Application of amide proton transfer imaging to pretreatment risk stratification of childhood neuroblastoma: comparison with neuron-specific enolase
Introduction
Neuroblastoma (NB) is a malignant embryonal tumor originating from primitive neural crest cells of the sympathetic nervous system, which occurs mainly in the abdomen, especially in the adrenal gland (1,2). NB represents the third most frequent pediatric malignancy and the most common extracranial solid tumor of childhood, and accounts for at least fifteen percent of cancer deaths in children (3). Many factors have been reported to be associated with the prognosis of NB, such as age, tumor stage, histopathology, MYCN amplification and DNA index (4). In 2009, the International Neuroblastoma Risk Group (INRG) classification system was established to stratify pretreatment patients into very-low-, low-, intermediate- and high-risk groups, according to clinical criteria and image-defined risk factors (5). Patients at very-low- and low-risk usually recover after surgical resection, and some may even undergo spontaneous regression. Intermediate-risk patients have a survival rate of more than 90% with surgery and chemotherapy. However, children with high-risk NB require intensive multimodality treatment, with dismal survival rates of 30% to 40% (6). In light of the variable therapies and prognosis for NB patients of different risk groups, accurate preoperative risk assessment is important for determining the treatment of this disease. Multiple clinical tests and imaging examinations are used to diagnose and assess NB (7). Except for incisional biopsy to confirm NB histologically, immunohistochemical tests can detect several useful markers for the diagnosis of NB, such as neuron-specific enolase (NSE), S-100 protein, and chromogranin (7). Notably, NSE is a glycogenolytic enzyme in the glycolysis pathway, and is routinely tested for NB via blood samples at our hospital. NSE is commonly present in neurons and neurogenic cells, and is released when cells are destroyed, and thus can be a useful index of neuroendocrine tumors (8). Since NB arises from neural crest cells, the NSE level in serum can serve as a biochemical marker for NB. Researchers have reported elevated NSE levels in NB, especially in advanced NB, and found that NSE indices are of diagnostic significance and easy to measure (9,10).
Computed tomography (CT) and magnetic resonance imaging (MRI) are routine imaging methods for NB risk evaluation (1). Both modalities allow delineation of the primary lesion, vessel encasement, adjacent involvement, and lymphatic invasion (11), while MRI is superior to CT in evaluating spinal canal extension and bone marrow metastasis (12). In addition, the CT examination requires intravenous contrast materials to enhance the soft-tissue contrast and is accompanied by substantial radiation exposure, which may threaten children’s health (13). On the other hand, though MRI is radiation-free, its prolonged acquisition often requires young children to be sedated to reduce motion artifacts (13). Besides, injection of gadolinium-based contrast agents in MRI is associated with gadolinium deposition and rare nephrogenic systemic fibrosis, limiting the use of contrast-enhanced MRI in certain situations (14).
Amide proton transfer (APT) imaging (15) is an emerging molecular MRI technique, which is a subtype of chemical exchange saturation transfer (CEST) imaging (16). APT MRI saturates the amide protons in mobile peptides and proteins with frequency-selective radiofrequency (RF) irradiation, and detects the chemical exchange between the amide protons and bulk water protons through the decreased water signal, thus indirectly reflecting the signal of proteins in tissue without exogenous contrast agents (17). Prior studies have suggested that the concentration of amide protons in tumors is higher than that in normal tissues (18). Moreover, APT imaging has been successfully applied to various diseases in humans, such as brain tumors (19,20), rectal cancer (21), ischemic stroke (22) and hepatocellular carcinoma (23), demonstrating that APT MRI is a non-invasive and promising molecular MRI technique for clinical use. A recent work explored the feasibility of APT imaging in preoperative NB risk stratification, and found that APT MRI was able to identify high-risk NB and outperformed conventional MRI in a cohort of 24 patients (24). This prior work used the conventional asymmetry analysis method, and discarded motion-corrupted tumor regions according to the smoothness of the z-spectrum.
Here, we aim to verify the clinical value of APT imaging for pretreatment risk assessment of pediatric neuroblastoma with advanced Lorentzian fitting methods for better handling of motion artifacts and including the whole tumor in the analysis. In addition, we compare the diagnostic performance of APT MRI with NSE indices in serum—a routine biomarker of NB in clinics. We present the article in accordance with the STARD reporting checklist (available at https://qims.amegroups.com/article/view/10.21037/qims-22-780/rc).
Methods
Patients
This prospective study was conducted following the Declaration of Helsinki (as revised in 2013) and was approved by the Ethics Committee of the Children’s Hospital at Zhejiang University School of Medicine (No. 2019-IRB-048), and informed consent was obtained from each volunteer’s legal guardian. From April 2019 to November 2021, eighty-six consecutive patients who underwent MR examinations at our hospital were consecutively enrolled in this study. The inclusion criteria we used were as follows: (I) suspected of NB; (II) under 18 years old; and (III) eligible for MRI. The exclusion criteria were: (I) none-NB lesion from pathology; (II) MR examination performed after treatment; (III) lack of clinical data (pathology or NSE results); (IV) incomplete MRI data; (V) tumor size less than 2 cm3; and (VI) poor quality of APT images. The patient selection flowchart is shown in Figure 1.
