Diagnostic and therapeutic performances of three score-based Thyroid Imaging Reporting and Data Systems after application of equal size thresholds
Introduction
Ultrasound (US) has long been recognized as the most effective method for detecting and characterizing thyroid nodules (1). Over the past two decades, professional organizations and other groups have developed a multitude of independent risk stratification systems (RSSs) (2-6). However, a worldwide communicable RSS does not presently exist.
In 2017, the American College of Radiology (ACR) proposed a 5-tier approach based on a quantitative scoring system (2), referred to as the ACR Thyroid Imaging Reporting and Data System (TIRADS), which showed superior diagnostic performance compared to other RSSs (7-11). However, concerns have been raised that the ACR TIRADS implements a quite complicated calculation algorithm. In 2019, Wildman-Tobriner et al. (4) proposed the artificial intelligence (AI) TIRADS that uses an AI algorithm to simplify the ACR TIRADS. The simple version of the AI TIRADS eliminates 6 scores and decreases 2 scores of US features. Recent studies have proven that the AI TIRADS has a better diagnostic performance and yields a lower unnecessary fine-needle aspiration (FNA) rate (UFR) compared with the ACR TIRADS (12-14).
The ACR TIRADS and the TIRADS proposed by Kwak (Kwak TIRADS) are score-based TIRADS. In contrast to the ARC TIRADS, the Kwak TIRADS applies points for all suspicious US features, and these points are added up to give a numeric score leading to a final category (15), an approach which has been proven to be practical and easily applicable (4,15). Recent studies have demonstrated that the diagnostic performance of the Kwak TIRADS was superior to that of the ACR TIRADS, without considering the size thresholds for FNA (16,17). Meanwhile, the Kwak TIRADS, which incorporates the size thresholds of the ACR TIRADS for FNA, showed higher diagnostic performance and a lower UFR than did the ACR TIRADS (6,18-20). The ACR TIRADS provides 2 different size thresholds for each category to determines the therapeutic recommendations of FNA: follow-up or no further evaluation (NFE). However, few studies have compared the diagnostic and therapeutic performances of the above 3 TIRADS in follow-up and NFE.
Therefore, the purpose of our study was to compare the diagnostic and therapeutic managerial performance (including FNA, follow-up and NFE) using 3 score-based TIRADSs (the ACR, AI, and Kwak TIRADS) and to apply the size threshold of the ACR TIRADS to FNA and follow-up. We present the following article in accordance with the STARD reporting checklist (available at https://qims.amegroups.com/article/view/10.21037/qims-22-592/rc).
Methods
The study was conducted in accordance with the Declaration of Helsinki (as revised in 2013). The Scientific Research and Clinical Trials Ethics Committee of the First Affiliated Hospital of Zhengzhou University of China (No. 2022-KY-0974-001) approved this study and granted a waiver of written informed consent for use of data due to the retrospective nature of the study.
Patients
This study was conducted from January 2010 to August 2017, during which time 4,022 nodules were identified in 2,714 consecutive patients who underwent US examinations followed by US-FNA or surgery to diagnose thyroid nodules at our institution. Of the 4,022 nodules, 189 were excluded due to a lack of definitive cytopathologic results or incomplete US imaging data (Figure 1).
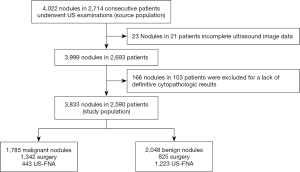
US examination and image analysis
Thyroid US was performed with a 5 to 12-MHz linear array transducer and a real-time US system (Aplio-300; Toshiba Corporation, Tokyo, Japan). US examinations were performed by a senior radiologist with 33 years of experience in thyroid imaging. All the US examinations complied with the American Institute of Ultrasound in Medicine (AIUM) protocol for thyroid scanning. US images of the thyroid nodules were acquired by carefully scanning the thyroid and adjacent tissues, both transversely and longitudinally. US features of the thyroid nodules that underwent US-FNA or surgery in 2 weeks were prospectively recorded by the radiologist according to composition, echogenicity, margins, calcification, and shape, which is similar to the ACR lexicon for describing thyroid nodules.
