The clinical significance of computed tomography texture features of renal cell carcinoma in predicting pathological T1–3 staging
Introduction
Worldwide, renal cell carcinoma (RCC) is the ninth most frequently diagnosed cancer in men, accounting for 2.7% of all tumor diagnoses (1). The T staging of RCC is an important auxiliary tool to guide clinical treatment, predict tumor prognosis, and communicate between different medical units (2). The main factors that impact the T staging of RCC include the tumor size; invasion of the perinephric fat, renal sinus, and Gerota fascia; development of tumor thrombus of the renal vein and/or inferior vena cava (IVC); and invasion of the ipsilateral adrenal gland (3). Pathological assessment is the gold standard for determining staging. However, the fact that the pathological diagnosis of the tumor relies on postoperative specimens impedes clinicians from making precise preoperative judgments on the T staging of RCC (4).
Computed tomography (CT) plays an important role over the course of RCC treatment, from diagnosing and staging the disease to assessing the response to treatment (5). However, CT as a clinical staging tool has limitations; for example, conventional CT scanning has low definition and resolution for soft tissue imaging. These limitations result in some RCCs being wrongly staged compared to the final pathological review, and there is often confusion between stages T3 and T1–2 (6,7). This discrepancy of radiological staging is not conducive to the precise treatment of RCC.
Recently, the potential of machine learning methods to improve the working efficiency of radiologists in complex medical image analysis has been demonstrated (8-10). In the field of RCC, the application of machine learning has focused on the identification and segmentation of medical images. It has also been dedicated to the purposeful classification of RCC by using its texture features, such as the differential diagnosis of benign and malignant renal tumors, the differential diagnosis of the histological subtypes of RCC, and the prediction of the Fuhrman grade of RCC (11-14). However, few studies have reported the value of CT texture features based on machine learning for the T staging of RCC.
With the increasing use of multidisciplinary treatment of RCC, precise T staging will continue to be a critical feature in treatment decision-making (4). Therefore, it is important to accurately predict the pathological T staging with CT, especially the T1–3 staging of RCC. In this study, we aimed to develop a framework that automatically segmented the CT images and extracted the CT texture features of RCC to predict the pathological T1–3 staging. Internal and external validation were subsequently conducted. The internal decision-making process of the prediction model may serve as reference for the diagnostic process of clinical imaging. We also analyzed the contribution of features to the particular decision of the model using a visualization strategy. We present the following article in accordance with the STARD reporting checklist (available at https://qims.amegroups.com/article/view/10.21037/qims-22-1043/rc) (15).
Methods
Patient cohorts and data preparation
We performed a comprehensive retrospective review of the medical records of patients with pathologically proven RCC treated at Sun Yat-sen University Cancer Center between May 2018 and March 2021. Patients were included if they were newly diagnosed, untreated, and had undergone unenhanced and enhanced CT imaging 2 weeks before the operation (Figure 1). Finally, 100 consecutive patients (64 men and 36 women; median age 55 years; range, 16–83 years) were enrolled, including those with clear cell carcinoma (n=78), papillary RCC (pRCC) (n=11), chromophobe renal carcinoma (n=6), and translocated renal carcinoma in the MIT family (n=5). The patients were classified according to the eighth tumor–node–metastasis (TNM) staging system as follows: T1 (n=37), T2 (n=29), and T3 (n=34). Additionally, we collected data from The Third Affiliated Hospital of Shenzhen University for external validation, including data from 29 consecutive patients with pathologically proven RCC who were treated in this hospital between October 2018 and May 2021 (15 men and 14 women; median age 58 years; range, 37–83 years; T1, n=16; T2, n=7; T3, n=6; Figure 2). See Table 1 for information about these patients.
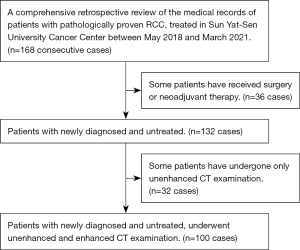
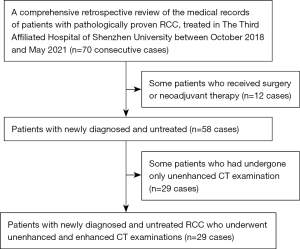
Table 1
Items | Training (n=70) | Internal testing (n=30) | External testing (n=29) | P value |
---|---|---|---|---|
T1, n [%] | 24 [34] | 13 [43] | 16 [55] | 0.858 |
T2, n [%] | 21 [30] | 8 [27] | 7 [24] | 0.383 |
T3, n [%] | 25 [36] | 9 [30] | 6 [21] | 0.598 |
RCC, renal cell carcinoma.
