Impact of tumour region of interest delineation method for mid-treatment FDG-PET response prediction in head and neck squamous cell carcinoma undergoing radiotherapy
Introduction
Definitive radiotherapy is a standard treatment for mucosal head and neck squamous cell carcinoma (HNSCC). Following treatment, up to 15–50% of patients still experience locoregional recurrence within the first 2 years (1,2). The treatment is also associated with significant toxicities impacting on quality of life. Hence, there is a need for personalised response adaptation by escalation or de-escalation of treatment based on risk of recurrence.
Studies have attempted to prognosticate treatment response based on anatomical changes in tumour volumes measured using mid-treatment computed tomography (CT) scans (3). Changes in functional imaging has been shown to precede anatomical changes in the tumour during treatment (4). 18F-fluoro-2-deoxy-D-glucose positron emission tomography (FDG-PET) provides an non-invasive method to characterise tumour biology (5). Several studies have shown FDG-PET performed during radiotherapy can be used as an early treatment response biomarker to predict recurrence in head and neck squamous cell carcinoma (6-9). These studies show relative change in volumetric FDG-PET parameters such as metabolic tumour volume (MTV) and total lesion glycolysis (TLG) to be optimal measures for response prediction (6,8,9). However, a critical factor on the derived FDG-PET parameters is the dependence on tumour region of interest (ROI) delineation method employed (5,10-15). Currently, there is considerable heterogeneity and a lack of consensus in the use of ROI delineation methodology in different published studies in head and neck squamous cell carcinoma (16,17). Fixed threshold PET metabolic volume segmentation method is the most commonly used method to derive PET metabolic volumes to provide prognostic biomarkers and to predict treatment outcomes in pre-treatment setting for head and neck cancer. In a meta-analysis of the prognostic value of pre-therapeutic MTV and TLG in head and neck cancer, nine of 13 studies used a fixed threshold, and eight of those used SUV cut off of 2.5 (8). They found a higher correlation to clinical outcomes using SUV cut off value of 2.5 (HR: 3.2 vs. 2.8).
Each method is expected to provide different quantitative measures depending on tumour size, shape, heterogeneity, tumour avidity and background FDG-PET avidity (10,15,18). Mid-treatment FDG-PET assessment during radiotherapy is further complicated by influence of treatment related inflammation and changing tumour morphology especially in complex anatomical head and neck regions (17). The data is much more limited to support the optimal PET segmentation method to be employed during radiotherapy to predict treatment outcomes, with most using threshold method (fixed, relative, background or adaptive) and/or qualitative (16). The data is lacking on the comparative effectiveness with gradient based segmentation method, despite its potential advantage over the threshold-based method in mid-treatment setting where the tumour tends to be smaller and with lower metabolic activity.
There are currently no studies that have explored the influence of different tumour ROI delineation methods in mid-treatment response assessment in head and neck squamous cell carcinoma. The additional value of mid-treatment FDG-PET over anatomical tumour volume change has also not been shown in this patient population.
Our hypothesis is that it is feasible to use gradient based method to assess metabolic PET tumour response during radiotherapy and choice of ROI delineation method can result in significantly different FDG-PET parameters extracted from baseline and mid-treatment FDG-PET imaging. We aim to measure the agreement in FDG-PET parameters extracted from baseline and week 3 FDG-PET using different ROI delineation methodology. We also aim to find the optimal tumour ROI delineation method for treatment response prediction in HNSCC by correlating resulting quantitative FDG-PET parameters to locoregional clinical outcomes. We present the following article in accordance with the STARD reporting checklist (available at https://qims.amegroups.com/article/view/10.21037/qims-22-798/rc).
Methods
Study design
Patients with newly diagnosed, biopsy-proven, non-metastatic mucosal head and neck squamous cell carcinoma treated with definitive radiotherapy with or without concurrent systemic therapy from two separate prospective quantitative imaging biomarker studies were evaluated (Figure 1) (19,20). Patients less than 18 years old, with previous radiotherapy in the head and neck region or contraindications to MRI or FDG-PET were excluded. The study was conducted in accordance with the Declaration of Helsinki (as revised in 2013). The study was approved by the South Western Sydney Local Health District research ethics committee and informed consent was taken from all individual participants. Trial ID ACTRN12616000534482, ANZCTR.
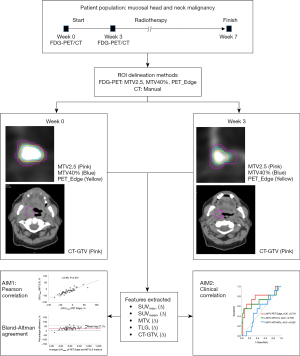
All patients were evaluated and reviewed by a multidisciplinary team consisting of radiation oncologists, medical oncologists, surgeons and radiologists. Patients were treated using an IMRT simultaneous integrated boost technique using daily image guidance over 35 fractions. Patients were treated at two tertiary hospitals from 2014 to 2019. Radiotherapy treatment volumes were defined using latest consensus international guidelines and underwent a stringent peer review process (21). All patients underwent radiotherapy to the primary site and bilateral neck.
All patients underwent FDG-PET before (week 0) and during (week 3) radiotherapy. Week 3 time-point was chosen based on previous published series from our institution and other centres (6,22-24). Week 3 time point is also early enough during radiotherapy to allow sufficient time for treatment adaptation in future clinical trials. Treatment response was evaluated with post-treatment FDG-PET and clinical examination including nasoendoscopy. Recurrences were confirmed histologically or via imaging following discussion at a multidisciplinary head and neck meeting.
The primary outcome for the study was 2-year locoregional recurrence measured from time of diagnosis. A 2-year time point was chosen because the vast majority of locoregional recurrences occur within this period (25).