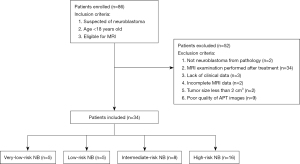
Without knowledge of APT and NSE indices, the risk stratification of NB patients was performed according to clinical information (age, tumor stage, histopathology, MYCN amplification, DNA index, etc.) required by the INRG classification system (5).
The sample size estimate for the study was based on a previous work (24) and our preliminary experiment. We compared the APT index between high-risk and non-high-risk NBs using receiver operating characteristic (ROC) analysis, with a standard area under the curve (AUC) of 0.5, and an expected AUC of 0.85. Alpha (α) was 0.05 (two-sided), and beta (β) was 0.10, with an allocation ratio of 1:1. PASS software (Version 21.0.3, NCSS LLC, Kaysville, USA) was used to calculate the sample size, and the calculated sample size was 11 per risk group. We also performed a multiple comparison of APT values in four risk groups using the one-way analysis of variance (ANOVA) test. The following settings were used in calculating sample size: α=0.05, β=0.1, number of groups =4; allocation ratio =1:1:1:3; group means =3.2%, 3.7%, 4.4% and 5.8%; and standard deviation =0.6%, 1.4%, 0.7% and 1.3%. Based on these, the estimated sample size was 4, 4, 4 and 12 for the very-low-, low-, intermediate- and high-risk groups, respectively.
NSE assay
The NSE concentration in serum was measured by an electro-chemiluminescence immunoassay on an automated analyzer (Roche Diagnostics, Mannheim, Germany). The NSE assay was done blinded to the other clinical data. The detection range was 0.05–370 ng/mL, and cases with NSE levels beyond this range would be excluded from the study (n=1) under the “lack of clinical data” category. An NSE value above 16.3 ng/mL was considered abnormal.
MRI
All participants were imaged on a 3.0 T MRI scanner (Achieva, Philips Healthcare, Best, The Netherlands) with a dual-channel body transmit coil and an eight-channel receive coil. Patients under 5 years old were sedated by rectal or oral administration of 10% chloral hydrate solution 30–40 minutes before the examination, and scanned after sleeping. Ear protection was used for children, and the MRI scan was performed under free-breathing, with T1-weighted (T1w), T2-weighted (T2w), fluid-attenuated inversion recovery (FLAIR), and APT imaging sequences.
The axial APT scans were implemented with a single-slice frequency-stabilized CEST sequence (25,26) using turbo-spin-echo readout (27). The transverse T2w image was used as a reference to locate the APT slice to the cross-section with the largest lesion. Four saturation pulses constituted the APT saturation module, each with a power of 2 µT and a duration of 0.2 s, in accordance with the APT consensus paper (28). Plus, a 10-ms-long and 10-mT/m-strong spoiler gradient was added between saturation pulses. A total of 63 APT source images were acquired for each patient, including an unsaturated reference image and 62 saturated images with frequency offsets at 0, ±0.25, ±0.5, ±0.75, ±1, ±1.5, ±2 [2], ±2.5 [2], ±3 [2], ±3.25 [2], ±3.5 [6], ±3.75 [2], ±4 [2], ±4.5, ±5, ±6, 10, 15.625, 20, 30, 40, 50, 60, 70, and 80 ppm, where the number in the brackets referred to the number of repetitions (25,29). Other key APT imaging parameters were as follows: repetition time =3,000 ms, echo time =6.7 ms, slice thickness =5 mm, field of view =350×308 mm2 and acquisition resolution =2.2×2.2 mm2. In addition, a vendor-provided MIX sequence (30) was executed for quantitative T1 mapping. The entire examination duration of all MRI sequences was 15–20 minutes.
