Deep-learning-based biomarker of spinal cartilage endplate health using ultra-short echo time magnetic resonance imaging
Introduction
The spinal cartilage endplate (CEP) is a thin layer of hyaline-like cartilage that separates the vertebral body and the intervertebral disc (1,2). In addition to resisting mechanical forces from intradiscal pressure (3), the CEP is a semi-permeable barrier that enables nutrient transport from the vertebral capillaries into the avascular disc (4-6). Low CEP permeability—which is influenced by aspects of CEP biochemical composition including the relative amounts of collagen and aggrecan (5,7)—is thought to be one etiologic factor contributing to poor disc nutrition and disc degeneration (7-12). Low CEP permeability could also contribute to heterogenous outcomes following treatment with intradiscal biologic therapies, an emerging class of therapies for chronic low back pain (cLBP) (13-17). By design, such therapies increase intradiscal nutrient demands, and hence therapeutic efficacy may depend on CEP permeability (7,13-16). The importance of the CEP in disc degeneration and regeneration motivates non-invasive assessment of CEP health for elucidating the etiology of disc degeneration and helping to identify patients who may be likely to benefit from biologic therapies.
Due to its rapid signal decay, the CEP is not visible with conventional T1- or T2-weighted magnetic resonance imaging (MRI) sequences. Instead, sequences with ultra-short echo times (UTE) are needed to detect signal from the CEP and other short-T2 tissues (18-20). T2* relaxation times measured in the CEP using UTE MRI associate with biochemical traits such as the ratio of collagen-to-glycosaminoglycan (GAG) and tissue hydration, among other factors which influence CEP permeability (7,11,18). This may explain why discs adjacent to CEPs with shorter T2* relaxation times—implying lower CEP permeability—are more severely degenerated than discs adjacent to CEPs with longer T2* relaxation times (8). Those findings suggest that UTE-based T2* measurements could serve as biomarkers of CEP health and (possibly) the disc’s regenerative potential.
Prior studies that assessed CEP health with UTE imaging used manual or semi-automated methods to segment the CEP (8,20,21). Such methods are time consuming and subjective; for example, a single annotator may spend between 30 and 60 min manually segmenting the lower lumbar (L4-S1) CEPs for one patient, depending on anatomic variations in CEP presentation, image quantity and quality, and annotator experience. Segmentation accuracy can also vary between and within annotators, which limits the reliability of CEP biomarkers.
The primary goal of this study was to develop an efficient, objective, and accurate technique for automatically segmenting the lower lumbar CEPs from UTE images to enable rapid and reliable computation of T2* biomarkers of CEP health. To do this, we developed a convolutional neural network based on the u-net architecture (22), which has been successfully used to segment various tissues on MRI with high levels of accuracy (23-26). A secondary goal was to relate variations in model performance with aspects of UTE image quality [e.g., signal-to-noise (SNR) and contrast-to-noise (CNR) ratios] to facilitate model usage with UTE images acquired on different scanners. Finally, we sought to assess the diagnostic accuracy of the models for stratifying CEPs into groups based on T2* biomarkers, since CEP stratification could eventually help phenotype patients. The following article is presented in accordance with the STARD reporting checklist (available at https://qims.amegroups.com/article/view/10.21037/qims-22-729/rc).
Methods
Subjects
Between December 2018 and August 2021, 84 patients with cLBP and 20 asymptomatic subjects were prospectively recruited for this study (Figure 1). The study was conducted in accordance with the Declaration of Helsinki (as revised in 2013) and was approved by the institutional review board of the University of California, San Francisco. Written informed consent was taken from all individual participants. Patients were consecutively recruited from the non-operative spine service at our institution who met the criteria for cLBP established by the National Institutes of Health Pain Consortium Research Task Force (27): low-back pain for at least three months and at least half of the days in past 6 months. Asymptomatic subjects were recruited with print advertisements and with the help of a cohort identification tool that queries our institution’s electronic health records. Major exclusion criteria were a history of spine surgery, known disc herniation, a history of vertebral fracture, autoimmune disorder (including ankylosing spondylitis, rheumatoid arthritis, and psoriatic arthritis), or malignancy. Patient-reported measures for disability and pain were collected using the Oswestry Disability Index (ODI) (28) and Visual Analogue Scale (VAS) (29), respectively.
MRI
Subjects were imaged with 3.0-Tesla MRI (Discovery MR750 scanner, GE Healthcare) using an 8-channel phased-array spine coil. Sagittal acquisitions of the lumbar spine were obtained with a 3D UTE cones sequence and a chemical shift encoding-based (CSE) water-fat sequence (30-33). The UTE sequence parameters were: echo times (TE) =0.248, 5.2, 10.2, 15.2, 20.2, 25.2 ms; repetition time (TR) =32 ms; field-of-view (FOV) =28 cm; flip angle =17°; in-plane resolution =0.5 mm; slice-thickness =3 mm (interpolated to 1 mm); receiver bandwidth = ±83.3 kHz. The CSE acquisition included a six-echo 3D spoiled gradient-recalled echo (SPGR) sequence with iterative decomposition of water and fat with echo asymmetry and least-squares estimation (IDEAL) reconstruction. The CSE sequence had the following parameters: TE =2, 3, 4, 5, 6, 7 ms; TR =6.2 ms; FOV =26 cm; flip angle =3°; in-plane resolution =1.0 mm; slice thickness =4 mm, receiver bandwidth =83.3 kHz. Together, the UTE and CSE MRI acquisitions required a total scan time of approximately 16 minutes (UTE =13 minutes; CSE =3 minutes).