US features were used to classify thyroid nodules in the ACR, AI, and Kwak TIRADS (Figure 2). Points were assigned to each nodule for the separate categories according to the different TIRADS guidelines (2,4,15). The sum of the points in each guideline determined the TIRADS level assigned to each nodule. According to the relevant guidelines, the Kwak TIRADS is divided into TR 3 (0 point), TR 4A (1 point), TR 4B (2 points), TR 4C (3–4 points), and TR 5 (5 points), while the ACR/AI TIRADS is divided into TR 1 (0–1 point), TR 2 (2 points), TR 3 (3 points), TR 4 (4–6 points), and TR 5 (≥7 points). Identification and evaluation of US features were determined retrospectively by 2 radiologists (with 13 and 12 years, respectively, of clinical experience in performing thyroid US scans and evaluating thyroid US images), with any disagreements being resolved by discussing it again and getting a unified result. The reviewers had no knowledge of the final pathological diagnosis, and they assessed the US features of the thyroid nodules according to the guidelines for lesion reporting published in the 2017 ARC TIRADS white paper.
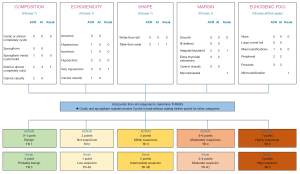
We applied the size thresholds proposed by the ACR TIRADS to the Kwak TIRADS according to the similar estimated malignancy rates (2,15). Table 1 shows the recommended size thresholds for biopsy and follow-up in the 3 TIRADSs. In our study, we defined the cutoffs that were suspicious for malignancy as ≥4 for the AI and ACR TIRADS and as ≥4B for the Kwak TIRADS. Additionally, thyroid nodules were classified as FNA or no-FNA nodules according to the size thresholds of the ACR TIRADS for FNA. Furthermore, no-FNA nodules were divided into follow-up and NFE according to the thresholds of the ACR TIRADS for follow-up.
Table 1
Suspicious | Recommended size thresholds | ACR/AI | Kwak |
---|---|---|---|
Mildly suspicious | FNA if ≥2.5 cm | TR 3 | TR 4A |
Follow if ≥1.5 cm | |||
Moderately suspicious | FNA if ≥1.5 cm | TR 4 | TR 4B |
Follow if ≥1.0 cm | |||
Highly suspicious | FNA if ≥1.0 cm | TR 5 | TR 4C |
Follow if ≥0.5 cm | TR 5 |
ACR, American College of Radiology; AI, artificial intelligence; TIRADS, Thyroid Imaging Reporting and Data System; FNA, fine needle aspiration; TR, Thyroid Imaging Reporting and Data System category.
Data and statistical analysis
The UFR was calculated as the proportion of benign nodules in the nodules recommended for FNA. The missed cancer rate (MCR) was calculated as the percentage of all malignant nodules recommended for NFE. The follow-up rate (FUR) was calculated as the proportion of follow-up nodules in the no-FNA nodules. The false-negative rate (FNR) was calculated as the proportion of no-FNA malignant nodules in all malignant nodules.
The demographics between patients with benign and malignant nodules were compared using the independent 2-sample t-test for continuous data and the chi-squared test for categorical data. All quantitative values are expressed as mean ± standard deviation (SD). We evaluated the diagnostic performance of the 3 TIRADSs, including specificity, sensitivity, positive predictive value (PPV), negative predictive value (NPV), and accuracy with 95% confidence intervals (CIs). We also evaluated the clinical management performance including the FNA rate (FNAR), UFR, FNR, and MCR. The above diagnostic and clinical management performances were compared using McNemar test or DeLong test. Areas under the receiver operating characteristic curve (AUCs) were calculated and compared using the DeLong method. The statistical analysis was performed with SPSS 26.0 (IBM Corp., Armonk, NY, USA) software and MedCalc 18.2.1 (MedCalc Software Ltd., Ostend, Belgium) software. A two-sided P value of <0.05 was considered to be statistically significant.
Results
Baseline clinicopathological characteristics
A total of 3,833 thyroid nodules in 2,590 patients were included in our study. Demographics of the patients and nodules are summarized in Table 2. There were more female patients than male patients (1,979 vs. 611; P<0.001). Patients with benign thyroid nodules were significantly older than patients with malignant nodules (mean 49.6±11.6 vs. 45.0±11.6 years; P=0.56). Malignant thyroid nodules were significantly smaller than benign nodules (mean 12.6±11.7 vs. 20.4±15.8 mm; P<0.001). Papillary thyroid carcinomas were the most frequently excised malignant nodules, with the number of excisions by malignant nodule type recorded as follows: 1,707 papillary thyroid carcinomas, 35 follicular carcinomas, 20 medullary carcinomas, and 23 others. Nodular goiters were the most commonly excised benign nodules, with the number of excisions by benign nodule type recorded as follows: 1,802 nodular goiters, 42 adenomas, 81 Hashimoto thyroiditis nodules, 41 inflammatory lesions, and 82 others).
