Improving visualization of free fibula flap perforators and reducing radiation dose in dual-energy CT angiography
Introduction
Surgical resection is the primary treatment method for most maxillofacial lesions but may eventually induce a large defect of the mandible, oral mucosa, and adjacent soft tissues (1). Subsequently, reconstruction of mandibular defects with a vascularized osteocutaneous free flap (OCFF) has been the gold standard for restoring the functions and aesthetics of the maxillofacial structure (2). Among the OCFFs, the fibula free flap (FFF), first reported by Hidalgo in 1989 (3), has been a workhorse flap for reconstruction after the resection of maxillofacial bone and soft tissue (4). Nevertheless, it is still a challenge to successfully harvest the FFF due to potential peripheral vascular disease and significant anatomic variants of the peroneal artery and its perforators (5). Therefore, preoperative comprehensive knowledge of the size, location, branching pattern, course, and anatomical variation of perforators of the FFF is essential for minimizing procedure-related complications and providing the reconstructive surgeon with a definitive road map and details for successfully harvesting the FFF and improving surgical planning (6,7).
Currently, various imaging techniques, such as digital subtraction angiography (DSA), magnetic resonance angiography (MRA), and computed tomography angiography (CTA), have been used for the preoperative evaluation of the anatomic variants of perforators of the FFF (8,9). For many years, DSA has been considered the diagnostic gold standard owing to its excellent spatial and temporal resolution, but the invasive nature of the procedure has limited its clinical application (3). Recently, CTA and MRA were introduced as noninvasive and convenient methods for the preoperative evaluation of perforators of the FFF (10,11). Compared to MRA, CTA is a more favorable method because it not only provides high-resolution images of vessels but also allows the simultaneous 3-dimensional reconstruction of adjacent soft tissues and bones (12). Several studies have used conventional CTA to demonstrate the size, diameter, course, location, and anatomical variability of the perforating branches of the leg to provide details of the vascular anatomy for harvesting the free flap (7,8). However, conventional CTA is only able to determine the major perforating arteries but cannot identify small perforating branches of the FFF due to its relatively low resolution. Furthermore, the cumulative radiation dose of the routine dual-phase or multi-phase CTA examination is inherently high due to the repeated scans in a single examination (13). Therefore, exploring new techniques to simultaneously improve the visualization of the tiny perforating arteries and reduce the radiation dose of the CTA examination is highly desirable.
Dual-energy computed tomography (DECT) is an emerging technology that can address both of these above concerns. In DECT, low- and high-kilovolt (kV) data sets are simultaneously acquired, allowing the generation of multiple imaging reconstructions and the performance of advanced tissue characterization based on the attenuation properties of different tissues and materials, with advancements in improved temporal and spatial resolution, increased anatomic coverage, radiation dose reduction strategies, and noise optimization (14-16). Currently, several DECT reconstructions, including virtual noncontrast (VNC) images, virtual monoenergetic imaging (VMI), iodine maps for perfusion analysis, and virtual noncalcium (VNCa) images, offer new prospects for addressing the deficiencies in conventional CT (14,17). Based on the principle of material separation, the iodine component can be removed from the original images, and VNC images may be generated using dual-energy postprocessing algorithms (18). Several studies have reported that VNC images can be generated to allow for radiation dose reduction by removing the performance of the noncontrast phase and, meanwhile, providing a comparable image quality (19-21). In addition, VMI reconstructions are synthesized at the image domain by the linear combination of the low- and high-energy level CT images. In low-energy level reconstructions, attenuation will notably increase due to the chosen energy level being close to the K-edge of iodine, which leads to an increased contrast-to-noise ratio (CNR) (14,22). Previously, VMI reconstructions at low keV values have been reported as a feasible method for improving the visibility of small arteries and salvaging the images in poor contrast conditions for CTA (22-26). To date, a few preliminary studies have applied VNC imaging and/or VMI simultaneously or alone to reduce the radiation dose and/or improve the visualization of tiny vessels in the DECT images (22,25,27,28). However, no studies have reported the potential benefits of VNC and VMI for the reduction of the radiation dose and improvement of the visualization of tiny perforators for the FFF harvest in the CTA examination.
The purposes of our study were to evaluate the possibility of using VNC images as an alternative to true noncontrast (TNC) images with a reduction of radiation. Additionally, we compared the subjective and objective data at various energy-level images reconstructed from dual-energy computed tomographic angiography (DE-CTA) to identify the optimal energy level in VMI reconstructions for improving visualization of the perforators of the FFF. We present the following article in accordance with the STROBE reporting checklist (available at https://qims.amegroups.com/article/view/10.21037/qims-22-734/rc).
Methods
Patients
The study was conducted in accordance with the Declaration of Helsinki (as revised in 2013). This retrospective cross-sectional study was approved by the Institutional Ethics Committee of Sun Yat-sen Memorial Hospital, and informed consent was acquired from the patients or the patients’ legal guardians. Between April 2021 and March 2022, patients with maxillofacial lesions who were scheduled to undergo lower-extremity DE-CTA in Sun Yat-sen Memorial Hospital, Sun Yat-sen University, were enrolled as participants in this study. The inclusion criteria were the following: (I) patients with maxillofacial lesions requiring surgical treatment, and (II) patients scheduled to undergo preoperative lower-extremity DE-CTA for harvesting of a FFF for maxillofacial reconstruction. The exclusion criteria were the following: (I) patients with contraindications for CTA examination (pregnancy, unstable clinical condition, allergy to iodine), (II) patients with diffuse bone abnormalities and severe muscular atrophy, (III) patients with images showing severe motion or shadow artifacts, and (IV) patients with images showing diffuse calcifications of a vessel wall or vascular occlusion that would hinder the region of interest (ROI) analysis. The patient enrollment process is shown in Figure 1. The variables in this study had no missing data.
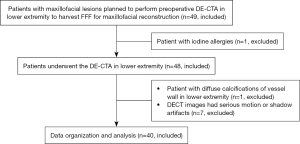
Scanning method and image processing
All examinations were performed on a third-generation dual-source CT system (SOMATOM Force; Siemens Healthcare, Erlangen, Germany). The scan range extended from the upper edge of the patella to within 1 cm of the lower edge of the lateral malleolus. The major parameters in the dual-source, dual-energy mode were the following: tube A 80 kVp, tube B 140 kVp, gantry rotation time 0.50 s, pitch 1, and collimation 128×0.6 mm. Automated tube-current modulation was performed in all studies (CAREdose4D; Siemens Healthineers). After the acquisition of the noncontrast phase, 1.5 mL/kg of nonionic contrast medium (ioversol, 350 mgI/mL; Hengrui Pharmaceutical Co, Ltd, Jiangsu, China) was injected intravenously into the median cubital vein at a flow rate of 4–5 mL/sec for a total of 60–100 mL, which was followed by the injection of a 30-mL saline chaser at the same flow rate. After the ROI was placed in the popliteal artery, the start of contrast-enhanced image acquisition was automatically launched at 10–12 s after the attenuation reached the predefined threshold of 120 HU using the bolus-tracking technique. The patients underwent noncontrast phase and arterial phase imaging in a DECT scan. The mixed images generated from the noncontrast images in DECT are hereafter referred to as M_0.5-TNC images.
