Deep learning for fully automated segmentation and volumetry of Couinaud liver segments and future liver remnants shown with CT before major hepatectomy: a validation study of a predictive model
Introduction
Post-resectional liver failure (PLF) is highly correlated with postoperative mortality and is regarded as one of the most severe complications of major hepatectomy (1,2). The future liver remnant (FLR) is the volume of the liver that will remain after the hepatectomy and has been accepted as the most important predictor of PLF (3,4). Existing automated methods for FLR assessment are accurate but rely on specialized software and manual “virtual cuts” by surgeons (1).
Several studies have reported the automated segmentation of Couinaud segments based on deep learning (DL) algorithms; however, these studies aimed to develop a new model and focused on technical feasibility and efficiency (5-8). Variations in attenuation and morphology of the liver (i.e., the presence of hepatic steatosis, cirrhosis, and hepatic tumors) may affect the performance of the DL models in real clinical practice. How these models perform in diverse liver conditions has not been investigated in these studies. This is the major concern of surgeons and radiologists in clinical practice.
Previous studies also reported the automated segmentation of the FLR for liver resection based on DL algorithms. However, the validations were inadequate and were only processed on a very small public data set (12 cases) (6) or were processed on clinical cases but lacked detailed quantitative and qualitative evaluation (7). The differences in the preoperative assessment of the FLR and the evaluation of resectability made by DL models and human doctors have not been discussed and remain unknown.
For a DL model to be applicable in the real workflow of surgical planning, fully external validation using clinical cases is mandatory, as spectrum bias and overfitting are unavoidable and lead to overestimating the accuracy of the DL model (9). The external validation data set in our study was extracted from medical imaging center of Peking University Shenzhen Hospital with a relatively large number of clinical cases (n=178) to minimize spectrum bias and overfitting. For robust verification, cases with variable morphology and attenuation of the liver (i.e., test data set 1, consisting of cases with normal liver, hepatic steatosis, or cirrhosis with or without hepatic lesions) and candidates for major hepatectomy (i.e., test data set 2) were included in the external validation cohort.
Our study aimed to develop and validate a DL model for the automatic assessment of the FLR in an external validation cohort to evaluate the feasibility of major hepatectomy. Our model can be used for the preoperative evaluation of major hepatectomy. In this clinical scenario, our model can provide an automated FLR assessment both in a quantitative output and as a 3-dimensional (3D) visualization for surgeons. We present the following article in accordance with the TRIPOD reporting checklist (available at https://qims.amegroups.com/article/view/10.21037/qims-22-1008/rc).
Methods
The retrospective study was conducted in accordance with the Declaration of Helsinki (as revised in 2013) and approved by the institutional review board (IRB) of Peking University First Hospital and Peking University Shenzhen Hospital [IRB No. 2019 (169), 2021 (071), and 2021 (071)-1]. The informed consent waiver for the computed tomography (CT) images used was granted.
Patient data
The training data set consisted of 170 portovenous phase (PVP) abdominal CT images from 170 patients and was derived from 2,283 patients who underwent abdominal contrast-enhanced CT scans for any reason between January 2018 and March 2019 at medical center A (Peking University First Hospital). Test data set 1 consisted of 146 PVP hepatic CT images from 146 patients, and test data set 2 consisted of 29 PVP hepatic CT images and 3 delayed phase CT images from 32 patients. The test data sets were derived from 1,774 patients who underwent abdominal contrast-enhanced CT scans between June 2019 and December 2021 at medical center B (Peking University Shenzhen Hospital). There was no difference between the modeled and validated data sets in terms of the study setting, the inclusion of study participants, or the outcome metrics.
The sample size (170 for the training data set and 178 for the external test data set) was the largest amount of data eligible for the study that could be obtained before we started our study. In the data collection, 26 cases with incomplete information were excluded (accounting for 0.75% of the total amount), including 15 cases from medical center A and 11 cases from medical center B. These cases were excluded because of missing portal venous phase CT data. A flowchart is presented in Figure 1.
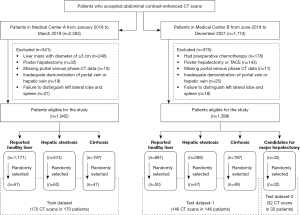
To enhance the robustness of the model, patients with various liver conditions, as confirmed by CT, were included in the training data set and test data set 1. Liver conditions included a healthy liver, steatosis (including nonalcoholic fatty liver disease and hepatic steatosis secondary to extensive chemotherapy), and cirrhosis (cirrhosis in chronic hepatitis B and C and alcohol-associated cirrhosis). The training data set included patients with hepatic lesions (i.e., hepatic cyst, hemangioma, focal nodular hyperplasia, and hepatocellular carcinoma no larger than 3 cm in diameter). Test data set 2 included candidates for major hepatectomy with hepatic lesions of hepatocellular carcinoma, cholangiocarcinoma, and metastatic adenocarcinoma. The demographic characteristics of the 3 data sets are shown in Table 1.