The study was conducted in accordance with the Declaration of Helsinki (as revised in 2013). This study was approved by the Institutional Review Boards at Sun Yat-sen University Cancer Center and the Third Affiliated Hospital of Shenzhen University, and informed consent for this retrospective analysis was waived. All data have been deposited at Sun Yat-sen University Cancer Center (https://www.researchdata.org.cn/; Research Data Deposit; No. RDDA2021358130).
CT scanning protocol
The 100 patients from Sun Yat-sen University Cancer Center underwent CT scanning on a dual-source spiral CT system (SOMATOM Force, Siemens Medical System, Forchheim, Germany; 120 kV; 200 mA; section thickness 5 and 1 mm). Data acquisition was performed in 3 phases: the precontrast phase (PCP), the corticomedullary phase (CMP; 30-second delay after contrast injection), and the nephrographic phase (NP; 90-second delay after contrast injection). Multiplanar reconstruction was performed for axial, coronal, and sagittal planes. After the unenhanced scan, an intravenous bolus injection of nonionic contrast media (350 mg/mL; 1.5 mL/kg) was administered at a rate of 4 mL/s through the median cubital vein.
CT scanning was also performed on the 29 patients at The Third Affiliated Hospital of Shenzhen University (see Appendix 1 for details).
Delineation of the gross target area
The CT images were manually segmented as the kidney and tumor (the renal vein was annotated in the NP) with ITK-SNAP software v.3.8.0 (16). This manual process was conducted by 2 radiologists with more than 10 years of experience in imaging diagnosis of abdominal tumors who accurately delineated the kidney and tumor boundaries. The segmentation results were then checked and amended by a specialist and observers repeatedly to ensure the accuracy of the delineation and prepare for future training.
Image segmentation and feature extraction
A 3-dimensional (3D) UNet-based structure was trained to automatically segment the CT images. For each enhanced CT image of the CMP, only the kidney and tumor were segmented on the image. For each enhanced CT image of the NP, the kidney, renal tumor, renal vein, and IVC below the diaphragm were segmented (Figure 3A,3B). We drew lesions with a well-known segmentation framework, nnU-Net (17), which was adapted to a given data set without user intervention. Hyperparameters, including the patch size, batch size, and some pooling operations, were chosen based on the properties of the data set. We combined 5 data sets and used 5-fold cross-validation to evaluate the model’s performance. The patients were randomly split into a training set (70%) and an internal testing set (30%).
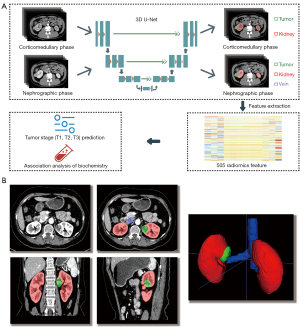
The segmentation results were used to extract the texture features with PyRadiomics v.3.0.1 (18), which is an open-source Python package for the extraction of texture features from medical images. The radiomic features roughly consisted of 3 classes: texture features, morphological features, and statistical features. The CMP images were segmented into the kidney and tumor regions, and the NP images were segmented into the kidney, tumor, and vein regions. Each segmented region generated 101 texture features. Overall, 505 texture features were extracted from each patient’s enhanced CT (the CMP and the NP) images.
The radiological T staging of RCC determined by expert radiologists
To compare the performance of the predictive model with that of the radiological T staging determined by expert radiologists, the CT images of 100 patients from Sun Yat-sen University Cancer Center were reviewed independently by 3 specialist abdominal radiologists. Radiologists 1, 2, and 3 had 16, 12, and 8 years of experience in renal imaging, respectively. The radiologists had no knowledge of clinical and pathological information. The criteria in RCC T staging through CT scanning were as follows: in stage T1, the tumor diameter was ≤7 cm, the tumor was confined to the renal capsule, and there was no extracellular proliferation; in stage T2, the tumor diameter was >7 cm, and there was no renal extracorporeal expansion; in stage T3, the tumor displayed a blurred boundary with renal calyces, renal sinuses, and perirenal fat, as well as the occurrence of renal vein/IVC tumor thrombus.