All DE-CTA images were reconstructed with a strength level of 3 and a kernel (Qr32) using the advanced modeled iterative reconstruction (ADMIRE; Siemens Healthineers). The thickness for reconstruction was 1 mm with an increment of 0.7 mm. All images were transferred to a dedicated DE postprocessing workstation (syngo.via, v. VB20A, Siemens Healthineers) with a multimodality DECT application. The “VNC” workflow was used for iodine subtraction to generate the VNC images. The VNC images, the linear-blended images in the arterial phase using a standard blending ratio of 0.5 (M_0.5-C), and the VMI series ranging from 40 to 100 keV (20-keV intervals) were then generated. The VMI series were generated via a Monoenergetic+ algorithm in a Siemens dual-source system (Siemens Healthineers ). Maximum intensity projection (MIP) was created for both the VMI and M_0.5-C images by a radiologist.
ROI assessment
Objective measurements were completed by an experienced radiologist (GZS, with 8 years of experience). The average attenuation and the corresponding standard deviation (SD) were obtained based on measurements of the cross-sectional images conducted 3 times in the popliteal, anterior tibial, posterior tibial, peroneal arteries, tibialis anterior, and fat by performing ROI analysis. The size of the ROIs depended on the diameter of the popliteal artery (ROI size: 13–30 mm2), anterior tibial artery (ROI size: 2–6 mm2), posterior tibial artery (ROI size: 2–5 mm2), and peroneal artery (ROI size: 2–5 mm2). The ROI sizes of the tibialis anterior and fat were 100 mm2 and 20 mm2, respectively. The ROIs were placed at the center of the vessels, and the area analyzed was obtained at the maximum diameter of the vessel, with calcifications, the vascular wall, and other influencing factors being avoided. Next, the signal-to-noise ratio (SNR) and CNR were calculated using the following formulas: SNR = HU vessel/noise; CNR = (HU vessel − HU muscle)/noise (in which noise represents the SD of vascular attenuation).
Reader assessment
Two radiologists blinded to the histological diagnosis (XHD and FZ, with 12 and 10 years of experience, respectively) evaluated the VNC and VMI series/MIP images (40 keV, 60 keV, 80 keV, and 100 keV) and compared them to the M_0.5-TNC and M_0.5-C images employing a 5-point Likert scale. The degree of contrast agent removal in the VNC images was evaluated compared to that in the M_0.5-TNC images with detailed scoring criteria used in a preceding study (22). In all patients, 8 individual arterial segments and a total of 162 perforators were evaluated and scored by each observer.
The subjective image quality of the VMI series and M_0.5-C images were assessed using the following parameters: noise, artifact, anatomic detail, and venous contamination. This was scored on the following 5-point scale: 5 points = excellent (no obvious image noise, artifacts, or venous contamination; excellent distinction of anatomic structures and details), 4 points = good (mild image noise, artifacts, and venous contamination; good visualization of anatomic structures and details but not an excellent evaluation of the images), 3 points = fair (moderate image noise and artifacts, acceptable anatomic details but insufficient for diagnosis), 2 points = poor (no clear anatomic detail and inadequate for diagnosis), and 1 point = unacceptable (obscured anatomic structures and no possibility of diagnosis) (29).
The depiction of perforators in the VMI reconstructions and M_0.5-C images were evaluated by assessing their continuity, vascular contrast, and diagnostic confidence, with scores ranging from 5 (excellent delineation of perforators, strong vascular contrast, and high diagnostic certainty) to 1 (poorly defined perforator, lack of contrast enhancement, and uncertain diagnosis) (30).
Radiation dose assessment
The dose-length product (DLP; mGy·cm) and CT volume dose index (CTDIvol; mGy) from the M_0.5-TNC and arterial phase images were recorded in each patient. To investigate the potential radiation dose reduction, the DLP and CTDIvol of the noncontrast phase and contrast-enhanced phase (noncontrast + CTA) group were compared with these of the contrast-enhanced phase (CTA) group. Radiation dose reduction was calculated according to the following formula: radiation dose reduction % = [(mean radiation dose of the noncontrast + CTA group – mean radiation dose of the CTA group)/mean radiation dose of the noncontrast + CTA group] ×100.
Statistical analysis
All data analysis were performed using SPSS v. 25.0 (IBM Corp., Armonk, NY, USA). Continuous variables were described as mean (SD) and categorical variables were described as percentages or frequencies. The distribution of data and homogeneity of variance were tested using the Shapiro-Wilk test and Levene test, respectively. The attenuation, noise, CNR and SNR, and radiation doses between the M_0.5-TNC and VNC images followed a normal distribution with homogenous variance and were compared using the independent samples t test. The attenuation, noise, CNR, and SNR of the VMI series and M_0.5-C images did not follow a normal distribution and homogeneity of variance and were compared using the Kruskal-Wallis test. The Wilcoxon signed-rank test was used to compare the subjective image quality. Cohen’s κ was used to assess the consistency of scores between the 2 readers. A κ value could be interpreted as slight agreement (<0.20), fair agreement (0.21–0.40), moderate agreement (0.41–0.60), substantial agreement (0.61–0.80), or excellent agreement (0.81–1.00). Significance was defined as a P<0.05.
Results
Participant characteristics
Of the 49 patients initially included in our study, 9 participants were excluded for the following reasons: iodine allergies (n=1), diffuse calcifications of a vessel wall in the lower extremity (n=1), and DECT images with serious motion or shadow artifacts (n=7). Finally, 40 patients with an age range from 14.0 to 83.0 years were included in this study. The baseline characteristics of the included patients are summarized in Table 1.
