Improving image quality and resolution of coronary arteries in coronary computed tomography angiography by using high-definition scans and deep learning image reconstruction
Introduction
Coronary atherosclerotic heart disease is caused by atherosclerotic lesions of the coronary artery, which in turn precipitate the stenosis or obstruction of the vascular cavity, myocardial ischemia, hypoxia, or necrosis. This condition is often called coronary heart disease. In recent years, the incidence rate of coronary heart disease and its mortality has been rapidly increasing (1-3). Coronary heart disease is more common in adults over 40 years old, and the incidence of the disease in males is earlier than in females, showing a younger trend in recent years. Coronary computed tomography angiography (CTA) is a powerful noninvasive imaging technology with a high temporal and spatial resolution that has improved risk stratification and management in patients with low-to-moderate coronary artery disease (CAD). Notably, coronary CTA is also an effective, safe, noninvasive, outpatient alternative to invasive coronary angiography (ICA) for patients with a high probability of clinically obstructive CAD (4-6).
However, there is an increasing need to further improve the spatial resolution of the coronary CTA system to improve diagnostic accuracy, especially in cases of calcification, and to assess the restenosis inside stents. Despite improvements in CT technology, extensive calcifications remain problematic, as the lesions are frequently uninterpretable, or their severity is overestimated (7). Assessment of stented lesions is another limitation of CTA. The quantification of in-stent lumens in coronary CTA is challenging due to beam-hardening artifacts caused by metallic stent struts, especially in stents with a diameter <3.0 mm. This is mainly attributable to the limited spatial resolution of standard-resolution computed tomography (CT) (8). Assessment of stented lesions is another limitation of CTA. Image resolution in coronary CTA may be increased by using high-definition (HD) scanning, the HD reconstruction mode, or high-pass reconstruction kernels. However, under the same radiation dose (signal strength), image resolution increase is often accompanied by image noise increase in the HD reconstruction mode with conventional reconstruction algorithms, which may adversely affect image quality and diagnostic accuracy. CT manufacturers have implemented automatic tube current modulation, bowtie filters, lower tube voltage (kVp), adaptive collimation, and iterative reconstruction (IR) algorithms to reduce the dose and optimize image quality (9,10). IR algorithms, such as the adaptive statistical iterative reconstruction-V (ASIR-V) algorithm, have been developed to reduce image noise. However, studies have shown that the use of medium-strength IR algorithms may not be adequate to overcome the noise increase. High-strength IR algorithms sometimes cause over-smoothing in images, reduce spatial resolution, and produce unnatural-looking image textures (11-13), which may negate the benefits of using the HD scanning and reconstruction mode.
Recently, several CT manufacturers have developed deep learning–based reconstruction algorithms that have demonstrated strong image noise-reduction ability (14-17). The algorithm developed by United Imaging uses a 3-dimensional (3D) residual convolutional network to learn the difference between low-dose images and normal-dose images (18), while the algorithm developed by Canon uses the images reconstructed with a model-based IR algorithm as the gold standard. GE Healthcare developed a deep learning image reconstruction (DLIR) algorithm based on a deep convolutional neural network. DLIR is the first of its kind in the world to be approved by the Food and Drug Administration (FDA, USA). A CT image reconstruction engine based on a deep neural network can generate high-quality TrueFidelity CT images. DLIR solves the current challenges of filtered back projection (FBP) and IR algorithms and represents a new era of CT image reconstruction (19). DLIR is trained by using standard-dose, high-quality FBP images, which, in theory, can provide powerful noise-reduction capabilities while maintaining the high spatial resolution of detailed structures (20). The aim of our study was to evaluate the feasibility of using DLIR at a high level (DLIR-H) with the HD reconstruction mode to improve image quality and spatial resolution for imaging calcified plaques and stents in coronary CTA in comparison with ASIR-V with the standard-definition (SD) reconstruction mode. We present the following article in accordance with the GRRAS reporting checklist (available at https://qims.amegroups.com/article/view/10.21037/qims-22-186/rc).