Feature classification and statistical analysis
We implemented a multiclassification task to predict the pathological T stage of RCC (T1, T2, T3) by using extreme gradient boosting (XGBoost) v.1.3.3 (19). The texture features were involved in the evaluation. Performance metrics, including the mean area under the curve (AUC) of the receiver operating characteristic (ROC) curve of multiple classes for each class, were calculated using micro- and macro-averaging (20). This machine learning experiment was conducted in Python (v.3.8.0). The sensitivity and specificity for each class of prediction model were calculated.
Furthermore, Shapley additive explanations (SHAP) (21) was used in our study. See the supplementary material for details.
Results
Prediction of pathological T1–3 staging
The extracted texture features were directly used to predict the pathological T staging of RCC (T1, T2, and T3). The combined AUCs of the ROC curves for all classes were 0.90 (95% CI: 0.84–1.00) using micro-averaging and 0.91 (95% CI: 0.86–1.00) using macro-averaging for the pathological tumor stage in the internal testing set (Figure 4A). In the external testing set, the micro- and macro-AUCs were 0.72 (95% CI: 0.66–0.84) and 0.78 (95% CI: 0.69–0.88), respectively (Figure 4B). Specifically, the AUC values of the ROC curves were 0.88 (95% CI: 0.74–1.00), 0.98 (95% CI: 0.95–1.00), and 0.88 (95% CI: 0.72–1.00) for stages T1, T2, and T3, respectively (Figure 4C-4E). The sensitivities for the T1, T2, and T3 classes of the prediction model were 77.8% (95% CI: 0.68–0.83), 87.5% (95% CI: 0.76–0.92), and 66.7% (95% CI: 0.58–0.75), respectively. The specificities for the T1, T2, and T3 classes of the prediction model were 93.7% (95% CI: 0.81–0.95), 88.0% (95% CI: 0.78–0.92), and 93.7% (95% CI: 0.81–0.95), respectively.
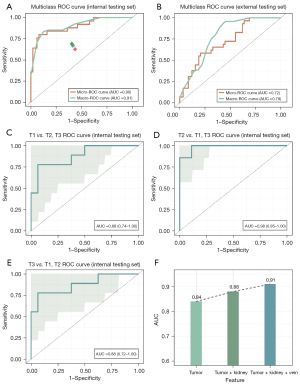
Explanation of the prediction model
The features were based on the CMP and the NP images of the enhanced CT. We compared the predictive results of the extracted features with different regions of interest (ROIs). The AUC values of the ROC curves were 0.84, 0.88, and 0.91 for tumor features, tumor-kidney features, and tumor-kidney-vein features, respectively (Figure 4F).
The SHAP value of each feature was calculated for each sample to evaluate the contribution of the extracted texture features to the model prediction (Figure 5). See Appendix 1 for details.
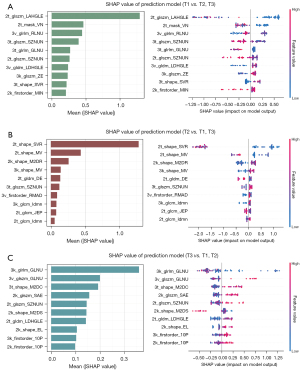
The performance of the predictive model compared to that of the radiological T staging determined by expert radiologists
For the radiological T staging determined by expert radiologists, the AUCs of the ROC curves for the T1, T2, and T3 classes were 0.69, 0.76, and 0.79, respectively, using micro-averaging, and 0.66, 0.71, and 0.77, respectively, using macro-averaging. All of these values were significantly lower than the AUC values of our prediction model. There was no statistically significant difference in AUC between radiologists (Welch two-tailed 2-sample t-test: t’=1.8; P=0.2).