Table 1
Patient characteristic | Value |
---|---|
Age (years old) | 55±18.0 |
Sex | |
Male | 28 (70.0) |
Female | 12 (30.0) |
BMI (kg/m2) | 23.52±2.48 |
Clinical diagnosis | |
Squamous cell carcinoma | 21 (52.5) |
Ameloblastoma | 5 (12.5) |
Osteonecrosis | 2 (5.0) |
Clear cell carcinoma | 1 (2.5) |
Other benign tumors | 11 (27.5) |
Continuous variables are described as mean ± SD, and categorical variables are presented as the number (percentage). BMI, body mass index.
Assessment of TNC and VNC
Table 2 shows the objective comparisons of the M_0.5-TNC and VNC images, and representative images are presented in Figure 2. No statistically significant difference was observed in the attenuation, noise, SNR, or CNR between the M_0.5-TNC and VNC images of the popliteal, anterior tibial, posterior tibial, and peroneal arteries and muscles (P>0.09 to P>0.99), but the attenuation of the fat was significantly different between the M_0.5-TNC (mean –100.2, SD 15.39; 95% CI: –104.0 to –97.76) and VNC images (mean –69.16, SD 9.79; 95% CI: –71.28 to –66.87) with P<0.001.
Table 2
Parameters | TNC (95% CI) | VNC (95% CI) | P value |
---|---|---|---|
Popliteal artery | |||
Attenuation (HU) | 47.33±6.77 (44.52, 47.57) | 44.98±7.10 (43.46, 46.84) | 0.22 |
SNR | 6.71±1.97 (6.25, 7.18) | 6.73±2.25 (6.29, 7.34) | 0.96 |
CNR | 1.24±0.98 (0.98, 1.43) | 1.30±1.26 (1.07, 1.66) | 0.73 |
Noise (HU) | 7.41±2.23 (6.86, 7.89) | 7.26±2.2 (6.67, 7.65) | 0.66 |
Anterior tibial artery | |||
Attenuation (HU) | 44.48±8.17 (42.44, 46.34) | 45.04±8.30 (43.00, 46.92) | 0.67 |
SNR | 7.34±2.45 (6.81, 7.97) | 7.25±2.65 (6.75, 7.99) | 0.82 |
CNR | 1.65± 1.17 (1.35, 1.88) | 1.44±1.31 (1.17, 1.79) | 0.28 |
Noise (HU) | 6.58±1.94 (6.13, 7.03) | 6.85±2.18 (6.34, 7.36) | 0.41 |
Posterior tibial artery | |||
Attenuation (HU) | 43.33±7.59 (41.59, 45.11) | 41.71±8.14 (39.79, 43.62) | 0.19 |
SNR | 7.25±2.75 (6.69, 7.98) | 7.17±2.74 (6.51, 7.72) | 0.85 |
CNR | 1.68±1.21 (1.35, 1.90) | 1.68±1.36 (1.43, 2.06) | 0.98 |
Noise (HU) | 6.75±2.63 (6.09, 7.32) | 6.56±2.55 (5.97, 7.03) | 0.64 |
Peroneal artery | |||
Attenuation (HU) | 46.43±8.75 (44.22, 48.29) | 44.27±7.92 (42.51, 46.27) | 0.10 |
SNR | 7.68±2.45 (7.17, 8.29) | 7.72±2.78 (7.14, 8.44) | 0.91 |
CNR | 1.51±1.53 (1.15, 1.85) | 1.50±1.41 (1.23, 1.90) | >0.99 |
Noise (HU) | 6.52±1.92 (6.01, 6.83) | 6.23±1.85 (5.75, 6.60) | 0.31 |
Muscle | |||
Attenuation (HU) | 52.96±4.23 (51.96, 53.96) | 51.79±4.50 (50.84, 52.94) | 0.09 |
SNR | 7.91±2.16 (7.36, 8.38) | 7.93±2.11 (7.51, 8.51) | 0.96 |
Noise (HU) | 7.21±1.79 (6.79, 7.64) | 6.85±1.65 (6.46, 7.24) | 0.18 |
Fat | |||
Attenuation (HU) | −100.2±15.39 (−104.00, −96.76) | −69.16±9.79 (−71.28, −66.87) | <0.001 |
SNR | −11.98±4.58 (−12.94, −10.82) | −10.95±4.49 (−11.95, −9.87) | 0.77 |
Noise (HU) | 9.43±3.47 (8.71, 10.34) | 7.3±3.17 (6.63, 8.10) | 0.20 |
Data are expressed as mean ± SD (95% CI). VNC, virtual noncontrast; TNC, true noncontrast; SNR, signal-to-noise ratio; CNR, contrast-to-noise ratio; HU, Hounsfield unit.
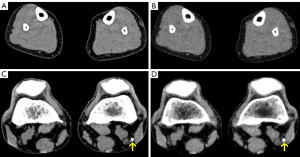
For the image-quality assessment of the VNC images with removal of contrast media, 2 radiologists rated 90% of cases with a score of 5 (complete removal of contrast media from M_0.5-TNC images) and 10% of VNC images with a score of 4 (almost complete removal of contrast media from M_0.5-TNC images). There was good agreement on the image-quality scores between the 2 observers for the VNC images (κ=0.773).
Radiation dose assessment
For the noncontrast + CTA group, the mean DLP was 303.94 mGy·cm (SD 33.21) and the mean CTDIvol was 5.64 mGy (SD 0.60). For the CTA group, the mean DLP was 147.66 mGy·cm (SD 27.74) and the mean CTDIvol was 2.79 mGy (SD 0.53). The potential dose savings from the CTA group were about 50% lower compared with the noncontrast + CTA group (P<0.001).
ROI assessment of VMI reconstructions
Table 3 provides the detailed results of the comparison between the serial VMI reconstructions and M_0.5-C images. The attenuation, noise, and CNR in the VMI series showed a similar trend, decreasing from low to high keV levels. Vascular attenuation in the VMI reconstructions was superior to that in the M_0.5-C images at 40 and 60 keV (P<0.001 to P=0.04) and increased approximately 165% and 33% at 40 and 60 keV, respectively. At 80 and 100 keV, the mean attenuation in the VMI reconstructions was significantly lower than that in the M_0.5-C images (P<0.001 to P=0.01), except for muscle, in which the attenuation at 80 keV was similar to that in the M_0.5 images (P=0.66). For all evaluated arteries and muscle, the image noise was significantly lower in the 80 and 100 keV VMI images than that in the M_0.5 images (all P<0.001); the image noise was similar between the 60 keV VMI images and the M_0.5 images (all P>0.99); and the image noise was significantly higher in the 40 keV VMI than in the M_0.5 (all P<0.001). Furthermore, noise was highest at 40 keV compared with all other VMI series with significant differences (all P<0.001).
