Using radiomics based on multicenter magnetic resonance images to predict isocitrate dehydrogenase mutation status of gliomas
Introduction
Glioma is the most common primary brain tumor, accounting for about 80% of malignant brain tumors (1). One of the most significant discoveries in brain glioma biology has been the identification of isocitrate dehydrogenase (IDH) mutation status as a biomarker for therapy and prognosis (2). Moreover, the 2021 World Health Organization (WHO) Classification of Tumors of the Central Nervous System adapted these molecular markers into the revised grading criteria of IDH mutant and IDH wild-type gliomas as a grading system within tumor types (3). Patients with IDH mutant gliomas and IDH wild-type gliomas have different treatments and prognostic performances. IDH wild-type gliomas are heterogeneous tumors that are more aggressive and infiltrative than are IDH mutant gliomas (4). Several studies reported that patients with IDH wild-type gliomas have a better response to chemoradiation therapy and longer overall survival than do those with IDH mutant gliomas (5,6). Moreover, patients with IDH mutant glioma have better prognoses than do those with IDH wild-type gliomas (7). Therefore, determining the mutation status of IDH before surgery is beneficial to implementing targeted therapy for patients with gliomas (8). Identifying the IDH mutation status becomes even more important for patients with brain tumors who cannot have a biopsy due to the high risk of injury.
Currently, the only way to definitively identify the IDH mutation status of gliomas is based on immunohistochemistry (IHC) or gene sequencing on tissue specimens obtained via biopsy or surgical resection. However, repeated invasive biopsies or surgical resections are not practical or feasible in clinical practice considering the tolerance of patients. Magnetic resonance (MR) spectroscopy can potentially be used to determine IDH mutation status. Mutations in IDH result in neomorphic activity of the enzyme catalyzing the production of the oncometabolite 2-hydroxyglutarate (2-HG) from alpha-ketoglutarate (alpha-KG) (9). MR spectroscopic methods have been developed to noninvasively identify 2-HG in gliomas (10-12). However, accurate detection of the 2-HG concentration by MR spectroscopy is highly dependent on the magnetic field intensity, and high false positivity rates and costs limit its clinical application (13). Conventional MR is noninvasive, is widely used in the preoperative clinical diagnosis of glioma, and can reflect the overall information of the tumor. However, MR images cannot objectively reflect the physiological and pathological characteristics of the tumors, and the results are easily affected by subjective and experience factors. “Radiomics” refers to the extraction and analysis of large amounts of advanced quantitative imaging features with high throughput from radiological images obtained with computed tomography, positron emission tomography, or MR imaging (MRI) (14). Unlike conventional MR images, radiomics can transform the subjective descriptions of traditional imaging diagnosis, such as tumor range, tumor size, and tumor location, into objective and quantitative parameters, such as histogram features and texture features (15,16), which can better reflect glioma heterogeneity. The appropriate machine learning models can be further constructed based on the selected radiomics features to achieve a remarkable prediction performance.
Radiomics features involve the extraction of predefined features, such as shape, intensity, and texture, from the segmented volumes of interest (VOI) (17). Past work has attempted to correlate radiomics features with predicting IDH mutation. For example, Gihr et al. (18) determined the relation of intensity features to IDH status, which found apparent diffusion coefficient (ADC) histogram-profiling could help differentiate tumor grade and estimate growth kinetics and probably prognostic relevant genetic as well as epigenetic alterations in low-grade gliomas. Jakola et al. (19) determined the feasibility of texture features to predict IDH status on fluid-attenuated inversion recovery (FLAIR). They found that homogeneity and volume could classify IDH status with an area under the curve (AUC) of 0.940 using the generalized linear model. Singh et al. (20) elucidated novel radiomic and radiogenomic workflow concepts and state-of-the-art descriptors in subvisual MR image processing, with relevant literature on applications of such machine learning techniques in glioma management. Radiomics application to preoperative MR images demonstrated promising results for predicting IDH mutation, methylguanine-methyltransferase (MGMT) methylation, and 1p/19q codeletion in glioma (21). The integrated study of data from radiographical and genomic scales was termed radiogenomic. Corr et al. (22) provided a broad and informative state-of-art picture and illustrated the latest developments in radiogenomic markers regarding prognosis. Radiomics could lead to a noninvasive, objective tool that captures molecular information important for clinical decision-making. Considering that MR image intensities seem to be sensitive to different protocols and machines, the effectiveness of radiomics models should be verified using multicenter data. Future studies should use multicenter data and external validation and investigate the clinical feasibility of radiomics models.
The aim of this multicenter study was to predict the IDH status of glioma patients using 4 preoperative routine MR modalities images (including T1C, T2, T1 FLAIR, and T2 FLAIR) using machine learning-based radiomics models. Meanwhile, we also explored the predictive performance using each of the 4 MR modalities. We present the following article in accordance with the STARD reporting checklist (available at https://qims.amegroups.com/article/view/10.21037/qims-22-836/rc).
Methods
Study design
The study was conducted in accordance with the Declaration of Helsinki (as revised in 2013). This study was approved by and registered with Shandong Provincial Hospital Involves the Ethics Committee of Biomedical Research on Humans (SWYX: No. 2021-470). Individual consent for this retrospective analysis was waived.
In this section, we first describe the patients used in our present work. Then, we introduce the 5 steps of our research in detail. Figure 1 shows the overall workflow of this paper, which includes 5 parts: (I) image acquisition; (II) VOI specification; (III) radiomics feature extraction; (IV) model training; and (V) performance testing.