Image processing
To facilitate training of a neural network to segment the lower lumbar (L4-L5 and L5-S1) CEPs, the UTE images were cropped to a 128×128 pixel (64×64 mm) region centered on the L5 vertebral body (Figure 2A,2B). Cropping was performed automatically by: (I) segmenting the lumbar vertebral bodies from the CSE images using a previously developed neural network (25); (II) using the resulting vertebral segmentations to compute the centroid of the L5 vertebral body; and (III) mapping the coordinates of the L5 centroid from CSE space to UTE space using a coordinate transformation based on spatial information embedded in the image metadata (see Appendix 1). This automated process enabled objective, repeatable, and efficient isolation of a region encompassing the L4-L5 and L5-S1 CEPs; however, this image pre-processing step can be done manually and is thus not required to use the models described below.
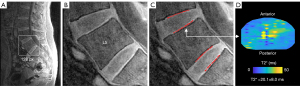
Using the cropped UTE images, two trained annotators manually segmented the superior and inferior CEPs at the L4-L5 and L5-S1 levels using custom segmentation software (IDL 8.8; Figure 2C). To facilitate CEP segmentation, this software up-samples the cropped images using bi-linear interpolation and normalizes the voxel signal intensities to the range 0–255 to magnify the anatomical features and enhance dynamic range and CEP contrast. The hyperintense CEP signal from the first UTE echo (TE =0.248 ms) guided manual CEP segmentation. Resulting CEP segmentations were typically 1–2 voxels thick (0.5–1.0 mm), which is consistent with histology measurements of CEP thickness (1,21).
CEP T2* relaxation-time biomarker
After CEP segmentation, T2* relaxation times were computed for all CEP voxels by fitting the signal decay of each voxel to the exponential decay function: where SIi denotes the signal intensity of voxel i (IDL 8.8).
The central CEP region is thought to be the predominant pathway for nutrient transport into the nucleus pulposus (4,10), thus the central CEP region was selected as the basis for CEP T2* biomarker computation (8,20). For each CEP, we identified the central transverse region adjacent to the nucleus pulposus by first determining the principal CEP orientation angle in the sagittal plane using the eigenvalues of the rotational inertia tensor computed from the segmented voxel data. After computing principal CEP angle, the 3D voxel data were rotated into a standard coordinate system and a kidney-bean-shaped template was applied defined by Mizrahi’s equation (MATLAB R2020b), as described previously (8,20). The central CEP region was defined so that it had a radius equal to 50% of the CEP’s outer margin. The CEP biomarker was defined as the mean T2* value in this central region (Figure 2D).
The inter- and intra-rater reliability of the segmentation and templating process for calculating mean CEP T2* values were assessed using the intraclass correlation coefficient (ICC). To do this, the annotators re-segmented a subset of 20 CEPs from five patients a minimum of four weeks after initial segmentation. Inter- and intra-rater ICC values were 0.83 [95% confidence interval (CI): 0.62–0.93] and 0.93 (0.81–0.97), respectively.
Deep learning models
Four independent convolutional neural networks were developed to predict CEP segmentation masks at the superior and inferior L4-L5 and L5-S1 levels (one neural network for each sublevel, e.g., L4-L5 superior). Four independent neural networks were developed instead of a single multi-class neural network because endplate damage at a given lumbar level—which is a common spinal pathology (34-36) and exclusion for biologic therapy—makes CEP segmentation difficult; using independent, level-specific neural networks facilitates robust model performance as the annotator prospectively chooses the subset of intact CEPs to segment in a given subject.
Images were split into training (80% of subjects) and testing groups (20% of subjects) for each level-specific neural network (37). Dataset division was done on a per-subject basis (as opposed to a per-image basis) because the mean CEP T2* value at each level is computed on a per-subject basis. The two groups were fully independent (that is, no images used in model training were from the same patient as those used in model testing). Separation into training and testing groups was done pseudo-randomly: the subjects were iteratively re-randomized until the distributions of mean CEP T2* values at each level for the training and testing group differed by <10%. This process ensured that both training and testing groups contained the clinically-observed range of CEP T2* values.
The level-specific neural networks were built using the unmodified u-net architecture (22) implemented in Python (3.7.2) and Keras (2.4.3) using Tensorflow (2.3.0). A binary cross-entropy loss function was used for model training with Adam optimization, learning rate of 10−4, and batch size of four. Random image augmentation was implemented to enhance the diversity of the training dataset and included rotation (±10°); shift, zoom, and shear (±20%); and histogram equalization (these model hyperparameters were fixed) (38). Models were trained for a minimum of 20 epochs and loss-function convergence was monitored and verified by inspection of the epoch versus loss decay curve. The inputs to the models were cropped UTE images (128×128 pixel area) and the outputs were probability distributions predicting the likelihood (range: 0–1) that each pixel was part of the CEP class. Based on these probability distributions, level-specific probability cutoffs for binarizing the CEP segmentations were chosen to maximize the 3D Dice scores in the test dataset (n=16 people). The 3D Dice scores were also plotted as a function of cutoff value in order to assess the sensitivity of model performance to the choice of cutoff value.
Model development and training was performed using a four-core 2.7 GHz Intel i7 CPU with 16 GB of memory. The trained models and instructions for use are available online (39).
SNR and CNR ratios
To explore imaging factors influencing neural network performance, we quantified CEP SNR and CNR. SNR was quantified for each image of the first UTE echo (TE =0.248 ms) as the mean CEP signal intensity normalized by the standard deviation (SD) of the image noise. Noise was quantified using the signal from nine circular regions-of-interest (ROI; each with an area of 1.2 cm2) placed randomly in an imaging region outside of the patient. CNR was quantified relative to the adjacent vertebral body and disc by offsetting the CEP segmentations by two pixels into the adjacent tissues (Figure 3). Contrast was calculated as the mean pixel-wise difference in signal intensity between the offset CEP segmentation and the actual CEP segmentation. Reported values for SNR and CNR reflect mean ± SD values computed using three consecutive mid-sagittal slices in each subject in the test dataset.