PET/CT acquisition
PET studies were acquired in radiotherapy treatment position on a GE DiscoveryTM MI 5-ring digital positron emission tomography (PET)-CT (GE Healthcare, Waukesha, MI). Patients received 2.2 MBq/kg of 18F-FDG after at least 4 h of fasting. The average blood sugar level was 5.7±1.2 mmol/L (range, 3.3–9.6 mmol/L). The staging and all sequential posttreatment scans were performed on the same scanner with the same acquisition and reconstruction protocols. The PET studies were acquired in three-dimensional (3D) mode with the patient lying on a radiotherapy flat-bed insert for a total acquisition time of 1.5–2.5 min per bed position adjusted according to the patient weight, from vertex to proximal femora at about 1-hour post injection. Transmission scans and attenuation corrections were obtained using 128-slice GE CT, using helical mode without the use of a contrast medium. The PET images were reconstructed using a GE VUE Point FX (Time of Flight) algorithm into a 256×256 matrix size with a slice thickness of 3.75 to 4.0 mm. The PET images were reconstructed using GE VUE Point FX (Time of Flight) algorithm into a 256×256 matrix size with a slice thickness of 3.75 to 4.0 mm. CT images were acquired at 3.75 to 5 mm slice thickness and reconstructed to a transaxial matrix size of 512×512. The current (30–40 mAs) and voltage (120–140 kV) were varied according to the patient weight. The baseline and mid-treatment scans were performed on the same scanner with the same acquisition and reconstruction protocols.
Image analysis
Primary region of interest (ROI) was the primary tumour. All PET images were viewed and had ROIs delineated using MIM Software (MIM Software Inc.; Beachwood, OH).
The primary tumours were volumetrically delineated by a radiation oncologist (YT) in consensus with a nuclear medicine physician (PL) on FDG-PET imaging and another radiation oncologist (ML) on CT imaging at baseline and week 3 mid-treatment who were blinded to clinical outcomes.
To explore the effect of various delineation methods on metabolic PET parameters, a tumour region of interest (ROI) was delineated using three commonly utilized semi-automated methods; a fixed SUV threshold, a relative threshold and a gradient based method (6,7,12,13). The fixed SUV threshold involved applying an isocontour of SUV =2.5 (‘MTV2.5’), this cut-off was chosen based on previous published literature (6,8,12,13,22). MTV2.5 was delineated using SUV threshold of 2.5 as the lowest limit of the segmentation criteria within a spherical volume of interest containing the tumor. The spherical volume was chosen to visually include the entire tumour and manual adjustments were only rarely allowed to exclude obvious non-involved regions of FDG-PET uptake. For relative threshold method, a 40% of maximum standardized uptake value (‘MTV40%’) was chosen based on a large prospective study and multiple previous clinical series (7,13,14,18,26-29). MTV40% delineated all voxels with SUV values above or equal to 40% of the maximum SUV within the same spherical volume of interest containing the tumor. A semi-automatic gradient based method involved applying a ‘PET Edge’ tool of the MIM software, this has been shown to closely approximate manual segmentation and pathological tumour volume (30,31). PET Edge gradient method calculates spatial derivatives along tumor radii then defines the tumor edge based on derivative levels and continuity of the tumor edge (32). PET Edge method was used as described previously by Werner-Wasik et al. (33). In brief, the method involved the clinician selecting the image slice where the tumour appears the largest, then placing a starting point near the center of the tumour. The clinician then drags out from the center of the lesion; spatial gradients are calculated along each axis interactively and the length of an axis is restricted when a large spatial gradient is calculated along that axis approximating the boundaries of the tumour. Following release of the mouse button the edges of the structure are automatically calculated and outlined volumetrically. Tumour volume was also manually delineated on CT images (CT-GTV) to compare the change in anatomical CT volumes to FDG-PET tumour volumes. An example of different delineated ROIs are provided in Figure 2.
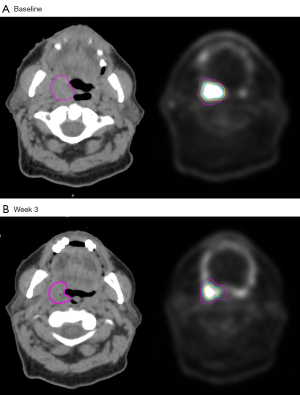
DICOM images containing ROIs were subsequently analysed using open-source PyRadiomics software (v2.2.0) (34). Four metabolic parameters; the maximum SUV uptake (SUVmax), and volumetric parameters mean SUV uptake (SUVmean), MTV and tumour lesion glycolysis (TLG = SUVmean × MTV) were measured for all three ROI delineation methods at both time points.
Percentage change (∆) in FDG-PET imaging parameters from baseline was calculated, defined as ∆ = [(week 3 − week 0)/week 0] × 100%.
Statistical analysis
Due to non-normal data distribution, PET parameter values from different ROI delineation methods were compared using the Wilcoxon signed-rank test.
Correlation between the delineation methods for absolute PET parameter values at baseline and relative change at week 3 were estimated using Pearson coefficients test. The level of agreement between different ROI delineation methods was determined using Bland-Altman analysis.
The absolute value and change (∆) in parameters were compared to locoregional recurrence using Mann-Whitney U test. For parameters with predictive value, receiver operator characteristic (ROC) analysis was performed using area under the curve (AUC) as an index of accuracy to differentiate between multiple predictive parameters. Optimal cut-off values for analysis were derived from the ROC curves aiming for best sensitivity and specificity by applying the Youden index (35). Locoregional recurrence-free survival (LRFS) curves were estimated using Kaplan-Meier analysis and compared using the log-rank (Mantel-Cox) test.
The data were analyzed using SPSS statistical software (Version 24.0; IBM Corp, Armonk, NY, USA). Statistical significance was considered as P<0.05.
Results
A total of 54 patients underwent week 0 pre-treatment FDG-PET. Two patients did not undergo week 3 mid-treatment FDG-PET resulting in 52 patients who were available for subsequent analysis. Patient and tumour details are summarised in Table 1. The median follow up was 31.2 months (range, 4–68 months). A total of 12 patients (22.2%) experienced locoregional recurrence.