Methods
Patient population
The study was conducted in accordance with the Declaration of Helsinki (as revised in 2013). This study was approved by our institutional review committee, and all patients or their family members signed informed consent for undergoing coronary CTA. The requirement for a signed consent form for using the images was waived due to the retrospective nature of this study.
From August to October 2020, 34 patients who underwent coronary CTA in The First Affiliated Hospital of Xi’an Jiaotong University including 15 males and 19 females, were included in the study (Table 1). These patients were diagnosed with CAD by imaging and laboratory pathological examination, and no other serious heart, liver, or kidney diseases were found. The sample size was 34, and the sampling method was random sampling.
Table 1
Characteristic | Value |
---|---|
All patients (n) | 34 |
Age (years), mean [range] | 63 [37–83] |
Men, n (%) | 15 (44.12) |
Women, n (%) | 19 (55.88) |
Height (cm) | 164±8.01 |
Weight (kg) | 60.93±6.58 |
Body mass index (kg/m2) | 22.50±3.29 |
Calcified plaques (n) | 45 |
Coronary stents (n) | 4 |
Date are presented as mean ± standard deviation, if not otherwise specified.
CT acquisition parameters
All patients were examined on a GE Revolution CT (GE Healthcare, Chicago, IL, USA). The HD scanning mode was used. The other scanning parameters were as follows: a tube voltage of 100 kV, a smart tube current (SmartmA) range of 300–570 mA, a gantry rotation speed of 0.28 s, a slice thickness of 0.625 mm, a slice spacing of 0.625 mm, and a collimation width of 256×0.625 mm.
Patients were scanned in the supine position and under free breathing. The scanning range was from 2 cm below the bifurcation of the trachea to 2 cm below the diaphragm. Patients were injected with the contrast agent of iohexol (370 gI/L). The contrast dose and flow rate were calculated according to the body mass index of the patient. The contrast dose was 35–50 mL, and the flow rate was 3.5–5 mL/s. Coronary CTA was triggered using the bolus-tracking technique and started after the descending aorta reached the threshold of 210 Hounsfield unit (HU).
Image reconstruction
After scanning, coronary CTA images were reconstructed in 3 reconstruction modes: SD with 50% ASIR-V (SD-ASIR-V50%), HD with 50% ASIR-V (HD-ASIR-V50%), and HD with high-level DLIR (HD-DLIR-H). The high level for DLIR was selected based on the previously published results for coronary CTA application (21) and to limit the scope of our study.
Image analysis
The 3 reconstruction groups were transferred to a postprocessing workstation (Advantage Workstation 4.7, GE Healthcare) for measurement and evaluation. CT values (the corresponding value of each tissue in the image corresponding to the X-ray attenuation coefficient) and their standard deviation values at 1 cm from the opening of the right coronary artery, left anterior descending branch, left circumflex branch, the aorta, and the pericardial fat at the left crown outlet were measured by 2 radiologists independently and averaged to produce the final results. The measured area was 150–200 mm2. Lesions, vascular calcification, and artifacts were avoided during measurement. Image noise (expressed as the standard deviation value), signal-to-noise ratio (SNR; SNR = mean HU value/noise), and contrast-to-noise ratio [CNR; (mean HU value of the aorta − mean HU value of the paraspinal muscle)/standard deviation of the subcutaneous fat] of the aorta were calculated. The diameter of calcified plaques at their maximum cross-section was measured to evaluate the impact of spatial image resolution of the 3 different scan-reconstruction combinations on image quality. For patients with stents, CT values of the lumen at 3 locations (in-stent, just out of the stent, and at the proximal and distal ends) were measured along the stented lumen. Smaller calcification size measurements and smaller differences among CT value measurements of the in- and out-stent lumens indicated fewer blurring artifacts and higher image spatial resolution.