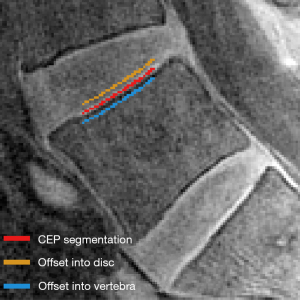
Second scanner analysis
The training and test datasets used to develop our models were acquired using a single MRI scanner. To assess the generalizability of the models to segment CEPs using images collected from a different scanner, we acquired additional UTE and CSE MRI data at 3.0-Tesla from a separate cohort of five patients with cLBP (age =57.8±14.0 years) imaged at a second site (Appendix 2). Model performance is reported for this group, though images acquired on the second scanner were not used as the primary test dataset since the cohort exhibited a much narrower range of CEP T2* values and comprised a much smaller sample size (the test dataset imaged using the primary scanner thus better reflects the full range of possible clinical conditions). Finally, we imaged phantoms comprised of varying agarose concentrations on the primary scanner (at two timepoints) and on the secondary scanner (single timepoint, see Appendix 2). The goal of this phantom analysis was to confirm that the UTE sequences that were implemented on the different scanners yielded similar T2* relaxation times for biochemically equivalent specimens.
Outcomes and statistical methods
Model performance was quantified in the unseen test dataset and in the separate cohort of patients imaged using a second scanner using the sensitivity, specificity, area under the receiver-operating characteristic curve (ROC-AUC), area under the precision-recall curve (PR-AUC), and Dice score (computed in 3D and for each sagittal slice) of the neural-network-generated segmentations relative to the manually-generated segmentations. Bland-Altman plots were used to compare CEP T2* values and CEP orientation angles from manually- versus model-generated segmentations (40). The Pearson correlation coefficient was used to test for associations between neural network performance metrics (sensitivity, specificity, and Dice score) and SNR and CNR.
To assess the diagnostic performance of the models, we stratified the CEPs into three equal-sized groups based on the tertiles of mean CEP T2* values observed in the manually segmented dataset: high CEP T2* (mean T2* > 66th percentile), medium (between 66th and 33rd percentile), and low (≤33rd percentile). The diagnostic performance of the models was tested by assessing the sensitivity and specificity of group stratifications made using the model-generated segmentations relative to manual segmentations. Statistical analyses were conducted in JMP Pro (16.0) and RStudio (1.2). Two-sided P<0.05 was considered statistically significant. Data are reported as mean ± SD or mean (95% CI).
Results
Complete MRI data were successfully acquired from all asymptomatic participants and 80/84 (95%) cLBP patients. Technical difficulties during MRI acquisition precluded including data from remaining 4/84 cLBP patients. Following exclusion based on CEP damage (17 subjects), 332 endplates from 83 subjects were included in the final analysis [n=20 asymptomatic subjects, mean ± SD age =40.3±11.0 years, 11 (55%) female and 9 (45%) male; n=63 with cLBP, age =40.2±12.0 years, 29 (46%) female and 34 (54%) male; Table 1]. Using this dataset, 67 subjects (5,628 images) were assigned to the training group and 16 subjects (1,344 images) to the testing group for each of the four spinal levels.
Table 1
Characteristic | Asymptomatic (n=20) | cLBP (n=63) | P value |
---|---|---|---|
Age (years) | 40.3±11.0 (23–67) | 40.2±12.0 (19–65) | 0.95 |
Female, male | 11 (55%), 9 (45%) | 29 (46%), 34 (54%) | 0.48 |
ODI | 0.8±2.3 (0–10) | 26.3±14.3 (2–74) | <0.001 |
VAS | 1.5±1.1 (1–5) | 6.6±2.5 (1–11) | <0.001 |
Data are presented as mean ± SD (min–max) or number (percent of total). Differences between groups were computed using Student’s t-tests and Chi-squared tests for continuous and categorical variables, respectively. cLBP, chronic low back pain; ODI, Oswestry Disability Index; VAS, Visual Analogue Scale; SD, standard deviation.
Segmentations produced by the trained neural networks appeared grossly similar to those generated manually (Figure 4). The sensitivity of the predicted segmentations (averaged for all subjects in the unseen test dataset) ranged from 0.798–0.894 and the 3D Dice score from 0.771–0.824, depending on spinal level (Table 2 upper). The specificity and ROC-AUC values were 0.99, primarily reflecting a relatively high true positive rate coupled with an imbalance on the order of 1:103 in the number of false-positives-to-true-negatives (thus yielding a false positive rate near zero). PR-AUC values, which are more informative in unbalanced datasets (41), ranged from 0.558–0.714. In addition to performance variations between different spinal levels, neural network performance also depended on sagittal image position. Segmentation performance (and hence the accuracy of intra-CEP spatial variation in T2* values) was highest mid-sagittally, particularly in the region comprising the mid-sagittal 50% where the central CEP is defined, and lowest laterally (Table 2 lower, Figure 5). The pixel probability cutoff values (range: 0–1) for binarizing the CEP segmentations to maximize the Dice scores were 0.24, 0.32, 0.24, and 0.30 for L4-L5 superior, L4-L5 inferior, L5-S1 superior, L5-S1 inferior, respectively (Figure 6). Dice scores were relatively insensitive to cutoff values over a wide range. For a given individual, the models required 14.1±0.2 s to segment all lumber CEPs.