Discussion
According to the National Comprehensive Cancer Network (NCCN) Guidelines published in 2021, patients with RCC with stages T1–2 of the disease should undergo simple partial resection, while patients with stages T3–4 might need radical resection plus neoadjuvant or adjuvant treatment (22). Teishima et al. (23) suggested that some cases with clinical T1 (cT1) RCC can be upstaged to pathological T3 (pT3), which is a risk factor for postoperative recurrence after a simple partial nephrectomy. Therefore, accurate preoperative T staging of RCC, especially T3, is crucial.
CT examination is of great value for diagnosing and staging RCC (5). It is not difficult to confirm the diagnosis of stage T4 RCC with Gerota fascia invasion or ipsilateral adrenal gland invasion using a routine CT examination. However, the influencing factors of T3-staged RCC, such as the invasion of renal calyces, renal sinuses, and perirenal fat, as well as the occurrence of renal vein tumor thrombus in some cases, are difficult to identify with conventional CT imaging, which may lead to a higher or lower radiographic staging (7,24). Our study revealed that, when expert radiologists determined the radiological T staging, the AUCs of the ROC curves for classes I, II, and III were 0.69, 0.76, and 0.79, respectively, using micro-averaging, and 0.66, 0.71, and 0.77, respectively, using macro averaging for pathological tumor stage. This result was still not satisfactory. Sokhi et al. (6) proposed that centrally situated renal tumors with irregular tumor edges that were inseparable from sinus structures or the perirenal fascia as well as CT features of tumor necrosis should alert the radiologists to the possibility of RCC that is stage T3a. These characteristics deserve more attention in the daily work of radiologists.
The texture features of CT images based on machine learning have shown advantages in differentially diagnosing renal tumors and predicting the pathological grade of renal cancer (11-14,25). The studies of Cui et al. (26). and Yu et al. (27) showed that CT texture analysis based on machine learning might be a credible quantitative strategy to differentiate benign renal tumors, such as angiomyolipoma (AML) and oncocytoma, from malignant renal tumors, such as clear cell RCC (ccRCC) and pRCC (26,27). Ding et al. (28) found that a CT-based radiomics model could predict a high grade of ccRCC. However, the value of texture features of CT images based on machine learning for predicting the pathological T staging of RCC remains uncertain.
In this study, we developed a framework to automatically segment 3D ROIs (kidney, tumor, and renal vein) and predict the pathological T1–3 staging of RCC based on features of those ROIs. Other studies only extracted 2-dimensional (2D) features of the tumor center in unenhanced CT images or only used tumor features to predict tumor stage (29,30). Unlike these other studies, our study extracted features that were not only based on the CMP and the NP images of the enhanced CT but also on 3D features with the accurate outline of the tumor edges. In addition to extracting the complete tumor features, the features of the kidneys and renal veins were also extracted. We found that, when used together, the features of the tumor, kidneys, and renal veins predicted the T staging with higher accuracy than did using the tumor features alone.
Our results had fairly good sensitivity and excellent specificity for each class of the prediction model. In addition, the AUC value of the ROC curve was 0.88 (0.72–1.00) for stage T3 vs. stages T1–2. This finding indicated that this model was helpful for the differential diagnosis of RCC at stage T3 RCC from stages T1–2. With this information, clinicians can identify patients with stage T3 disease for neoadjuvant or adjuvant therapy (22). Our study also showed that the predictive performance of our model was superior to that of the radiological T staging determined by an expert radiologist. Therefore, this prediction model is expected to be an auxiliary tool for the preoperative staging for RCC.
Our study had one main limitation. The enrolled sample size, especially the external testing data set, was relatively small, and the distribution of the number of patients in stages T1–3 was slightly uneven. In the future, we look forward to adding more samples to achieve a more convincing validation effect. However, in addition to extracting complete tumor features based on the CMP and the NP images of the enhanced CT, we also extracted 3D features with the accurate outline of the tumor edges and the features of the kidney and renal vein. This process made the prediction performance of our prediction model more accurate and enabled our prediction model to have wider generalizability.
Conclusions
Our study developed a framework based on texture features for predicting the pathological T1–3 staging of RCC. Identifying patients with stage T3 RCC has great clinical value for individualized therapies for treating RCC. We also explored feature extraction methods to improve the accuracy of the prediction model and described the decision-making process of the model, which was found to be a reasonable and evidence-based method for determining the pathological T staging of RCC.