Image processing
All APT source images were registered to the saturated frame at +3.5 ppm (31) using the FLIRT module in FSL (V6.0, FMRIB, Oxford University) to correct the motion artifacts (31,32). Then, we applied the water saturation shift referencing (WASSR) method (33) to the registered APT images for correcting the main magnetic field inhomogeneity. A 4-pool Lorentzian fit of the z-spectrum (34) was used to separate the APT signal from the other CEST signals and mitigate residual motion artifacts. Representative fitting results of a tumor voxel are illustrated in Figure 2. The APT effect, direct water saturation, semisolid magnetization transfer signal, and nuclear Overhauser effect were identified by the fit at Δω= +3.5, 0, −2, and −3.5 ppm, respectively. Starting points and boundaries of the fit are given in Table 1. Then, we measured the isolated APT effect at +3.5 ppm with the following formula:
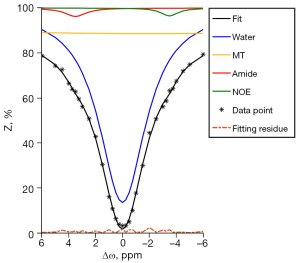
Table 1
Pool | Parameter | Starting values | Lower bound | Upper bound |
---|---|---|---|---|
Water (0 ppm) | Awater | 60 | 0.6 | 100 |
Γwater | 6 | 0.3 | 12 | |
MT (−2 ppm) | AMT | 10 | 0.1 | 100 |
ΓMT | 25 | 10 | 100 | |
Amide (+3.5 ppm) | Aamide | 2.5 | 0.025 | 20 |
Γamide | 4 | 0.2 | 8 | |
NOE (−3.5 ppm) | ANOE | 5 | 0.25 | 40 |
ΓNOE | 2 | 0.1 | 6 |
CEST component of each pool with its fixed resonance frequency is shown in the parentheses. The amplitude (A) of each pool is given in %. The linewidth (Γ) of each pool is given in ppm. MT, magnetization transfer; NOE, nuclear Overhauser effect; CEST, chemical exchange saturation transfer.
where Zlab represents the label spectrum, i.e., the full fitted z-spectrum, Zref is the reference spectrum which excludes the Lorentzian function of the APT signal from Zlab, and the subtraction of the two spectra retains only the APT pool. For comparison, we calculated the APT-weighted (APTw) signal with the conventional asymmetry analysis,
where Z represents the experimental spectrum.
Two senior pediatric radiologists (X. J. and X. M. with 18 and 17 years of clinical experience, respectively) independently defined the region of interest (ROI) on the unsaturated APT image, with reference to the conventional structural images. They were blinded to all patients’ clinical and histopathological data. The ROI was delineated along the edge of the tumor, avoiding the blood vessel, cystic degeneration, hemorrhage and calcification areas as much as possible. We calculated the APT indices in the ROIs from the two readers and averaged them for the following analysis. All the image processing except motion correction was performed with an in-house MATLAB (R2020a, MathWorks, Natick, MA, USA) tool.
Statistical analysis
The statistical analyses were performed using SPSS (Version 21.0, IBM SPSS Statistics, Chicago, USA) and MedCalc (Version 20.011, MedCalc Software, Ostend, Belgium). We used the intraclass correlation coefficient (ICC) to assess the consistency of APT indices measured from ROIs defined by two readers, with an ICC >0.9 considered excellent agreement. The ANOVA test with Bonferroni correction was used to compare the NSE and APT values of each risk group. And the differences in parameters between the non-high-risk and high-risk NB were analyzed using the independent-sample t-test (for normally distributed variables) or Mann-Whitney U-test (for non-normally distributed variables). The AUC of the ROC analysis and 95% confidence intervals (CI) were calculated to evaluate the performance of APT and NSE values in stratifying the risk groups of NB. Furthermore, we compared the AUCs of the two indices with the Delong test.
Results
As shown in Figure 1, among the 86 enrolled patients, 2 patients were not NB from pathological results, 34 subjects were excluded due to medical treatment before the MRI scan, 3 subjects were excluded for lacking clinical data, and 2 subjects had incomplete MRI data. Furthermore, 2 patients with tumor size less than 2 cm3, and 9 subjects with poor quality of APT images due to severe motion artifacts were excluded. Eventually, 34 patients (mean age: 38.6±32.4 months; age range: 7 days to 165 months; 24 males and 10 females) were included in the final analysis. The risk group of each participant was rated according to the criteria of the INRG classification system (5). Among the 34 patients included, 5 subjects were with very-low-risk NB, 5 with low-risk NB, 8 with intermediate-risk NB, and 16 with high-risk NB. Considering that patients with high-risk NB require more aggressive treatment and have a substantially lower survival rate than the others (6), we combined the very-low-risk, low-risk, and intermediate-risk NB into the non-high-risk group in this work. The demographics of included patients are summarized in Table 2.
Table 2
Risk group | No. of patients | Gender (males:females) | Age (months) |
---|---|---|---|
Non-high | |||
Very-low | 5 | 4:1 | 48.6±49.0 |
Low | 5 | 4:1 | 18.1±22.7 |
Intermediate | 8 | 4:4 | 33.0±7.4 |
High | 16 | 12:4 | 44.7±35.8 |
Total | 34 | 24:10 | 38.6±32.4 |
Ages are expressed in mean ± standard deviation.
Figure 3 displays the anatomical and APT images of patients with very-low-risk, low-risk, intermediate-risk and high-risk NB. As shown in the third column, the APT contrast was rather heterogeneous throughout the tumor and its signal strength positively correlated with the risk group of NB, reflecting different levels of amide proton content within and between tumors. The ICC of APT values measured from the ROIs defined by two readers was 0.91 (95% CI: 0.83–0.95), indicating an excellent inter-reader agreement of APT measurements.