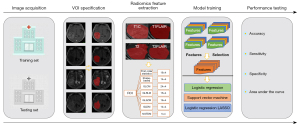
Patients
The multimodal MR images of patients with gliomas were collected from Shandong Provincial Hospital (SPH) and The Cancer Genome Atlas (TCGA) (23). Participants from SPH and TCGA formed a consecutive and random series. The flow diagram of the study population is shown in Figure 2. A total of 174 patients who underwent preoperative MRI for newly diagnosed gliomas from January 2018 to December 2019 at SPH were considered for inclusion. Meanwhile, we downloaded 128 MR images of patients with glioma from TCGA data set and performed the same screening criteria as for the patients from SPH. In our study, the time interval between MR and pathology was 2 weeks because if the time interval was shorter than this, the mutation status of IDH would have hardly changed. The inclusion criteria were as follows: (I) gliomas confirmed by pathology; (II) known IDH mutation status; (III) preoperative MR protocol, including T1C, T2, T1 FLAIR, and T2 FLAIR images; and (IV) age over 18 years. The exclusion criteria were as follows: (I) history of biopsy or surgery for a brain tumor; (II) the absence of T1C, T2, T1 FLAIR, or T2 FLAIR; (III) unknown IDH status; and (IV) age under 18 years.
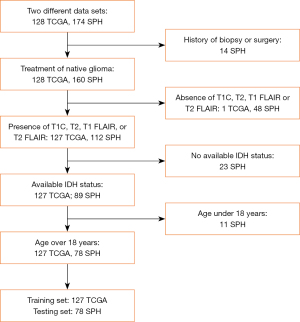
Finally, 78 patients (43 males and 35 females; mean age 51.06±13.42 years; range, 18–79 years; 38 IDH-mutated, 40 IDH wild-type) from SPH met the inclusion criteria. All diagnoses were histopathologically proven after surgical resection or tumor biopsy according to the 2016 WHO Classification of Tumors of the Central Nervous System tumors by neuropathologists who were blinded to the MR images and the patients’ clinical information. These patients were allocated to the testing set. In addition, a total of 127 patients (66 males and 61 females; mean age 51.30±15.39 years; range, 18–84 years; 52 IDH-mutated, 75 IDH wild-type) met the inclusion criteria from TCGA and were used as the training set. The clinical characteristics of all the selected patients from SPH and TCGA are summarized in Table 1. The IDH mutation type was marked as 1, and the wild-type was marked as 0.
Table 1
Type | SPH (n=78) | TCGA (n=127) | P value (statistical method) |
---|---|---|---|
Age (years), mean ± SD | 51.06±13.42 | 51.30±15.39 | 0.77 (Mann-Whitney) |
Sex, n (%) | 0.67 (chi-squared) | ||
Male | 43 (55.13) | 66 (51.97) | |
Female | 35 (44.87) | 61 (49.03) | |
IDH status, n (%) | 0.31 (chi-squared) | ||
Wild-type | 40 (51.28) | 75 (59.06) | |
Mutation | 38 (48.72) | 52 (40.94) | |
WHO grade, n (%) | 0.001 (Kruskal-Wallis) | ||
II | 33 (42.31) | 28 (22.05) | |
III | 19 (24.36) | 30 (23.62) | |
IV | 26 (33.33) | 69 (54.33) |
SPH, Shandong Provincial Hospital; TCGA, The Cancer Genome Atlas; SD, standard deviation; IDH, isocitrate dehydrogenase; WHO, World Health Organization.
Image acquisition and VOI specification
The patients came from 2 centers, and the MR image acquisition from these centers had different parameters. All patients from SPH were examined in a supine position with a 3.0 T MR machine (Magnetom Skyra, Siemens Healthineers, Erlangen, Germany) using a transmit/receive quadrature 16-channel head and neck combined coil. The detailed parameters of T1C, T2, T1 FLAIR, and T2 FLAIR were as follows: T1C—repetition time/time to echo (TR/TE) 2,300/2.3 ms, inversion time (TI) 900 ms, field of view (FOV) 240 mm × 240 mm, slice thickness 1 mm, flip angle (FA) 8°, and 192 slices; T2—TR/TE 3,700/109 ms, FOV 220 mm × 220 mm, slice thickness 5 mm, FA 150°, and 19 slices; T1 FLAIR—TR/TE 1,820/13 ms, FOV 230 mm × 230 mm, slice thickness 5 mm, FA 150°, and 19 slices; and T2 FLAIR—TR/TE 8,000/81 ms, FOV 220 mm × 220 mm, slice thickness 5 mm, and FA 150°, and 18 slices.
For the data from TCGA, the VOIs were specified. The VOIs for data from SPH were defined as the ones that covered the tumor area, which was delineated on the 4 modalities with 3 dimensions. T2, T2 flair, and T1 FLAIR were registered to T1C, and the segmentation was performed manually on postcontrast T1C images and then applied to the registered T2, T2 FLAIR, and T1 FLAIR. Manual segmentation was performed by a radiologist with 15 years of experience in neuroradiology, and the results were confirmed by another radiologist with 10 years of experience in neuroradiology using the noncommercial software ITK-SNAP (version 3.6.0; http://www.itksnap.org). A consensus was reached after a careful discussion if divergence existed. Only the whole tumors, including both enhancing and necrotic areas, were covered for segmentation, and adjacent vessels were avoided. Any tumors that occupied over 2 axial slices were segmented according to their size.
Radiomics features extraction
Before features were extracted, MR images of 4 modalities were preprocessed using N4 bias correction to remove radiofrequency inhomogeneity (24) and intensity normalization to the zero-mean and unit variance.
Then, we extracted 7 types of features from each MR image’s modality (25): (I) 18 first-order statistics; (II) 14 three-dimensional (3D) shape-based features; (III) 24 gray-level co-occurrence matrices (GLCM); (IV) 16 gray-level run length matrixes (GLRLM); (V) 16 gray-level size zone matrices (GLSZM); (VI) 14 neighboring gray-tone difference matrices (NGTDM); and (VII) 5 gray-level dependence matrices (GLDM). Since 4 different modalities were involved in the present work, a total of 428 radiomics features were extracted for each patient.