Table 1
Characteristics | Patients (n=52) | Nil LRR (n=40) | LRR (n=12) | P value |
---|---|---|---|---|
Age at diagnosis (years) | 62±9.4 | 61±9.9 | 66±6.4 | 0.038 |
Gender | 0.487 | |||
Male | 48 [89] | 38 [79] | 10 [21] | |
Female | 6 [11] | 4 [67] | 2 [33] | |
Smoker | 0.934 | |||
No | 14 [26] | 11 [79] | 3 [21] | |
Yes | 40 [74] | 31 [78] | 9 [23] | |
Alcohol intake | 0.327 | |||
Nil | 13 [24] | 11 [85] | 2 [15] | |
<1 SD/day | 16 [30] | 11 [69] | 5 [31] | |
1–3 SD/day | 7 [13] | 7 [100] | 0 [0] | |
>3 SD/day | 11 [20] | 7 [64] | 4 [36] | |
Ex-heavy (3 SD/day) | 7 [13] | 6 [86] | 1 [14] | |
Primary tumour site | 0.086 | |||
Tonsil | 19 [35] | 16 [84] | 3 [16] | |
Base of tongue | 14 [26] | 13 [93] | 1 [7] | |
Soft palate | 4 [7] | 4 [100] | 0 [0] | |
PPW | 4 [7] | 3 [75] | 1 [25] | |
Larynx | 6 [11] | 3 [50] | 3 [50] | |
Hypopharynx | 7 [13] | 3 [43] | 4 [57] | |
TNM stage | 0.861 | |||
Stage 2 | 6 [11] | 5 [83] | 1 [17] | |
Stage 3 | 13 [24] | 9 [69] | 4 [31] | |
Stage 4a | 31 [57] | 25 [81] | 6 [19] | |
Stage 4b | 4 [7] | 3 [75] | 1 [25] | |
T stage | 0.558 | |||
T1 | 4 [7] | 3 [75] | 1 [25] | |
T2 | 22 [41] | 19 [86] | 3 [14] | |
T3 | 23 [43] | 17 [74] | 6 [26] | |
T4 | 5 [9] | 3 [60] | 2 [40] | |
Grade | 0.037 | |||
Well differentiated | 2 [4] | 1 [50] | 1 [50] | |
Mod differentiated | 9 [17] | 4 [44] | 5 [56] | |
Poor differentiated | 18 [33] | 15 [83] | 3 [17] | |
Unknown | 25 [46] | 22 [88] | 3 [12] | |
P16 status | 0.693 | |||
Negative | 6 [11] | 5 [83] | 1 [17] | |
Positive | 20 [37] | 17 [85] | 3 [15] | |
Unknown/NA | 28 [52] | 20 [71] | 8 [29] |
Continuous variables are presented in mean ± standard deviation and compared using Mann-Whitney U test. Categorical data are presented as numbers [%] and compared using Chi-square (χ2) test. LRR, locoregional recurrence; PPW, posterior pharyngeal wall; N/A, not applicable.
A significant difference in week 0 and relative change in SUVmean, MTV and TLG values were noted between different ROI delineation methods (Wilcoxon signed rank test, P<0.05). The largest week 0 MTVs and TLGs were obtained using MTV2.5 method (mean: 17.9 mL, 106.5 g), compared to PET Edge (mean: 10.5 mL, 79.9 g) and MTV40% (mean: 7.8 mL, 64.9 g). The largest change in ∆MTV and ∆TLG at week 3 was noted using the PET Edge method, −47.9% and −58.0% respectively. Change in CT-GTV volume at week 3 was only −25.3% in comparison (Table 2). Box plots of PET parameters at week 0 and their relative change for the three ROI delineation methods are shown in Figure 3.
Table 2
Delineation method | Baseline | Change at week 3 | |||||||
---|---|---|---|---|---|---|---|---|---|
SUVmax | SUVmean | MTV | TLG | ∆SUVmax | ∆SUVmean | ∆MTV | ∆TLG | ||
PET Edge | 13.06±4.80 | 7.13±2.19 | 10.53±11.64 | 79.86±92.20 | −31.8%±25% | −20.6%±27% | −47.9%±35% | −58.0%±34% | |
MTV2.5 | 13.06±4.80 | 5.30±1.33 | 17.86±14.22 | 106.49±109.60 | −31.8%±25% | −16.9%±20% | −37.5%±50% | −44.4%±55% | |
MTV40% | 13.06±4.80 | 7.56±2.96 | 7.83±6.88 | 64.93±70.86 | −31.6%±26% | −30.4%±25% | −2.9%±52% | −29.3%±41% | |
CT GTV volume | – | – | 14.57±12.55 | – | – | – | −25.3%±20% | – |
Data are presented in mean ± standard deviation; ∆ parameter = (week 3 − baseline)/baseline × 100%. MTV, metabolic tumour volume; TLG, total lesion glycolysis; GTV, gross tumour volume; FDG-PET, 18F-fluoro-2-deoxy-D-glucose positron emission tomography.
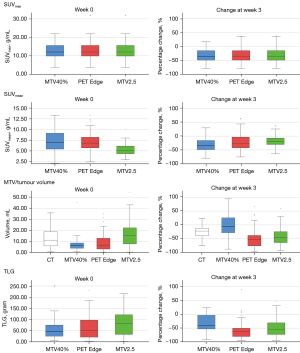
For week 0 FDG-PET parameters, good or excellent correlation was found between delineation methods for SUVmax, SUVmean, MTV and TLG (r>0.81, range: 0.87–1.00, P<0.05; Figure S1). When measuring agreement for FDG-PET parameter values at week 0, a greater agreement was seen between PET Edge and MTV40% methods (Figure S2). On average, the difference in week 0 SUVmax, SUVmean, MTV and TLG values measured using PET Edge and MTV40% methods were 0.0%, 2.9%, 2.2% and 1.2% respectively.
For relative change (∆) in FDG-PET parameters at week 3, good correlation was found for ∆SUVmean (r>0.89, range: 0.89–0.94, P<0.05; Figure S1). Only moderate to poor correlation was noted for ∆MTV and ∆TLG between different ROI delineation methods (r<0.68, range: 0.17–0.68, P<0.05). When measuring agreement for relative change in FDG-PET parameter values at week 3, a greater agreement was seen between PET Edge and MTV2.5 methods (Figure S2). On average, the difference in ∆SUVmax, ∆SUVmean, ∆MTV, ∆TLG values measured using PET Edge and MTV2.5 methods were 0.0%, 3.6%, 10.3% and 13.6% respectively.