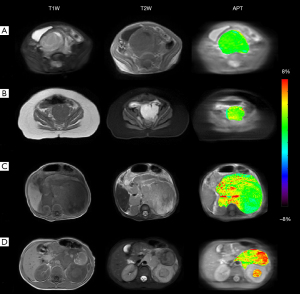
The NSE and APT values for different risk groups are summarized in Table 3. The mean NSE level was 19.32±5.91 ng/mL (mean ± standard deviation) for patients with very-low-risk NB, 53.62±48.30 ng/mL for subjects with low-risk NB, 47.68±21.90 ng/mL for cases with intermediate-risk NB, and 93.05±97.14 ng/mL for patients with high-risk NB. The one-way ANOVA test showed that there was no statistically significant difference (P=0.19) in the NSE values between any two risk groups. Moreover, the mean NSE value of the non-high-risk group was 41.45±30.99 ng/mL, which was not significantly different (P=0.06) from that of the high-risk group.
Table 3
Risk group | NSE (ng/mL) | APT (%) |
---|---|---|
Non-high | 41.45±30.99 | 3.88±1.01 |
Very-low | 19.32±5.91 | 3.23±0.57 |
Low | 53.62±48.30 | 3.65±1.40 |
Intermediate | 47.68±21.90 | 4.43±0.70 |
High | 93.05±97.14 | 5.80±1.27 |
P | 0.06 | <0.001 |
P values are for the difference between non-high-risk and high-risk groups. NSE, neuron-specific enolase; APT, amide proton transfer.
Differently, the mean APT value was 3.23%±0.57% for very-low-risk NB, 3.65%±1.40% for low-risk NB, 4.43%±0.70% for intermediate-risk NB, and 5.80%±1.27% for high-risk NB. There was a trend of increasing APT signals with the advancement of the NB risk. Moreover, the APT index of NB in the high-risk group was significantly higher than that in the very-low-risk (P<0.001), low-risk (P=0.004) and intermediate-risk (P=0.046) groups. In addition, the APT value was 3.88%±1.01% for non-high-risk NB, which was significantly lower than that for the high-risk group (P<0.001). Figure 4 presents boxplots of the NSE and APT values for patients with different risk groups.
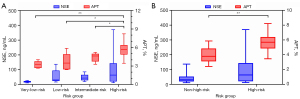
Figure 5 shows the ROC curves of using NSE and APT values for preoperatively differentiating pediatric patients with high-risk NB from those with non-high-risk NB. And the detailed ROC parameters are presented in Table 4. The AUC of NSE was only 0.639 (95% CI: 0.46–0.80), while the AUC of the mean APT value was 0.885 (95% CI: 0.73–0.97). Moreover, the Delong test revealed that the AUC of mean APT in stratifying the risk groups of NB was significantly higher than that of NSE (P=0.03).
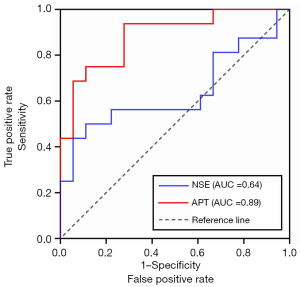
Table 4
Index | AUC | ROC threshold | Sensitivity | Specificity | P |
---|---|---|---|---|---|
NSE | 0.64 (0.46, 0.80) | 85.67 ng/mL | 43.8% | 94.4% | 0.17 |
APT | 0.89 (0.73, 0.97) | 5.03% | 75.0% | 88.9% | <0.001 |
Data in parentheses are 95% confidence intervals for AUCs. NSE, neuron-specific enolase; APT, amide proton transfer; NB, neuroblastoma; AUC, area under the ROC curve; ROC, receiver operating characteristic.
Furthermore, the mean (± standard deviation) T1 values were 1,479±139.2, 1,358±162.2, 1,404±167.6, and 1,343±154.4 ms for very-low-risk, low-risk, intermediate-risk and high-risk NB, respectively. The AUC of T1 in differentiating high-risk NB from non-high-risk NB was 0.590 (95% CI: 0.41–0.76), which was significantly lower than that of APT (P=0.008). In addition, the mean (± standard deviation) APTw value was 1.93%±0.84% for very-low-risk NB, 1.75%±1.26% for low-risk NB, 2.14%±0.91% for intermediate-risk NB, and 2.69%±0.91% for high-risk NB. And the AUC of the APTw metric in stratifying high-risk and non-high-risk NB was 0.691 (95% CI: 0.51–0.84), which was significantly lower than that of the fitted APT metric (P=0.02). These results demonstrated the advantage of the Lorentzian-fitted APT metric versus T1 and APTw indices.