It should be noted that the sample contained more patients with IDH wild-type than those with IDH mutant type, which led to an imbalanced data set in machine learning. To correct the imbalance of the training data set, we adopted the synthetic minority over-sampling technique (SMOTE) to balance the positive and negative samples (26). The idea of SMOTE can be summarized as interpolating between minority samples to generate additional samples. In detail, the training data set was divided into a majority sample set Tmaj and a minority sample set Tmin. We randomly selected a sample named X from Tmin. The K-nearest neighbor method was used to find the K samples closest to X in Tmin, where the distance was defined as the Euclidean distance between the samples in the feature space. Then, one of the K neighboring samples, Xk, was randomly selected, and the following formula was used to generate a new sample Xnew:
Where δ is a random number between 0 and 1. We repeated the above steps to bring the data into balance.
The distribution of feature values may be inconsistent. Different radiomics features may have different units and ranges depending on the distribution of feature values, and some features might be given a larger weight compared to others (27). To avoid the occurrence of the above situation, we applied z-score normalization to the feature values, making the range of each feature relatively uniform (28).
Model training
As shown in Figure 1, the model training phase was divided into 2 steps: feature selection processing and the classification phase.
To select the most discriminative features, we calculated the Pearson correlation coefficient (PCC) for the feature pair to compare the similarity (29). We randomly eliminated 1 of them if the PCC value of the feature pair was larger than 0.99. After this process, the dimension of the feature space was reduced, and each feature was independent of the other. After reducing the dimensionality of the feature value, we used recursive feature elimination (RFE) to select features (30). The goal of RFE was to select features based on a classifier by recursively considering a smaller set of features, and the weight of each feature remained consistent. In this way, the dimension of features was constantly reduced.
With the selected key features, we adopted 3 classifiers, namely logistic regression (LR) (31), support vector machine (SVM) (32), and LR least absolute shrinkage and selection operator (LASSO) (33), along with the trial and error method, to generate predictive models of IDH mutation status based on 4-modality MR images. LR classifier used the linear combination of features as an independent variable and a logistic function to map the independent variable to [0, 1]. The SVM algorithm was trained to maximize the margins that separated values belonging to the 2 categories in the feature space. It is one of the most commonly used classifiers for classification in machine learning. LR LASSO adds an L1-norm term based on standard linear regression, which, in this study, helped to alleviate overfitting by thinning model parameters.
Moreover, to exploit the use of each modality of MR images, we constructed the models corresponding with T1C, T2, T1 FLAIR, and T2 FLAIR. For each model, we repeated the process several times in the training cohort, which was randomly divided into 5 folds. Then, the best number of features and parameters for the models with the maximum mean cross-validation AUC for the classification of the IDH mutation status were adopted.
Performance testing
In order to demonstrate the model performance using our multicenter MR radiomics in predicting IDH mutation status, we calculated their accuracy (ACC), sensitivity (SEN), specificity (SPEC), and AUC, and drew the receiver operating characteristic (ROC) curves based on Python.
In addition, we calculated the evaluation metrics of ACC, SEN, SPEC, and AUC, and drew ROC curves to evaluate whether the image-fusion model that used the 4-modalility MR images could predict IDH status more accurately than could each modality MR image alone.
Results
Construction of the radiomic signature
For each patient’s MR sequence (T1C, T2, T1 FLAIR, and T2 FLAIR), the number of extracted radiomics features totaled 428, and 107 features were extracted from each MR sequence. Subsequently, the PCC and RFE algorithms were adopted to select useful features. As shown in Figure 3, to select the appropriate number of features to interpret glioma characteristics, we conducted multiple classification experiments with different numbers of features (range, 1–40; interval 1) combined with different classifiers (i.e., LR, LR LASSO, and SVM) with an idiomatic model optimization method. In addition, we found that when the feature number was set to 24, the ACC evaluation metric with different classifiers showed a better classification performance compared with other feature number settings. Hence, the number of selected features was set to 24 in this study. In addition, in order to better understand the source of the selected features in the selected 24 features, we visualized the weights and sources of the selected features, as shown in Figure 4. We found that 10 features, 7 features, 4 features, and 3 features were from T2, TIC, T1 flair, and T2 FLAIR MR sequences, respectively. Furthermore, the weight of each MR sequence was generally balanced, which verified the complementary role of the multimodality sequence in predicting IDH mutation status of gliomas.
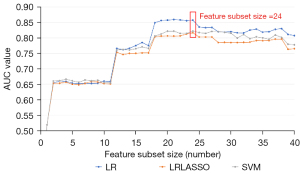
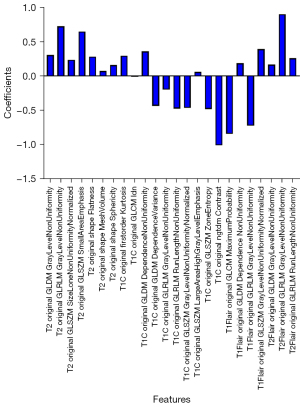
Generally, results from multiple experiments we performed proved that the multimodality features based on radiomics were essential. A better classification result could be obtained when the feature number was set to 24 compared with the other number settings.
Model training and validation
After the feature selection phase, a total of 24 radiomics features were obtained. These features were regarded as the input for 3 different classifiers to obtain the classification performance, along with the 5-fold cross-validation method. After classification performance the training phase, the trained model was verified using the testing data set.
First, based on the classification performance results, the evaluation metrics of ACC, SEN, SPEC, and AUC were obtained. Based on these, the cross-validation performance combined with 3 baseline classifiers was obtained and is shown in Table 2. Figure 5 shows the ROC of the training set and the testing set of the 3 classifiers. The selected feature vector of multimodality MR images combined with the 3 baseline classifiers (LR/SVM/LR LASSO) presented good classification results (ACC: 0.91/0.91/0.90; SEN: 0.8462/0.8462/0.9423; SPEC: 0.96/0.96/0.8667; AUC: 0.9541/0.9513/0.9574) in the model training phase. The metrics of ACC and AUC of the 3 classifiers in the model training groups were all greater than 0.9, which indicated that the classifiers were successful in the model training groups. The LR classifier model had the best training performance among the 3 classifier models. In addition, the average cross-validation of the LR, SVM, and LR LASSO classifiers was 0.894, 0.876, and 0.888, respectively, which meant that there was less chance of overfitting during the model training phase.