Table 2
Parameter | Total | Malignant | Benign | P value |
---|---|---|---|---|
Sex | <0.001 | |||
Male | 611 (23.6) | 282 (22.6) | 329 (24.5) | |
Female | 1,979 (76.4) | 965 (77.4) | 1,014 (75.5) | |
Nodules | 3,833 | 1,785 (46.6) | 2,048 (53.4) | |
Age (years) | 47.2±12.1 | 45.0±11.6 | 49.6±11.6 | 0.56 |
Nodule size (mm) | 16.7±14.5 | 12.6±11.7 | 20.4±15.8 | <0.001 |
Values are presented as mean ± standard deviation or n (%).
US features of the nodules are summarized in Table 3. Among the 1,785 malignant nodules, surgery was performed on 1,342 nodules and FNA was performed on the remaining nodules. Among the 2,048 benign modules, surgery was performed on 825 nodules and FNA was performed on the remaining nodules. Almost all of the malignant nodules were solid or almost completely solid (92.5%). Compared to the benign modules, the malignant nodules were hypoechoic (80.8% vs. 41.2%), their margins were more often irregular or lobulated (65.8% vs. 13.6%), and they showed more punctate echogenic foci (56.1% vs. 12.5%). All suspicious features documented on thyroid US were significantly more frequent in the malignant nodules than in the benign nodules.
Table 3
Parameter | Total, n (%) | Malignant, n (%) | Benign, n (%) | P value |
---|---|---|---|---|
Composition | <0.001 | |||
Cystic | 91 (2.4) | 9 (0.5) | 82 (4.0) | |
Mixed cystic and solid | 1,114 (29.1) | 124 (6.9) | 990 (48.3) | |
Solid or almost solid | 2,622 (68.4) | 1,652 (92.5) | 970 (47.3) | |
Spongiform | 6 (0.2) | 0 (0) | 6 (0.3) | |
Echogenicity | <0.001 | |||
Anechoic | 91 (2.4) | 9 (0.5) | 82 (4.0) | |
Hyperechoic | 1,255 (32.7) | 173 (9.7) | 1,082 (52.8) | |
Hypoechoic | 2,286 (59.6) | 1,443 (80.8) | 843 (41.2) | |
Very hypoechoic | 174 (4.5) | 150 (8.4) | 24 (1.2) | |
Cannot be determined | 27 (0.7) | 10 (0.5) | 17 (0.8) | |
Shape | <0.001 | |||
Not taller than wide | 3,023 (78.9) | 1,147 (64.3) | 1,876 (91.6) | |
Taller than wide | 810 (21.1) | 638 (35.7) | 172 (8.4) | |
Margin | <0.001 | |||
Smooth | 1,299 (33.9) | 139 (7.8) | 1,160 (56.6) | |
Ill-defined | 853 (22.3) | 268 (15.0) | 585 (28.6) | |
Irregular or lobulated | 1,453 (37.9) | 1,174 (65.8) | 279 (13.6) | |
Extrathyroidal extension | 228 (5.9) | 204 (11.4) | 24 (1.2) | |
Echogenic foci | <0.001 | |||
No | 2,098 (54.7) | 594 (33.3) | 1,504 (73.4) | |
Large comet-tail | 70 (1.8) | 3 (0.2) | 67 (3.3) | |
Macrocalcifications | 378 (9.9) | 170 (9.5) | 208 (10.2) | |
Peripheral calcifications | 29 (0.8) | 16 (0.9) | 13 (0.6) | |
Punctate echogenic foci | 1,258 (32.8) | 1,002 (56.1) | 256 (12.5) |
The diagnostic performance according to US-based final assessment categories
To investigate the diagnostic performance of the ACR, AI, and Kwak TIRADS, the sensitivity, specificity, accuracy, PPV, NPV, and AUC were calculated and compared using the McNemar or DeLong methods (Table 4 and Figure 3).