Change in CT-GTV did not correlate to locoregional recurrence. No baseline FDG-PET parameters correlated to locoregional recurrence. Only relative change (∆) in volumetric FDG-PET parameters, MTV and closely related TLG measured using PET Edge and MTV2.5 methods correlated to locoregional recurrence (Table 3). Relative change (∆) in FDG-PET parameters had a stronger correlation to locoregional recurrence than absolute values.
Table 3
Timepoint | Parameter | Nil LRR (mean ± SD) | LRR (mean ± SD) | P value* | ROC (AUC) |
---|---|---|---|---|---|
PET Edge | |||||
Week 0 | SUVmax | 12.6±4.0 | 14.7±6.8 | 0.261 | |
SUVmean | 7.0±2.1 | 7.5±2.5 | 0.270 | ||
MTV | 10.0±11.9 | 12.4±10.9 | 0.466 | ||
TLG | 71.3±87.2 | 110.0±130.0 | 0.492 | ||
Change at week 3 (%) | ∆SUVmax | −34.5%±23.2% | −21.7%±31.5% | 0.155 | |
∆SUVmean | −22.1%±23.1% | −14.8%±36.8% | 0.553 | ||
∆MTV | −55.4%±29.7% | −19.8%±41.8% | 0.008† | 0.761 | |
∆TLG | −64.9%±29.7% | −32.1%±39.8% | 0.010† | 0.756 | |
MTV2.5 | |||||
Week 0 | SUVmax | 12.6±4.0 | 14.7±6.8 | 0.261 | |
SUVmean | 5.2±1.1 | 5.7±1.8 | 0.298 | ||
MTV | 17.2±13.8 | 20.3±16.1 | 0.693 | ||
TLG | 97.1±95.1 | 139.2±150.6 | 0.533 | ||
Change at week 3 (%) | ∆SUVmax | −34.5%±23.2% | −21.7%±31.5% | 0.155 | |
∆SUVmean | −17.7%±18.6% | −13.9%±25.0% | 0.388 | ||
∆MTV | −48.0%±24.6% | 1.8%±89.7% | 0.038† | 0.705 | |
∆TLG | −55.8%±26.1% | −1.6%±102.2% | 0.043† | 0.701 | |
MTV40% | |||||
Week 0 | SUVmax | 12.6±4.0 | 14.7±6.8 | 0.261 | |
SUVmean | 7.3±2.6 | 8.5±3.9 | 0.289 | ||
MTV | 7.8±7.3 | 8.0±5.6 | 0.787 | ||
TLG | 60.0±66.0 | 82.2±86.7 | 0.533 | ||
Change at week 3 (%) | ∆SUVmax | −34.5%±23.2% | −21.7%±31.5% | 0.161 | |
∆SUVmean | −32.8%±22.2% | −21.5%±33.9% | 0.222 | ||
∆MTV | 4.0%±57.0% | −1.1%±34.4% | 0.797 | ||
∆TLG | −32.7%±41.2% | −19.2%±41.3% | 0.175 | ||
CT | |||||
Week 0 | GTV | 13.9±11.8 | 18.4±15.8 | 0.519 | |
Change (%) | ∆GTV | −28.1%±18.2% | −14.9%±25.3% | 0.071 |
*, parameters compared to locoregional recurrence status using Mann-Whitney U test, ∆ parameter = (week 3 − week 0)/week 0 × 100%. †, significant (P<0.05), Mann-Whitney U test. Units: SUVmax (g/mL), SUVmean (g/mL), MTV (mL), TLG (g), GTV (mL). ROC, receiver operator characteristics; AUC, area under the curve; MTV, metabolic tumour volume; TLG, total lesion glycolysis; GTV, gross tumour volume; LRR, nil locoregional recurrence.
Relative change in MTV at week 3 (∆MTV) measured using PET Edge was the best predictor of locoregional recurrence (AUC =0.761, 95% CI: 0.573–0.948, P=0.001; Figure 4). In patients with locoregional recurrence, a significant difference in ∆MTV measured using PET Edge was noted compared to those without locoregional recurrence (−55.4% vs. −19.8%, P=0.008). Optimal cut-off of ∆MTV calculated using PET Edge for predicting local recurrence was <50.4% drop in MTV; resulting in sensitivity of 82% (9/11; 95% CI: 74–89%), specificity of 66% (27/41; 95% CI: 62–69%) and accuracy of 69% (36/52; 95% CI: 66–72%). A statistically significant difference was found on Kaplan-Meier survival analysis based on primary tumour ∆MTV optimal cut-off value. The corresponding 2-year locoregional recurrence rate was 7% vs. 35%, P=0.001 (log rank). Comparison of tumour volumes measured using three FDG-PET ROI delineation methods and CT (CT-GTV) stratified by locoregional recurrence status are shown in Figure 5.
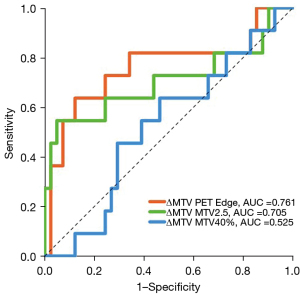
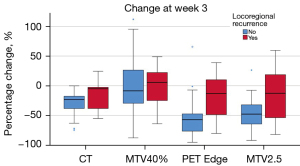
Discussion
Our study is the first to explore the impact of ROI delineation method in mid-treatment FDG-PET response prediction in HNSCC. We identified PET Edge, a gradient based method performed best for mid-treatment response assessment in our patient population. Our data suggests that change in MTV measured at week 3 is a better predictor for treatment response compared to other PET derived parameters or CT based tumour volume. We demonstrated that impact of different ROI methods on extracted features were magnified when utilising mid-treatment imaging. Our results aid in the design and analysis of future FDG-PET guided response-adaptive radiotherapy clinical trials in HNSCC.