Table 1
Parameter | Training data set | Test data set 1 | Test data set 2 |
---|---|---|---|
Patients | 170 | 146 | 32 |
Female patients, n (%) | 64 (37.65) | 62 (42.47) | 7 (21.88) |
Mean age (years), mean ± SD | 50.23±13.77 | 49.04±13.15 | 54.59±14.29 |
Liver conditions, n (%) | |||
Reported healthy livers | 61 (35.88) | 50 (34.25) | 27 (84.38) |
Steatosis | 62 (36.47) | 47 (32.19) | 3 (9.38) |
Cirrhosis | 47 (27.65) | 49 (33.56) | 2 (6.25) |
Focal hepatic lesion, n (%) | |||
No lesion | 103 (60.59) | 55 (31.61) | NA |
Hepatic cyst | 51 (30.00) | 72 (41.38) | 0 |
Benign lesion | 29 (17.06) | 43 (24.71) | 9 (25.00) |
Malignant tumor | 4 (2.35) | 14 (8.05) | 29 (75.00) |
Total volume of all hepatic lesions (mL), mean ± SD (range) | 2.02±4.13 (0.00–49.40) | 1.87±7.92 (0.00–86.05) | 448.80±608.60 (9.14–2426.10) |
Imaging system, n | |||
GE Light Speed VCT | 39 | NA | NA |
GE Discovery CT750 HD | 34 | NA | NA |
GE revolution | NA | 93 | 14 |
Philips Brilliance iCT 256 | 72 | NA | NA |
Siemens definition flash | 25 | 53 | 18 |
Imaging parameters | |||
Section thickness (mm) | 1.0 | 1.0/1.25 | 1.0/1.25 |
SD, standard deviation; NA, not applicable.
CT imaging
The images were acquired with CT scanners from 3 different manufacturers: GE Healthcare, Siemens Healthineers, and Philips. Iodinated contrast agents were used in amounts ranging from 80 to 140 mL. The thicknesses of the CT images were 1.0 and 1.25 mm.
As portal veins and hepatic veins formed the outer frame of Couinaud liver segments, only CT images with the clearest visualization of both the portal veins and hepatic veins were included (a total of 345 scans were acquired at PVP, and 3 scans of cirrhosis cases were acquired at the delayed phase in test data set 2) to obtain better segmentation.
Imaging processing and labeling
The images were processed with ITK-SNAP version 3.8.0. The images were labeled according to Couinaud liver segments by a hepatic radiologist (with 8 years of experience) in consensus with a radiologist with 20 years’ experience in abdomen radiology. The latter radiologist performed a quality check and adjusted the annotations of the former. The process was performed at all stages of development and validation of the DL models. Manual segmentation was regarded as the ground truth for segmentation and volumetry. For all data sets, the inferior vena cava and the main trunk of the portal vein were excluded from the labeling, and vessels enclosed by the hepatic parenchyma were included.
For all data sets, the first step was liver segmentation, followed by the segmentation of the hepatic lesions and Couinaud liver segments, and finally prediction of the FLR. The key steps in this process are demonstrated in Figure 2.
Model development
We used the 3D U-Net network described by Çiçek et al. (10) for the segmentation of Couinaud liver segments. Three cascaded 3D U-Net frameworks were trained. The first 3D U-Net framework was trained for liver segmentation with an average dice similarity coefficient (DSC) of 0.98 and a volumetric similarity (VS) of 0.99. The next model was trained for the Couinaud segmentation of liver segments based on liver segmentation. The third was trained for the segmentation of hepatic lesions with a detection rate of 100%, an average DSC of 0.69, and a VS of 0.78.
The input of the network was a 3D CT image with manual annotation of 8 Couinaud liver segments. The output was the same shape as the input image and with the predicted annotation of the 8 Couinaud liver segments. The Dice loss was used as the loss function in the segmentation task. During the training of our model, we checked the prediction accuracy of the model on a validation data set. We kept track of the prediction accuracy on the validation data set, and as soon as the validation accuracy started to decrease, we stopped our training to prevent overfitting. The image resolution was set as 128×192×256. The window width and window level were set as 300 and 30 HU, respectively. Image amplification methods, such as random noise, translation, and affine transform, were used. The adaptive movement estimation algorithm (ADAM) gradient descent optimization algorithm was used, the initial learning rate was 1×10−4, the batch size was set as 2, and the number of epochs was 400. The programming language used in the study was Python. The hardware for model training was a GPU NVIDIA Tesla P100 16 G, and the software included Python3.6, PyTorch 0.4.1, Opencv, Numpy, and SimpleITK.