Table 3
Parameters | 40 keV (95% CI) | 60 keV (95% CI) | 80 keV (95% CI) | 100 keV (95% CI) | M_0.5 (95% CI) |
---|---|---|---|---|---|
Popliteal artery | |||||
Attenuation, HU | 1,218.88±265.09* (1,152.88, 1,285.09) | 589.51±107.94* (558.31, 613.84) | 322.13±63.34* (304.16, 336.31) | 206.03±36.67* (196.62, 215.44) | 451.88±103.07 (431.79, 484,06) |
Noise, HU | 26.94±3.50* (26.06, 27.81) | 11.99±1.63 (11.71, 12.87) | 6.76±1.47* (6.38, 7.13) | 6.19±1.77* (5.75, 6.63) | 11.69±1.78 (11.02, 12.09) |
SNR | 45.74±10.59 (43.09, 48.39) | 48.50±11.61* (45.59, 51.40) | 49.65±14.51* (46.02, 53.27) | 36.33±13.49 (32.96, 39.69) | 40.55±12.55 (37.96, 44.24) |
CNR | 61.81±19.16* (57.02, 66.59) | 50.38±15.17* (46.49, 54.26) | 36.41±12.02 (33.26, 39.46) | 24.97±8.80* (22.77, 27.16) | 40.77 ±14.94 (36.95, 44.60) |
Anterior tibial artery | |||||
Attenuation, HU | 1,090.85±235.12* (1,032. 1,149.57) | 554.6 8±92.37* (527.13, 577.71) | 303.27±55.28* (289.35, 317.19) | 194.81±32.87* (186.60, 203.02) | 418.13±90.98 (396.19, 445.91) |
Noise, HU | 26.74±4.67* (25.57, 27.91) | 12.52±2.14 (11.71, 13.10) | 7.19±2.05* (6.68, 7.73) | 5.59±2.13* (5.06, 6.12) | 12.58±2.08 (12.20, 13.64) |
SNR | 41.83±11.11 (39.06, 44.61) | 43.16±11.08* (40.04, 45.34) | 45.05±13.76* (41.25, 48.31) | 41.64±21.93 (36.17, 47.12) | 36.71±11.16 (34.18, 39.75) |
CNR | 54.86±16.98* (50.62, 59.10) | 46.87±13.2* (42.94, 49.48) | 33.91±10.36 (31.16, 36.48) | 23.09±7.51* (21.22, 24.97) | 37.68±13.38 (34.13, 41.23) |
Posterior tibial artery | |||||
Attenuation, HU | 1,090.53±254.31* (1,027.01, 1,154.06) | 565.48±99.98* (533.73, 589.61) | 302.47±64.60* (286.33, 318.61) | 192.8 2± 38.92* (183.10, 202.55) | 413.09± 93.10 (387.43, 438.75) |
Noise, HU | 27.46±4.22* (26.41, 28.52) | 12.70±2.07 (12.01, 13.32) | 6.94±1.88* (6.45, 7.41) | 5.27±2.21* (4.71, 5.82) | 12.78±2.16 (12.10, 13.46) |
SNR | 40.80±12.65 (37.64, 43.96) | 42.61±12.99* (39.36, 45.85) | 47.21±19.02* (42.13, 51.90) | 41.3±16.41 (37.20, 45.40) | 36.33±12.25 (33.75, 39.90) |
CNR | 54.69±17.35* (50.36, 59.02), | 48.48±12.61* (44.96, 51.98) | 33.50±10.70 (30.59, 36.09) | 22.67±7.91* (20.69, 24.64) | 36.58±12.53 (33.53, 40.25) |
Peroneal artery | |||||
Attenuation, HU | 1,126.48±247.03* (1,064.77, 1,188.18) | 558.32±102.99* (528.05, 582.13) | 304.07±60.20* (289.11, 319.48) | 197.35±53.03* (184.11, 210.60) | 425.89±100.10 (402.67, 455.28) |
Noise, HU | 27.58±4.55* (26.44, 28.71) | 12.78±2.07 (12.40, 13,71) | 6.91±1.43* (6.57, 7.30) | 5.47±2.49* (4.84, 6.09) | 12.89±2.01 (12.00, 13.21) |
SNR | 42.14±11.32* (39.31, 44.97) | 43.34±11.31* (40.51, 46.16) | 45.73±13.22* (42.02, 48.42) | 40.26±16.80 (36.06, 44.45) | 35.57±10.04 (33.25, 38.28) |
CNR | 56.46±16.37* (52.37, 60.55) | 48.38±12.51* (44.60, 51.25) | 33.69±10.25 (31.12, 36.24) | 23.09±8.52* (20.97, 25.22) | 37.12±12.23 (34.22, 40.64) |
Muscle | |||||
Attenuation, HU | 78.22±17.01* (73.97, 82.47) | 63.45±7.53* (61.43, 65.47) | 55.71±5.33 (54.43, 57.16) | 52.91±4.49* (51.78, 54.03) | 57.98±5.58 (56.44, 59.51) |
Noise, HU | 19.04±2.92* (18.31, 19.76) | 10.68±1.73 (9.87, 10.94) | 7.60±1.40* (7.21, 7,92) | 6.44±1.36* (6.10, 6.78) | 9.98±1.80 (9.68, 10.77) |
SNR | 4.21±1.17* (3.91, 4.50) | 5.96±1.29 (5.63, 6.28) | 7.61±1.67* (7.18, 8.02) | 8.59±2.06* (8.08, 9.11) | 6.17±1.26 (5.85, 6.48) |
Data are expressed as mean ± SD (95% CI). *, the difference was statistically significant between VMI reconstructions and M_0.5-C images. VMI, virtual monoenergetic imaging; M_0.5-C, 0.5 linear image blending image in arterial phase; keV, kiloelectron volt; SNR, signal-to-noise ratio; CNR, contrast-to-noise ratio; HU, Hounsfield unit.
The highest CNR value of all the evaluated arteries and muscle was found at 40 keV in comparison with other reconstructions (all P<0.001), except for 60 keV (P>0.15 to P>0.99). Significantly higher CNR values were observed at 60 keV for VMI reconstructions in comparison with the M_0.5-C images (P<0.001 to P=0.02). A similar CNR value was observed at 80 keV (P>0.99), and 100-keV VMI reconstructions showed inferior CNR values compared with the M_0.5-C images (all P<0.001).
For all evaluated arteries, SNR was increased at 60 keV for VMI reconstructions compared with the M_0.5-C series (P<0.001 to P=0.02). Similar SNR values were observed at 60 keV in muscle and at 40 keV in the popliteal, anterior tibial, and posterior tibial arteries for VMI reconstructions compared with the M_0.5-C images (P=0.05 to P>0.99). The SNR of all evaluated arteries showed a higher value at 80 keV in comparison with 60 keV, but these differences were not statistically significant (all P>0.99).