Table 2
Type | Classifier | ACC | SEN | SPEC | AUC | 95% CI |
---|---|---|---|---|---|---|
Multimodality | LR | 0.9100/0.8346 | 0.8462/0.8077 | 0.9600/0.8533 | 0.9541/0.8941 | 0.9134–0.9872/0.8311–0.9486 |
SVM | 0.9100/0.8346 | 0.8462/0.7308 | 0.9600/0.9067 | 0.9513/0.8762 | 0.9087–0.9856/0.8055–0.9372 | |
LR LASSO | 0.9000/0.8583 | 0.9423/0.8077 | 0.8667/0.8933 | 0.9574/0.8879 | 0.9168–0.9890/0.8194–0.9451 | |
T1C | LR | 0.8819/0.8583 | 0.9231/0.7692 | 0.8533/0.9200 | 0.9487/0.8931 | 0.9045–0.9839/0.8291–0.9508 |
SVM | 0.9055/0.8268 | 0.8462/0.7308 | 0.9467/0.8933 | 0.9497/0.8556 | 0.9048–0.9855/0.7789–0.9247 | |
LR LASSO | 0.8898/0.8661 | 0.9036/0.7500 | 0.8800/0.9467 | 0.9508/0.8813 | 0.9090–0.9855/0.8119–0.9436 | |
T1 FLAIR | LR | 0.8661/0.8189 | 0.7692/0.7692 | 0.9333/0.8533 | 0.9185/0.8644 | 0.8858–0.9512/0.7907–0.9344 |
SVM | 0.8819/0.8189 | 0.7885/0.7500 | 0.9467/0.8667 | 0.9146/0.8564 | 0.8871–0.9421/0.7802–0.9278 | |
LR LASSO | 0.8740/0.8110 | 0.7500/0.7692 | 0.9600/0.8400 | 0.9190/0.8528 | 0.8702–0.9678/0.7732–0.9251 | |
T2 | LR | 0.8661/0.8346 | 0.7692/0.6923 | 0.9333/0.9333 | 0.9185/0.8354 | 0.8510–0.9669/0.7521–0.9179 |
SVM | 0.8819/0.8268 | 0.7885/0.7308 | 0.9467/0.8667 | 0.9146/0.8356 | 0.8556–0.9627/0.7530–0.9089 | |
LR LASSO | 0.8740/0.8110 | 0.7500/0.7308 | 0.9600/0.8933 | 0.9190/0.8382 | 0.8596–0.9648/0.7549–0.9158 | |
T2 FLAIR | LR | 0.8976/0.8346 | 0.7885/0.7308 | 0.9733/0.9067 | 0.9223/0.8631 | 0.8628–0.9737/0.7854–0.9326 |
SVM | 0.8976/0.8110 | 0.7885/0.7115 | 0.9733/0.8000 | 0.9200/0.8529 | 0.8624–0.9713/0.7729–0.9236 | |
LR LASSO | 0.8898/0.8425 | 0.7885/0.8269 | 0.9600/0.9333 | 0.9187/0.8556 | 0.8617–0.9688/0.7772–0.9289 |
Each performance value was calculated by averaging the results of the 5-fold cross-validation. The first and the second values in each column represent evaluation metrics using 3 baseline classifiers in the training and cross-validation phases, respectively. ACC, accuracy; SEN, sensitivity; SPEC, specificity; AUC, area under the curve; CI, confidence interval; LR, logistic regression; SVM, support vector machine; LASSO, least absolute shrinkage and selection operator; FLAIR, fluid-attenuated inversion recovery.
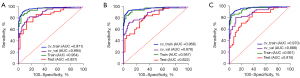
As mentioned above, the selected features combined with the LR classifier achieved good classification performance. Hence, for the single modal MR sequence, we compared the classification results obtained by the different MR sequences with the LR classifier. For the T1C, T1 FLAIR, T2, and T2 FLAIR MR sequence, the ACC metrics were 0.8819, 0.8661, 0.8661, and 0.8976, respectively; the SEN metrics were 0.9231, 0.7692, 0.7692, and 0.7885, respectively; and the SPEC metrics were 0.8533, 0.9333, 0.9333, and 0.9733, respectively. The value of the AUC metrics was 0.9487, 0.9185, 0.9185, and 0.9223, respectively. By comparing the experimental results, we found that the classification performance of multimodality MR sequences was better than that of any single modality.
The model we obtained was constructed based on optimal model parameters and radiomics features after feature selection. Table 3 shows the results obtained using test data to verify the validity of the model obtained in the training phase. The selected feature vector of multimodality MR images combined with the 3 baseline classifiers (LR/SVM/LR LASSO) had good classification results (ACC: 0.8077/0.8064/0.8041; SEN: 0.7368/0.8421/0.8947; SPEC: 0.875/0.675/0.625; AUC: 0.8572/0.8217/0.8164). As shown in Figure 5, the values of the AUC of the testing datasets of the 3 classifier models were all greater than 0.8, indicating that the constructed models still had a good ability to distinguish the testing sets of the different centers.