Model evaluation and qualitative assessment
To evaluate the performance of the segmentation model, the DSC was calculated. DSC is defined as the voxel overlap between the prediction (X) and the ground truth (Y) and is calculated as follows:
To test the clinical usefulness (the consistency between the model and human doctors on the evaluation of a major hepatectomy based on FLR%) of our DL model, we performed a qualitative analysis using the following formula:
VTotalLiver and VHepaticLesion represent the volume of the entire liver, including hepatic lesions and the volume of hepatic lesions within the liver that are planned to be removed, respectively (11).
The differences in FLR and FLR% between the model and human doctors were analyzed. For test data set 1, the virtual FLR and virtual FLR% assessment for 4 types of a virtual major hepatectomy (1, complete right hepatectomy; 2, extended right hepatectomy; 3, complete left hepatectomy; and 4, extended left hepatectomy) were calculated for each case. For test data set 2, the FLR and FLR% assessment were calculated according to the actual resection procedures recorded in the operation notes.
To define the consistency, we used 2 criteria: (I) the prediction of resectability for a major hepatectomy was consistent between the model and the human doctor according to the estimated FLR%, and (II) the absolute difference of the FLR% between predictions and manually labeled masks was less than 5%. If the difference in the FLR% between the model and the human doctor was within 5%, the evaluation of resectability was considered consistent.
The FLR% cutoffs of 20%, 30%, and 40% in patients with healthy livers, hepatic steatosis, and cirrhosis, respectively, were accepted as the minimum FLR% values for major hepatectomy in this study (12-14).
Statistical analysis and evaluation
The Shapiro-Wilk test was performed to test the normality of distributions. Continuous variables are expressed as the mean ± standard deviation or as the median and interquartile range depending on the normality of the data. Categorical variables are expressed as frequencies and percentages. The Levene test was performed to analyze the homogeneity of variance. Significance was defined as a P value less than 0.05 (2-tailed). We used commercially available software (GraphPad Prism v7.00, GraphPad Software; SPSS for Mac, version 22.0, IBM Corp.) to perform the statistical analyses.
To quantitatively evaluate the accuracy of segmentation, the DSC values between the predictions and the manually labeled Couinaud segmentation were computed. The differences in DSC among those with healthy livers, steatosis, or cirrhosis, and candidates for major hepatectomy were tested using the Kruskal-Wallis test. The differences between subgroups were tested using the Mann-Whitney test with Bonferroni correction. To evaluate the accuracy of volumetry, the FLR, FLR%, and absolute difference of the FLR% between manual and automated segmentation were compared using the Mann-Whitney test and Bland-Altman analysis. To test the clinical utility, the evaluation of resectability between the model and the human doctor was compared using the McNemar test.
Results
Patients and image characteristics
The test data set consisted of 178 patients who underwent abdominal contrast-enhanced CT scans at medical center B. Test data set 1 consisted of 146 cases with an average age of 49.04±13.15 years, while test data set 2 consisted of 32 cases with an average age of 54.59±14.29 years. The demographic characteristics of each data set are shown in Table 1.
Automated segmentation results
Our method was capable of accurately segmenting 8 functional Couinaud liver segments and the FLR in various liver conditions both in test data set 1 and test data set 2. The automated segmentation results are shown in Figure 3. The differences in the segmentation results between automated and manual segmentation are shown in Figure 4.
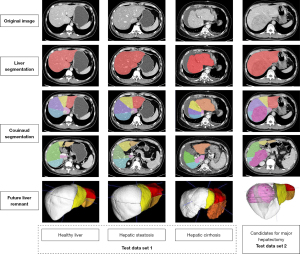
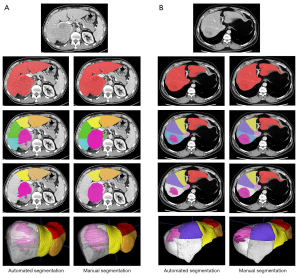
Segmentation accuracy of Couinaud liver segments
The DSC for the segmentation of each Couinaud liver segment ranged from 0.93±0.01 (95% CI: 0.92–0.94) to 0.95±0.00 (95% CI: 0.94–0.96) in test data sets 1 and 2 (Table S1, Figure 5). The DSC differences between those with healthy livers, those with hepatic steatosis, and candidates for major hepatectomy were statistically significant (P<0.001); however, the differences between those with healthy livers and those with cirrhosis, between those with healthy livers and candidates for a major hepatectomy, and between those with livers with cirrhosis and candidates for a major hepatectomy were not significant (P=0.86, P=0.32, and P=0.15, respectively; Figure 6). Segment VIII and segment I provided the highest and lowest DSC values in the test data set, respectively. Compared with the methods of Jia et al. (5) and Han et al. (8), ours could obtain higher DSC values. The results are shown in Table 2.