Reader assessment of VMI reconstructions
Table 4 summarizes the scores of the subjective assessment and Figure 3 demonstrates representative images. The higher scores were achieved at 40 and 60 keV compared with all other VMI images. The overall subjective analysis of image quality was rated best at 60 keV for VMI reconstructions, followed by 40 keV, with statistically significant differences (all P<0.001). The M_0.5-C images received superior scores compared with the 80-keV and 100-keV VMI reconstructions (all P<0.001), but the 40-keV and 60-keV reconstructions were significantly superior to the M_0.5-C images (all P<0.001).
Table 4
Score | 5 | 4 | 3 | 2 | 1 | κ value |
---|---|---|---|---|---|---|
Image quality in M_0.5 and VMI reconstructions (N=80), n (%) | ||||||
40 keV | 28 (35.0) | 48 (60.0) | 4 (5.0) | 0 (0.0) | 0 (0.0) | 0.927 |
60 keV | 66 (82.5) | 14 (17.5) | 0 (0.0) | 0 (0.0) | 0 (0.0) | 0.918 |
80 keV | 0 (0.0) | 20 (25.0) | 50 (62.5) | 10 (12.5) | 0 (0.0) | 0.856 |
100 keV | 0 (0.0) | 4 (5.0) | 50 (62.5) | 26 (32.5) | 0 (0.0) | 0.905 |
M_0.5-C | 26 (32.5) | 42 (52.5) | 12 (1.5) | 0 (0.0) | 0 (0.0) | 0.873 |
Visualization of perforators (N=162), n (%) | ||||||
40 keV | 84 (51.8) | 56 (34.6) | 15 (9.3) | 0 (0.0) | 7 (4.3) | 0.921 |
60 keV | 74 (45.7) | 64 (39.5) | 17 (10.5) | 0 (0.0) | 7 (4.3) | 0.911 |
80 keV | 0 (0.0) | 9 (5.6) | 34 (21.0) | 112 (69.1) | 7 (4.3) | 0.875 |
100 keV | 0 (0.0) | 0 (0.0) | 8 (4.9) | 147 (90.8) | 7 (4.3) | 0.803 |
M_0.5-C | 7 (4.3) | 52 (32.1) | 39 (24.1) | 57 (35.2) | 7 (4.3) | 0.840 |
VMI, virtual monoenergetic imaging; M_0.5-C, 0.5 linear image blending image in arterial phase; keV, kiloelectron volt.
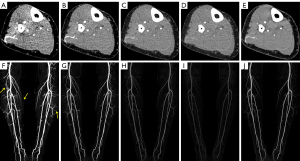
For perforator visualization, compared with the M_0.5-C images, superior scores were observed for VMI reconstructions at 40 keV and 60 keV (all P<0.001), while lower scores were found at 80 keV and 100 keV (all P<0.001). The best vascular visualization ratings were observed in VMI reconstructions at 40 keV in comparison with all other image series with statistically significant differences (all P<0.001), except for VMI reconstructions at 60 keV (P=0.31). The scores assessed at 60 keV for VMI were significantly superior to the M_0.5-C images and the 80-keV and 100-keV VMI reconstructions (all P<0.001).
Discussion
In our study, the VNC images from the third-generation DECT were comparable to the M_0.5-TNC images; thus, VNC images could potentially replace M_0.5-TNC images to substantially reduce radiation dose. In addition, VMI reconstructions from the arterial phase in our study could provide advantages by improving image quality, visualization of perforators, and clinical diagnostic confidence compared with M_0.5-C images. The 60-keV VMI reconstructions obtained the most optimal subjective and objective assessments of all of the VMI series.
DECT is a tremendous innovation in CT technology that enables the generation of a wide range of images via different postprocessing algorithms. DECT-derived images [such as VMI, VNC images, material density (MD) images, and VNCa images] can characterize specific materials; reduce the use of contrast medium, radiation dose and artifacts; and allow numerous quantitations of tissue, thereby improving lesion detection and diagnostic confidence (31,32). Several previous studies have combined VNC and VMI to maintain high-quality images and simultaneously reduce the radiation dose in the diagnosis of renal cell carcinoma (RCC) (33) and in the depiction of abdominal vessels (22). Zhang et al. (33) indicated that the application of VNC images and VMI to replace TNC and corticomedullary phase images could obtain comparable image quality while reducing the radiation dose by 50.5%. Lennartz et al (22) found that VNC images and 40-keV VMI reconstructions generated from single venous-phase images could obtain comparable image quality for detecting the major abdominal vessels as compared to TNC and arterial phase CTA images. Nevertheless, the simultaneous use of VNC imaging and VMI from DECT to improve the image quality and perforator visualization of the FFF has, to our knowledge, not yet been reported.
The VNC image is generated by removing the iodine from the enhanced DECT image, and this can reduce the X-ray exposure dose and scanning time in replacing the TNC for diagnosis (15). To demonstrate the feasibility of reducing the radiation dose by replacing the TNC images with VNC images, we evaluated the image quality of the major arteries and adjacent muscle of the lower leg in M_0.5-TNC and VNC images. Our results showed that the VNC images from the arterial phase were similar to the M_0.5-TNC images with respect to attenuation, noise, SNR, and CNR, and achieved excellent removal of contrast media. Additionally, approximately 50% of the radiation dose was reduced if the VNC images derived from the arterial phase replaced the M_0.5-TNC images. These results were similar with most of those reported in previous studies which have indicated that VNC imaging can potentially replace TNC images due to its ability to reduce contrast media (15,18-21). However, some inconsistencies were found with a few studies (34,35). De Cecco et al. (34) investigated the differences in image quality, attenuation, and artifacts between the TNC and VNC images of a liver lesion on a second-generation DECT system and found that the VNC images could not replace the TNC images due to the limitations in material subtraction, which is different to our results. This discrepancy might be explained by the variability in TNC settings and patient thickness. In De Cecco et al.’s study, a standard single kilovoltage peak acquisition was applied for TNC images; in contrast, we used the DECT protocol for the noncontrast phase. In addition, the thickness of abdominal regions in De Cecco et al.’s study was much thicker than that of the lower extremity in our study, which might have resulted in more scattering artifacts in the former. Botsikas et al. (35) demonstrated a significantly higher attenuation of adrenal adenomas on VNC than on TNC imaging. The inconsistency may be explained by the difference in fat attenuation between TNC and VNC imaging, which may introduce uncertain factors which influence the assessment of adrenal lesion characterization. In the present study, the difference of attenuation in fat was found between the VNC and M_0.5-TNC images, but it did not have an effect on the image quality or diagnostic confidence. Taken together, we can deduce that VNC images are not equivalent to TNC images even though they may have similar properties because VNC images are generated by removing the iodine from enhanced DECT images based on a material decomposition algorithm to simulate the TNC images. Nevertheless, VNC images derived from DECT could serve as a surrogate for TNC images in clinical practice to reduce the radiation dose for patients undergoing FFF harvest.