The same 2 radiologists with more than 5 years of experience evaluated subjective image quality in terms of overall image noise, blood vessels edge display, clarity of calcification, and the display of the in-stent lumen using a 5-point scoring system. For each image, there was no annotation to ensure readers were blinded to patient information and the algorithms used for reconstruction. The scores of the 2 radiologists were averaged to generate the final score. Scoring criteria were as follows: 5 points, excellent image quality with no artifacts, very low noise, excellent vessel enhancement and contrast from surrounding areas, clear and sharp edges of calcified plaques, and clear display of stent and in-stent lumen; 4 points, good image quality with no artifacts, low noise, good vessel enhancement and contrast from surrounding tissues, clear edges of calcified plaque, and somewhat clear display of stent and in-stent lumen without obvious interference; 3 points, average image quality with some artifacts, moderate image noise, adequate vessel enhancement and contrast from surrounding tissue, somewhat blurred edge of calcified plaque, and stent and in-stent lumen observable but somewhat blurred; 2 points, poor image quality with artifacts, heavy image noise, poor vessel enhancement and poor contrast between blood vessels and surrounding tissues, blurred edge of calcified plaque, and unidentifiable stent and in-stent lumen; and 1 point, unacceptable image quality with heavy artifacts, excessive image noise, poor vessel enhancement and poor contrast between the blood vessels and the surrounding tissue, blurred edge of calcified plaque, and a lack of discernability between the stent and in-stent lumen.
Statistical analysis
SPSS 21.0 (IBM Corp, Armonk, NY, USA) was used for data analysis. The measurement data are expressed as the mean ± standard deviation. The data were tested for normality using the Kolmogorov-Smirnov test. Continuous data following normal distribution from the 3 reconstruction groups were compared using repeated-measures analysis of variance (ANOVA), and post hoc pairwise comparisons were adjusted for multiple comparisons with the Bonferroni correction. The image quality scores that were ordinal and continuous data and did not follow the normal distribution were tested using Friedman test. A P value <0.05 was considered statistically significant.
Results
The image quality of the 3 reconstruction modes met the diagnostic requirements with scores greater or equal to 3. The mean subjective evaluation scores were 4.27±0.63 for the SD-ASIR-V50% group, 3.90±0.64 for the HD-ASIR-V50% group, and 4.50±0.63 for the HD-DLIR-H group. The differences between the SD-ASIR-V50% and HD-DLIR-H groups and between the HD-ASIR-V50% and HD-DLIR-H groups were all statistically significant (all P<0.05). Interobserver agreement of the image quality assessment was good to excellent (HD-DLIR-H: κ value =0.783; HD-ASIR-V50%: κ value =0.789; SD-ASIR-V50%: κ value =0.671).
The CT value, noise, SNR, and CNR of the aorta of the 3 reconstruction modes were compared. The HD-DLIR-H group had the lowest image noise and highest SNR and CNR values (Table 2). The HD-DLIR-H group also had the smallest calcified plaque diameter measurement of 2.59±1.87 mm, followed by the HD-ASIR-V50% group (3.46±2.07 mm) and the SD-ASIR-V50% group (4.06±2.49 mm; Figure 1). The CT values of the 3 points in the lumen among the stent in the HD-DLIR-H group were also the closest (Figure 2). The SD-ASIR-V50% had the highest in-stent lumen CT values, indicating that HD-DLIR-H images had the highest spatial resolution and the smallest beam-hardening artifacts.