Table 3
Type | Classifier | ACC | SEN | SPEC | AUC | 95% CI |
---|---|---|---|---|---|---|
Multimodality | LR | 0.8077 | 0.7368 | 0.875 | 0.8572 | 0.7697–0.9314 |
SVM | 0.8064 | 0.8421 | 0.675 | 0.8217 | 0.7349–0.9125 | |
LR LASSO | 0.8041 | 0.8947 | 0.625 | 0.8164 | 0.7192–0.9000 | |
T1C | LR | 0.7564 | 0.8684 | 0.65 | 0.8086 | 0.6757–0.8692 |
SVM | 0.7308 | 0.8684 | 0.6 | 0.7763 | 0.6691–0.8703 | |
LR LASSO | 0.7308 | 0.8158 | 0.65 | 0.7987 | 0.6726–0.8684 | |
T1 FLAIR | LR | 0.6667 | 0.7105 | 0.625 | 0.6895 | 0.5522–0.7838 |
SVM | 0.6923 | 0.7105 | 0.675 | 0.6974 | 0.5313–0.7727 | |
LR LASSO | 0.6795 | 0.5789 | 0.775 | 0.6875 | 0.5461–0.7863 | |
T2 | LR | 0.7564 | 0.7368 | 0.775 | 0.7493 | 0.5789–0.8278 |
SVM | 0.6795 | 0.8421 | 0.525 | 0.7185 | 0.5688–0.8155 | |
LR LASSO | 0.7564 | 0.6579 | 0.85 | 0.7586 | 0.5985–0.8366 | |
T2 FLAIR | LR | 0.641 | 0.5526 | 0.725 | 0.6671 | 0.5304–0.7785 |
SVM | 0.641 | 0.6316 | 0.65 | 0.6743 | 0.5183–0.7647 | |
LR LASSO | 0.641 | 0.6316 | 0.65 | 0.6704 | 0.5292–0.7741 |
Each performance value was calculated on the test set. ACC, accuracy; SEN, sensitivity; SPEC, specificity; AUC, area under the curve; CI, confidence interval; LR, logistic regression; SVM, support vector machine; LASSO, least absolute shrinkage and selection operator; FLAIR, fluid-attenuated inversion recovery.
The 3 machine learning algorithms selected in this study reached an ACC of more than 80%, showing that radiomics could predict IDH mutation status effectively. Similar to the training phase, the best classifier performance in the testing phase was LR. After integrating the ROC image analyses of the 3 classifiers, we found that the classification performance of the LR model was higher than that of the other 2 models, and the AUCs of the 3 models were all higher than 0.8, indicating that radiomics had certain potential in predicting the IDH mutation of gliomas.
Discussion
IDH mutation status has become an important marker for the treatment strategy selection and prognosis evaluation of gliomas. In order to predict the IDH mutation status of gliomas, in this multicenter study, we proposed an optimal model integrating the radiomics features based on multimodality MR images.
First, the results showed that the model based on multimodality radiomic signatures after feature selection and 3 baseline classifiers had better classification performance than did the single-modality models. Second, the multimodality radiomic signatures combined with the LR classifier achieved optimal results. In addition, we found that the multimodality radiomic signatures for the feature selection phase demonstrated good differentiation efficacy with high ACC. Furthermore, the number of features was also validated. We conducted multiple classification experiments with different numbers of features (range, 1–40; interval 1) combined with different classifiers (i.e., LR, LR LASSO, and SVM) with an idiomatic model optimization method. Through weight scoring of the selected 24 features, we found that features of the 4 MR sequences had a balanced effect using weight visualization operation, which indicated that the information of the 4 MR sequences could complement each other to achieve high classification ACC.
Radiomics can extract potential radiomics feature parameters from preoperative MR images. It has been widely used in the diagnosis, treatment, and prognosis of glioma and has shown satisfactory diagnostic efficiency. The AUCs of the 3 classic radiomics models in this study were all greater than 0.8 with an ACC rate greater than 80%, which showed that the diagnostic performance was satisfactory. Our study verified that the radiomics model was competent in diagnosing preoperative gliomas. The results of this study showed that, based on the same MR images, different machine learning models had different diagnostic performances. Among them, the LR model based on multimodal images had the highest AUC of 0.8572 on the test data.
Regardless of the histological grade, the prognosis and treatment response of gliomas with IDH mutation was reported to be superior to IDH wild-type gliomas (34,35). This finding led to the IDH mutation status being adopted as a decisive marker for glioma classification in the updated fourth edition of the WHO Classification of Tumors of the Central Nervous System [2016].
Regardless of IDH status, maximum tumor resection has been the standard treatment for gliomas, but it was discovered that predicting IDH status before surgery could help in planning treatments, including surgery (36). This finding led to research on predicting the IDH status. In one study, the estimation of the extent of lesion enhancement in a qualitative manner with an visual assessment was prone to both intraobserver and interobserver variability (37). Another study found that radiomics could extract large amounts of quantitative features from imaging that could complement visual assessment, may information related to tumor heterogeneity and microenvironment, and was less susceptible to intraobserver and interobserver variability (38). Zhou et al. (39) compared the results of the same radiomics model using texture features and visually accessible rembrandt images (VASARI) annotations features for IDH status prediction. They found that the radiomics model with the texture feature achieved higher ACC than did the model using the VASARI features.
In the screening of radiomics features, histogram features and GLCM features accounted for a larger proportion. The possible reason for this was that the histogram and GLCM features were more related to the biological information of the tumor than were other features. In the comparison of histogram features, GLCM features, and GLRLM features, Ryu et al. (40) found that the entropy in GLCM features showed good diagnostic performance in distinguishing high- and low-grade gliomas and grade III and IV gliomas (AUC of 0.83 and 0.94, respectively), which was better than its histogram features and GLRLM features. Meanwhile, Qin et al. (41) found that entropy was significantly related to the glial fibrillary acidic protein (GFAP) expression of glioma cells, which is the specific marker related to the malignant degree of gliomas. It was further reported that as the expression of GFAP decreased, and the degree of tumor malignancy increases (42). This finding indicates that entropy could reflect the degree of tumor malignancy.
Recently, deep learning based on radiomics has become the preferred methodology to substantially improve the performance of existing machine learning algorithms and has shown good applied prospects in tumor diagnosis and prognosis prediction. For example, Park et al. (43) established a fully automated hybrid model for IDH status prediction that was based on two-dimensional (2D) tumor images and radiomic features from 3D tumor shape and loci. Their model achieved accuracies of 78.8–93.8% and an AUC of 0.86–0.96. Bangalore Yogananda et al. (44) developed a highly accurate, MRI-based, deep learning IDH classification network using only T2-weighted MR images. Their network achieved a mean cross-validation testing ACC of 97.4%, representing an important milestone toward clinical translation. Karabacak et al. (45) predicted the diagnostic performance of glioma IDH mutations by combining the Bayes theorem with deep learning. With a known pretest probability of 80.2%, the Bayes theorem yielded a posttest probability of 97.6% and 96.0% for a positive test and 27.0% and 30.6% for a negative test for the training sets and validation sets, respectively. Although deep learning based on radiomics has shown excellent performance for predicting IDH status, deep learning requires more training samples compared to conventional machine learning approaches. This issue is a challenge for practical use.