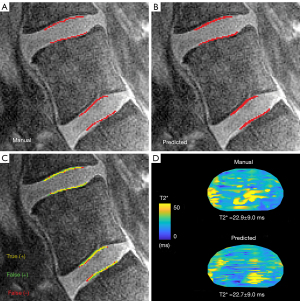
Table 2
Level | Sensitivity | Specificity | ROC-AUC | PR-AUC | 3D Dice score |
---|---|---|---|---|---|
Full volume | |||||
L4-L5 | |||||
Superior | 0.828 (0.781, 0.876) | 0.999 (0.999, 1.000) | 0.999 (0.998, 1.000) | 0.558 (0.465, 0.650) | 0.772 (0.734, 0.811) |
Inferior | 0.894 (0.878, 0.911) | 0.999 (0.999, 0.999) | 1.000 (1.000, 1.000) | 0.682 (0.635, 0.729) | 0.824 (0.805, 0.843) |
L5-S1 | |||||
Superior | 0.798 (0.748, 0.847) | 1.000 (0.999, 1.000) | 0.999 (0.999, 1.000) | 0.560 (0.469, 0.560) | 0.771 (0.735, 0.807) |
Inferior | 0.857 (0.800, 0.915) | 1.000 (0.999, 1.000) | 0.999 (0.999, 1.000) | 0.714 (0.662, 0.765) | 0.823 (0.789, 0.856) |
Mid-sagittal region | |||||
L4-L5 | |||||
Superior | 0.854 (0.806, 0.902) | 0.999 (0.999, 1.000) | 0.999 (0.998, 1.000) | 0.607 (0.510, 0.704) | 0.796 (0.760, 0.833) |
Inferior | 0.908 (0.891, 0.925) | 0.999 (0.999, 0.999) | 0.999 (0.999, 0.999) | 0.744 (0.712, 0.776) | 0.852 (0.841, 0.864) |
L5-S1 | |||||
Superior | 0.840 (0.788, 0.891) | 0.999 (0.999, 1.000) | 0.999 (0.998, 0.999) | 0.609 (0.511, 0.707) | 0.800 (0.760, 0.840) |
Inferior | 0.886 (0.830, 0.943) | 0.999 (0.999, 0.999) | 0.999 (0.999, 1.000) | 0.771 (0.723, 0.819) | 0.848 (0.815, 0.880) |
The data are shown as mean (95% CI) and reflect performance in the unseen test dataset (n=16 subjects per level). MRI, magnetic resonance imaging; CEP, cartilage endplate; ROC, receiver operating characteristic; AUC, area under the curve; PR, precision-recall; CI, confidence interval.
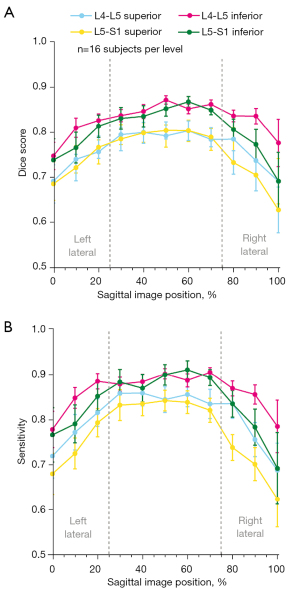
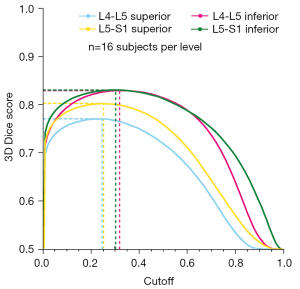
CEP SNR values were on average 10.6±3.6 (range: 4.9–20.6). CNR values between the CEP and adjacent vertebra (4.9±2.0, range: 1.5–10.0) were greater than those between the CEP and adjacent disc (2.1±0.9, range: 0.9–4.9).
The ability of the neural networks to accurately predict CEP segmentations depended on CEP SNR and CNR in the UTE images (Table 3). Among the parameters tested, model performance was most strongly correlated with CNR between the CEP and the adjacent vertebra (Pearson’s r=0.20–0.22, P<0.006 each for sensitivity, specificity, and Dice score). CNR between the CEP and the adjacent disc, and overall SNR, were associated with model sensitivity (r=0.17, P=0.02 each) but did not reach statistical significance for the Dice score (P=0.06 and 0.08 for disc CNR and SNR, respectively).
Table 3
Metric | SNR | CNR: CEP-disc | CNR: CEP-vertebra |
---|---|---|---|
Sensitivity | 0.166* | 0.165** | 0.220** |
Specificity | 0.117* | 0.231** | 0.197** |
2D Dice score | 0.125* | 0.134** | 0.210** |
* and ** indicate P<0.05 and P<0.01, respectively. SNR, signal-to-noise ratio; CNR, contrast-to-noise ratio; CEP, cartilage endplate.
Bland-Altman plots showed that model-predicted segmentations yielded unbiased estimates of mean T2* values in the central CEP (Figure 7A) and of principal CEP angle (Figure 7B). Mean (95% CI) bias in the T2* estimates was 0.33 ms (−0.26, 0.93); mean bias in the angle estimates was 0.36° (−0.30, 1.03). There were high levels of overall agreement; specifically, the majority (60/64, 94%) of predicted CEP T2* values and angles were within ±4.0 ms and ±5° of the respective values computed using manual segmentations.
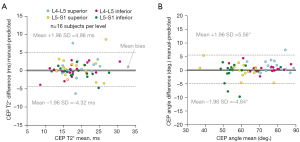
The distribution of T2* values in the test dataset were similar between manually-generated segmentations (mean T2* =18.9±4.7 ms, range: 6.8–32.0 ms) and model-generated segmentations (mean T2* =18.6±4.4 ms, range: 10.6–29.4 ms). The distribution computed from manual segmentations was used to stratify CEPs into tertiles denoting low (≤16.5 ms), medium (>16.5 and ≤21.0 ms), and high (>21.0 ms) T2* groups. Model-predicted stratification of individual CEPs into low, medium, and high T2* groups (Table 4) had sensitivities of 0.86, 0.86, and 0.77, respectively, and specificities of 0.93, 0.86, and 0.95, respectively.
Table 4
Manual | Predicted | |||
---|---|---|---|---|
Low | Mid | High | All | |
Low (≤16.5 ms) | 18 | 2 | 1 | 21 |
Medium (>16.5 and ≤21.0 ms) | 2 | 18 | 1 | 21 |
High (>21.0 ms) | 1 | 4 | 17 | 22 |
All | 21 | 24 | 19 | 64 |
n=64 CEPs from 16 subjects. CEP, cartilage endplate.