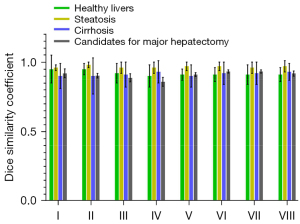
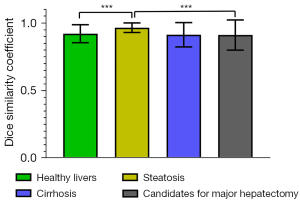
Table 2
Methods | Number of patients | Images | Segment | Mean | |||||||
---|---|---|---|---|---|---|---|---|---|---|---|
I | II | III | IV | V | VI | VII | VIII | ||||
Jia et al. | 59 | MR | 0.805 | 0.838 | 0.868 | 0.907 | 0.924 | 0.915 | 0.916 | 0.872 | 0.890 |
Han et al. | 100 | MR | 0.932 | 0.922 | 0.910 | 0.922 | 0.881 | 0.875 | 0.893 | 0.881 | 0.902 |
Our method | 178 | CT | 0.931 | 0.939 | 0.935 | 0.933 | 0.938 | 0.948 | 0.947 | 0.949 | 0.940 |
DSC, dice similarity coefficient.
Volumetric accuracy for Couinaud liver segments, FLR, and FLR% of test data set 1+2
The volume of each Couinaud liver segment obtained with manual and automated segmentation is shown in Table S1. The automated FLR and FLR% assessments ranged from 77.77 to 1,746.13 mL (mean volume 493.51±284.77 mL) and 6.47% to 87.52% (mean value 38.53%±19.38%) in test data set 1 and test data set 2, respectively. The manual FLR and FLR% assessments ranged from 74.11 to 1,753.28 mL (mean volume 500.92±284.38 mL) and 6.08% to 89.67% (mean value 38.35%±19.14%) in test data set 1 and test data set 2, respectively. The FLR and FLR% assessments for subgroups are shown in Figures 7,8 and Table 3. No significant differences in volumetry of the Couinaud liver segment, FLR, or FLR% assessments were noted between the automated and manual values according to the Mann-Whitney test (P=0.70, P=0.50, P=0.82, respectively) for test data sets 1 and 2.
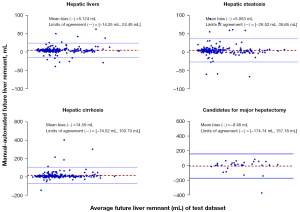
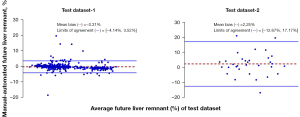
Table 3
Volumetry | Test data set 1 | Test data set 2 | |||
---|---|---|---|---|---|
Type 1: complete right hepatectomy | Type 2: extended right hepatectomy | Type 3: complete left hepatectomy | Type 4: extended left hepatectomy | ||
FLR (M) | 463.46±150.51 (438.84, 488.08) | 270.80±102.09 (254.10, 287.50) | 869.71±243.53 (829.87, 909.54) | 350.40±106.15 (333.03, 367.76) | 725.99±253.09 (634.74, 817.24) |
FLR (A) | 454.12±143.78 (430.60, 477.64) | 258.73±92.77 (243.55, 273.90) | 864.18±243.75 (824.31, 904.05) | 344.21±98.88 (328.03, 360.38) | 734.47±263.08 (639.62, 829.32) |
FLR% (M) | 35.27±7.28 (34.08, 36.46) | 20.81±6.59 (19.73, 21.89) | 65.90±6.97 (64.76, 67.04) | 26.85±6.17 (25.84, 27.86) | 59.19±16.56 (53.22, 65.16) |
FLR% (A) | 35.47±7.16 (34.30, 36.64) | 20.44±6.33 (19.41, 21.48) | 67.12±6.85 (66.00, 68.24) | 27.04±5.42 (26.15, 27.92) | 56.94±16.24 (51.08, 62.80) |
The absolute difference of the FLR% | 0.82±1.23 (0.62, 1.02) | 0.52±1.75 (0.24, 0.81) | 1.45±0.96 (1.29, 1.61) | 1.19±2.41 (0.79, 1.58) | 5.65±5.49 (3.67, 7.63) |
Data are expressed as the mean ± standard deviation, and data in parentheses are the 95% CI. FLR, future liver remnant; FLR% (M), FLR% obtained by manual segmentation; FLR% (A), FLR% obtained by automated segmentation; the absolute difference of the FLR%, the absolute difference of the FLR% between manual and automated segmentation.