Table 4
TIRADS | SEN (%) | SPE (%) | PPV (%) | NPV (%) | ACC (%) |
---|---|---|---|---|---|
ACR | 96.0 | 57.4 | 66.3 | 94.3 | 75.3 |
N | 1,714/1,875 | 1,175/2,048 | 1,714/2,587 | 1,175/1,246 | 2,889/3,833 |
95% CI | 95.1–96.9 | 55.1–59.5 | 64.6–68.2 | 93.0–95.6 | 74.0–76.9 |
AI | 94.5 | 64.6 | 70.0 | 93.0 | 78.5 |
N | 1,686/1,875 | 1,324/2,048 | 1,686/2410 | 1,324/1,423 | 3,010/3,833 |
95% CI | 93.4–95.4 | 62.7–66.8 | 68.2–71.9 | 91.8–94.2 | 77.3–79.8 |
Kwak | 96.1 | 52.9 | 64.0 | 93.9 | 73.0 |
N | 1,715/1,875 | 1,084/2,048 | 1,715/2,679 | 1,084/1,154 | 2,799/3,833 |
95% CI | 95.1–97.0 | 50.8–55.2 | 62.1–65.8 | 92.5–95.3 | 71.7–74.4 |
ACR, American College of Radiology; AI, artificial intelligence; TIRADS, Thyroid Imaging Reporting and Data System; SEN, sensitivity; SPE, specificity; PPV, positive predictive value; NPV, negative predictive value; ACC, accuracy; N, number; CI, confidence interval.
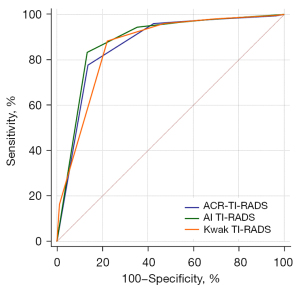
Among the guidelines, specificity, PPV, and accuracy were highest with the AI TIRADS (64.6%, 95% CI: 62.7–66.8%; 70.0%, 95% CI: 68.2–71.9%; and 78.5%, 95% CI: 77.3–79.8%), followed by the ACR TIRADS (57.4%, 95% CI: 55.1–59.5%; 66.3%, 95% CI: 64.6–68.2%; and 75.3%, 95% CI: 74.0–76.9%) and the Kwak TIRADS (52.9%, 95% CI: 50.8–55.2%; 64.0%, 95% CI: 62.1–65.8%; and 73.0%, 95% CI: 71.7–74.4%). The sensitivity was similar among the 3 TIRADSs (AI TIRADS: 94.5%, 95% CI: 93.4–95.4%; ACR TIRADS: 96.0%, 95% CI: 95.1–96.9%; Kwak TIRADS: 96.1%, 95% CI: 95.1–97.0%). The NPV was also similar among the 3 TIRADSs (AI TIRADS: 93.0%, 95% CI: 91.8–94.2%; ACR TIRADS: 94.3%, 95% CI: 93.0–95.6%; Kwak TIRADS: 93.9%, 95% CI: 92.5–95.3%).
Therapeutic performance according to size thresholds of the ACR TIRADS
We evaluated the impact on therapeutic performance using the FNAR, UFR, FUR, FNR, and MCR (Table 5). The AI TIRADS had lower FNAR, UFR, and UR (30.9%, 41.1%, and 34.2%, respectively) than did the ACR TIRADS (34.4%, 47.8%, and 37.7%, respectively) and Kwak TIRADS (36.9%, 48.7%, and 41.0%, respectively), but the FNR was similar among the 3 TIRADS (61.4%, 61.0%, and 59.3%, respectively). Meanwhile, our data showed no significant difference in MCR between the ACR and AI TIRADS (25.8% vs. 24.1%; P>0.05), or between the Kwak and AI TIRADS (21.8% vs. 24.1%; P>0.05). The Kwak TIRADS had a lower MCR (21.8%) than did the ACR TIRADS (25.8%) and the AI TIRADS (24.1%). Furthermore, the number of malignant nodules recommended for follow-up was 635, 657, and 669 for the ACR, AI, and Kwak TIRADS, respectively. The number of malignant nodules recommended for NFE was 424, 370, and 356 for the ACR, AI, and Kwak TIRADS, respectively.