Acknowledgments
We thank Doctor Yaru Liu from the Department of Radiology, The Third Affiliated Hospital of Shenzhen University (Luohu Hospital Group), and Boya Li from the Department of Medical Imaging, Sun Yat-sen University Cancer Center, State Key Laboratory of Oncology in South China, Collaborative Innovation Center for Cancer Medicine, for their contributions to the medical image processing.
Funding: This work was supported by the National Natural Science Foundation Fund of China (No. 61931024).
Footnote
Reporting Checklist: The authors have completed the STARD reporting checklist. Available at https://qims.amegroups.com/article/view/10.21037/qims-22-1043/rc
Conflicts of Interest: All authors have completed the ICMJE uniform disclosure form (available at https://qims.amegroups.com/article/view/10.21037/qims-22-1043/coif). The authors have no conflicts of interest to declare.
Ethical Statement: The authors are accountable for all aspects of the work in ensuring that questions related to the accuracy or integrity of any part of the work are appropriately investigated and resolved. The study was conducted in accordance with the Declaration of Helsinki (as revised in 2013). This study was approved by the Institutional Review Boards at Sun Yat-sen University Cancer Center and the Third Affiliated Hospital of Shenzhen University, and informed consent for this retrospective analysis was waived.
Open Access Statement: This is an Open Access article distributed in accordance with the Creative Commons Attribution-NonCommercial-NoDerivs 4.0 International License (CC BY-NC-ND 4.0), which permits the non-commercial replication and distribution of the article with the strict proviso that no changes or edits are made and the original work is properly cited (including links to both the formal publication through the relevant DOI and the license). See: https://creativecommons.org/licenses/by-nc-nd/4.0/.
References
- Sung H, Ferlay J, Siegel RL, Laversanne M, Soerjomataram I, Jemal A, Bray F. Global Cancer Statistics 2020: GLOBOCAN Estimates of Incidence and Mortality Worldwide for 36 Cancers in 185 Countries. CA Cancer J Clin 2021;71:209-49. [Crossref] [PubMed]
- Moch H, Gasser T, Amin MB, Torhorst J, Sauter G, Mihatsch MJ. Prognostic utility of the recently recommended histologic classification and revised TNM staging system of renal cell carcinoma: a Swiss experience with 588 tumors. Cancer 2000;89:604-14. [Crossref] [PubMed]
- Warren AY, Harrison D. WHO/ISUP classification, grading and pathological staging of renal cell carcinoma: standards and controversies. World J Urol 2018;36:1913-26. [Crossref] [PubMed]
- Williamson SR, Taneja K, Cheng L. Renal cell carcinoma staging: pitfalls, challenges, and updates. Histopathology 2019;74:18-30. [Crossref] [PubMed]
- Rossi SH, Prezzi D, Kelly-Morland C, Goh V. Imaging for the diagnosis and response assessment of renal tumours. World J Urol 2018;36:1927-42. [Crossref] [PubMed]
- Sokhi HK, Mok WY, Patel U. Stage T3a renal cell carcinoma: staging accuracy of CT for sinus fat, perinephric fat or renal vein invasion. Br J Radiol 2015;88:20140504. [Crossref] [PubMed]
- Russell CM, Lebastchi AH, Chipollini J, Niemann A, Mehra R, Morgan TM, Miller DC, Palapattu GS, Hafez KS, Sexton WJ, Spiess PE, Weizer AZ. Multi-institutional Survival Analysis of Incidental Pathologic T3a Upstaging in Clinical T1 Renal Cell Carcinoma Following Partial Nephrectomy. Urology 2018;117:95-100. [Crossref] [PubMed]
- Ardila D, Kiraly AP, Bharadwaj S, Choi B, Reicher JJ, Peng L, Tse D, Etemadi M, Ye W, Corrado G, Naidich DP, Shetty S. End-to-end lung cancer screening with three-dimensional deep learning on low-dose chest computed tomography. Nat Med 2019;25:954-61. [Crossref] [PubMed]