Discussion
In this study, we investigated the potential of APT imaging with advanced Lorentzian fitting for preoperatively stratifying the risk groups of abdominal NB in children. In addition, we compared the performance of the APT signal with the NSE level in serum which is a commonly used biomarker for the prognosis of NB in routine clinics. It was found that the APT signal of high-risk NB was much higher than those of very-low-risk (P<0.001), low-risk (P=0.004) and intermediate-risk (P=0.046) NB. Plus, the APT index successfully distinguished high-risk NB from non-high-risk NB (P<0.001). In contrast, the NSE level did not exhibit any significant difference between risk groups, and it failed to discriminate high-risk NB from non-high-risk NB (P=0.18). Furthermore, the AUC of the APT metric (0.89) in classifying high-risk and non-high-risk NB was significantly higher (P=0.03) than that of NSE (0.64), reflecting the advantage of the novel molecular MRI technique.
A recent preliminary study (24) applied APT imaging to abdominal tumors in children, and showed that patients with high-risk NB had higher APT values than those with non-high-risk NB in part of the tumor, while quantitative T1 and T2 values failed to identify high-risk NB. Here, we increased the sample size and compared the performance of APT and NSE indices. Notably, in this study, we used a 4-pool Lorentzian fitting method to further mitigate residual motion artifacts after the image registration step (Figure S1), and isolate the true APT effect in the whole tumor. As a result, the AUC of APT MRI for distinguishing the high-risk NB from non-high-risk NB was 0.885, agreeing with the previous conclusion (24). Moreover, the APT signal increased with the NB risk (Figure 4 and Table 3). And the APT values between high-risk NB and the other three groups all showed significant differences (P<0.05).
Previous studies have demonstrated that the enriched amide protons of proteins and peptides in malignant tumors lead to hyperintensity on APT images (19,35). In addition, it has been reported that APT imaging can predict the histological grade of several cancers, such as diffuse glioma (36) and rectal tumor (21), and the APT signal intensity is associated with the cell density and proliferation index (36,37). Neuroblastoma derives from the abnormal proliferation of neural crest cells, and its histopathology, including tumor maturation, differentiation and nuclear morphology, is related to the distribution and aggressiveness of tumor cells (38). The amplification of the MYCN oncogene, another risk factor of NB, was reported to induce cell proliferation (39). Therefore, the successful application of APT imaging to classifying the risk groups of NB might be attributed to its ability to measure the protein content that correlated with cancer cell growth, proliferation and differentiation. In addition, the APT signal in NB might be related to the upregulated cytosolic, endoplasmic reticulum, and secreted proteins (40), cancer-promoting VIM and stathmin proteins (41), and decreased bound proteins in necrotic areas.
NSE is a protein produced during the differentiation of neurons and neuroendocrine cells, and a high NSE level often indicates cells’ abnormal or aggressive behavior (10). Thus, the NSE level in serum is often used as a biomarker for NB. Elevated NSE values were found in late-stage NB and metastatic NB, and associated with poor prognosis (9,10). In this study, we found that the mean NSE value of non-high-risk NB was 41.45±30.99 ng/mL, which was not significantly (P=0.06) different from that of the high-risk group (93.05±97.14 ng/mL). There was no statistically significant difference between risk groups either (P=0.19). Besides, the AUC value of NSE in differentiating NB in the high-risk group from that of the non-high-risk group was only 0.64. Prior literature also reported that NSE was not a reliable marker for monitoring the recurrence or progression of NB (42). Since NSE is just one of the multiple proteins involved in the cell proliferation of NB, it may not always be accurate in evaluating NB. In contrast, the APT values showed a good diagnostic performance in predicting the risk group of NB (AUC =0.89), which was significantly (P=0.03) superior to serum NSE. This result indicated that, in comparison with the serum NSE level, APT MRI might be more comprehensive and accurate in detecting the over-expressed proteins during the abnormal proliferation of NB cells.
Limitations
There were several limitations in this study. First, due to the restriction of the long acquisition time of APT MRI, the abdominal APT images in this study were acquired with a single-slice sequence. The full information about the tumor could not be obtained, although the monolayer images were successful in NB risk stratification. Second, no respiratory gating was implemented to maintain the correct timing of the APT sequence. Third, it is unclear which specific proteins detected by the APT MRI technique contributed to the risk stratification of NB values, with NSE potentially among the elevated proteins probed. Fourth, the multi-pool Lorentzian fitting approach adopted is not entirely accurate for a saturation power of 2 uT (43), and may not be able to yield a clean APT effect. Last, the sample size of this study was not large enough, and the data were all from a single center.
Conclusions
In summary, APT imaging can stratify the risk group of NB by detecting the difference in protein content of tumors at the molecular level. The diagnostic performance of the APT parameter was superior to that of the serum NSE index, demonstrating a promising prospect for clinical applications in risk stratification of preoperative NB in children.