Spearman correlation analysis for the FLR assessment showed that the automated measurements strongly correlated with the manual measurements in test data sets 1 and 2 (r=0.99; R2=0.99; slope =0.99; intercept =11.59). For the FLR% assessment, the automated measurements strongly correlated with the manual measurements (r=0.99; R2=0.98; slope =0.98; intercept =0.65) in test data sets 1 and 2. The FLR% assessments obtained using the automated methods slightly overestimated the manual measurements in test data set 1 [bias =−0.31%; P<0.05; 95% limits of agreement (LoA): −4.14% and 3.52%] but slightly underestimated the manual measurements in test data set 2 (bias =2.25%; P<0.05; 95% LoA: −12.67% and 17.17%; Figure 8). The FLR assessments obtained using the automated methods slightly underestimated the manual measurements in test data set 1 (bias =8.28 mL; P<0.01; 95% LoA: −48.12 and 64.68 mL) but slightly overestimated the manual measurements in test data set 2 (bias =–8.48 mL; P<0.05; 95% LoA: −174.14 and 157.18 mL; Figure 7).
For volumetric assessment, our results were compared with similar studies at the lobe level (Figure 9). We obtained results similar to those of Huang et al. (15), Ruskó et al. (16), Butdee et al. (17), and Le et al. (6), with a difference of less than 5%. However, our results were quite different from those of Chen et al. (18), with a difference of 9% in volumetry at the lobe level. However, no detailed information on the test data set was provided in Chen et al.’s study (i.e., how many cases, the liver conditions included, where the data set was extracted); therefore, the reason for the relatively large difference in volumetry was not analyzed.
Qualitative analysis results
The absolute difference of the FLR%
The absolute difference of the FLR% obtained by automatic and manual segmentation ranged from 0% to 18.62% (mean value 0.99%±1.64%) in test data set 1 and 0.02% to 21.01% (mean value 5.65%±5.49%) in test data set 2, with an average of 1.23%±2.27% in test data sets 1 and 2. The Mann-Whitney test suggested that there was a significant difference in the absolute difference of the FLR% between test data set 1 and test data set 2 (P<0.0001; U=2,839). Figure 10 demonstrates that the absolute difference of the FLR% in test data set 2 (median value 4.34±5.49) was larger than that in test data set 1 (median value 0.54±1.64). The results were within 5% for 96.27% of all cases (573/584 in test data set 1, 20/32 in test data set 2).
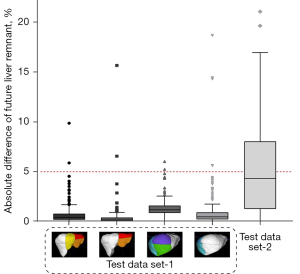
Comparison of the prediction of resectability in test data sets 1 and 2 on criteria (a)
A total of 1,232 (146×4×2 + 32×2) FLR% assessment results were compared. The number of cases categorized as candidates for major hepatectomy is shown in Table 4. For test data set 1, 106, 23, 146, and 57 cases were categorized with automated segmentation of the FLR% as candidates for types 1–4 virtual major hepatectomy, respectively; meanwhile, manual segmentation of the FLR% categorized 107, 23, 146, and 57 cases for types 1–4, respectively. The McNemar test suggested no significant differences between the automatic segmentation model and human doctors in the prediction of resectability based on FLR% assessments (P>0.99; McNemar test statistic 0.00) in test data set 1. However, 6 cases (1.03%) were categorized as candidates according to manual segmentation but were rejected for resection according to automated segmentation, and 7 cases (1.20%) were rejected for resection according to manual segmentation but were categorized as candidates according to automated segmentation.
Table 4
Methods | Test data set 1 (n=146) | Test data set 2 (n=32) | |||
---|---|---|---|---|---|
Type 1: complete right hepatectomy | Type 2: extended right hepatectomy | Type 3: complete left hepatectomy | Type 4: extended left hepatectomy | ||
Manual segmentation | 107 | 23 | 146 | 57 | 32 |
Automated segmentation | 106 | 23 | 146 | 57 | 32 |
In test data set 2, both manual or automated segmentation categorized all cases as candidates for major hepatectomy.
Discussion
Several DL models have been developed for the automated segmentation of Couinaud liver segments and preoperative volumetric assessment (5-8). However, these studies mainly concerned technical feasibility. The performance of these models has not been fully evaluated using clinical cases and various liver conditions. How well these models perform in clinical practice, particularly in patients with pathological livers and prior to major hepatectomy, remains unknown. This is a major concern for surgeons and radiologists in clinical practice.
In this study, we developed a DL model to segment Couinaud liver segments and FLR and applied this technique for preoperative FLR% assessment. The key advantages of this study were that the segmentation performance was validated using clinical cases with various liver conditions, including those with healthy livers, hepatic steatosis, or cirrhosis (Figure 3) as well as candidates for major hepatectomy with large hepatic lesions including hepatocellular carcinoma, cholangiocarcinoma, or hemangioma (Figure 11); moreover, we used a relatively large amount of CT data (178 cases) and conducted a full comparison with human doctors to produce quantitative and qualitative results. These are the main distinguishing features of our study compared to those previous, as we believe it is essential to investigate DL models before they can be used in future prospective studies in a clinical setting.