Our study also investigated whether low-keV VMI reconstructions from the arterial phase could improve image quality and thus provide adequate details for the visualization of perforators. Generally, improved visualization of enhanced small vessels is usually reliant on vascular enhancement and image noise. CNR is the major objective parameter of vascular enhancement. It has been reported that VMI reconstructions derived from DECT can improve the CNR of enhanced vessels and thus may help to visualize the blood vessels much better, without increasing the radiation dose and volume of contrast agent (23,36). The VMI, reconstructed by using a “frequency-split” technique that combines high- and low-energy CT data, can increase the attenuation at low-keV levels due to the chosen energy level which approximates the K-edge of iodine although the noise increases at the same time (14). Consequently, the increased iodine attenuation can significantly improve the vessel visibility. In our study, the vascular attenuation and CNR at 40 and 60 keV were superior to those in the M_0.5-C images, indicating that low-keV VMI reconstructions may significantly improve the quantitative image quality of vascular structures compared with M_0.5-C images. Our qualitative assessment also seems to support these findings. These results were in line with current studies showing that VMI reconstructions at low keV could meaningfully increase vascular attenuation and raise the enhancement of vessels (23,37).
On the other hand, with decreasing energy levels, the increased noise and appearance of venous pollution will impair the overall image quality, which may hinder the observation of small perforator vessels of FFF. Therefore, an optimal keV level of VMI reconstructions must be selected carefully to balance the vessel contrast, image noise, and venous pollution to obtain the highest image quality for visualization of the tiny perforators of FFF (27). At present, no study has applied VMI reconstructions to improve the visualization of tiny perforators for FFF harvest in CTA examination, and the optimal keV for visualization of tiny perforators remains unknown. In addition, although some studies (38-40) have used single low-keV VMI reconstructions for the assessment of carotid and cerebral arteries and thoracic and abdominal vessels, few studies have compared the image quality of VMI reconstructions at various keV levels to determine the optimal keV for vasculature visualization. In these limited studies, however, the optimal keV of VMI reconstructions for vascular visualization has still been inconsistent, ranging from 40 to 60 keV compared with standard linearly blended images (22,27,30,37). In the present study, we found that significant image noise and severe venous contamination impaired the diagnostic confidence in perforator visualization, although readers in our study preferred VMI reconstructions at 40 keV for the depiction of perforators. The attenuation, SNR, CNR, visualization of perforators, and overall image quality were improved at 60 keV, and the noise and venous pollution were acceptable. Thus, the VMI reconstructions at 60 keV were preferably chosen over other VMI series in objective and subjective assessments. These results are consistent with previous results in which 60-keV VMI reconstructions showed the best image quality in the visualization of lower extremity vessels (27,37). However, Sudarski et al. (27) also pointed out that VMI reconstructions at 70 keV received the best overall image quality compared to virtual 120-kV data sets of the abdominal arteries. Albrecht et al. (30) showed optimal visualization of small arterial branches at 40 keV for VMI reconstructions and yielded the highest score in contrast conditions at 50 keV in thoracic and abdominal CTA. These differences may be related to the amount of tissue around the vessels and the diameter of the vessels. The lower extremity with comparatively little surrounding soft tissue is subject to fewer scattering artifacts, allowing the use of lower keV images to provide higher attenuation for improving CNR. However, the determination for visualization of the perforators, a kind of small vessel, needs considerably less image noise, fewer artifacts, lower venous contamination, and comparably higher CNR to provide increased visualization and diagnostic confidence. The perforator assessment with improved attenuation and CNR derived from 60-keV reconstructions in DE-CTA could achieve the best visual effect after MIP reconstructions to improve diagnostic confidence. Therefore, we prefer VMI reconstructions at 60 keV for visualization of perforators in the FFF harvest.
Our study had several limitations. First, our study was a single-center study with a relatively small sample size. Larger samples are required to validate our preliminary results in a future study. Second, a 20-keV energy interval for VMI reconstructions in this study might have missed a few details, and we did not evaluate the impact of the algorithms on our results. Lastly, the diagnostic accuracy of the visualization of the lower extremity artery and perforators by DSA and VMI should be compared in further research.
Conclusions
Our results found that VNC images reconstructed from DECT can be used to replace the M_0.5-TNC images to reduce radiation dose and provide acceptable images. Furthermore, the present study shows that a low-keV VMI series of DE-CTA can improve vascular contrast, SNR, CNR, image quality, and diagnostic confidence compared with M_0.5-C images. VMI reconstructions at 60 keV provide the optimal image quality with considerably improved attenuation and CNR, demonstrating the potential to improve the visualization of lower extremity arteries and their perforators for FFF harvest.
Acknowledgments
Funding: None.
Footnote
Reporting Checklist: The authors have completed the STROBE reporting checklist. Available at https://qims.amegroups.com/article/view/10.21037/qims-22-734/rc
Conflicts of Interest: All authors have completed the ICMJE uniform disclosure form (available at https://qims.amegroups.com/article/view/10.21037/qims-22-734/coif). The authors have no conflicts of interest to declare.
Ethical Statement: The authors are accountable for all aspects of the work in ensuring that questions related to the accuracy or integrity of any part of the work are appropriately investigated and resolved. The study was conducted in accordance with the Declaration of Helsinki (as revised in 2013). This study was approved by the Institutional Ethics Committee of Sun Yat-sen Memorial Hospital and informed consent was taken from the patients or patients’ legal guardians.
Open Access Statement: This is an Open Access article distributed in accordance with the Creative Commons Attribution-NonCommercial-NoDerivs 4.0 International License (CC BY-NC-ND 4.0), which permits the non-commercial replication and distribution of the article with the strict proviso that no changes or edits are made and the original work is properly cited (including links to both the formal publication through the relevant DOI and the license). See: https://creativecommons.org/licenses/by-nc-nd/4.0/.