Table 2
Variable | SD-ASIR-V50% | HD-ASIR-V50% | HD-DLIR-H | P value |
---|---|---|---|---|
CT value of RCA | 416.22±36.34 | 398.97±61.67 | 384.66±23.79 | >0.05 |
CT value of LAD | 441.04±37.43 | 486.42±53.67 | 382.46±21.07 | >0.05 |
CT value of LCX | 420.14±38.66 | 442.16±62.40 | 370.13±22.54 | >0.05 |
Aorta (HU) | 482.99±32.65 | 484.27±53.05 | 464.79±22.97 | >0.05 |
Aorta noise (HU) | 35.02±8.09+ | 57.7±12.03+ | 22.59±3.59*° | <0.001 |
Signal-to-noise ratio | 12.77±1.59+ | 8.16±1.86+ | 18.3±4.88*° | <0.001 |
Contrast-to-noise ratio | 15.67±1.92+ | 10.01±2.39+ | 26.56±6.33*° | <0.001 |
Date are presented as the mean ± standard deviation. Post-hoc pairwise comparison with Bonferroni adjustment for multiple testing reveals significant mean differences from SD with 50% ASIR-V (SD-ASIR-V50%) (*), HD with 50% ASIR-V (HD-ASIR-V50%) (°), and HD with high-level DLIR (HD-DLIR-H) (+) (P<0.05). ASIR-V, adaptive statistical iterative reconstruction-V; CT, computed tomography; DLIR, deep learning image reconstruction; HD, high definition; HU, Hounsfield unit; LAD, left anterior descending; LCX, left circumflex; RCA, right coronary artery; SD, standard definition.

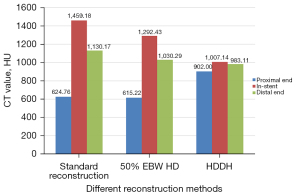
Discussion
In this study, coronary CTA was carried out in HD scanning mode but with different reconstruction modes (SD vs. HD) and algorithms (ASIR-V vs. DLIR) to compare the effects on image noise, spatial resolution, and overall image quality. By measuring image noise, calcification size, and CT value differences among the in-stent lumen, we demonstrated that combining the HD scan and reconstruction with a newly developed DLIR algorithm could increase the resolution enabled by the HD scanning mode while containing or even reducing image noise in coronary CTA.
Coronary CTA has been widely used in the clinic because it has rapid imaging speed, less trauma, and high sensitivity and specificity in diagnosing CAD (22). However, due to the limitation of spatial resolution, the beam-hardening artifacts caused by objects with a high atomic number and the partial volume effect still affect the display of calcified plaques, stents, and the in-stent lumen, resulting in inaccurate evaluation of in-stent lumen restenosis (23). Coronary CTA image spatial resolution may be increased by using HD scanning and the HD reconstruction mode. However, the use of HD scanning and reconstruction modes typically also significantly increases the image noise, which may have a negative impact on image quality and diagnostic accuracy. For this reason, it is not routinely used in coronary CTA (10). IRs are often used to reduce image noise, but their noise reduction power is limited due to the risk of generating images with unnatural image textures when high-strength IR is used (24). DLIR was developed to overcome the shortcomings of IR algorithms by using a brand-new approach based on a deep convolutional neural network and trained using standard-dose, high-quality FBP images, which, in theory, can provide powerful noise reduction capabilities while maintaining the high spatial resolution of detailed structures (25). Benz et al. (7) reconstructed images using ASIR-V 70% with SD and HD kernels and DLIR with an HD kernel at moderate and high levels to evaluate image noise, image quality, and coronary artery stenosis. They compared the diagnostic accuracy with that of ICA. Their results indicated that, compared with ASIR-V, DLIR significantly reduced image noise in coronary CTA and produced superior image quality at the same diagnostic accuracy. In our study, we extended the work by Benz et al. (7) by investigating the feasibility of combining the HD scanning mode and HD reconstruction mode with the DLIR algorithm to realize the competing goals in coronary CTA of increasing spatial resolution while reducing image noise. We focused our study on coronary CTA patients with calcifications and stents. We did not directly measure the image spatial resolution in our study. Instead, we used the size measurement of calcifications and CT number uniformity along the stented lumen to reflect the system spatial resolution and the degree of beam-hardening artifacts caused by the dense materials. Spatial resolution can be measured using the point spread function (PSF); the smaller the PSF is, the better the spatial resolution. Therefore, the apparent diameter of the calcified plaques in images is closely related to the spatial resolution. When the spatial resolution is insufficient, the boundary of the objects in images is not sufficiently clear. Lower spatial resolution causes more blurring of the boundaries and makes objects appear larger than they really are. Therefore, the measured size of dense and small objects can be an indicator of the