The neural networks demonstrated similar levels of performance when applied to images collected using a second scanner (Appendix 2). In the five patients imaged, CNR and SNR values from the second scanner were approximately 1.5- and 2-fold higher, respectively, than in the primary scanner. Model-predicted segmentations yielded unbiased estimates of mean T2* values and orientation angles when compared to the manual segmentations: mean (95% CI) bias in T2* estimates was 0.03 ms (−0.97, 1.02) and mean bias in the angle estimates was 1.41° (−0.10, 2.91). As with the test dataset imaged with the primary scanner, the model application to the dataset from the second scanner showed high levels of overall agreement: 94% (16/17) of predicted CEP T2* values were within ±4.0 ms, and 88% (15/17) of predicted angles were within ±5° of the respective values computed from manually-generated segmentations.
Results from the agarose phantoms showed that T2* values were similar between phantoms imaged at both sites, and at the two timepoints imaged at the primary site, demonstrating that the UTE sequences implemented on the different scanners yielded similar T2* relaxation time values for biochemically equivalent specimens (Appendix 2).
Discussion
The neural networks developed here produced accurate segmentations of the lower lumbar CEPs on UTE images, which enabled efficient and reliable computation of T2*-based biomarkers of CEP health. The imaging data used to develop these models were acquired from subjects spanning a wide range of ages, spinal morphologies, and clinical conditions, including both asymptomatic subjects and patients with cLBP. We found that segmentation performance measured using the Dice similarity score varied between 0.77–0.85, depending on spinal level, sagittal image position, and aspects of image quality including the contrast between the CEP and adjacent vertebra. Voxel-wise errors in model-generated segmentations did not propagate to biomarker computation: in the unseen dataset used for model testing and the smaller independent dataset imaged using a second scanner, 94% (60/64 test dataset, 16/17 second scanner dataset) of predicted mean CEP T2* values were within ±4.0 ms of manual values with an overall mean bias of <0.5 ms. The magnitudes of those errors are small relative to the inter- and intra-subject heterogeneity in CEP T2* values (8,20). Thus, we conclude that these trained deep-learning-based models enable accurate, automated CEP segmentations and T2* biomarker computations that are statistically similar to those generated manually, and in doing so, address limitations with inefficiency and subjectivity associated with manual methods.
We showed that stratifying CEPs into T2*-based sub-groups using automated segmentations was possible with a relatively high combination of sensitivity (0.77–0.86) and specificity (0.86–0.95). In general, stratifying CEPs in this manner could help phenotype patients by identifying patients and levels in which CEP composition is most or least likely to influence disc degeneration. However, the specific T2* thresholds for stratification were chosen based on the observed tertiles in our sample distribution, since the actual T2* thresholds that implicate the CEP as a primary factor in disc degeneration remain unknown. Future studies in larger cohorts are required to establish the full clinical range of CEP T2* values and to clarify whether there are distinct T2* phenotypes that influence disc degeneration and prognosticate treatment response following intradiscal biologic therapy.
Deep-learning-based techniques including convolutional neural networks based on the u-net architecture have been successfully used to address the limitations of manual segmentation for a variety of tissues and imaging modalities (22-26). One factor challenging the clinical utility of such models is that the mechanistic factors explaining model performance are difficult to discern and quantify (42); a lack of understanding of how algorithms make decisions—the so-called “black box” concept (43)—obscures algorithm generalizability. In part for this reason, the World Health Organization (44) along with international radiology societies (45) have recently advocated that artificial intelligence tools be explainable to some extent. Here, we found that model performance depended on aspects of UTE image quality including SNR and CNR (particularly the contrast between the CEP and the adjacent vertebral body), and we reported SNR and CNR benchmarks to help facilitate comparable levels of model performance across different imaging systems. Supporting the external validity and generalizability of our models, we found similar levels of model performance in a separate cohort of cLBP patients imaged with UTE MRI in a second scanner with high SNR and CNR values.
We recently showed (8) that CEPs with lower mean T2* values were associated with more severe disc degeneration in patients with cLBP, which supports the clinical relevance of assessing CEP composition using UTE-T2* biomarkers. The clinical implications of non-invasive assessment of CEP health are also underscored by the need to understand heterogenous treatment outcomes observed in cLBP patients treated with intradiscal biologic therapies. For example, clinical trials of intradiscal biologic therapies have not produced consistent results (13), with several randomized controlled studies showing no difference from placebo (17). The inconsistent therapeutic efficacy of such therapies may partly relate to deficits in disc nutrient supply, since factors such as low CEP permeability could limit the number of cells that can survive in the avascular disc (13-15). Thus, the techniques described here for rapid and reliable computation of CEP compositional biomarkers from UTE MRI have clinical implications for elucidating the role of CEP composition in disc degeneration and regeneration.
This study has several limitations. First, the datasets used to train and test the models met a particular set of clinical conditions, and model performance in patients and levels not meeting those criteria is unknown. Factors that could affect the signal in the vertebral body relative to the CEP, including chemical shift and partial volume effects (which can vary depending on imaging parameters), and signal intensity changes caused by pathologies such as vertebral endplate bone marrow lesions [Modic changes (46)] or hemangiomas, could impact model performance. Nonetheless, the datasets used here include a variety of clinical conditions including asymptomatic subjects and patients with cLBP spanning a wide ranges of disability scores, ages, and approximately equal numbers of females and males. Further, the training and testing datasets each comprised the clinically-observed range of CEP T2* values: differentiating groups in this way was done to limit dataset bias (47). Related, dataset division was done according to a two-group design [one training group (80% of patients) and one testing group (20% of patients)] as opposed to a three-group design (training, validation, and testing). This design was chosen because a previous study in different subjects had determined the model hyperparameters (38), which is one purpose of the validation dataset meant to mitigate overfitting. Thus, the two-group design enabled a larger unseen dataset in which to test model performance. Importantly, images used for model training were from different subjects than those used for model testing (47). We also tested the model in an additional independent cohort of patients imaged on a second scanner (Appendix 2) to assess model generalizability; model performance is reported for this group, but it was not used as the primary test dataset since the cohort exhibited a much narrower range of CEP T2* values and comprised a much smaller sample size (the test dataset imaged using the primary scanner thus better reflects the full range of possible clinical conditions). Nevertheless, users should be aware of the limited clinical criteria used to develop and test these models.
