||||||
In&Peri vs. In&Peri&P | 0.037 | 0.120 (0.065–0.176) | <0.001 | 0.045 | 0.095 (0–0.189) | 0.049 | |
In&P vs. In&Peri&P | 0.063 | 0.171 (0.107–0.234) | <0.001 | 0.048 | 0.113 (0.017–0.210) | 0.043 |
Data in parentheses are 95% CIs. In&Peri = intratumoral & peritumoral model; In&P = intratumoral & parenchymal model; In&Peri&P = intratumoral & peritumoral & parenchymal model. AUC, area under the receiver operator characteristic curve; CI, confidence interval; IDI, integrated discrimination improvement.
Performance of the radiomic signature score
The Rad-score showed satisfying discrimination and calibration in the training and test cohorts (Figure 3). The Rad-score of malignant lesions was significantly higher than that of benign lesions (Mann-Whitney U test, all P<0.001). Univariate and multivariate analyses showed that the Rad-score remained the strongest risk factor beyond the clinical data, whether in training or the test cohorts (Table S6). The optimal cutoff value of the Rad-score was set to be 0.5. The Rad-score had significantly higher specificity and accuracy while lower sensitivity than the BI-RADS category. The Rad-BI-RADS category significantly improved specificity and accuracy and maintained sensitivity of the BI-RADS category (Table S7). Figure 4 shows the risk classification of breast lesions for the Rad-BI-RADS category.
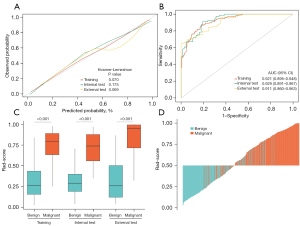
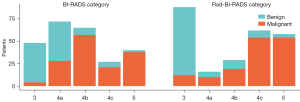
Discussion
In this study, we demonstrated the ability of ultrasound-based radiomic features extracted from peritumoral and parenchymal regions to diagnose breast cancer. The multiregional (In&Peri&P) model showed an improved lesion classification performance compared with the intratumoral, In&Peri, and In&P models by integrating different regional radiomic information. The Rad-score can be considered a helpful tool in aiding breast cancer diagnosis.
Ultrasound-based radiomics can identify benign from malignant breast lesions (8,13). Discrimination of the intratumoral model (AUC values 0.849 and 0.838) in our study was comparable to that of previous studies (8,13,14). Our study improved the discrimination of the intratumoral model by adding peritumoral and ipsilateral parenchymal radiomic analyses. Breast cancer consists not only of neoplastic cells but also significant changes in the surrounding stroma or tumor microenvironment (17). Associations between the heterogeneity of peritumoral radiomics and the immune response at the molecular and morphometric scales have also been reported, suggesting that peritumoral radiomics could reflect the tumor microenvironment (20,34,35). Recent studies (27,36) have demonstrated the potential of peritumoral radiomics in diagnosing breast cancer, identifying subtypes, and predicting neoadjuvant chemotherapy response. Niu et al. (36) demonstrated that digital breast tomosynthesis-based peritumoral radiomic features could diagnose malignant breast lesions with an AUC of 0.851. In this study, the In&Peri model achieved higher classification performance (AUC values 0.892 and 0.866) than the intratumoral model. Therefore, radiomics within the peritumoral region may add value to breast lesion classification. The diagnostic ability of peritumoral radiomics may be that first-order (Maximum) and textural features (such as RunLengthNonUniformity and Busyness) reflected the cluttered and dense arrangement of various components of the breast cancer microenvironment (such as suppressive immune cells, soluble factors, and extracellular matrix) and morphological changes in medical imaging caused by the migration of tumor cells from primary to peritumoral regions.