spatial resolution of imaging systems. In addition, high-density objects, such as stents, cause more beam-hardening artifacts under lower spatial resolutions, and beam-hardening artifacts alter the CT values. Therefore, the smaller differences between the in-stent and out-stent (which has no beam-hardening artifacts) CT value measurements demonstrate fewer blurred artifacts from the stents and higher image spatial resolution. Our results indicated that, when the high-resolution scanning mode was adopted, the spatial resolution was improved. Calcifications were more concentrated to yield smaller sizes in images. The in-stent lumen had less contamination from the stents to have similar CT attenuation values as the lumen sections just outside the stents. The in-stent lumen was also better visualized with an HD scan and reconstruction mode with the DLIR algorithm. When the HD reconstruction mode was used with the current ASIR-V algorithm, image noise was significantly increased, resulting in lower overall image quality. However, the use of HD reconstruction with DLIR significantly suppressed image noise while maintaining higher spatial resolution. Our results indicated that the image noise in HD-DLIR-H images was even lower than that of SD-ASIR-V, suggesting further dose reduction is feasible in coronary CTA with DLIR in the future. In addition, Sun et al. (15) in their feasibility study of calcium defects in coronary CTA in the field of artificial intelligence [Enhanced Superresolution Generative Adversarial Network (ESRGAN)], demonstrated the impact of improved image spatial resolution on the diagnostic accuracy for patients with calcifications. Their results showed that ESRGAN-processed images inhibited the blooming artifacts associated with severe coronary artery calcification and improved the specificity and positive predictive values.
There were still some limitations in this study. First, due to the small number of stents included in our study, a detailed analysis using the stent as an important influencing factor could not be carried out, and this needs to be further explored in the future. Second, coronary angiography was not included in the study as the gold standard, and we did not evaluate the diagnostic accuracy of different approaches. Further research is needed to compare the diagnostic efficacy in the future.
In conclusion, coronary CTA with the HD scanning mode together with HD reconstruction using DLIR-H significantly improved the spatial resolution for displaying calcifications and the in-stent lumen while reducing image noise and improving overall image quality.
Acknowledgments
Funding: This work was supported by the Key R&D Program of Shaanxi Province Universities and Colleges (No. 2020GXLH-Y-026), the 3D Printing Medical Research Funding Project of the First Affiliated Hospital of Xi’an Jiaotong University (No. XJTU1AF-3D-2018-003), and the Science Development Foundation of the First Affiliated Hospital of Xi’an Jiaotong University (No. 2018MS-27).
Footnote
Reporting Checklist: The authors have completed the GRRAS reporting checklist. Available at https://qims.amegroups.com/article/view/10.21037/qims-22-186/rc
Conflicts of Interest: All authors have completed the ICMJE uniform disclosure form (available at https://qims.amegroups.com/article/view/10.21037/qims-22-186/coif). The authors have no conflicts of interest to declare.
Ethical Statement: The authors are accountable for all aspects of the work in ensuring that questions related to the accuracy or integrity of any part of the work are appropriately investigated and resolved. The study was conducted in accordance with the Declaration of Helsinki (as revised in 2013). This study was approved by our institutional review committee, and all patients or their family members signed informed consent for undergoing coronary CTA. As a retrospective study, the requirement for a signed consent form for using the images was waived due to the retrospective nature of this study.
Open Access Statement: This is an Open Access article distributed in accordance with the Creative Commons Attribution-NonCommercial-NoDerivs 4.0 International License (CC BY-NC-ND 4.0), which permits the non-commercial replication and distribution of the article with the strict proviso that no changes or edits are made and the original work is properly cited (including links to both the formal publication through the relevant DOI and the license). See: https://creativecommons.org/licenses/by-nc-nd/4.0/.
References
- He Z, Zhang JY, Fei F, Xu M, Li Y, Deng X, Wan YD. Initial study about image quality of small branches in coronary CTA: using different reconstruction algorithm by high definition CT. Journal of China Clinic Medical Imaging 2016;27:634-9.