Acknowledgments
Funding: This study received funding by the National Natural Science Foundation of China (grant No. 81971605, to Y Zhang), Key R&D Program of Zhejiang Province (grant No. 2022C04031, to Y Zhang), Leading Innovation and Entrepreneurship Team of Zhejiang Province (grant No. 2020R01003, to Y Zhang) and MOE Frontier Science Center for Brain Science & Brain-Machine Integration, Zhejiang University (to Y Zhang).
Footnote
Reporting Checklist: The authors have completed the STARD reporting checklist. Available at https://qims.amegroups.com/article/view/10.21037/qims-22-780/rc
Conflicts of Interest: All authors have completed the ICMJE uniform disclosure form (available at https://qims.amegroups.com/article/view/10.21037/qims-22-780/coif). YZ serves as an unpaid editorial board member of Quantitative Imaging in Medicine and Surgery. YZ reports that this study was supported by the National Natural Science Foundation of China (grant No. 81971605), Key R&D Program of Zhejiang Province (grant No. 2022C04031), Leading Innovation and Entrepreneurship Team of Zhejiang Province (grant No. 2020R01003) and MOE Frontier Science Center for Brain Science & Brain-Machine Integration, Zhejiang University. WC is an employee of Philips Healthcare. The other authors have no conflicts of interest to declare.
Ethical Statement: The authors are accountable for all aspects of the work in ensuring that questions related to the accuracy or integrity of any part of the work are appropriately investigated and resolved. This study was conducted following the Declaration of Helsinki (as revised in 2013) and was approved by the Ethics Committee of the Children’s Hospital at Zhejiang University School of Medicine (No. 2019-IRB-048). Written informed consent was obtained from each volunteer’s legal guardian.
Open Access Statement: This is an Open Access article distributed in accordance with the Creative Commons Attribution-NonCommercial-NoDerivs 4.0 International License (CC BY-NC-ND 4.0), which permits the non-commercial replication and distribution of the article with the strict proviso that no changes or edits are made and the original work is properly cited (including links to both the formal publication through the relevant DOI and the license). See: https://creativecommons.org/licenses/by-nc-nd/4.0/.
References
- Maris JM, Hogarty MD, Bagatell R, Cohn SL. Neuroblastoma. Lancet 2007;369:2106-20. [Crossref] [PubMed]
- Park JR, Eggert A, Caron H. Neuroblastoma: biology, prognosis, and treatment. Pediatr Clin North Am 2008;55:97-120. x. [Crossref] [PubMed]
- Kamihara J, Bourdeaut F, Foulkes WD, Molenaar JJ, Mossé YP, Nakagawara A, Parareda A, Scollon SR, Schneider KW, Skalet AH, States LJ, Walsh MF, Diller LR, Brodeur GM. Retinoblastoma and Neuroblastoma Predisposition and Surveillance. Clin Cancer Res 2017;23:e98-e106. [Crossref] [PubMed]
- Maris JM. The biologic basis for neuroblastoma heterogeneity and risk stratification. Curr Opin Pediatr 2005;17:7-13. [Crossref] [PubMed]
- Cohn SL, Pearson AD, London WB, Monclair T, Ambros PF, Brodeur GM, Faldum A, Hero B, Iehara T, Machin D, Mosseri V, Simon T, Garaventa A, Castel V, Matthay KK. INRG Task Force. The International Neuroblastoma Risk Group (INRG) classification system: an INRG Task Force report. J Clin Oncol 2009;27:289-97. [Crossref] [PubMed]
- Sharp SE, Gelfand MJ, Shulkin BL. Pediatrics: diagnosis of neuroblastoma. Semin Nucl Med 2011;41:345-53. [Crossref] [PubMed]
- Swift CC, Eklund MJ, Kraveka JM, Alazraki AL. Updates in Diagnosis, Management, and Treatment of Neuroblastoma. Radiographics 2018;38:566-80. [Crossref] [PubMed]
- Marangos PJ, Schmechel DE. Neuron specific enolase, a clinically useful marker for neurons and neuroendocrine cells. Annu Rev Neurosci 1987;10:269-95. [Crossref] [PubMed]
- Ishiguro Y, Kato K, Ito T, Nagaya M, Yamada N, Sugito T. Nervous system-specific enolase in serum as a marker for neuroblastoma. Pediatrics 1983;72:696-700. [Crossref] [PubMed]