The breast parenchyma may indicate a precancerous state since parenchyma stromal cells play an important role in breast tumor growth and development (18,24). The breast parenchymal pattern could assess the risk of breast cancer (22,23). Brisson et al. (37) found that parenchymal radiomic phenotype with higher complexity was breast cancer risk factors (25). Li et al. (24) demonstrated that mammography-based parenchymal radiomic features had discrimination in breast cancer diagnosis (AUC value 0.67), and combined intratumoral features improved the performance (AUC value 0.84). These studies provide theoretical and practical support for the value of radiomic analysis in breast parenchyma. In this study, the In&P model (AUC values 0.866 and 0.863) improved the performance of the intratumoral model. Elevated expression of skewness and strength features were components of our multiregional models, capturing a higher degree of complexity in the parenchyma. We used a strip-shaped parenchymal region 10–20 mm from the target lesion as the parenchymal ROI rather than a rectangular region behind the nipple, as reported in previous studies (24,25), since ultrasound can flexibly show the parenchymal sections according to the operation of radiologists.
Furthermore, the multiregional model was developed and validated with the best performance among our radiomic models. The In&Peri&P model yielded better performance (AUC values 0.929 and 0.911) than the intratumoral, In&Peri, and In&P models in distinguishing malignant breast lesions from benign lesions in the study. The improvement might be for the multiregional model integrating different discriminative data in different regions. Radiomics within the intratumoral region reflected the shape and texture of the tumor, those within the peritumoral region reflected the breast cancer microenvironment and the metastases around the tumor, and those within the parenchymal region reflected parenchymal complexity. Our correlation analysis showed that no feature of single-ROI models was deleted through correlation analysis in the feature selection process of the multiple-ROIs models, suggesting different diagnostic information in the different regions. Therefore, the multiregional model may optimize diagnostic information from ultrasound images to improve diagnostic performance.
The malignancy probability of the In&Peri&P model (Rad-score) was an independent risk factor in breast cancer diagnosis. The Rad-score improved the accuracy and specificity of the BI-RADS category in diagnosing malignant breast lesions. Although the Rad-score had a significantly lower false-positive rate, it resulted in a significantly higher false-negative rate. Therefore, this study provides a novel approach to combine risk classification guidelines and radiomics. Based on the cutoff value of the Rad-score (0.5), the BI-RADS category of each lesion was upgraded or downgraded, resulting in a novel category. The Rad-BI-RADS category improved the specificity and accuracy and maintained the sensitivity of the BI-RADS. Thus, combining the multiregional radiomic model with the risk stratification guideline may develop a breast screening tool with good sensitivity and little misdiagnose.
Our study had several limitations. First, this study was retrospective; thus, selection bias may be inevitable. A prospective study is needed to validate our models in the future. Second, the collection methods in our dataset were heterogeneous and utilized various ultrasound instruments, which may slightly impact the analysis of the results. Third, ultrasound has limitations in operator dependence and risk of interpretive error, which may impact the results. Fourth, the selection of peritumoral and parenchymal ROIs was based on breast size, lesion size, ultrasound operation, and references from previous studies (20,24,25,27); however, different ROIs, such as different ranges of the peritumoral region and location of the parenchyma, may affect the result. Finally, many radiogenomics studies on breast cancer have already been published (11), but this study lacks direct links between radiomics and tumor biological factors.
Conclusions
In conclusion, we show that ultrasound-based radiomic features extracted from peritumoral and parenchymal regions may improve diagnostic performance for breast cancer over intratumoral features alone. Breast cancer might be characterized by a round shape, dense and heterogeneous texture within and around the lesion, and ipsilateral complex parenchyma. The multiregional model may optimize radiomics in discriminating malignant from benign breast lesions, which suggests its potential in assisting the diagnosis of the risk stratification guideline.
Acknowledgments
Funding: None.
Footnote
Reporting Checklist: The authors have completed the TRIPOD reporting checklist. Available at https://qims.amegroups.com/article/view/10.21037/qims-22-939/rc
Conflicts of Interest: All authors have completed the ICMJE uniform disclosure form (available at https://qims.amegroups.com/article/view/10.21037/qims-22-939/coif). The authors have no conflicts of interest to declare.