Table 5
Guidelines | FNAR | UFR | FUR | FNR | MCR |
---|---|---|---|---|---|
ACR | 0.34 (1,320/3,833) | 0.48 (631/1,320) | 0.38 (948/2,513) | 0.61 (1,096/1,785) | 0.26 (461/1,785) |
AI | 0.31 (1,183/3,833) | 0.38 (486/1,183) | 0.34 (905/2,650) | 0.61 (1,088/1,785) | 0.24 (431/1,785) |
Kwak | 0.37 (1,416/3,833) | 0.49 (689/1,416) | 0.41 (990/2,417) | 0.59 (1,058/1,785) | 0.22 (389/1,785) |
ACR, American College of Radiology; AI, artificial intelligence; TIRADS, Thyroid Imaging Reporting and Data System; FNAR, FNA rate; UFR, unnecessary FNA rate; FNA, fine needle aspiration; FUR, follow-up rate; FNR, false-negative rate; MCR, missed cancer rate.
Discussion
US is the primary diagnostic tool used in the diagnosis and management of thyroid nodules (21). Previous studies have summarized different ultrasonic signs of thyroid nodules and further proposed TIRADSs to distinguish the degree of malignancy (2-5). However, there is no globally unified RSS. Our study proved that the AI TIRADS has better overall diagnostic performance (AUC and accuracy) and specificity than do the ACR and Kwak TIRADS. Our results also indicated that the AI TIRADS has better clinical management performance (lower FNAR, UFR, and FUR) than do the ACR and Kwak TIRADS using the size thresholds of the ACR TIRADS. In addition, the Kwak TIRADS incorporating the size thresholds of the ACR TIRADS was almost similar to the ACR TIRADS in diagnostic and therapeutic performance. These results suggested that the AI TIRADS could be better at avoiding overdiagnosis and mitigating the risk of potentially missed cancer.
First, we need to acknowledge that our data had a high malignant rate of thyroid nodules; however, this phenomenon also appears in previous studies to varying degrees (22) and arises due to several factors impacting the composition of the selected data. For example, different surgical or FNA indications in the researcher’s hospital may lead to different malignant rates. It may also be closely related to a patient’s own willingness to operate or undergo FNA. In addition, a higher nodule grade on US or distinctive aspects of a patient’s clinical history and examination may also be associated with different malignant rates. Meanwhile, patients are far more likely to undergo resection if there are nondiagnostic, indeterminate, or suspicious findings on cytology.
Our results showed that the AI TIRADS has a better diagnostic performance than do the ACR and Kwak TIRADS in terms of specificity, accuracy, AUC, and PPV. The diagnostic performance was calculated according to the 3 TIRADSs using US feature-based final assessment categories. The US features of the ACR TIRADS were based on a literature review, expert consensus, and the partial analysis of a database of proven nodules. Early studies of this system have been encouraging. The AI TIRADS, which is a simplified version of the ACR TIRADS (4), eliminates 6 and decreases 2 scores of US features. For example, in the composition category, the AI TIRADS suggests that these 2 features be assigned new point values of 0 and that solid nodules be assigned 3 points. This simplified scheme focuses on solid nodules, which is in line with data from Middleton et al. (23) who showed that solid nodules had a 4-time higher risk of malignancy than did mixed cystic and solid thyroid nodules. AI has also been used in other TIRADSs and showed better diagnostic performance (4). For instance, Wang et al. incorporated the Google AutoML, a machine learning algorithm, into the TIRADS scoring system to establish a study model, which improved the performance of the radiologist (24).
In the present study, compared to the ACR and Kwak TIRADS, the AI TIRADS demonstrated better therapeutic performance using the ACR TIRADS, achieving a lower FNAR, UFR, and FUR. On the one hand, the FNAR and UFR were calculated and compared using FNA thresholds. The lower UFR was likely attributable to the specificity and the size of the FNA thresholds (12,25,26). Specificity is the ability of a test to correctly identify people without the disease. Some recent studies demonstrated that the AI TIRADS had a lower UFR, which is in line with our results (12-14). In order to exclude the effect of the size thresholds of FNA, we compared and calculated the therapeutic performance according to the same thresholds (i.e., the thresholds of the ACR TIRADS) (2). On the other hand, the FUR and MCR were calculated and compared using follow-up thresholds. Reducing the UFR may cause a higher MCR and/or FUR. Interestingly, our results showed that the AI TIRADS had a similar MCR to the ACR and Kwak TIRADS but a lower FUR than the ACR and Kwak TIRADS. This observation indicated that more malignant nodules were able to be assigned for follow-up or FNA in the AI TIRADS. In our results, there were at least 697 (39.0%), 689 (38.6%), and 727 (40.7%) malignant nodules indicated as FNA in the AI, ACR, and Kwak TIRADS, respectively. Interestingly, more than one half of the malignant nodules were not categorized as FNA in all 3 guidelines, and when we introduced the concept of follow-up, at least 657 (36.8%), 635 (35.6%), and 669 (37.5%) malignant nodules were indicated for follow-up in the AI, ACR, and Kwak TIRADS, respectively. As to the NFE nodules, our results showed that the majority of missed cancers were smaller than 1 cm in size, in accordance with the study conducted by Middleton et al. (27).