- Nakamura Y, Asaumi Y, Miyagi T, Nakai M, Nishimura K, Sugane H, Matama H, Kataoka Y, Miyamoto Y, Takeishi Y, Noguchi T, Yasuda S. Comparison of Long-Term Mortality in Patients With Previous Coronary Artery Bypass Grafting Who Underwent Percutaneous Coronary Intervention With Versus Without Optimal Medical Therapy. Am J Cardiol 2018;122:206-12. [Crossref] [PubMed]
- Liu CY, Tang CX, Zhang XL, Chen S, Xie Y, Zhang XY, Qiao HY, Zhou CS, Xu PP, Lu MJ, Li JH, Lu GM, Zhang LJ. Deep learning powered coronary CT angiography for detecting obstructive coronary artery disease: The effect of reader experience, calcification and image quality. Eur J Radiol 2021;142:109835. [Crossref] [PubMed]
- Rudziński PN, Kruk M, Demkow M, Oleksiak A, Schoepf JU, Mach M, Dzielińska Z, Pręgowski J, Witkowski A, Rużyłło W, Kępka C. Efficacy and safety of coronary computed tomography angiography in patients with a high clinical likelihood of obstructive coronary artery disease. Kardiol Pol 2022;80:56-63. [Crossref] [PubMed]
- Rudziński PN, Kruk M, Kępka C, Schoepf UJ, Duguay T, Dzielińska Z, Pręgowski J, Witkowski A, Rużyłło W, Demkow M. The value of Coronary Artery computed Tomography as the first-line anatomical test for stable patients with indications for invasive angiography due to suspected Coronary Artery Disease: CAT-CAD randomized trial. J Cardiovasc Comput Tomogr 2018;12:472-9. [Crossref] [PubMed]
- Kruk M, Rudziński PN, Demkow M, Kępka C. Is the Majority Benefitting at the Costs of the Minority Among Patients Undergoing CTA as the First-Line Diagnostic in Highly Suspected Coronary Artery Disease? JACC Cardiovasc Imaging 2019;12:944. [Crossref] [PubMed]
- Benz DC, Benetos G, Rampidis G, von Felten E, Bakula A, Sustar A, Kudura K, Messerli M, Fuchs TA, Gebhard C, Pazhenkottil AP, Kaufmann PA, Buechel RR. Validation of deep-learning image reconstruction for coronary computed tomography angiography: Impact on noise, image quality and diagnostic accuracy. J Cardiovasc Comput Tomogr 2020;14:444-51. [Crossref] [PubMed]
- Tanaka R, Yoshioka K, Muranaka K, Chiba T, Ueda T, Sasaki T, Fusazaki T, Ehara S. Improved evaluation of calcified segments on coronary CT angiography: a feasibility study of coronary calcium subtraction. Int J Cardiovasc Imaging 2013;29:75-81. [Crossref] [PubMed]
- Motoyama S, Ito H, Sarai M, Nagahara Y, Miyajima K, Matsumoto R, Doi Y, Kataoka Y, Takahashi H, Ozaki Y, Toyama H, Katada K. Ultra-High-Resolution Computed Tomography Angiography for Assessment of Coronary Artery Stenosis. Circ J 2018;82:1844-51. [Crossref] [PubMed]
- Cui X, Li T, Li X, Zhou W. High-definition computed tomography for coronary artery stents imaging: Initial evaluation of the optimal reconstruction algorithm. Eur J Radiol 2015;84:834-9. [Crossref] [PubMed]
- Yu L, Li H, Fletcher JG, McCollough CH. Automatic selection of tube potential for radiation dose reduction in CT: a general strategy. Med Phys 2010;37:234-43. [Crossref] [PubMed]
- Singh R, Digumarthy SR, Muse VV, Kambadakone AR, Blake MA, Tabari A, Hoi Y, Akino N, Angel E, Madan R, Kalra MK. Image Quality and Lesion Detection on Deep Learning Reconstruction and Iterative Reconstruction of Submillisievert Chest and Abdominal CT. AJR Am J Roentgenol 2020;214:566-73. [Crossref] [PubMed]
- Willemink MJ, Noël PB. The evolution of image reconstruction for CT-from filtered back projection to artificial intelligence. Eur Radiol 2019;29:2185-95. [Crossref] [PubMed]
- Yi Y, Xu C, Xu M, Yan J, Li YY, Wang J, Yang SJ, Guo YB, Wang Y, Li YM, Jin ZY, Wang YN. Diagnostic Improvements of Deep Learning-Based Image Reconstruction for Assessing Calcification-Related Obstructive Coronary Artery Disease. Front Cardiovasc Med 2021;8:758793. [Crossref] [PubMed]
- Sun Z, Ng CKC. Artificial Intelligence (Enhanced Super-Resolution Generative Adversarial Network) for Calcium Deblooming in Coronary Computed Tomography Angiography: A Feasibility Study. Diagnostics (Basel) 2022.