Patients in our study had their primary tumour delineated at baseline and week 3 mid-treatment using three commonly utilised semi-automated ROI delineation methods which have previously shown to be reproducible and with predictive capability in HNSCC (6,22,23). Semi-automated methods have the advantage of improving interobserver variability and reduce time required for delineation. However, each method has individual limitations depending on tumour size, uptake, tumour heterogeneity and background FDG uptake dependent on tumour site.
Our results are consistent with previous studies utilising baseline FDG-PET that have shown significant differences in metabolic PET parameters extracted from different ROI delineation methods (10,14). We found that MTV and TLG values were largest using MTV2.5 method and smallest using MTV40% method. However, only limited studies have measured the level of agreement of metabolic PET parameters values between the different ROI methods on baseline imaging in HNSCC (12,14). We found that on baseline imaging good agreement was found only between PET Edge and MTV40% method, with a mean % bias of <3%. These results are consistent with study by Guezennec et al., who analysed 43 HNSCC tumour MTV on baseline FDG-PET using three ROI delineation methods including PET Edge and MTV40%. They also found that MTV volumes were larger using PET Edge method with reasonable agreement between PET Edge and MTV40% derived MTV volumes (14). Head and mucosal sites have a background level of low grade FDG uptake that can overestimate the true primary tumour size when using absolute SUV measure such as MTV2.5, hence resulting in poor agreement with other methods (13).
Tumour delineation on mid-treatment FDG-PET imaging is further complicated by inflammation and reducing tumour uptake as a result of radiotherapy response. There are currently no studies that have measured the impact of different ROI delineation method on extracted parameters from mid-treatment imaging in HNSCC. We found the impact of ROI delineation method were amplified when measuring change in metabolic PET parameters as evidenced by worse agreement in relative change in values compared to baseline values. Even though the tumour volume calculated by MTV2.5 were consistently larger then PET Edge on mid-treatment imaging, the relative change from baseline were moderately similar. Hence, only a moderate agreement in change in metabolic PET parameters between PET Edge and MTV2.5 method was found, with a mean % bias of <15%. This is likely explained by a limitation of the MTV40% method on the absolute value of SUVmax from which it is derived. In patients with good treatment response, if week 3 tumour SUVmax approaches background uptake of irradiated mucosa the true tumour volume can be vastly overestimated using the MTV40% method. This is consistent with a small study containing 10 patients by Edet-Sanson et al., which measured the influence of different delineation methods including MTV40%, manual delineation and ‘adaptive threshold’ method on calculated tumour volume (MTV) from baseline and weekly mid-treatment FDG-PET in non-small cell lung cancer (27). Using extensive imaging during treatment, they were able to show that MTV calculated using different delineation methods magnified as treatment progressed. They found that MTV40% delineation method was not useful in delineating tumour beyond week 1 mid-treatment based on visual interpretation of the volume due to background treatment related inflammation (27). The study by Edet-Sanson et al. did not perform a gradient based method for comparison and they did not correlate their findings to treatment outcome for its value in response prediction.
Currently the optimal ROI method for tumour delineation in HNSCC is unknown (17,36). Studies that have utilised mid-treatment FDG-PET changes for prediction of clinical outcomes have used varying ROI delineation methodology resulting in differing predictive parameters (6,22,23,37). We found that change in FDG-PET parameters (∆MTV and ∆TLG) at week 3 during radiotherapy measured using PET Edge and MTV2.5 predicted for clinical response. Based on our data, change in MTV (∆MTV) measured using PET Edge had the strongest correlation to locoregional tumour control. Based on our data, a greater percentage decrease in MTV (∆>50.4%) was highly predictive of tumour control. Our results confirm findings from previous studies that changes in FDG-PET metabolic parameters are better treatment response markers compared to absolute values (22,23). Studies by Min et al. and Pollom et al. have shown that ∆TLG from mid-treatment FDG-PET can predict clinical outcomes (23). The studies utilised differing methodologies to delineate the MTV and hence limits direct comparison. In our study, absolute or change in SUVmax and SUVmean did not correlate to clinical outcome, this is consistent with previous studies (6,23). No FDG-PET parameters measured using MTV40% correlated to clinical outcome. It is likely that gradient based method outperformed other methods in our population because it was more accurate at differentiating tumour margins from changes to surrounding inflamed tissue during radiotherapy, hence has higher correlation to clinical outcomes (38). To date, this is the first study in HNSCC that has attempted to identify the optimal ROI delineation method for mid-treatment response assessment.
When comparing the tumour volume changes during treatment on FDG-PET and CT; we found greater reduction in tumour volume measured using PET Edge method (−48%) and MTV2.5 (−38%), compared to CT-GTV (−25%). Therefore, our results confirm findings from previous studies that show that metabolic changes on FDG-PET precede anatomical changes measured on CT (39,40). We also found that anatomical CT based primary tumour volume changes did not correlate to locoregional recurrence. Study by Kabarriti et al. measured CT based primary and nodal volumes at week 3 during radiotherapy in 96 oropharyngeal cancer patients (3). They found a similar reduction in CT based tumour volume (19%) to our study. However, they found that a greater than 19% reduction in total tumour volume was an independent predictor for locoregional recurrence. The differing results could be due to larger number of included patients in their study and inclusion of nodal tumour volume measurements. A potential limitation of using CT based imaging biomarkers it the low reproducibility due to significant inter-observer variability in manual target delineation in a complex anatomic site of head and neck malignancies. Due to treatment related changes this variability has been shown to further increase when utilising mid-treatment CT images (41). Semi-automated PET based tumour delineation are reproducible and have greater clinically applicability as an imaging biomarker in future response-adaptive clinical trials.