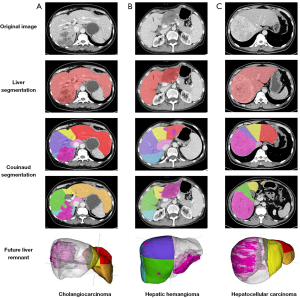
The results of our study suggest that our model allowed for accurate, fully automatic segmentation of Couinaud segments and volumetry of the FLR% on CT images and is robust in various liver conditions. The effectiveness of the DL model was validated using clinical cases in another medical center and in candidates for major hepatectomy. Our model has the potential to be used to assist surgical planning by providing FLR% assessment automatically.
The performance of our DL model was compared with that of Jia et al. (5) and Han et al. (8) at the functional segment level. The higher DSC values yielded by our model demonstrated its high consistency with human doctors. The performance was also compared among various liver conditions. Similar segmentation performances were obtained in cases with hepatic steatosis and cirrhosis and in candidates for major hepatectomy compared with healthy livers, indicating the robustness and generalizability of our model to different clinical settings (shown in Figure 5 and Table S1).
The qualitative analysis included 2 criteria to evaluate the difference between the model and manual segmentation. To classify consistency and inconsistency quantitatively, the threshold was defined as 5% in the absolute difference of the FLR% between the model and manual segmentation. FLR% assessment differences between 2 human doctors or between model and manual segmentation have rarely been reported. Therefore, there is a lack of a reference standard for setting the threshold. Marinelli et al. (19) defined an absolute percentage of liver volume difference between model and manual segmentation of less than 10% as successful segmentation; however, this study focused on the volumetry of the whole liver. For the volumetry of the FLR, a threshold of lower than 10% would be more appropriate. Therefore, we defined an absolute difference in the FLR% assessment of less than 5% as successfully classifying resectability.
The absolute difference in the FLR% in test data set 2 was larger than that in test data set 1 (Figure 9). According to the computing method of the FLR%, the difference in volumetry of the FLR, total liver, and hepatic lesion between the evaluation made by the model and human doctor contributed to the absolute difference of the FLR%. The higher DSC values of the liver segmentation model (mean DSC, 0.98) and the Couinaud segmentation model (average DSC, of 0.94) indicate that the differences in the volumetry of the total liver and FLR between the model and human doctors are minimal. The worse performance of the hepatic lesion model (mean DSC, 0.69; VS, 0.78) mainly contributed to the difference in the FLR% between the model and human doctors. This factor affected the absolute difference in the FLR% in test data set 2 more than in test data set 1 because of the larger hepatic lesions present in test data set 2 (mean total hepatic lesion volume of 448.80±608.60 mL in test data set 2 vs. 1.87±7.92 mL in test data set 1).
For the calculation of the FLR%, we used the ratio of the FLR to the total functional liver volume but not the ratio of the FLR to the standard liver volume (SLV) [SLV = (1,267.28 × body surface area) − 794.41]. However, there is controversy concerning which calculation method best reflects the total liver function (11). FLR is not the only predictor of PLF. PLF is now recognized as having multifactorial causation, and the predictors include patient-dependent factors and surgery-dependent factors (13). These patient-dependent factors include preoperative bilirubin, international normalized ratio, and creatinine (14). In addition, the presence of malnutrition, diabetes mellitus, obesity, and liver damage due to chemotherapy is also associated with an increased risk of PLF (12). Surgery-dependent risk factors include intraoperative blood loss of more than 1,000–1,200 mL, the extent of surgery (i.e., minor hepatectomy, major hepatectomy, or extra major hepatectomy), an FLR <20%, and a prolonged operative time of more than 240 min (14). Among all these factors, the FLR (including the FLR volume and function) is considered one of most important predictors of PLF.
For the preoperative assessment of FLRs, the indocyanine green retention rate at 15 min (ICG R-15) is regarded as a reference standard. However, it provides functional information on the whole liver rather than FLRs (20). Gd-EOB-DTPA (gadolinium ethoxybenzyl-diethylenetriaminepentaacetic acid)-enhanced MRI, a functional imaging technique, can simultaneously evaluate function and remnant volume and is regarded as a promising indicator of FLRs (21). The volumetry of the FLR does not exactly reflect the function of the FLR, especially in pathological livers. However, volumetry is basically a surrogate method for functional assessment, and inadequate volume of the FLR remains an absolute contraindication of major hepatectomy (12,22,23). Volumetry on CT images is highly accessible and robust, and has a short acquisition time; therefore, volumetry of the FLR on CT images plays a major role in the preoperative assessment of major hepatectomy. Manual segmentation of the remnant liver on CT images should be regarded as the final gold standard in the evaluation of the FLR in future prospective studies using DL.