A second limitation relates to the time-saving benefits of the software pipeline. CEP segmentation itself is only one step in CEP T2* biomarker computation. UTE image pre-processing, T2* relaxation-time mapping, and CEP transformation and templating are also necessary steps, and those steps are not facilitated by the neural networks. However, those additional steps are rapid (5–10 minutes) relative to the time required for manual segmentation and could be further automated.
A final limitation relates to the clinical applicability of CEP T2* measurements. CEP signal intensity and T2* values are sensitive to imaging parameters such as bandwidth influencing chemical shift and partial volume effects (48), in addition to orientation in the scanner due to magic angle effects (18,49). Thus, clinical studies using CEP T2* values as biomarkers should report the imaging parameters used, and also account for the effects of CEP orientation on T2* values—for example, by limiting CEP T2* analysis to spinal levels oriented near the magic angle so that inter-CEP variations in T2* values primarily reflect differences in biochemical composition and not differences in CEP orientation (8,18). For this reason, we reported principal CEP orientation and prediction accuracy (±5° of manually-generated values 94% of the time). Future studies are needed to clarify both the biochemical factors influencing T2* relaxation times in the CEP, and the detailed effects of imaging orientation on CEP T2* values.
Conclusions
In summary, this study demonstrates the feasibility and accuracy of automatically segmenting human lumbosacral CEPs on UTE images. This enables efficient, objective, and reliable computation of T2*-based biomarkers of CEP composition that are statistically similar to the T2* biomarkers derived from manual segmentations. These automated techniques help address limitations in inefficiency and subjectivity associated with manual methods, and may therefore aid in the clinical adoption of UTE MRI for elucidating the role of CEP composition in disc degeneration etiology and guiding emerging intradiscal biologic therapies for cLBP.
Acknowledgments
The authors acknowledge Ronit Gupta for his help in data collection that contributed to the results shown here.
Funding: The research reported in this publication was supported by the National Institute Of Arthritis And Musculoskeletal And Skin Diseases of the National Institutes of Health under award numbers UH2AR076719, UH3AR076719, U19AR076737, and F32AR081139, and through R01AR070198. The content is solely the responsibility of the authors and does not necessarily represent the official views of the National Institutes of Health.
Footnote
Reporting Checklist: The authors have completed the STARD reporting checklist. Available at https://qims.amegroups.com/article/view/10.21037/qims-22-729/rc
Conflicts of Interest: All authors have completed the ICMJE uniform disclosure form (available at https://qims.amegroups.com/article/view/10.21037/qims-22-729/coif). RK and AJF report that the study was funded by a grant from the National Institute of Arthritis and Musculoskeletal and Skin Diseases (NIAMS). The other authors have no conflicts of interest to declare.
Ethical Statement: The authors are accountable for all aspects of the work in ensuring that questions related to the accuracy or integrity of any part of the work are appropriately investigated and resolved. The study was conducted in accordance with the Declaration of Helsinki (as revised in 2013). This study was approved by the institutional review board of the University of California, San Francisco and written informed consent was taken from all individual participants.
Open Access Statement: This is an Open Access article distributed in accordance with the Creative Commons Attribution-NonCommercial-NoDerivs 4.0 International License (CC BY-NC-ND 4.0), which permits the non-commercial replication and distribution of the article with the strict proviso that no changes or edits are made and the original work is properly cited (including links to both the formal publication through the relevant DOI and the license). See: https://creativecommons.org/licenses/by-nc-nd/4.0/.
References
- Roberts S, Menage J, Urban JP. Biochemical and structural properties of the cartilage end-plate and its relation to the intervertebral disc. Spine (Phila Pa 1976) 1989;14:166-74. [Crossref] [PubMed]
- Moore RJ. The vertebral end-plate: what do we know? Eur Spine J 2000;9:92-6. [Crossref] [PubMed]
- Berg-Johansen B, Fields AJ, Liebenberg EC, Li A, Lotz JC. Structure-function relationships at the human spinal disc-vertebra interface. J Orthop Res 2018;36:192-201. [PubMed]
- Nachemson A, Lewin T, Maroudas A, Freeman MA. In vitro diffusion of dye through the end-plates and the annulus fibrosus of human lumbar inter-vertebral discs. Acta Orthop Scand 1970;41:589-607. [Crossref] [PubMed]
- Roberts S, Urban JP, Evans H, Eisenstein SM. Transport properties of the human cartilage endplate in relation to its composition and calcification. Spine (Phila Pa 1976) 1996;21:415-20. [Crossref] [PubMed]