Strengths of our study include the number of patients and reproducible methodology employed for response assessment. Patients in our study were prospectively recruited, underwent standardised imaging protocol and had consensus based ROI delineation. We also correlated CT and FDG-PET based tumour volumes to locoregional clinical outcomes which remains a gold-standard for response assessment. There are a few limitations of our study. Ours was a single institutional study with serial imaging undertaken on the same PET machine. Cross facility standardisation of SUV values is required to translate and validate our results in prospective multicentre trials. The heterogeneities can be minimised through phantom calibrations, but inherent limitations remain taking into account different PET scanners with different sensitivities and blurred edge due to partial volume effects, acquisition and reconstruction protocols. We believe that parameters measuring relative change from baseline using serial PET imaging if done on the same PET scanner provides auto-normalisation of measurements compared to using a single static value. In this respect, the use of relative reduction as treatment response criteria would facilitate more reproducible, readily accessible and interpretable predictive imaging biomarkers to facilitate future multicentre trials for image guided adaptive therapy. Studies also indicate the importance of timing of mid-treatment imaging on extracted imaging parameters and hence extrapolation of our results to other imaging timepoints should be done with caution (11). Another limitation of the gradient based method used in this study is that it is only available within the MIM Maestro® software. Results from gradient based method used in this study should not be generalized to other gradient based methods without additional comparison. We have also not compared our results with less commonly utilised methods for tumour delineation such as ‘background-related’ threshold and manual PET contouring methods due to the significant inter-observer variability in implementation in a complex anatomic site such as head and neck.
Conclusions
Our study highlights the critical importance of ROI delineation method choice in mid-treatment response assessment. Our findings suggest that it is feasible to use gradient based method to assess metabolic PET tumour response during radiotherapy and offers advantage in predicting treatment outcomes compared with threshold-based methods.
Acknowledgments
Funding: None.
Footnote
Reporting Checklist: The authors have completed the STARD reporting checklist. Available at https://qims.amegroups.com/article/view/10.21037/qims-22-798/rc
Conflicts of Interest: All authors have completed the ICMJE uniform disclosure form (available at https://qims.amegroups.com/article/view/10.21037/qims-22-798/coif). MGJ is currently an employee of GenesisCsare and declares institutional research agreements between GenesisCare and Elekta AB, MIM Software Inc., ViewRay Technologies and Brainlab AB. MGJ declares a licencing agreement with Standard Imaging Inc. During part of his involvement in the study, MGJ was an employee of the Sydney South West Local Health District and was supported by a NSW Cancer Institute Fellowship. He is also supported by an Australian Government NHMRC Leadership Fellowship. MGJ and PK declares that they are supported by an Australian Government NHMRC Leadership Fellowship. PC receives funding from South Western Sydney Local Health District and University of New South Wales. The other authors have no conflicts of interest to declare.
Ethical Statement: The authors are accountable for all aspects of the work in ensuring that questions related to the accuracy or integrity of any part of the work are appropriately investigated and resolved. The study was conducted in accordance with the Declaration of Helsinki (as revised in 2013). The study was approved by the South Western Sydney Local Health District research ethics committee and informed consent was taken from all individual participants.
Open Access Statement: This is an Open Access article distributed in accordance with the Creative Commons Attribution-NonCommercial-NoDerivs 4.0 International License (CC BY-NC-ND 4.0), which permits the non-commercial replication and distribution of the article with the strict proviso that no changes or edits are made and the original work is properly cited (including links to both the formal publication through the relevant DOI and the license). See: https://creativecommons.org/licenses/by-nc-nd/4.0/.
References
- Evangelista L, Cervino AR, Chondrogiannis S, Marzola MC, Maffione AM, Colletti PM, Muzzio PC, Rubello D. Comparison between anatomical cross-sectional imaging and 18F-FDG PET/CT in the staging, restaging, treatment response, and long-term surveillance of squamous cell head and neck cancer: a systematic literature overview. Nucl Med Commun 2014;35:123-34. [Crossref] [PubMed]
- Chang JH, Wu CC, Yuan KS, Wu ATH, Wu SY. Locoregionally recurrent head and neck squamous cell carcinoma: incidence, survival, prognostic factors, and treatment outcomes. Oncotarget 2017;8:55600-12. [Crossref] [PubMed]
- Kabarriti R, Brodin NP, Lundgren G, Ohri N, Tomé WA, Kalnicki S, Garg MK. Early Response Assessment on Mid-treatment Computed Tomography Predicts for Locoregional Recurrence in Oropharyngeal Cancer Patients Treated With Definitive Radiation Therapy. Int J Radiat Oncol Biol Phys 2018;102:1036-45. [Crossref] [PubMed]