Radiomics has significant obstacles when it is applied to clinical implementation. First, powerful tumor segmentation is a major challenge for radiomics. Second, for radiomic features, a major cause of limited reproducibility is the lack of a standard method for the computation of intensity features. Therefore, radiomics has not been well applied to the clinical diagnosis of brain tumors. To generate convincing results regarding the potential clinical value of radiomics as a practical tool, we must consider larger patient cohorts and the variety of technical infrastructure at different centers. However, there are few relevant studies on the generalizability of radiomics models. The proof of generalizability must be carried out in a test set with a different similarity to the training set. In our study, we attempted to simulate the feasible clinical scenario in which a training model was developed on one data set and applied to data sets from another data center.
Our study had several limitations that could be improved in future research. First, this was a retrospective study with a relatively limited sample size. A larger dataset is necessary to improve the reliability and clinical application of this radiomics study. Second, the number of samples in the training set was not balanced, and the results may be biased. Third, we only explored the classification of gliomas according to IDH genotype, and for the sake of ACC, the effects of other molecular derivatives on gliomas should be explored.
Conclusions
We demonstrated that radiomics models could accurately predict IDH mutation status on different datasets from multiple centers. This means that the radiomics model has good stability and generalizability in clinical practice.
Acknowledgments
Funding: This work was supported by the National Nature Science Foundation of China (Nos. 61971413 and 62271481), the Youth Innovation Promotion Association of Chinese Academy of Sciences (CAS) (No. 2021324), the Jinan Innovation Team (No. 2018GXRC017), and the Taishan Scholars Project (No. tsqn201812147).
Footnote
Reporting Checklist: The authors have completed the STARD reporting checklist. Available at https://qims.amegroups.com/article/view/10.21037/qims-22-836/rc
Conflicts of Interest: All authors have completed the ICMJE uniform disclosure form (available at https://qims.amegroups.com/article/view/10.21037/qims-22-836/coif). The authors have no conflicts of interest to declare.
Ethical Statement: The authors are accountable for all aspects of the work in ensuring that questions related to the accuracy or integrity of any part of the work are appropriately investigated and resolved. The study was conducted in accordance with the Declaration of Helsinki (as revised in 2013). This retrospective study was approved by Shandong Provincial Hospital Involves the Ethics Committee of Biomedical Research on Humans (SWYX: No. 2021-470), and individual consent for this retrospective analysis was waived.
Open Access Statement: This is an Open Access article distributed in accordance with the Creative Commons Attribution-NonCommercial-NoDerivs 4.0 International License (CC BY-NC-ND 4.0), which permits the non-commercial replication and distribution of the article with the strict proviso that no changes or edits are made and the original work is properly cited (including links to both the formal publication through the relevant DOI and the license). See: https://creativecommons.org/licenses/by-nc-nd/4.0/.
References
- Filippini G. Epidemiology of primary central nervous system tumors. Handb Clin Neurol 2012;104:3-22. [Crossref] [PubMed]
- Louis DN, Perry A, Reifenberger G, von Deimling A, Figarella-Branger D, Cavenee WK, Ohgaki H, Wiestler OD, Kleihues P, Ellison DW. The 2016 World Health Organization Classification of Tumors of the Central Nervous System: a summary. Acta Neuropathol 2016;131:803-20. [Crossref] [PubMed]
- Louis DN, Perry A, Wesseling P, Brat DJ, Cree IA, Figarella-Branger D, Hawkins C, Ng HK, Pfister SM, Reifenberger G, Soffietti R, von Deimling A, Ellison DW. The 2021 WHO Classification of Tumors of the Central Nervous System: a summary. Neuro Oncol 2021;23:1231-51. [Crossref] [PubMed]
- Eckel-Passow JE, Lachance DH, Molinaro AM, Walsh KM, Decker PA, Sicotte H, et al. Glioma Groups Based on 1p/19q, IDH, and TERT Promoter Mutations in Tumors. N Engl J Med 2015;372:2499-508. [Crossref] [PubMed]
- Zhang CB, Bao ZS, Wang HJ, Yan W, Liu YW, Li MY, Zhang W, Chen L, Jiang T. Correlation of IDH1/2 mutation with clinicopathologic factors and prognosis in anaplastic gliomas: a report of 203 patients from China. J Cancer Res Clin Oncol 2014;140:45-51. [Crossref] [PubMed]
- Dang L, White DW, Gross S, Bennett BD, Bittinger MA, Driggers EM, Fantin VR, Jang HG, Jin S, Keenan MC, Marks KM, Prins RM, Ward PS, Yen KE, Liau LM, Rabinowitz JD, Cantley LC, Thompson CB, Vander Heiden MG, Su SM. Cancer-associated IDH1 mutations produce 2-hydroxyglutarate. Nature 2009;462:739-44. [Crossref] [PubMed]
- Dahuja G, Gupta A, Jindal A, Jain G, Sharma S, Kumar A. Clinicopathological Correlation of Glioma Patients with respect to Immunohistochemistry Markers: A Prospective Study of 115 Patients in a Tertiary Care Hospital in North India. Asian J Neurosurg 2021;16:732-7. [Crossref] [PubMed]