- Holm S, Maroudas A, Urban JP, Selstam G, Nachemson A. Nutrition of the intervertebral disc: solute transport and metabolism. Connect Tissue Res 1981;8:101-19. [Crossref] [PubMed]
- Wong J, Sampson SL, Bell-Briones H, Ouyang A, Lazar AA, Lotz JC, Fields AJ. Nutrient supply and nucleus pulposus cell function: effects of the transport properties of the cartilage endplate and potential implications for intradiscal biologic therapy. Osteoarthritis Cartilage 2019;27:956-64. [Crossref] [PubMed]
- Bonnheim NB, Wang L, Lazar AA, Zhou J, Chachad R, Sollmann N, Guo X, Iriondo C, O'Neill C, Lotz JC, Link TM, Krug R, Fields AJ. The contributions of cartilage endplate composition and vertebral bone marrow fat to intervertebral disc degeneration in patients with chronic low back pain. Eur Spine J 2022;31:1866-72. [Crossref] [PubMed]
- Shirazi-Adl A, Taheri M, Urban JP. Analysis of cell viability in intervertebral disc: Effect of endplate permeability on cell population. J Biomech 2010;43:1330-6. [Crossref] [PubMed]
- Rajasekaran S, Babu JN, Arun R, Armstrong BR, Shetty AP, Murugan S. ISSLS prize winner: A study of diffusion in human lumbar discs: a serial magnetic resonance imaging study documenting the influence of the endplate on diffusion in normal and degenerate discs. Spine (Phila Pa 1976) 2004;29:2654-67. [Crossref] [PubMed]
- Dolor A, Sampson SL, Lazar AA, Lotz JC, Szoka FC, Fields AJ. Matrix modification for enhancing the transport properties of the human cartilage endplate to improve disc nutrition. PLoS One 2019;14:e0215218. [Crossref] [PubMed]
- Sampson SL, Sylvia M, Fields AJ. Effects of dynamic loading on solute transport through the human cartilage endplate. J Biomech 2019;83:273-9. [Crossref] [PubMed]
- Binch ALA, Fitzgerald JC, Growney EA, Barry F. Cell-based strategies for IVD repair: clinical progress and translational obstacles. Nat Rev Rheumatol 2021;17:158-75. [Crossref] [PubMed]
- Huang YC, Urban JP, Luk KD. Intervertebral disc regeneration: do nutrients lead the way? Nat Rev Rheumatol 2014;10:561-6. [Crossref] [PubMed]
- Vedicherla S, Buckley CT. Cell-based therapies for intervertebral disc and cartilage regeneration- Current concepts, parallels, and perspectives. J Orthop Res 2017;35:8-22. [Crossref] [PubMed]
- Moriguchi Y, Alimi M, Khair T, Manolarakis G, Berlin C, Bonassar LJ, Härtl R. Biological Treatment Approaches for Degenerative Disk Disease: A Literature Review of In Vivo Animal and Clinical Data. Global Spine J 2016;6:497-518. [Crossref] [PubMed]
- Ju DG, Kanim LE, Bae HW. Is There Clinical Improvement Associated With Intradiscal Therapies? A Comparison Across Randomized Controlled Studies. Global Spine J 2022;12:756-64. [Crossref] [PubMed]
- Fields AJ, Han M, Krug R, Lotz JC. Cartilaginous end plates: Quantitative MR imaging with very short echo times-orientation dependence and correlation with biochemical composition. Radiology 2015;274:482-9. [Crossref] [PubMed]
- Robson MD, Gatehouse PD, Bydder M, Bydder GM. Magnetic resonance: an introduction to ultrashort TE (UTE) imaging. J Comput Assist Tomogr 2003;27:825-46. [Crossref] [PubMed]
- Wang L, Han M, Wong J, Zheng P, Lazar AA, Krug R, Fields AJ. Evaluation of human cartilage endplate composition using MRI: Spatial variation, association with adjacent disc degeneration, and in vivo repeatability. J Orthop Res 2021;39:1470-8. [Crossref] [PubMed]
- Berg-Johansen B, Han M, Fields AJ, Liebenberg EC, Lim BJ, Larson PE, Gunduz-Demir C, Kazakia GJ, Krug R, Lotz JC. Cartilage Endplate Thickness Variation Measured by Ultrashort Echo-Time MRI Is Associated With Adjacent Disc Degeneration. Spine (Phila Pa 1976) 2018;43:E592-600. [Crossref] [PubMed]
- Ronneberger O, Fischer P, Brox T. U-net: convolutional networks for biomedical image segmentation. In: Navab N, Hornegger J, Wells W, Frangi A. editors. Medical Image Computing and Computer-Assisted Intervention. Cham: Springer, 2015:16591-603.
- Wang Y, Zhang Y, Wen Z, Tian B, Kao E, Liu X, Xuan W, Ordovas K, Saloner D, Liu J. Deep learning based fully automatic segmentation of the left ventricular endocardium and epicardium from cardiac cine MRI. Quant Imaging Med Surg 2021;11:1600-12. [Crossref] [PubMed]
- Hamabe A, Ishii M, Kamoda R, Sasuga S, Okuya K, Okita K, Akizuki E, Sato Y, Miura R, Onodera K, Hatakenaka M, Takemasa I. Artificial intelligence-based technology for semi-automated segmentation of rectal cancer using high-resolution MRI. PLoS One 2022;17:e0269931. [Crossref] [PubMed]
- Zhou J, Damasceno PF, Chachad R, Cheung JR, Ballatori A, Lotz JC, Lazar AA, Link TM, Fields AJ, Krug R. Automatic Vertebral Body Segmentation Based on Deep Learning of Dixon Images for Bone Marrow Fat Fraction Quantification. Front Endocrinol (Lausanne) 2020;11:612. [Crossref] [PubMed]
- Hsu LM, Wang S, Walton L, Wang TW, Lee SH, Shih YI. 3D U-Net Improves Automatic Brain Extraction for Isotropic Rat Brain Magnetic Resonance Imaging Data. Front Neurosci 2021;15:801008. [Crossref] [PubMed]
- Deyo RA, Dworkin SF, Amtmann D, Andersson G, Borenstein D, Carragee E, Carrino J, Chou R, Cook K, DeLitto A, Goertz C, Khalsa P, Loeser J, Mackey S, Panagis J, Rainville J, Tosteson T, Turk D, Von Korff M, Weiner DK. Report of the NIH Task Force on research standards for chronic low back pain. J Pain 2014;15:569-85. [Crossref] [PubMed]