In contrast to the current study, a study by Huh et al. showed that the Kwak TIRADS incorporating the threshold of the ACR TIRADS showed a lower UFR than did the ACR TIRADS (18). The results are likely attributable to the malignant rate and population size in the sample of the study. The malignant rate and the proportion of nodules ≥10 mm were 24.8% and 100%, respectively, in the study by Huh et al., vs. 36.3% and 55.8%, respectively, in our study. Other factors contributing to the results might have been the lack of consensus on the definition of US features. The US descriptors recorded in the study by Huh et al. were not defined by exactly the same definitions. However, we used the definitions of US features according to the ACR’s 207 white paper on the ACR TIRADS.
Some limitations of our study should be considered. First, the surgical series accounted for the majority of patients recruited into this study, which might have led to selection bias. Second, our institution is a tertiary referral center, with most of the patients attending for diagnosis and/or treatment for malignant disease. This fact may lead to the relatively high malignancy rate of thyroid nodules in our study. Third, when US descriptors were recorded in this study, we used the ACR’s definitions of US features. This was not considered during data analysis, and might have led to differences in the final assessments made in real-time examinations.
Conclusions
Our findings suggested that the ACR TIRADS may be simplified by AI (i.e., as the AI TIRADS). Simplification of the ACR TIRADS into the AI TIRADS potentially enhances its applicability, reduces the learning curve required of radiologists, and, moreover, may improve their performance. Our results also indicated that the methods of the score-based TIRADS (counting in the Kwak TIRADS and weighting in the ACR and AI TIRADS) might not determine the diagnostic and therapeutic performance of the TIRADS. In the future, we would need to extend the objective of the present study to demonstrate this phenomenon.
Acknowledgments
Funding: None.
Footnote
Reporting Checklist: The authors have completed the STARD reporting checklist. Available at https://qims.amegroups.com/article/view/10.21037/qims-22-592/rc
Conflicts of Interest: All authors have completed the ICMJE uniform disclosure form (available at https://qims.amegroups.com/article/view/10.21037/qims-22-592/coif). The authors have no conflicts of interest to declare.
Ethical Statement: The authors are accountable for all aspects of the work in ensuring that questions related to the accuracy or integrity of any part of the work are appropriately investigated and resolved. The study was conducted in accordance with the Declaration of Helsinki (as revised in 2013). The Scientific Research and Clinical Trials Ethics Committee of the First Affiliated Hospital of Zhengzhou University of China (No. 2022-KY-0974-001) approved this study and granted a waiver of written informed consent for use of data due to the retrospective nature of the study.
Open Access Statement: This is an Open Access article distributed in accordance with the Creative Commons Attribution-NonCommercial-NoDerivs 4.0 International License (CC BY-NC-ND 4.0), which permits the non-commercial replication and distribution of the article with the strict proviso that no changes or edits are made and the original work is properly cited (including links to both the formal publication through the relevant DOI and the license). See: https://creativecommons.org/licenses/by-nc-nd/4.0/.