- Liu X, Li Y, Li S, Fan X, Sun Z, Yang Z, Wang K, Zhang Z, Jiang T, Liu Y, Wang L, Wang Y. IDH mutation-specific radiomic signature in lower-grade gliomas. Aging (Albany NY) 2019;11:673-96. [Crossref] [PubMed]
- Pope WB, Prins RM, Albert Thomas M, Nagarajan R, Yen KE, Bittinger MA, et al. Non-invasive detection of 2-hydroxyglutarate and other metabolites in IDH1 mutant glioma patients using magnetic resonance spectroscopy. J Neurooncol 2012;107:197-205. [Crossref] [PubMed]
- Choi C, Ganji SK, DeBerardinis RJ, Hatanpaa KJ, Rakheja D, Kovacs Z, Yang XL, Mashimo T, Raisanen JM, Marin-Valencia I, Pascual JM, Madden CJ, Mickey BE, Malloy CR, Bachoo RM, Maher EA. 2-hydroxyglutarate detection by magnetic resonance spectroscopy in IDH-mutated patients with gliomas. Nat Med 2012;18:624-9. [Crossref] [PubMed]
- de la Fuente MI, Young RJ, Rubel J, Rosenblum M, Tisnado J, Briggs S, et al. Integration of 2-hydroxyglutarate-proton magnetic resonance spectroscopy into clinical practice for disease monitoring in isocitrate dehydrogenase-mutant glioma. Neuro Oncol 2016;18:283-90. [Crossref] [PubMed]
- Tietze A, Choi C, Mickey B, Maher EA, Parm Ulhøi B, Sangill R, Lassen-Ramshad Y, Lukacova S, Østergaard L, von Oettingen G. Noninvasive assessment of isocitrate dehydrogenase mutation status in cerebral gliomas by magnetic resonance spectroscopy in a clinical setting. J Neurosurg 2018;128:391-8. [Crossref] [PubMed]
- Bertolino N, Marchionni C, Ghielmetti F, Burns B, Finocchiaro G, Anghileri E, Bruzzone MG, Minati L. Accuracy of 2-hydroxyglutarate quantification by short-echo proton-MRS at 3 T: a phantom study. Phys Med 2014;30:702-7. [Crossref] [PubMed]
- Kumar V, Gu Y, Basu S, Berglund A, Eschrich SA, Schabath MB, Forster K, Aerts HJ, Dekker A, Fenstermacher D, Goldgof DB, Hall LO, Lambin P, Balagurunathan Y, Gatenby RA, Gillies RJ. Radiomics: the process and the challenges. Magn Reson Imaging 2012;30:1234-48. [Crossref] [PubMed]
- Lambin P, Rios-Velazquez E, Leijenaar R, Carvalho S, van Stiphout RG, Granton P, Zegers CM, Gillies R, Boellard R, Dekker A, Aerts HJ. Radiomics: extracting more information from medical images using advanced feature analysis. Eur J Cancer 2012;48:441-6. [Crossref] [PubMed]
- Wang S, Xiao F, Sun W, Yang C, Ma C, Huang Y, Xu D, Li L, Chen J, Li H, Xu H. Radiomics Analysis Based on Magnetic Resonance Imaging for Preoperative Overall Survival Prediction in Isocitrate Dehydrogenase Wild-Type Glioblastoma. Front Neurosci 2022;15:791776. [Crossref] [PubMed]
- Xue C, Yuan J, Lo GG, Chang ATY, Poon DMC, Wong OL, Zhou Y, Chu WCW. Radiomics feature reliability assessed by intraclass correlation coefficient: a systematic review. Quant Imaging Med Surg 2021;11:4431-60. [Crossref] [PubMed]
- Gihr GA, Horvath-Rizea D, Hekeler E, Ganslandt O, Henkes H, Hoffmann KT, Scherlach C, Schob S. Histogram Analysis of Diffusion Weighted Imaging in Low-Grade Gliomas: in vivo Characterization of Tumor Architecture and Corresponding Neuropathology. Front Oncol 2020;10:206. [Crossref] [PubMed]
- Jakola AS, Zhang YH, Skjulsvik AJ, Solheim O, Bø HK, Berntsen EM, Reinertsen I, Gulati S, Förander P, Brismar TB. Quantitative texture analysis in the prediction of IDH status in low-grade gliomas. Clin Neurol Neurosurg 2018;164:114-20. [Crossref] [PubMed]
- Singh G, Manjila S, Sakla N, True A, Wardeh AH, Beig N, Vaysberg A, Matthews J, Prasanna P, Spektor V. Radiomics and radiogenomics in gliomas: a contemporary update. Br J Cancer 2021;125:641-57. [Crossref] [PubMed]
- Jian A, Jang K, Manuguerra M, Liu S, Magnussen J, Di Ieva A. Machine Learning for the Prediction of Molecular Markers in Glioma on Magnetic Resonance Imaging: A Systematic Review and Meta-Analysis. Neurosurgery 2021;89:31-44. [Crossref] [PubMed]
- Corr F, Grimm D, Saß B, Pojskić M, Bartsch JW, Carl B, Nimsky C, Bopp MHA. Radiogenomic Predictors of Recurrence in Glioblastoma-A Systematic Review. J Pers Med 2022;12:402. [Crossref] [PubMed]
- Tomczak K, Czerwińska P, Wiznerowicz M. The Cancer Genome Atlas (TCGA): an immeasurable source of knowledge. Contemp Oncol (Pozn) 2015;19:A68-77. [Crossref] [PubMed]
- Tustison NJ, Avants BB, Cook PA, Zheng Y, Egan A, Yushkevich PA, Gee JC. N4ITK: improved N3 bias correction. IEEE Trans Med Imaging 2010;29:1310-20. [Crossref] [PubMed]
- Zhang B, Tian J, Dong D, Gu D, Dong Y, Zhang L, Lian Z, Liu J, Luo X, Pei S, Mo X, Huang W, Ouyang F, Guo B, Liang L, Chen W, Liang C, Zhang S. Radiomics Features of Multiparametric MRI as Novel Prognostic Factors in Advanced Nasopharyngeal Carcinoma. Clin Cancer Res 2017;23:4259-69. [Crossref] [PubMed]
- Blagus R, Lusa L. SMOTE for high-dimensional class-imbalanced data. BMC Bioinformatics 2013;14:106. [Crossref] [PubMed]
- Cho HH, Lee SH, Kim J, Park H. Classification of the glioma grading using radiomics analysis. PeerJ 2018;6:e5982. [Crossref] [PubMed]
- Curtis AE, Smith TA, Ziganshin BA, Elefteriades JA. The Mystery of the Z-Score. Aorta (Stamford) 2016;4:124-30. [Crossref] [PubMed]
- Shriberg LD, Austin D, Lewis BA, McSweeny JL, Wilson DL. The percentage of consonants correct (PCC) metric: extensions and reliability data. J Speech Lang Hear Res 1997;40:708-22. [Crossref] [PubMed]
- Escanilla NS, Hellerstein L, Kleiman R, Kuang Z, Shull JD, Page D. Recursive Feature Elimination by Sensitivity Testing. Proc Int Conf Mach Learn Appl 2018;2018:40-7.