Some limitations to our study should be noted. First, livers with severe morphological anomalies, such as posthepatectomy, were excluded. A more robust model is needed for the subsegmentation of such livers. Second, similar to previous studies focusing on the preoperative assessment of the FLR% (1,24,25), our study did not exclude large hepatic vessels, which may cause the estimated value from the CT volumetric analysis to deviate from the real volumetry. Integrating liver vascular subsegmentation based on this model should be performed in future studies. Third, the unsatisfactory performance of the hepatic lesion model decreased the accuracy of the automatic preoperative FLR% assessment. A more robust model is needed for the segmentation of hepatic lesions.
Conclusions
In conclusion, fully automated segmentation and volumetry are feasible for preoperative FLR assessment of major hepatectomy, even in various liver conditions and for different clinical settings. The DL model demonstrated comparable performance to that of a human doctor in the final evaluation of resectability prior to major hepatectomy in the external validation cohort. Future prospective studies should be performed to test the reliability of the model. Further studies are needed to investigate what effect the model has on decreasing the incidence of PLF and how this model can affect the workflow of a surgeon in surgical planning.
Acknowledgments
Funding: This study was supported by the Research Foundation of Peking University Shenzhen Hospital (No. JCYJ2020007). The funder had no role in the study design, data collection, data analysis, data interpretation, or writing of the report. The corresponding authors had full access to all the data in the study.
Footnote
Reporting Checklist: The authors have completed the TRIPOD reporting checklist (available at https://qims.amegroups.com/article/view/10.21037/qims-22-1008/rc).
Conflicts of Interest: All authors have completed the ICMJE uniform disclosure form (available at https://qims.amegroups.com/article/view/10.21037/qims-22-1008/coif). YZ and DZ are employees of Beijing Smart Tree Medical Technology Co., Ltd. The other authors have no conflicts of interest to declare.
Ethical Statement: The authors are accountable for all aspects of the work in ensuring that questions related to the accuracy or integrity of any part of the work are appropriately investigated and resolved. The retrospective study was conducted in accordance with the Declaration of Helsinki (as revised in 2013) and was approved by the IRB of Peking University First Hospital and Peking University Shenzhen Hospital [IRB No. 2019 (169), 2021 (071), and 2021 (071)-1]. The informed consent waiver for CT images used was granted.
Open Access Statement: This is an Open Access article distributed in accordance with the Creative Commons Attribution-NonCommercial-NoDerivs 4.0 International License (CC BY-NC-ND 4.0), which permits the non-commercial replication and distribution of the article with the strict proviso that no changes or edits are made and the original work is properly cited (including links to both the formal publication through the relevant DOI and the license). See: https://creativecommons.org/licenses/by-nc-nd/4.0/.
References
- Lodewick TM, Arnoldussen CW, Lahaye MJ, van Mierlo KM, Neumann UP, Beets-Tan RG, Dejong CH, van Dam RM. Fast and accurate liver volumetry prior to hepatectomy. HPB (Oxford) 2016;18:764-72. [Crossref] [PubMed]
- Zhang T, Li Q, Wei Y, Yao S, Yuan Y, Deng L, Wu D, Nie L, Wei X, Tang H, Song B. Preoperative evaluation of liver regeneration following hepatectomy in hepatocellular carcinoma using magnetic resonance elastography. Quant Imaging Med Surg 2022;12:5433-51. [Crossref] [PubMed]
- Gotra A, Sivakumaran L, Chartrand G, Vu KN, Vandenbroucke-Menu F, Kauffmann C, Kadoury S, Gallix B, de Guise JA, Tang A. Liver segmentation: indications, techniques and future directions. Insights Imaging 2017;8:377-92. [Crossref] [PubMed]
- Dello SA, Stoot JH, van Stiphout RS, Bloemen JG, Wigmore SJ, Dejong CH, van Dam RM. Prospective volumetric assessment of the liver on a personal computer by nonradiologists prior to partial hepatectomy. World J Surg 2011;35:386-92. [Crossref] [PubMed]
- Jia X, Qian C, Yang Z, Xu H, Han X, Ren H, Wu X, Ma B, Yang D, Min H. Boundary-Aware Dual Attention Guided Liver Segment Segmentation Model. KSII Transactions on Internet and Information Systems 2022;16:16-37.
- Le DC, Chansangrat J, Keeratibharat N, Horkaew P. Functional segmentation for preoperative liver resection based on hepatic vascular networks. IEEE Access 2021;9:15485-98.
- Zhang Q, Fan Y, Wan J, Liu Y. An Efficient and Clinical-Oriented 3D Liver Segmentation Method. IEEE Access 2017;5:18737-44.