Ethical Statement: The authors are accountable for all aspects of the work in ensuring that questions related to the accuracy or integrity of any part of the work are appropriately investigated and resolved. This study was conducted in accordance with the Declaration of Helsinki (as revised in 2013). The study was approved by the local medical ethics committee of the First Affiliated Hospital of Nanchang University (No. 220204013). All data used in this study was anonymized and did not involve personal privacy or commercial interests. Thus, the need for informed consent from all patients was waived.
Open Access Statement: This is an Open Access article distributed in accordance with the Creative Commons Attribution-NonCommercial-NoDerivs 4.0 International License (CC BY-NC-ND 4.0), which permits the non-commercial replication and distribution of the article with the strict proviso that no changes or edits are made and the original work is properly cited (including links to both the formal publication through the relevant DOI and the license). See: https://creativecommons.org/licenses/by-nc-nd/4.0/.
References
- DeSantis CE, Ma J, Gaudet MM, Newman LA, Miller KD, Goding Sauer A, Jemal A, Siegel RL. Breast cancer statistics, 2019. CA Cancer J Clin 2019;69:438-51. [Crossref] [PubMed]
- Lin X, Zhuang S, Yang S, Lai D, Chen M, Zhang J. Development and internal validation of a conventional ultrasound-based nomogram for predicting malignant nonmasslike breast lesions. Quant Imaging Med Surg 2022;12:5452-61. [Crossref] [PubMed]
- Michell MJ. Breast screening review--a radiologist's perspective. Br J Radiol 2012;85:845-7. [Crossref] [PubMed]
- Gonzaga MA. How accurate is ultrasound in evaluating palpable breast masses? Pan Afr Med J 2010;7:1. [PubMed]
- Irurhe NK, Adekola OO, Awosanya GO, Adeyomoye AO, Olowoyeye OA, Awolola NA, Olajide TO. The accuracy of ultrasonography in the diagnosis of breast pathology in symptomatic women. Nig Q J Hosp Med 2012;22:236-9. [PubMed]
- Berg WA, Bandos AI, Mendelson EB, Lehrer D, Jong RA, Pisano ED. Ultrasound as the Primary Screening Test for Breast Cancer: Analysis From ACRIN 6666. J Natl Cancer Inst 2015;108:djv367. [Crossref] [PubMed]
- Mendelson EB, Böhm-Vélez M, Berg WA, et al. ACR BI-RADS® ultrasound. ACR BI-RADS® atlas, breast imaging reporting and data system 2013;149. Available online: https://www.acr.org/Clinical-Resources/Reporting-and-Data-Systems/Bi-Rads#Ultrasound, is this what you need?
- Luo WQ, Huang QX, Huang XW, Hu HT, Zeng FQ, Wang W. Predicting Breast Cancer in Breast Imaging Reporting and Data System (BI-RADS) Ultrasound Category 4 or 5 Lesions: A Nomogram Combining Radiomics and BI-RADS. Sci Rep 2019;9:11921. [Crossref] [PubMed]
- Gu Y, Tian JW, Ran HT, Ren WD, Chang C, Yuan JJ, et al. The Utility of the Fifth Edition of the BI-RADS Ultrasound Lexicon in Category 4 Breast Lesions: A Prospective Multicenter Study in China. Acad Radiol 2022;29 Suppl 1:S26-34.