- Zeltzer PM, Marangos PJ, Evans AE, Schneider SL. Serum neuron-specific enolase in children with neuroblastoma. Relationship to stage and disease course. Cancer 1986;57:1230-4. [Crossref] [PubMed]
- Hiorns MP, Owens CM. Radiology of neuroblastoma in children. Eur Radiol 2001;11:2071-81. [Crossref] [PubMed]
- Sarioglu FC, Salman M, Guleryuz H, Ozer E, Cecen E, Ince D, Olgun N. Radiological staging in neuroblastoma: computed tomography or magnetic resonance imaging? Pol J Radiol 2019;84:e46-53. [Crossref] [PubMed]
- Brisse HJ, McCarville MB, Granata C, Krug KB, Wootton-Gorges SL, Kanegawa K, Giammarile F, Schmidt M, Shulkin BL, Matthay KK, Lewington VJ, Sarnacki S, Hero B, Kaneko M, London WB, Pearson AD, Cohn SL, Monclair TInternational Neuroblastoma Risk Group Project. Guidelines for imaging and staging of neuroblastic tumors: consensus report from the International Neuroblastoma Risk Group Project. Radiology 2011;261:243-57. [Crossref] [PubMed]
- Fraum TJ, Ludwig DR, Bashir MR, Fowler KJ. Gadolinium-based contrast agents: A comprehensive risk assessment. J Magn Reson Imaging 2017;46:338-53. [Crossref] [PubMed]
- Zhou J, Payen JF, Wilson DA, Traystman RJ, van Zijl PC. Using the amide proton signals of intracellular proteins and peptides to detect pH effects in MRI. Nat Med 2003;9:1085-90. [Crossref] [PubMed]
- van Zijl PC, Yadav NN. Chemical exchange saturation transfer (CEST): what is in a name and what isn't? Magn Reson Med 2011;65:927-48. [Crossref] [PubMed]
- Zhou J. Zijl PCMv. Chemical exchange saturation transfer imaging and spectroscopy. Prog Nucl Magn Reson Spectrosc 2006;48:109-36. [Crossref]
- Jones CK, Schlosser MJ, van Zijl PC, Pomper MG, Golay X, Zhou J. Amide proton transfer imaging of human brain tumors at 3T. Magn Reson Med 2006;56:585-92. [Crossref] [PubMed]
- Wen Z, Hu S, Huang F, Wang X, Guo L, Quan X, Wang S, Zhou J. MR imaging of high-grade brain tumors using endogenous protein and peptide-based contrast. Neuroimage 2010;51:616-22. [Crossref] [PubMed]
- Zhang H, Yong X, Ma X, Zhao J, Shen Z, Chen X, Tian F, Chen W, Wu D, Zhang Y. Differentiation of low- and high-grade pediatric gliomas with amide proton transfer imaging: added value beyond quantitative relaxation times. Eur Radiol 2021;31:9110-9. [Crossref] [PubMed]
- Nishie A, Takayama Y, Asayama Y, Ishigami K, Ushijima Y, Okamoto D, Fujita N, Tsurumaru D, Togao O, Manabe T, Oki E, Kubo Y, Hida T, Hirahashi-Fujiwara M, Keupp J, Honda H. Amide proton transfer imaging can predict tumor grade in rectal cancer. Magn Reson Imaging 2018;51:96-103. [Crossref] [PubMed]
- Foo LS, Harston G, Mehndiratta A, Yap WS, Hum YC, Lai KW, Mohamed Mukari SA, Mohd Zaki F, Tee YK. Clinical translation of amide proton transfer (APT) MRI for ischemic stroke: a systematic review (2003-2020). Quant Imaging Med Surg 2021;11:3797-811. [Crossref] [PubMed]
- Lin Y, Luo X, Yu L, Zhang Y, Zhou J, Jiang Y, Zhang C, Zhang J, Li C, Chen M. Amide proton transfer-weighted MRI for predicting histological grade of hepatocellular carcinoma: comparison with diffusion-weighted imaging. Quant Imaging Med Surg 2019;9:1641-51. [Crossref] [PubMed]
- Jia X, Wang W, Liang J, Ma X, Chen W, Wu D, Lai C, Zhang Y. Risk stratification of abdominal tumors in children with amide proton transfer imaging. Eur Radiol 2022;32:2158-67. [Crossref] [PubMed]
- Liu R, Zhang H, Niu W, Lai C, Ding Q, Chen W, Liang S, Zhou J, Wu D, Zhang Y. Improved chemical exchange saturation transfer imaging with real-time frequency drift correction. Magn Reson Med 2019;81:2915-23. [Crossref] [PubMed]
- Liu R, Zhang H, Qian Y, Hsu YC, Fu C, Sun Y, Wu D, Zhang Y. Frequency-stabilized chemical exchange saturation transfer imaging with real-time free-induction-decay readout. Magn Reson Med 2021;85:1322-34. [Crossref] [PubMed]
- Zhang Y, Zu T, Liu R, Zhou J. Acquisition sequences and reconstruction methods for fast chemical exchange saturation transfer imaging. NMR Biomed 2022; Epub ahead of print. [Crossref] [PubMed]