- Han X, Wu X, Wang S, Xu L, Xu H, Zheng D, Yu N, Hong Y, Yu Z, Yang D, Yang Z. Automated segmentation of liver segment on portal venous phase MR images using a 3D convolutional neural network. Insights Imaging 2022;13:26. [Crossref] [PubMed]
- England JR, Cheng PM. Artificial Intelligence for Medical Image Analysis: A Guide for Authors and Reviewers. AJR Am J Roentgenol 2019;212:513-9. [Crossref] [PubMed]
- Çiçek Ö, Abdulkadir A, Lienkamp SS, Brox T, Ronneberger O. 3D U-Net: Learning Dense Volumetric Segmentation from Sparse Annotation. In: Ourselin S, Joskowicz L, Sabuncu M, Unal G, Wells W, eds. Medical Image Computing and Computer-Assisted Intervention – MICCAI 2016. MICCAI 2016. Lecture Notes in Computer Science, vol 9901. Cham, Switzerland: Springer, 2016;424-32.
- Kishi Y, Vauthey JN. Issues to be considered to address the future liver remnant prior to major hepatectomy. Surg Today 2021;51:472-84. [Crossref] [PubMed]
- Khan AS, Garcia-Aroz S, Ansari MA, Atiq SM, Senter-Zapata M, Fowler K, Doyle MB, Chapman WC. Assessment and optimization of liver volume before major hepatic resection: Current guidelines and a narrative review. Int J Surg 2018;52:74-81. [Crossref] [PubMed]
- Dasari BVM, Hodson J, Roberts KJ, Sutcliffe RP, Marudanayagam R, Mirza DF, Isaac J, Muiesan P. Developing and validating a pre-operative risk score to predict post-hepatectomy liver failure. HPB (Oxford) 2019;21:539-46. [Crossref] [PubMed]
- Hanafy AS. Prediction and Prevention of Post-hepatectomy Liver Failure: Where Do We Stand? J Clin Transl Hepatol 2021;9:281-2. [Crossref] [PubMed]
- Huang SH, Wang BL, Cheng M, Wu WL, Huang XY, Ju Y. A Fast Method to Segment the Liver According to Couinaud Classification. In: Gao X, Muller H, Loomes MJ, Comley R, Luo S, Eds. Medical Imaging and Informatics. Berlin, Germany: Springer, 2008:270-6.
- Ruskó L, Mátéka I, Kriston A. Virtual volume resection using multi-resolution triangular representation of B-spline surfaces. Comput Methods Programs Biomed 2013;111:315-29. [Crossref] [PubMed]
- Butdee C, Pluempitiwiriyawej C, Tanpowpong N. 3D plane cuts and cubic Bézier curve for CT liver volume segmentation according to Couinaud classification. Songklanakarin Journal of Science and Technology 2017;39:793-801.
- Chen Y, Yue X, Zhong C, Wang G. Functional Region Annotation of Liver CT Image Based on Vascular Tree. Biomed Res Int 2016;2016:5428737. [Crossref] [PubMed]
- Marinelli B, Kang M, Martini M, Zech JR, Titano J, Cho S, Costa AB, Oermann EK. Combination of Active Transfer Learning and Natural Language Processing to Improve Liver Volumetry Using Surrogate Metrics with Deep Learning. Radiol Artif Intell 2019;1:e180019. [Crossref] [PubMed]
- Duan T, Jiang H, Xia C, Chen J, Cao L, Ye Z, Wei Y, Song B, Lee JM. Assessing Liver Function in Liver Tumors Patients: The Performance of T1 Mapping and Residual Liver Volume on Gd-EOBDTPA-Enhanced MRI. Front Med (Lausanne) 2020;7:215. [Crossref] [PubMed]
- Lin WH, Li K. Recent advances in preoperative assessment of hepatic functional reserve for hepatectomy. Zhonghua Wai Ke Za Zhi 2021;59:392-6. [PubMed]
- Pulitano C, Crawford M, Joseph D, Aldrighetti L, Sandroussi C. Preoperative assessment of postoperative liver function: the importance of residual liver volume. J Surg Oncol 2014;110:445-50. [Crossref] [PubMed]
- Shimada S, Kamiyama T, Kakisaka T, Orimo T, Nagatsu A, Asahi Y, Sakamoto Y, Kamachi H, Kudo Y, Nishida M, Taketomi A. The impact of elastography with virtual touch quantification of future remnant liver before major hepatectomy. Quant Imaging Med Surg 2021;11:2572-85. [Crossref] [PubMed]
- Kwon HJ, Kim KW, Kim B, Kim SY, Lee CS, Lee J, Song GW, Lee SG. Resection plane-dependent error in computed tomography volumetry of the right hepatic lobe in living liver donors. Clin Mol Hepatol 2018;24:54-60. [Crossref] [PubMed]
- Wang K, Mamidipalli A, Retson T, Bahrami N, Hasenstab K, Blansit K, Bass E, Delgado T, Cunha G, Middleton MS, Loomba R, Neuschwander-Tetri BA, Sirlin CB, Hsiao A. members of the NASH Clinical Research Network. Automated CT and MRI Liver Segmentation and Biometry Using a Generalized Convolutional Neural Network. Radiol Artif Intell 2019;1:180022. [Crossref] [PubMed]