References
- Lonie S, Herle P, Paddle A, Pradhan N, Birch T, Shayan R. Mandibular reconstruction: meta-analysis of iliac- versus fibula-free flaps. ANZ J Surg 2016;86:337-42. [Crossref] [PubMed]
- Urken ML, Buchbinder D, Weinberg H, Vickery C, Sheiner A, Parker R, Schaefer J, Som P, Shapiro A, Lawson W, Biller HF. Functional evaluation following microvascular oromandibular reconstruction of the oral cancer patient: A comparative study of reconstructed and nonreconstructed patients. Laryngoscope 2015;125:1512. [Crossref] [PubMed]
- Gryseleyn R, Schlund M, Pigache P, Wojcik T, Raoul G, Ferri J. Influence of preoperative imaging on fibula free flap harvesting. J Stomatol Oral Maxillofac Surg 2017;118:265-70. [Crossref] [PubMed]
- Fliss E, Yanko R, Bracha G, Teman R, Amir A, Horowitz G, Muhanna N, Fliss DM, Gur E, Zaretski A. The Evolution of the Free Fibula Flap for Head and Neck Reconstruction: 21 Years of Experience with 128 Flaps. J Reconstr Microsurg 2021;37:372-9. [Crossref] [PubMed]
- Garvey PB, Chang EI, Selber JC, Skoracki RJ, Madewell JE, Liu J, Yu P, Hanasono MM. A prospective study of preoperative computed tomographic angiographic mapping of free fibula osteocutaneous flaps for head and neck reconstruction. Plast Reconstr Surg 2012;130:541e-9e. [Crossref] [PubMed]
- Kelly AM, Cronin P, Hussain HK, Londy FJ, Chepeha DB, Carlos RC. Preoperative MR angiography in free fibula flap transfer for head and neck cancer: clinical application and influence on surgical decision making. AJR Am J Roentgenol 2007;188:268-74. [Crossref] [PubMed]
- Ribuffo D, Atzeni M, Saba L, Guerra M, Mallarini G, Proto EB, Grinsell D, Ashton MW, Rozen WM. Clinical study of peroneal artery perforators with computed tomographic angiography: implications for fibular flap harvest. Surg Radiol Anat 2010;32:329-34. [Crossref] [PubMed]
- Knitschke M, Baumgart AK, Bäcker C, Adelung C, Roller F, Schmermund D, Böttger S, Howaldt HP, Attia S. Computed Tomography Angiography (CTA) before Reconstructive Jaw Surgery Using Fibula Free Flap: Retrospective Analysis of Vascular Architecture. Diagnostics (Basel) 2021.
- Wales CJ, Morrison J, Drummond R, Devine JC, McMahon J. Pre-operative evaluation of vascularised fibula donor sites: a UK maxillofacial e-survey. Br J Oral Maxillofac Surg 2010;48:192-4. [Crossref] [PubMed]
- Battaglia S, Maiolo V, Savastio G, Zompatori M, Contedini F, Antoniazzi E, Cipriani R, Marchetti C, Tarsitano A. Osteomyocutaneous fibular flap harvesting: Computer-assisted planning of perforator vessels using Computed Tomographic Angiography scan and cutting guide. J Craniomaxillofac Surg 2017;45:1681-6. [Crossref] [PubMed]
- Schuderer JG, Meier JK, Klingelhöffer C, Gottsauner M, Reichert TE, Wendl CM, Ettl T. Magnetic resonance angiography for free fibula harvest: anatomy and perforator mapping. Int J Oral Maxillofac Surg 2020;49:176-82. [Crossref] [PubMed]
- Jin KN, Lee W, Yin YH, Choi SI, Jae HJ, Chung JW, Park JH. Preoperative evaluation of lower extremity arteries for free fibula transfer using MDCT angiography. J Comput Assist Tomogr 2007;31:820-5. [Crossref] [PubMed]
- Lehti L, Söderberg M, Höglund P, Wassélius J. Comparing Arterial- and Venous-Phase Acquisition for Optimization of Virtual Noncontrast Images From Dual-Energy Computed Tomography Angiography. J Comput Assist Tomogr 2019;43:770-4. [Crossref] [PubMed]
- Albrecht MH, Vogl TJ, Martin SS, Nance JW, Duguay TM, Wichmann JL, De Cecco CN, Varga-Szemes A, van Assen M, Tesche C, Schoepf UJ. Review of Clinical Applications for Virtual Monoenergetic Dual-Energy CT. Radiology 2019;293:260-71. [Crossref] [PubMed]
- Ma G, Han D, Dang S, Yu N, Yang Q, Yang C, Jin C, Dou Y. Replacing true unenhanced imaging in renal carcinoma with virtual unenhanced images in dual-energy spectral CT: a feasibility study. Clin Radiol 2021;76:81.e21-7. [Crossref] [PubMed]
- Murray N, Darras KE, Walstra FE, Mohammed MF, McLaughlin PD, Nicolaou S. Dual-Energy CT in Evaluation of the Acute Abdomen. Radiographics 2019;39:264-86. [Crossref] [PubMed]
- Zeng Y, Geng D, Zhang J. Noise-optimized virtual monoenergetic imaging technology of the third-generation dual-source computed tomography and its clinical applications. Quant Imaging Med Surg 2021;11:4627-43. [Crossref] [PubMed]
- Graser A, Johnson TR, Hecht EM, Becker CR, Leidecker C, Staehler M, Stief CG, Hildebrandt H, Godoy MC, Finn ME, Stepansky F, Reiser MF, Macari M. Dual-energy CT in patients suspected of having renal masses: can virtual nonenhanced images replace true nonenhanced images? Radiology 2009;252:433-40. [Crossref] [PubMed]
- Ananthakrishnan L, Rajiah P, Ahn R, Rassouli N, Xi Y, Soesbe TC, Lewis MA, Lenkinski RE, Leyendecker JR, Abbara S. Spectral detector CT-derived virtual non-contrast images: comparison of attenuation values with unenhanced CT. Abdom Radiol (NY) 2017;42:702-9. [Crossref] [PubMed]
- Lacroix M, Mulé S, Herin E, Pigneur F, Richard P, Zegai B, Baranes L, Djabbari M, Brunetti F, de'Angelis N, Laurent A, Tacher V, Kobeiter H, Luciani A. Virtual unenhanced imaging of the liver derived from 160-mm rapid-switching dual-energy CT (rsDECT): Comparison of the accuracy of attenuation values and solid liver lesion conspicuity with native unenhanced images. Eur J Radiol 2020;133:109387. [Crossref] [PubMed]