- Yan J, Xue X, Gao C, Guo Y, Wu L, Zhou C, Chen F, Xu M. Predicting the Ki-67 proliferation index in pulmonary adenocarcinoma patients presenting with subsolid nodules: construction of a nomogram based on CT images. Quant Imaging Med Surg 2022;12:642-52. [Crossref] [PubMed]
- Conti A, Duggento A, Indovina I, Guerrisi M, Toschi N. Radiomics in breast cancer classification and prediction. Semin Cancer Biol 2021;72:238-50. [Crossref] [PubMed]
- Gu J, Jiang T. Ultrasound radiomics in personalized breast management: Current status and future prospects. Front Oncol 2022;12:963612. [Crossref] [PubMed]
- Romeo V, Cuocolo R, Apolito R, Stanzione A, Ventimiglia A, Vitale A, Verde F, Accurso A, Amitrano M, Insabato L, Gencarelli A, Buonocore R, Argenzio MR, Cascone AM, Imbriaco M, Maurea S, Brunetti A. Clinical value of radiomics and machine learning in breast ultrasound: a multicenter study for differential diagnosis of benign and malignant lesions. Eur Radiol 2021;31:9511-9. [Crossref] [PubMed]
- Zhang Q, Xiao Y, Suo J, Shi J, Yu J, Guo Y, Wang Y, Zheng H. Sonoelastomics for Breast Tumor Classification: A Radiomics Approach with Clustering-Based Feature Selection on Sonoelastography. Ultrasound Med Biol 2017;43:1058-69. [Crossref] [PubMed]
- Moustafa AF, Cary TW, Sultan LR, Schultz SM, Conant EF, Venkatesh SS, Sehgal CM. Color Doppler Ultrasound Improves Machine Learning Diagnosis of Breast Cancer. Diagnostics (Basel) 2020.
- Jiang M, Li CL, Chen RX, Tang SC, Lv WZ, Luo XM, Chuan ZR, Jin CY, Liao JT, Cui XW, Dietrich CF. Management of breast lesions seen on US images: dual-model radiomics including shear-wave elastography may match performance of expert radiologists. Eur J Radiol 2021;141:109781. [Crossref] [PubMed]
- Soysal SD, Tzankov A, Muenst SE. Role of the Tumor Microenvironment in Breast Cancer. Pathobiology 2015;82:142-52. [Crossref] [PubMed]
- Mao Y, Keller ET, Garfield DH, Shen K, Wang J. Stromal cells in tumor microenvironment and breast cancer. Cancer Metastasis Rev 2013;32:303-15. [Crossref] [PubMed]
- Andre F, Berrada N, Desmedt C. Implication of tumor microenvironment in the resistance to chemotherapy in breast cancer patients. Curr Opin Oncol 2010;22:547-51. [Crossref] [PubMed]
- Braman N, Prasanna P, Whitney J, Singh S, Beig N, Etesami M, Bates DDB, Gallagher K, Bloch BN, Vulchi M, Turk P, Bera K, Abraham J, Sikov WM, Somlo G, Harris LN, Gilmore H, Plecha D, Varadan V, Madabhushi A. Association of Peritumoral Radiomics With Tumor Biology and Pathologic Response to Preoperative Targeted Therapy for HER2 (ERBB2)-Positive Breast Cancer. JAMA Netw Open 2019;2:e192561. [Crossref] [PubMed]
- Weigelt B, Bissell MJ. Unraveling the microenvironmental influences on the normal mammary gland and breast cancer. Semin Cancer Biol 2008;18:311-21. [Crossref] [PubMed]
- Saftlas AF, Szklo M. Mammographic parenchymal patterns and breast cancer risk. Epidemiol Rev 1987;9:146-74. [Crossref] [PubMed]
- McCormack VA, dos Santos Silva I. Breast density and parenchymal patterns as markers of breast cancer risk: a meta-analysis. Cancer Epidemiol Biomarkers Prev 2006;15:1159-69. [Crossref] [PubMed]
- Li H, Mendel KR, Lan L, Sheth D, Giger ML. Digital Mammography in Breast Cancer: Additive Value of Radiomics of Breast Parenchyma. Radiology 2019;291:15-20. [Crossref] [PubMed]