- Zhou J, Zaiss M, Knutsson L, Sun PZ, Ahn SS, Aime S, et al. Review and consensus recommendations on clinical APT-weighted imaging approaches at 3T: Application to brain tumors. Magn Reson Med 2022;88:546-74. [Crossref] [PubMed]
- Heo HY, Zhang Y, Jiang S, Lee DH, Zhou J. Quantitative assessment of amide proton transfer (APT) and nuclear overhauser enhancement (NOE) imaging with extrapolated semisolid magnetization transfer reference (EMR) signals: II. Comparison of three EMR models and application to human brain glioma at 3 Tesla. Magn Reson Med 2016;75:1630-9. [Crossref] [PubMed]
- In den Kleef JJ, Cuppen JJ. RLSQ: T1, T2, and rho calculations, combining ratios and least squares. Magn Reson Med 1987;5:513-24. [Crossref] [PubMed]
- Zhang Y, Heo HY, Lee DH, Zhao X, Jiang S, Zhang K, Li H, Zhou J. Selecting the reference image for registration of CEST series. J Magn Reson Imaging 2016;43:756-61. [Crossref] [PubMed]
- Jenkinson M, Bannister P, Brady M, Smith S. Improved optimization for the robust and accurate linear registration and motion correction of brain images. Neuroimage 2002;17:825-41. [Crossref] [PubMed]
- Kim M, Gillen J, Landman BA, Zhou J, van Zijl PC. Water saturation shift referencing (WASSR) for chemical exchange saturation transfer (CEST) experiments. Magn Reson Med 2009;61:1441-50. [Crossref] [PubMed]
- Zaiss M, Schmitt B, Bachert P. Quantitative separation of CEST effect from magnetization transfer and spillover effects by Lorentzian-line-fit analysis of z-spectra. J Magn Reson 2011;211:149-55. [Crossref] [PubMed]
- Law BKH, King AD, Ai QY, Poon DMC, Chen W, Bhatia KS, Ahuja AT, Ma BB, Ka-Wai Yeung D, Fai Mo FK, Wang YX, Yuan J. Head and Neck Tumors: Amide Proton Transfer MRI. Radiology 2018;288:782-90. [Crossref] [PubMed]
- Togao O, Yoshiura T, Keupp J, Hiwatashi A, Yamashita K, Kikuchi K, Suzuki Y, Suzuki SO, Iwaki T, Hata N, Mizoguchi M, Yoshimoto K, Sagiyama K, Takahashi M, Honda H. Amide proton transfer imaging of adult diffuse gliomas: correlation with histopathological grades. Neuro Oncol 2014;16:441-8. [Crossref] [PubMed]
- Jiang S, Eberhart CG, Lim M, Heo HY, Zhang Y, Blair L, Wen Z, Holdhoff M, Lin D, Huang P, Qin H, Quinones-Hinojosa A, Weingart JD, Barker PB, Pomper MG, Laterra J, van Zijl PCM, Blakeley JO, Zhou J. Identifying Recurrent Malignant Glioma after Treatment Using Amide Proton Transfer-Weighted MR Imaging: A Validation Study with Image-Guided Stereotactic Biopsy. Clin Cancer Res 2019;25:552-61. [Crossref] [PubMed]
- Shimada H, Chatten J, Newton WA Jr, Sachs N, Hamoudi AB, Chiba T, Marsden HB, Misugi K. Histopathologic prognostic factors in neuroblastic tumors: definition of subtypes of ganglioneuroblastoma and an age-linked classification of neuroblastomas. J Natl Cancer Inst 1984;73:405-16. [Crossref] [PubMed]
- Liu CJ, Lu MY, Liu YL, Ko CL, Ko KY, Tzen KY, Chang HH, Yang YL, Jou ST, Hsu WM, Yen RF. Risk Stratification of Pediatric Patients With Neuroblastoma Using Volumetric Parameters of 18F-FDG and 18F-DOPA PET/CT. Clin Nucl Med 2017;42:e142-8. [Crossref] [PubMed]
- Yan K, Fu Z, Yang C, Zhang K, Jiang S, Lee DH, Heo HY, Zhang Y, Cole RN, Van Eyk JE, Zhou J. Assessing Amide Proton Transfer (APT) MRI Contrast Origins in 9 L Gliosarcoma in the Rat Brain Using Proteomic Analysis. Mol Imaging Biol 2015;17:479-87. [Crossref] [PubMed]
- Yu F, Zhu X, Feng C, Wang T, Hong Q, Liu Z, Tang S. Proteomics-based identification of spontaneous regression-associated proteins in neuroblastoma. J Pediatr Surg 2011;46:1948-55. [Crossref] [PubMed]
- Simon T, Hero B, Hunneman DH, Berthold F. Tumour markers are poor predictors for relapse or progression in neuroblastoma. Eur J Cancer 2003;39:1899-903. [Crossref] [PubMed]
- Ji Y, Zhou IY, Qiu B, Sun PZ. Progress toward quantitative in vivo chemical exchange saturation transfer (CEST) MRI. Isr J Chem 2017;57:809-24. [Crossref]