References
- Smith-Bindman R, Lebda P, Feldstein VA, Sellami D, Goldstein RB, Brasic N, Jin C, Kornak J. Risk of thyroid cancer based on thyroid ultrasound imaging characteristics: results of a population-based study. JAMA Intern Med 2013;173:1788-96. [Crossref] [PubMed]
- Tessler FN, Middleton WD, Grant EG, Hoang JK, Berland LL, Teefey SA, Cronan JJ, Beland MD, Desser TS, Frates MC, Hammers LW, Hamper UM, Langer JE, Reading CC, Scoutt LM, Stavros AT. ACR Thyroid Imaging, Reporting and Data System (TI-RADS): White Paper of the ACR TI-RADS Committee. J Am Coll Radiol 2017;14:587-95. [Crossref] [PubMed]
- Haugen BR, Alexander EK, Bible KC, Doherty GM, Mandel SJ, Nikiforov YE, Pacini F, Randolph GW, Sawka AM, Schlumberger M, Schuff KG, Sherman SI, Sosa JA, Steward DL, Tuttle RM, Wartofsky L. 2015 American Thyroid Association Management Guidelines for Adult Patients with Thyroid Nodules and Differentiated Thyroid Cancer: The American Thyroid Association Guidelines Task Force on Thyroid Nodules and Differentiated Thyroid Cancer. Thyroid 2016;26:1-133. [Crossref] [PubMed]
- Wildman-Tobriner B, Buda M, Hoang JK, Middleton WD, Thayer D, Short RG, Tessler FN, Mazurowski MA. Using Artificial Intelligence to Revise ACR TI-RADS Risk Stratification of Thyroid Nodules: Diagnostic Accuracy and Utility. Radiology 2019;292:112-9. [Crossref] [PubMed]
- Gharib H, Papini E, Garber JR, Duick DS, Harrell RM, Hegedüs L, Paschke R, Valcavi R, Vitti P. AACE/ACE/AME Task Force on Thyroid Nodules. American Association of Clinical Endocrinologists, American College of Endocrinology, and Associazione Medici Endocrinologi Medical Guidelines For Clinical Practice for the Diagnosis and Management of Thyroid Nodules--2016 Update. Endocr Pract 2016;22:622-39. [PubMed]
- Shin JH, Baek JH, Chung J, Ha EJ, Kim JH, Lee YH, et al. Ultrasonography Diagnosis and Imaging-Based Management of Thyroid Nodules: Revised Korean Society of Thyroid Radiology Consensus Statement and Recommendations. Korean J Radiol 2016;17:370-95. [Crossref] [PubMed]
- Wu XL, Du JR, Wang H, Jin CX, Sui GQ, Yang DY, Lin YQ, Luo Q, Fu P, Li HQ, Teng DK. Comparison and preliminary discussion of the reasons for the differences in diagnostic performance and unnecessary FNA biopsies between the ACR TIRADS and 2015 ATA guidelines. Endocrine 2019;65:121-31. [Crossref] [PubMed]
- Merhav G, Zolotov S, Mahagneh A, Malchin L, Mekel M, Beck-Razi N. Validation of TIRADS ACR Risk Assessment of Thyroid Nodules in Comparison to the ATA Guidelines. J Clin Imaging Sci 2021;11:37. [Crossref] [PubMed]
- Middleton WD, Teefey SA, Reading CC, Langer JE, Beland MD, Szabunio MM, Desser TS. Comparison of Performance Characteristics of American College of Radiology TI-RADS, Korean Society of Thyroid Radiology TIRADS, and American Thyroid Association Guidelines. AJR Am J Roentgenol 2018;210:1148-54. [Crossref] [PubMed]
- Grani G, Lamartina L, Ascoli V, Bosco D, Biffoni M, Giacomelli L, Maranghi M, Falcone R, Ramundo V, Cantisani V, Filetti S, Durante C. Reducing the Number of Unnecessary Thyroid Biopsies While Improving Diagnostic Accuracy: Toward the "Right" TIRADS. J Clin Endocrinol Metab 2019;104:95-102. [Crossref] [PubMed]
- Watkins L, O'Neill G, Young D, McArthur C. Comparison of British Thyroid Association, American College of Radiology TIRADS and Artificial Intelligence TIRADS with histological correlation: diagnostic performance for predicting thyroid malignancy and unnecessary fine needle aspiration rate. Br J Radiol 2021;94:20201444. [Crossref] [PubMed]
- Tan L, Tan YS, Tan S. Diagnostic accuracy and ability to reduce unnecessary FNAC: A comparison between four Thyroid Imaging Reporting Data System (TI-RADS) versions. Clin Imaging 2020;65:133-7. [Crossref] [PubMed]
- Wang YC, Yang B, Huang PF, Xie YD. A comparison between ACR TI-RADS and artificial intelligence TI-RADS regarding to diagnostic efficacy and ability to reduce unnecessary fine-needle aspiration cytology. Chinese Journal of Ultrasonography 2021;30:408-13.
- Kang YN, Fu C, Ma X, Guo YF, Xu CY, Li J, Cui KF. Comparison of Diagnostic Value and Unnecessary FNA Rate of AI TI-RADS and ACR TI-RADS. Chinese Journal of Ultrasound in Medicine 2021;37:367-71.