- Kontos D, Winham SJ, Oustimov A, Pantalone L, Hsieh MK, Gastounioti A, Whaley DH, Hruska CB, Kerlikowske K, Brandt K, Conant EF, Vachon CM. Radiomic Phenotypes of Mammographic Parenchymal Complexity: Toward Augmenting Breast Density in Breast Cancer Risk Assessment. Radiology 2019;290:41-9. [Crossref] [PubMed]
- Obuchowski NA. ROC analysis. AJR Am J Roentgenol 2005;184:364-72. [Crossref] [PubMed]
- Braman NM, Etesami M, Prasanna P, Dubchuk C, Gilmore H, Tiwari P, Plecha D, Madabhushi A. Intratumoral and peritumoral radiomics for the pretreatment prediction of pathological complete response to neoadjuvant chemotherapy based on breast DCE-MRI. Breast Cancer Res 2017;19:57. [Crossref] [PubMed]
- Shafiq-Ul-Hassan M, Zhang GG, Latifi K, Ullah G, Hunt DC, Balagurunathan Y, Abdalah MA, Schabath MB, Goldgof DG, Mackin D, Court LE, Gillies RJ, Moros EG. Intrinsic dependencies of CT radiomic features on voxel size and number of gray levels. Med Phys 2017;44:1050-62. [Crossref] [PubMed]
- Beaumont H, Iannessi A, Bertrand AS, Cucchi JM, Lucidarme O. Harmonization of radiomic feature distributions: impact on classification of hepatic tissue in CT imaging. Eur Radiol 2021;31:6059-68. [Crossref] [PubMed]
- Pencina MJ, D'Agostino RB Sr, Demler OV. Novel metrics for evaluating improvement in discrimination: net reclassification and integrated discrimination improvement for normal variables and nested models. Stat Med 2012;31:101-13. [Crossref] [PubMed]
- Kramer AA, Zimmerman JE. Assessing the calibration of mortality benchmarks in critical care: The Hosmer-Lemeshow test revisited. Crit Care Med 2007;35:2052-6. [Crossref] [PubMed]
- Holm S. A simple sequentially rejective multiple test procedure. Scandinavian Journal of Statistics 1979;6:65-70.
- Lambin P, Leijenaar RTH, Deist TM, Peerlings J, de Jong EEC, van Timmeren J, Sanduleanu S, Larue RTHM, Even AJG, Jochems A, van Wijk Y, Woodruff H, van Soest J, Lustberg T, Roelofs E, van Elmpt W, Dekker A, Mottaghy FM, Wildberger JE, Walsh S. Radiomics: the bridge between medical imaging and personalized medicine. Nat Rev Clin Oncol 2017;14:749-62. [Crossref] [PubMed]
- Hu Y, Xie C, Yang H, Ho JWK, Wen J, Han L, Chiu KWH, Fu J, Vardhanabhuti V. Assessment of Intratumoral and Peritumoral Computed Tomography Radiomics for Predicting Pathological Complete Response to Neoadjuvant Chemoradiation in Patients With Esophageal Squamous Cell Carcinoma. JAMA Netw Open 2020;3:e2015927. [Crossref] [PubMed]
- Beig N, Khorrami M, Alilou M, Prasanna P, Braman N, Orooji M, Rakshit S, Bera K, Rajiah P, Ginsberg J, Donatelli C, Thawani R, Yang M, Jacono F, Tiwari P, Velcheti V, Gilkeson R, Linden P, Madabhushi A. Perinodular and Intranodular Radiomic Features on Lung CT Images Distinguish Adenocarcinomas from Granulomas. Radiology 2019;290:783-92. [Crossref] [PubMed]
- Niu S, Yu T, Cao Y, Dong Y, Luo Y, Jiang X. Digital breast tomosynthesis-based peritumoral radiomics approaches in the differentiation of benign and malignant breast lesions. Diagn Interv Radiol 2022;28:217-25. [Crossref] [PubMed]
- Brisson J, Diorio C, Mâsse B. Wolfe's parenchymal pattern and percentage of the breast with mammographic densities: redundant or complementary classifications? Cancer Epidemiol Biomarkers Prev 2003;12:728-32. [PubMed]