- Ho LM, Marin D, Neville AM, Barnhart HX, Gupta RT, Paulson EK, Boll DT. Characterization of adrenal nodules with dual-energy CT: can virtual unenhanced attenuation values replace true unenhanced attenuation values? AJR Am J Roentgenol 2012;198:840-5. [Crossref] [PubMed]
- Lennartz S, Laukamp KR, Tandon Y, Jordan M, Große Hokamp N, Zopfs D, Pennig L, Obmann M, Gilkeson RC, Herrmann KA, Ramaiya N, Gupta A. Abdominal vessel depiction on virtual triphasic spectral detector CT: initial clinical experience. Abdom Radiol (NY) 2021;46:3501-11. [Crossref] [PubMed]
- Neuhaus V, Große Hokamp N, Abdullayev N, Maus V, Kabbasch C, Mpotsaris A, Maintz D, Borggrefe J. Comparison of virtual monoenergetic and polyenergetic images reconstructed from dual-layer detector CT angiography of the head and neck. Eur Radiol 2018;28:1102-10. [Crossref] [PubMed]
- Nomura T, Niwa T, Ozawa S, Imai Y, Hashimoto J. Visibility of bronchial arteries using virtual and advanced virtual monoenergetic imaging. Acta Radiol 2020;61:1618-27. [Crossref] [PubMed]
- Schabel C, Bongers M, Sedlmair M, Korn A, Grosse U, Mangold S, Claussen CD, Thomas C. Assessment of the hepatic veins in poor contrast conditions using dual energy CT: evaluation of a novel monoenergetic extrapolation software algorithm. Rofo 2014;186:591-7. [Crossref] [PubMed]
- Ren H, Zhen Y, Gong Z, Wang C, Chang Z, Zheng J. Feasibility of low-dose contrast media in run-off CT angiography on dual-layer spectral detector CT. Quant Imaging Med Surg 2021;11:1796-804. [Crossref] [PubMed]
- Sudarski S, Apfaltrer P, Nance JW Jr, Schneider D, Meyer M, Schoenberg SO, Fink C, Henzler T. Optimization of keV-settings in abdominal and lower extremity dual-source dual-energy CT angiography determined with virtual monoenergetic imaging. Eur J Radiol 2013;82:e574-81. [Crossref] [PubMed]
- Arendt CT, Czwikla R, Lenga L, Wichmann JL, Albrecht MH, Booz C, Martin SS, Leithner D, Tischendorf P, Blandino A, Vogl TJ, D'Angelo T. Improved coronary artery contrast enhancement using noise-optimised virtual monoenergetic imaging from dual-source dual-energy computed tomography. Eur J Radiol 2020;122:108666. [Crossref] [PubMed]
- Lv P, Liu J, Wu R, Hou P, Hu L, Gao J. Use of non-linear image blending with dual-energy CT improves vascular visualization in abdominal angiography. Clin Radiol 2014;69:e93-9. [Crossref] [PubMed]
- Albrecht MH, Trommer J, Wichmann JL, Scholtz JE, Martin SS, Lehnert T, Vogl TJ, Bodelle B. Comprehensive Comparison of Virtual Monoenergetic and Linearly Blended Reconstruction Techniques in Third-Generation Dual-Source Dual-Energy Computed Tomography Angiography of the Thorax and Abdomen. Invest Radiol 2016;51:582-90. [Crossref] [PubMed]
- Parakh A, Lennartz S, An C, Rajiah P, Yeh BM, Simeone FJ, Sahani DV, Kambadakone AR, Dual-Energy CT. Images: Pearls and Pitfalls. Radiographics 2021;41:98-119. [Crossref] [PubMed]
- Marin D, Boll DT, Mileto A, Nelson RC. State of the art: dual-energy CT of the abdomen. Radiology 2014;271:327-42. [Crossref] [PubMed]
- Zhang X, Zhang G, Xu L, Bai X, Lu X, Yu S, Sun H, Jin Z. Utilisation of virtual non-contrast images and virtual mono-energetic images acquired from dual-layer spectral CT for renal cell carcinoma: image quality and radiation dose. Insights Imaging 2022;13:12. [Crossref] [PubMed]
- De Cecco CN, Darnell A, Macías N, Ayuso JR, Rodríguez S, Rimola J, Pagés M, García-Criado A, Rengo M, Laghi A, Ayuso C. Virtual unenhanced images of the abdomen with second-generation dual-source dual-energy computed tomography: image quality and liver lesion detection. Invest Radiol 2013;48:1-9. [Crossref] [PubMed]
- Botsikas D, Triponez F, Boudabbous S, Hansen C, Becker CD, Montet X. Incidental adrenal lesions detected on enhanced abdominal dual-energy CT: can the diagnostic workup be shortened by the implementation of virtual unenhanced images? Eur J Radiol 2014;83:1746-51. [Crossref] [PubMed]
- Liang HW, Zhou Y, Zhang ZW, Yan GW, Du SL, Zhang XH, Li XY, Lv FJ, Zheng Q, Li YM. Dual-energy CT with virtual monoenergetic images to improve the visualization of pancreatic supplying arteries: the normal anatomy and variations. Insights Imaging 2022;13:21. [Crossref] [PubMed]
- Ren H, Zhen Y, Gong Z, Wang C, Chang Z, Zheng J. Assessment of Virtual Monoenergetic Images in Run-off Computed Tomography Angiography: A Comparison Study to Conventional Images From Spectral Detector Computed Tomography. J Comput Assist Tomogr 2021;45:232-7. [Crossref] [PubMed]
- Zhao L, Li F, Zhang Z, Zhang Z, Jiang Y, Wang X, Gu J, Li D. Assessment of an advanced virtual monoenergetic reconstruction technique in cerebral and cervical angiography with third-generation dual-source CT: Feasibility of using low-concentration contrast medium. Eur Radiol 2018;28:4379-88. [Crossref] [PubMed]
- Delesalle MA, Pontana F, Duhamel A, Faivre JB, Flohr T, Tacelli N, Remy J, Remy-Jardin M. Spectral optimization of chest CT angiography with reduced iodine load: experience in 80 patients evaluated with dual-source, dual-energy CT. Radiology 2013;267:256-66. [Crossref] [PubMed]
- Albrecht MH, Scholtz JE, Hüsers K, Beeres M, Bucher AM, Kaup M, Martin SS, Fischer S, Bodelle B, Bauer RW, Lehnert T, Vogl TJ, Wichmann JL. Advanced image-based virtual monoenergetic dual-energy CT angiography of the abdomen: optimization of kiloelectron volt settings to improve image contrast. Eur Radiol 2016;26:1863-70. [Crossref] [PubMed]