- Sun Z, Ng CKC. High calcium scores in coronary CT angiography: Effects of image post-processing on visualization and measurement of coronary lumen diameter. J Med Imaging Health Inf 2015;5:110-6. [Crossref]
- Sun Z, Ng CKC, Xu L, Fan Z, Lei J, Coronary CT. Angiography in Heavily Calcified Coronary Arteries: Improvement of Coronary Lumen Visualization and Coronary Stenosis Assessment With Image Postprocessing Methods. Medicine (Baltimore) 2015;94:e2148. [Crossref] [PubMed]
- Liu J, Zhang Y, Zhao Q, Lv T, Wu W, Cai N, Quan G, Yang W, Chen Y, Luo L, Shu H, Coatrieux JL. Deep iterative reconstruction estimation (DIRE): approximate iterative reconstruction estimation for low dose CT imaging. Phys Med Biol 2019;64:135007. [Crossref] [PubMed]
- Hsieh J, Liu E, Nett B, Tang J, Thibault J, Sahney S. A new era of image reconstruction: TrueFidelityTM Technical white paper on deep learning image reconstruction. White paper (JB68676XX). GE Healthcare; 2019.
- Greffier J, Hamard A, Pereira F, Barrau C, Pasquier H, Beregi JP, Frandon J. Image quality and dose reduction opportunity of deep learning image reconstruction algorithm for CT: a phantom study. Eur Radiol 2020;30:3951-9. [Crossref] [PubMed]
- Li W, Diao K, Wen Y, Shuai T, You Y, Zhao J, Liao K, Lu C, Yu J, He Y, Li Z. High-strength deep learning image reconstruction in coronary CT angiography at 70-kVp tube voltage significantly improves image quality and reduces both radiation and contrast doses. Eur Radiol 2022;32:2912-20. [Crossref] [PubMed]
- Siegrist PT, Sumitsuji S, Kumada M, Kaneda H, Tachibana K, Nanto S. Contrast-free diagnosis and treatment of coronary artery disease guided by integrated cardiac imaging: concept and first clinical experience. Cardiovasc Interv Ther 2016;31:51-5. [Crossref] [PubMed]
- Tan S, Xu Z. Intelligent Algorithm-Based Multislice Spiral Computed Tomography to Diagnose Coronary Heart Disease. Comput Math Methods Med 2022;2022:4900803. [Crossref] [PubMed]
- Noid G, Tai A, Li X. SU-E-I-04: Improving CT Quality for Radiation Therapy of Patients with High Body Mass Index Using Iterative Reconstruction Algorithms. Medical Physics 2015;42:3242. [Crossref]
- Akagi M, Nakamura Y, Higaki T, Narita K, Honda Y, Zhou J, Yu Z, Akino N, Awai K. Deep learning reconstruction improves image quality of abdominal ultra-high-resolution CT. Eur Radiol 2019;29:6163-71. [Crossref] [PubMed]