- Martens RM, Noij DP, Ali M, Koopman T, Marcus JT, Vergeer MR, de Vet H, de Jong MC, Leemans CR, Hoekstra OS, de Bree R, de Graaf P, Boellaard R, Castelijns JA. Functional imaging early during (chemo)radiotherapy for response prediction in head and neck squamous cell carcinoma; a systematic review. Oral Oncol 2019;88:75-83. [Crossref] [PubMed]
- Cook GJR, Azad G, Owczarczyk K, Siddique M, Goh V. Challenges and Promises of PET Radiomics. Int J Radiat Oncol Biol Phys 2018;102:1083-9. [Crossref] [PubMed]
- Min M, Lin P, Lee MT, Shon IH, Lin M, Forstner D, Bray V, Chicco A, Tieu MT, Fowler A. Prognostic role of metabolic parameters of (18)F-FDG PET-CT scan performed during radiation therapy in locally advanced head and neck squamous cell carcinoma. Eur J Nucl Med Mol Imaging 2015;42:1984-94. [Crossref] [PubMed]
- Wong KH, Panek R, Dunlop A, Mcquaid D, Riddell A, Welsh LC, Murray I, Koh DM, Leach MO, Bhide SA, Nutting CM, Oyen WJ, Harrington KJ, Newbold KL. Changes in multimodality functional imaging parameters early during chemoradiation predict treatment response in patients with locally advanced head and neck cancer. Eur J Nucl Med Mol Imaging 2018;45:759-67. [Crossref] [PubMed]
- Pak K, Cheon GJ, Nam HY, Kim SJ, Kang KW, Chung JK, Kim EE, Lee DS. Prognostic value of metabolic tumor volume and total lesion glycolysis in head and neck cancer: a systematic review and meta-analysis. J Nucl Med 2014;55:884-90. [Crossref] [PubMed]
- Lin P, Min M, Lai K, Lee M, Holloway L, Xuan W, Bray V, Fowler A, Lee CS, Yong J. Mid-treatment Fluorodeoxyglucose Positron Emission Tomography in Human Papillomavirus-related Oropharyngeal Squamous Cell Carcinoma Treated with Primary Radiotherapy: Nodal Metabolic Response Rate can Predict Treatment Outcomes. Clin Oncol (R Coll Radiol) 2021;33:e586-98. [Crossref] [PubMed]
- Hatt M, Cheze-le Rest C, van Baardwijk A, Lambin P, Pradier O, Visvikis D. Impact of tumor size and tracer uptake heterogeneity in (18)F-FDG PET and CT non-small cell lung cancer tumor delineation. J Nucl Med 2011;52:1690-7. [Crossref] [PubMed]
- Hatt M, Visvikis D, Albarghach NM, Tixier F, Pradier O, Cheze-le Rest C. Prognostic value of 18F-FDG PET image-based parameters in oesophageal cancer and impact of tumour delineation methodology. Eur J Nucl Med Mol Imaging 2011;38:1191-202. [Crossref] [PubMed]
- Moon SH, Choi JY, Lee HJ, Son YI, Baek CH, Ahn YC, Park K, Lee KH, Kim BT. Prognostic value of 18F-FDG PET/CT in patients with squamous cell carcinoma of the tonsil: comparisons of volume-based metabolic parameters. Head Neck 2013;35:15-22. [Crossref] [PubMed]
- Schinagl DA, Span PN, Oyen WJ, Kaanders JH. Can FDG PET predict radiation treatment outcome in head and neck cancer? Results of a prospective study. Eur J Nucl Med Mol Imaging 2011;38:1449-58. [Crossref] [PubMed]
- Guezennec C, Bourhis D, Orlhac F, Robin P, Corre JB, Delcroix O, Gobel Y, Schick U, Salaün PY, Abgral R. Inter-observer and segmentation method variability of textural analysis in pre-therapeutic FDG PET/CT in head and neck cancer. PLoS One 2019;14:e0214299. [Crossref] [PubMed]
- Wang XY, Zhao YF, Liu Y, Yang YK, Zhu Z, Wu N. Comparison of different automated lesion delineation methods for metabolic tumor volume of 18F-FDG PET/CT in patients with stage I lung adenocarcinoma. Medicine (Baltimore) 2017;96:e9365. [Crossref] [PubMed]
- Garibaldi C, Ronchi S, Cremonesi M, Gilardi L, Travaini L, Ferrari M, Alterio D, Kaanders JHAM, Ciardo D, Orecchia R, Jereczek-Fossa BA, Grana CM. Interim (18)F-FDG PET/CT During Chemoradiation Therapy in the Management of Head and Neck Cancer Patients: A Systematic Review. Int J Radiat Oncol Biol Phys 2017;98:555-73. [Crossref] [PubMed]
- Hentschel M, Appold S, Schreiber A, Abolmaali N, Abramyuk A, Dörr W, Kotzerke J, Baumann M, Zöphel K. Early FDG PET at 10 or 20 Gy under chemoradiotherapy is prognostic for locoregional control and overall survival in patients with head and neck cancer. Eur J Nucl Med Mol Imaging 2011;38:1203-11. [Crossref] [PubMed]
- Burger IA, Casanova R, Steiger S, Husmann L, Stolzmann P, Huellner MW, Curioni A, Hillinger S, Schmidtlein CR, Soltermann A. 18F-FDG PET/CT of Non-Small Cell Lung Carcinoma Under Neoadjuvant Chemotherapy: Background-Based Adaptive-Volume Metrics Outperform TLG and MTV in Predicting Histopathologic Response. J Nucl Med 2016;57:849-54. [Crossref] [PubMed]
- Rumley CN, Lee MT, Holloway L, Rai R, Min M, Forstner D, Fowler A, Liney G. Multiparametric magnetic resonance imaging in mucosal primary head and neck cancer: a prospective imaging biomarker study. BMC Cancer 2017;17:475. [Crossref] [PubMed]
- Min M, Lee MT, Lin P, Holloway L, Wijesekera Dj, Gooneratne D, Rai R, Xuan W, Fowler A, Forstner D, Liney G. Assessment of serial multi-parametric functional MRI (diffusion-weighted imaging and R2*) with (18)F-FDG-PET in patients with head and neck cancer treated with radiation therapy. Br J Radiol 2016;89:20150530. [Crossref] [PubMed]
- Grégoire V, Ang K, Budach W, Grau C, Hamoir M, Langendijk JA, Lee A, Le QT, Maingon P, Nutting C, O'Sullivan B, Porceddu SV, Lengele B. Delineation of the neck node levels for head and neck tumors: a 2013 update. DAHANCA, EORTC, HKNPCSG, NCIC CTG, NCRI, RTOG, TROG consensus guidelines. Radiother Oncol 2014;110:172-81. [Crossref] [PubMed]