- Fairbank JC, Pynsent PB. The Oswestry Disability Index. Spine (Phila Pa 1976) 2000;25:2940-52; discussion 2952. [Crossref] [PubMed]
- Chiarotto A, Maxwell LJ, Ostelo RW, Boers M, Tugwell P, Terwee CB. Measurement Properties of Visual Analogue Scale, Numeric Rating Scale, and Pain Severity Subscale of the Brief Pain Inventory in Patients With Low Back Pain: A Systematic Review. J Pain 2019;20:245-63. [Crossref] [PubMed]
- Law T, Anthony MP, Chan Q, Samartzis D, Kim M, Cheung KM, Khong PL. Ultrashort time-to-echo MRI of the cartilaginous endplate: technique and association with intervertebral disc degeneration. J Med Imaging Radiat Oncol 2013;57:427-34. [Crossref] [PubMed]
- Bae WC, Statum S, Zhang Z, Yamaguchi T, Wolfson T, Gamst AC, Du J, Bydder GM, Masuda K, Chung CB. Morphology of the cartilaginous endplates in human intervertebral disks with ultrashort echo time MR imaging. Radiology 2013;266:564-74. [Crossref] [PubMed]
- Sollmann N, Bonnheim NB, Joseph GB, Chachad R, Zhou J, Akkaya Z, Pirmoazen AM, Bailey JF, Guo X, Lazar AA, Link TM, Fields AJ, Krug R. Paraspinal Muscle in Chronic Low Back Pain: Comparison Between Standard Parameters and Chemical Shift Encoding-Based Water-Fat MRI. J Magn Reson Imaging 2022;56:1600-8. [Crossref] [PubMed]
- Gee CS, Nguyen JT, Marquez CJ, Heunis J, Lai A, Wyatt C, Han M, Kazakia G, Burghardt AJ, Karampinos DC, Carballido-Gamio J, Krug R. Validation of bone marrow fat quantification in the presence of trabecular bone using MRI. J Magn Reson Imaging 2015;42:539-44. [Crossref] [PubMed]
- Lotz JC, Fields AJ, Liebenberg EC. The role of the vertebral end plate in low back pain. Global Spine J 2013;3:153-64. [Crossref] [PubMed]
- Rundell SD, Sherman KJ, Heagerty PJ, Mock CN, Dettori NJ, Comstock BA, Avins AL, Nedeljkovic SS, Nerenz DR, Jarvik JG. Predictors of Persistent Disability and Back Pain in Older Adults with a New Episode of Care for Back Pain. Pain Med 2017;18:1049-62. [PubMed]
- Jensen TS, Karppinen J, Sorensen JS, Niinimäki J, Leboeuf-Yde C. Vertebral endplate signal changes (Modic change): a systematic literature review of prevalence and association with non-specific low back pain. Eur Spine J 2008;17:1407-22. [Crossref] [PubMed]
- Rácz A, Bajusz D, Héberger K. Effect of Dataset Size and Train/Test Split Ratios in QSAR/QSPR Multiclass Classification. Molecules 2021;26:1111. [Crossref] [PubMed]
- Wang L, Zhou J, Guo X, Zheng P, Krug R, Fields AJ. Deep learning-based automated segmentation of the human cartilage endplate for T2* measurement with UTE MRI. Orthopaedic Research Society Annual Meeting Virtual 2021.
- Bonnheim NB, Wang L, Lazar AA, Chachad R, Zhou J, Guo X, O’Neill C, Krug R, Fields AJ. CEP-seg: automatic segmentation of cartilage endplate (CEP). 2022. Available online: https://github.com/wanglss/CEP-seg
- Altman DG, Bland JM. Measurement in medicine: the analysis of method comparison studies. Journal of the Royal Statistical Society. Series D (The Statistician) 1983;32:307-17. [Crossref]
- Saito T, Rehmsmeier M. The precision-recall plot is more informative than the ROC plot when evaluating binary classifiers on imbalanced datasets. PLoS One 2015;10:e0118432. [Crossref] [PubMed]
- Markus AF, Kors JA, Rijnbeek PR. The role of explainability in creating trustworthy artificial intelligence for health care: A comprehensive survey of the terminology, design choices, and evaluation strategies. J Biomed Inform 2021;113:103655. [Crossref] [PubMed]
- Bluemke DA, Moy L, Bredella MA, Ertl-Wagner BB, Fowler KJ, Goh VJ, Halpern EF, Hess CP, Schiebler ML, Weiss CR. Assessing Radiology Research on Artificial Intelligence: A Brief Guide for Authors, Reviewers, and Readers-From the Radiology Editorial Board. Radiology 2020;294:487-9. [Crossref] [PubMed]
- World Health Organization. Ethics and governance of artificial intelligence for health: WHO guidance. 2021. Available online: https://www.who.int/publications/i/item/9789240029200
- Geis JR, Brady AP, Wu CC, Spencer J, Ranschaert E, Jaremko JL, Langer SG, Borondy Kitts A, Birch J, Shields WF, van den Hoven van Genderen R, Kotter E, Wawira Gichoya J, Cook TS, Morgan MB, Tang A, Safdar NM, Kohli M. Ethics of Artificial Intelligence in Radiology: Summary of the Joint European and North American Multisociety Statement. Radiology 2019;293:436-40. [Crossref] [PubMed]
- Modic MT, Steinberg PM, Ross JS, Masaryk TJ, Carter JR. Degenerative disk disease: assessment of changes in vertebral body marrow with MR imaging. Radiology 1988;166:193-9. [Crossref] [PubMed]
- Varoquaux G, Cheplygina V. Machine learning for medical imaging: methodological failures and recommendations for the future. NPJ Digit Med 2022;5:48. [Crossref] [PubMed]
- Bydder M, Carl M, Bydder GM, Du J. MRI chemical shift artifact produced by center-out radial sampling of k-space: a potential pitfall in clinical diagnosis. Quant Imaging Med Surg 2021;11:3677-83. [Crossref] [PubMed]
- Erickson SJ, Prost RW, Timins ME. The "magic angle" effect: background physics and clinical relevance. Radiology 1993;188:23-5. [Crossref] [PubMed]