- Stoltzfus JC. Logistic regression: a brief primer. Acad Emerg Med 2011;18:1099-104. [Crossref] [PubMed]
- Wang Z, Dreyer F, Pulvermüller F, Ntemou E, Vajkoczy P, Fekonja LS, Picht T. Support vector machine based aphasia classification of transcranial magnetic stimulation language mapping in brain tumor patients. Neuroimage Clin 2021;29:102536. [Crossref] [PubMed]
- Wang H, Xu Q, Zhou L. Large unbalanced credit scoring using Lasso-logistic regression ensemble. PLoS One 2015;10:e0117844. [Crossref] [PubMed]
- Hartmann C, Hentschel B, Wick W, Capper D, Felsberg J, Simon M, Westphal M, Schackert G, Meyermann R, Pietsch T, Reifenberger G, Weller M, Loeffler M, von Deimling A. Patients with IDH1 wild type anaplastic astrocytomas exhibit worse prognosis than IDH1-mutated glioblastomas, and IDH1 mutation status accounts for the unfavorable prognostic effect of higher age: implications for classification of gliomas. Acta Neuropathol 2010;120:707-18. [Crossref] [PubMed]
- Houillier C, Wang X, Kaloshi G, Mokhtari K, Guillevin R, Laffaire J, Paris S, Boisselier B, Idbaih A, Laigle-Donadey F, Hoang-Xuan K, Sanson M, Delattre JY. IDH1 or IDH2 mutations predict longer survival and response to temozolomide in low-grade gliomas. Neurology 2010;75:1560-6. [Crossref] [PubMed]
- Li Y, Qin Q, Zhang Y, Cao Y. Noninvasive Determination of the IDH Status of Gliomas Using MRI and MRI-Based Radiomics: Impact on Diagnosis and Prognosis. Curr Oncol 2022;29:6893-907. [Crossref] [PubMed]
- Pereira SP, Oldfield L, Ney A, Hart PA, Keane MG, Pandol SJ, Li D, Greenhalf W, Jeon CY, Koay EJ, Almario CV, Halloran C, Lennon AM, Costello E. Early detection of pancreatic cancer. Lancet Gastroenterol Hepatol 2020;5:698-710. [Crossref] [PubMed]
- Horvat N, Veeraraghavan H, Pelossof RA, Fernandes MC, Arora A, Khan M, Marco M, Cheng CT, Gonen M, Golia Pernicka JS, Gollub MJ, Garcia-Aguillar J, Petkovska I. Radiogenomics of rectal adenocarcinoma in the era of precision medicine: A pilot study of associations between qualitative and quantitative MRI imaging features and genetic mutations. Eur J Radiol 2019;113:174-81. [Crossref] [PubMed]
- Zhou H, Vallières M, Bai HX, Su C, Tang H, Oldridge D, Zhang Z, Xiao B, Liao W, Tao Y, Zhou J, Zhang P, Yang L. MRI features predict survival and molecular markers in diffuse lower-grade gliomas. Neuro Oncol 2017;19:862-70. Erratum in: Neuro Oncol 2017;19:1701. [Crossref] [PubMed]
- Ryu YJ, Choi SH, Park SJ, Yun TJ, Kim JH, Sohn CH. Glioma: application of whole-tumor texture analysis of diffusion-weighted imaging for the evaluation of tumor heterogeneity. PLoS One 2014;9:e108335. [Crossref] [PubMed]
- Qin JB, Liu Z, Zhang H, Shen C, Wang XC, Tan Y, Wang S, Wu XF, Tian J. Grading of Gliomas by Using Radiomic Features on Multiple Magnetic Resonance Imaging (MRI) Sequences. Med Sci Monit 2017;23:2168-78. [Crossref] [PubMed]
- Akhoundzadeh K, Shafia S. Association between GFAP-positive astrocytes with clinically important parameters including neurological deficits and/or infarct volume in stroke-induced animals. Brain Res 2021;1769:147566. [Crossref] [PubMed]
- Park CJ, Choi YS, Park YW, Ahn SS, Kang SG, Chang JH, Kim SH, Lee SK. Diffusion tensor imaging radiomics in lower-grade glioma: improving subtyping of isocitrate dehydrogenase mutation status. Neuroradiology 2020;62:319-26. [Crossref] [PubMed]
- Bangalore Yogananda CG, Shah BR, Vejdani-Jahromi M, Nalawade SS, Murugesan GK, Yu FF, Pinho MC, Wagner BC, Mickey B, Patel TR, Fei B, Madhuranthakam AJ, Maldjian JA. A novel fully automated MRI-based deep-learning method for classification of IDH mutation status in brain gliomas. Neuro Oncol 2020;22:402-11. [Crossref] [PubMed]
- Karabacak M, Ozkara BB, Mordag S, Bisdas S. Deep learning for prediction of isocitrate dehydrogenase mutation in gliomas: a critical approach, systematic review and meta-analysis of the diagnostic test performance using a Bayesian approach. Quant Imaging Med Surg 2022;12:4033-46. [Crossref] [PubMed]