- Lin P, Min M, Lee M, Holloway L, Forstner D, Bray V, Fowler A. Nodal parameters of FDG PET/CT performed during radiotherapy for locally advanced mucosal primary head and neck squamous cell carcinoma can predict treatment outcomes: SUVmean and response rate are useful imaging biomarkers. Eur J Nucl Med Mol Imaging 2017;44:801-11. [Crossref] [PubMed]
- Pollom EL, Song J, Durkee BY, Aggarwal S, Bui T, von Eyben R, Li R, Brizel DM, Loo BW, Le QT, Hara WY. Prognostic value of midtreatment FDG-PET in oropharyngeal cancer. Head Neck 2016;38:1472-8. [Crossref] [PubMed]
- Hoshikawa H, Mori T, Kishino T, Yamamoto Y, Inamoto R, Akiyama K, Mori N, Nishiyama Y. Changes in (18)F-fluorothymidine and (18)F-fluorodeoxyglucose positron emission tomography imaging in patients with head and neck cancer treated with chemoradiotherapy. Ann Nucl Med 2013;27:363-70. [Crossref] [PubMed]
- Denaro N, Merlano MC, Russi EG. Follow-up in Head and Neck Cancer: Do More Does It Mean Do Better? A Systematic Review and Our Proposal Based on Our Experience. Clin Exp Otorhinolaryngol 2016;9:287-97. [Crossref] [PubMed]
- Lu L, Lv W, Jiang J, Ma J, Feng Q, Rahmim A, et al. Robustness of Radiomic Features in [(11)C]Choline and [(18)F]FDG PET/CT Imaging of Nasopharyngeal Carcinoma: Impact of Segmentation and Discretization. Mol Imaging Biol 2016;18:935-45. [Crossref] [PubMed]
- Edet-Sanson A, Dubray B, Doyeux K, Back A, Hapdey S, Modzelewski R, Bohn P, Gardin I, Vera P. Serial assessment of FDG-PET FDG uptake and functional volume during radiotherapy (RT) in patients with non-small cell lung cancer (NSCLC). Radiother Oncol 2012;102:251-7. [Crossref] [PubMed]
- Grootjans W, Usmanij EA, Oyen WJ, van der Heijden EH, Visser EP, Visvikis D, Hatt M, Bussink J, de Geus-Oei LF. Performance of automatic image segmentation algorithms for calculating total lesion glycolysis for early response monitoring in non-small cell lung cancer patients during concomitant chemoradiotherapy. Radiother Oncol 2016;119:473-9. [Crossref] [PubMed]
- Zwezerijnen GJC, Eertink JJ, Burggraaff CN, Wiegers SE, Shaban EAIN, Pieplenbosch S, Oprea-Lager DE, Lugtenburg PJ, Hoekstra OS, de Vet HCW, Zijlstra JM, Boellaard R. Interobserver Agreement on Automated Metabolic Tumor Volume Measurements of Deauville Score 4 and 5 Lesions at Interim (18)F-FDG PET in Diffuse Large B-Cell Lymphoma. J Nucl Med 2021;62:1531-6. [Crossref] [PubMed]
- Obara P, Liu H, Wroblewski K, Zhang CP, Hou P, Jiang Y, Chen P, Pu Y. Quantification of metabolic tumor activity and burden in patients with non-small-cell lung cancer: Is manual adjustment of semiautomatic gradient-based measurements necessary? Nucl Med Commun 2015;36:782-9. [Crossref] [PubMed]
- Sridhar P, Mercier G, Tan J, Truong MT, Daly B, Subramaniam RM. FDG PET metabolic tumor volume segmentation and pathologic volume of primary human solid tumors. AJR Am J Roentgenol 2014;202:1114-9. [Crossref] [PubMed]
- Fedrigo R, Segars WP, Martineau P, Gowdy C, Bloise I, Uribe CF, Rahmim A. Development of scalable lymphatic system in the 4D XCAT phantom: Application to quantitative evaluation of lymphoma PET segmentations. Med Phys 2022;49:6871-84. [Crossref] [PubMed]
- Werner-Wasik M, Nelson AD, Choi W, Arai Y, Faulhaber PF, Kang P, Almeida FD, Xiao Y, Ohri N, Brockway KD, Piper JW, Nelson AS. What is the best way to contour lung tumors on PET scans? Multiobserver validation of a gradient-based method using a NSCLC digital PET phantom. Int J Radiat Oncol Biol Phys 2012;82:1164-71. [Crossref] [PubMed]
- van Griethuysen JJM, Fedorov A, Parmar C, Hosny A, Aucoin N, Narayan V, Beets-Tan RGH, Fillion-Robin JC, Pieper S, Aerts HJWL. Computational Radiomics System to Decode the Radiographic Phenotype. Cancer Res 2017;77:e104-7. [Crossref] [PubMed]
- Fluss R, Faraggi D, Reiser B. Estimation of the Youden Index and its associated cutoff point. Biom J 2005;47:458-72. [Crossref] [PubMed]
- Hentschel M, Appold S, Schreiber A, Abramyuk A, Abolmaali N, Kotzerke J, Baumann M, Zöphel K. Serial FDG-PET on patients with head and neck cancer: implications for radiation therapy. Int J Radiat Biol 2009;85:796-804. [Crossref] [PubMed]
- Lin Q, Yang R, Sun L, Chen S, Wu H. Biological response of nasopharyngeal carcinoma to radiation therapy: a pilot study using serial 18F-FDG PET/CT scans. Cancer Invest 2012;30:528-36. [Crossref] [PubMed]
- Stevenson MG, Been LB, Hoekstra HJ, Suurmeijer AJH, Boellaard R, Brouwers AH. Volume of interest delineation techniques for (18)F-FDG PET-CT scans during neoadjuvant extremity soft tissue sarcoma treatment in adults: a feasibility study. EJNMMI Res 2018;8:42. [Crossref] [PubMed]
- Herrmann K, Bundschuh RA, Rosenberg R, Schmidt S, Praus C, Souvatzoglou M, Becker K, Schuster T, Essler M, Wieder HA, Friess H, Ziegler SI, Schwaiger M, Krause BJ. Comparison of different SUV-based methods for response prediction to neoadjuvant radiochemotherapy in locally advanced rectal cancer by FDG-PET and MRI. Mol Imaging Biol 2011;13:1011-9. [Crossref] [PubMed]
- Geets X, Tomsej M, Lee JA, Duprez T, Coche E, Cosnard G, Lonneux M, Grégoire V. Adaptive biological image-guided IMRT with anatomic and functional imaging in pharyngo-laryngeal tumors: impact on target volume delineation and dose distribution using helical tomotherapy. Radiother Oncol 2007;85:105-15. [Crossref] [PubMed]
- Apolle R, Appold S, Bijl HP, Blanchard P, Bussink J, Faivre-Finn C, Khalifa J, Laprie A, Lievens Y, Madani I, Ruffier A, de Ruysscher D, van Elmpt W, Troost EGC. Inter-observer variability in target delineation increases during adaptive treatment of head-and-neck and lung cancer. Acta Oncol 2019;58:1378-85. [Crossref] [PubMed]