- Kwak JY, Han KH, Yoon JH, Moon HJ, Son EJ, Park SH, Jung HK, Choi JS, Kim BM, Kim EK. Thyroid imaging reporting and data system for US features of nodules: a step in establishing better stratification of cancer risk. Radiology 2011;260:892-9. [Crossref] [PubMed]
- Gao L, Xi X, Jiang Y, Yang X, Wang Y, Zhu S, Lai X, Zhang X, Zhao R, Zhang B. Comparison among TIRADS (ACR TI-RADS and KWAK- TI-RADS) and 2015 ATA Guidelines in the diagnostic efficiency of thyroid nodules. Endocrine 2019;64:90-6. [Crossref] [PubMed]
- Shen Y, Liu M, He J, Wu S, Chen M, Wan Y, Gao L, Cai X, Ding J, Fu X. Comparison of Different Risk-Stratification Systems for the Diagnosis of Benign and Malignant Thyroid Nodules. Front Oncol 2019;9:378. [Crossref] [PubMed]
- Huh S, Yoon JH, Lee HS, Moon HJ, Park VY, Kwak JY. Comparison of diagnostic performance of the ACR and Kwak TIRADS applying the ACR TIRADS' size thresholds for FNA. Eur Radiol 2021;31:5243-50. [Crossref] [PubMed]
- Migda B, Migda M, Migda AM, Bierca J, Slowniska-Srzednicka J, Jakubowski W, Slapa RZ. Evaluation of Four Variants of the Thyroid Imaging Reporting and Data System (TIRADS) Classification in Patients with Multinodular Goitre - initial study. Endokrynol Pol 2018;69:156-62. [PubMed]
- Chandramohan A, Khurana A, Pushpa BT, Manipadam MT, Naik D, Thomas N, Abraham D, Paul MJ. Is TIRADS a practical and accurate system for use in daily clinical practice? Indian J Radiol Imaging 2016;26:145-52. [Crossref] [PubMed]
- Yim Y, Na DG, Ha EJ, Baek JH, Sung JY, Kim JH, Moon WJ. Concordance of Three International Guidelines for Thyroid Nodules Classified by Ultrasonography and Diagnostic Performance of Biopsy Criteria. Korean J Radiol 2020;21:108-16. [Crossref] [PubMed]
- Zhu H, Yang Y, Wu S, Chen K, Luo H, Huang J. Diagnostic performance of US-based FNAB criteria of the 2020 Chinese guideline for malignant thyroid nodules: comparison with the 2017 American College of Radiology guideline, the 2015 American Thyroid Association guideline, and the 2016 Korean Thyroid Association guideline. Quant Imaging Med Surg 2021;11:3604-18. [Crossref] [PubMed]
- Middleton WD, Teefey SA, Reading CC, Langer JE, Beland MD, Szabunio MM, Desser TS. Multiinstitutional Analysis of Thyroid Nodule Risk Stratification Using the American College of Radiology Thyroid Imaging Reporting and Data System. AJR Am J Roentgenol 2017;208:1331-41. [Crossref] [PubMed]
- Wang S, Xu J, Tahmasebi A, Daniels K, Liu JB, Curry J, Cottrill E, Lyshchik A, Eisenbrey JR. Incorporation of a Machine Learning Algorithm With Object Detection Within the Thyroid Imaging Reporting and Data System Improves the Diagnosis of Genetic Risk. Front Oncol 2020;10:591846. [Crossref] [PubMed]
- Kim PH, Suh CH, Baek JH, Chung SR, Choi YJ, Lee JH. Unnecessary thyroid nodule biopsy rates under four ultrasound risk stratification systems: a systematic review and meta-analysis. Eur Radiol 2021;31:2877-85. [Crossref] [PubMed]
- Ha SM, Baek JH, Na DG, Suh CH, Chung SR, Choi YJ, Lee JH. Diagnostic Performance of Practice Guidelines for Thyroid Nodules: Thyroid Nodule Size versus Biopsy Rates. Radiology 2019;291:92-9. [Crossref] [PubMed]
- Middleton WD, Teefey SA, Tessler FN, Hoang JK, Reading CC, Langer JE, Beland MD, Szabunio MM, Desser TS. Analysis of Malignant Thyroid Nodules That Do Not Meet ACR TI-RADS Criteria for Fine-Needle Aspiration. AJR Am J Roentgenol 2021;216:471-8. [Crossref] [PubMed]