- Nagpal K, Foote D, Liu Y, Chen PC, Wulczyn E, Tan F, Olson N, Smith JL, Mohtashamian A, Wren JH, Corrado GS, MacDonald R, Peng LH, Amin MB, Evans AJ, Sangoi AR, Mermel CH, Hipp JD, Stumpe MC. Development and validation of a deep learning algorithm for improving Gleason scoring of prostate cancer. NPJ Digit Med 2019;2:48. [Crossref] [PubMed]
- McKinney SM, Sieniek M, Godbole V, Godwin J, Antropova N, Ashrafian H, et al. International evaluation of an AI system for breast cancer screening. Nature 2020;577:89-94. [Crossref] [PubMed]
- Zhou C, Ban X, Lv J, Cheng L, Xu J, Shen X. Role of computed tomography features in the differential diagnosis of chromophobe renal cell carcinoma from oncocytoma and angiomyolipoma without visible fat. Quant Imaging Med Surg 2022;12:2332-43. [Crossref] [PubMed]
- Zhou L, Zhang Z, Chen YC, Zhao ZY, Yin XD, Jiang HB. A Deep Learning-Based Radiomics Model for Differentiating Benign and Malignant Renal Tumors. Transl Oncol 2019;12:292-300. [Crossref] [PubMed]
- Deng Y, Soule E, Samuel A, Shah S, Cui E, Asare-Sawiri M, Sundaram C, Lall C, Sandrasegaran K. CT texture analysis in the differentiation of major renal cell carcinoma subtypes and correlation with Fuhrman grade. Eur Radiol 2019;29:6922-9. [Crossref] [PubMed]
- Bektas CT, Kocak B, Yardimci AH, Turkcanoglu MH, Yucetas U, Koca SB, Erdim C, Kilickesmez O. Clear Cell Renal Cell Carcinoma: Machine Learning-Based Quantitative Computed Tomography Texture Analysis for Prediction of Fuhrman Nuclear Grade. Eur Radiol 2019;29:1153-63. [Crossref] [PubMed]
- Bossuyt PM, Reitsma JB, Bruns DE, Gatsonis CA, Glasziou PP, Irwig L, Lijmer JG, Moher D, Rennie D, de Vet HC, Kressel HY, Rifai N, Golub RM, Altman DG, Hooft L, Korevaar DA, Cohen JF. STARD 2015: an updated list of essential items for reporting diagnostic accuracy studies. BMJ 2015;351:h5527. [Crossref] [PubMed]
- Yushkevich PA, Piven J, Hazlett HC, Smith RG, Ho S, Gee JC, Gerig G. User-guided 3D active contour segmentation of anatomical structures: significantly improved efficiency and reliability. Neuroimage 2006;31:1116-28. [Crossref] [PubMed]
- Isensee F, Jaeger PF, Kohl SAA, Petersen J, Maier-Hein KH. nnU-Net: a self-configuring method for deep learning-based biomedical image segmentation. Nat Methods 2021;18:203-11. [Crossref] [PubMed]
- van Griethuysen JJM, Fedorov A, Parmar C, Hosny A, Aucoin N, Narayan V, Beets-Tan RGH, Fillion-Robin JC, Pieper S, Aerts HJWL. Computational Radiomics System to Decode the Radiographic Phenotype. Cancer Res 2017;77:e104-7. [Crossref] [PubMed]
- Chen T, Guestrin C. XGBoost: A Scalable Tree Boosting System. Proceedings of the 22nd ACM SIGKDD International Conference on Knowledge Discovery and Data Mining. San Francisco, California, USA: Association for Computing Machinery; 2016:785-94.
- Sokolova M, Lapalme G. A systematic analysis of performance measures for classification tasks. Information Processing & Management 2009;45:427-37. [Crossref]
- Lundberg SM, Erion G, Chen H, DeGrave A, Prutkin JM, Nair B, Katz R, Himmelfarb J, Bansal N, Lee SI. From Local Explanations to Global Understanding with Explainable AI for Trees. Nat Mach Intell 2020;2:56-67. [Crossref] [PubMed]
- Motzer RJ, Jonasch E, Boyle S, Carlo MI, Manley B, Agarwal N, et al. NCCN Guidelines Insights: Kidney Cancer, Version 1.2021. J Natl Compr Canc Netw 2020;18:1160-70. [Crossref] [PubMed]
- Teishima J, Hayashi T, Kitano H, Sadahide K, Sekino Y, Goto K, Inoue S, Honda Y, Sentani K, Awai K, Yasui W, Matsubara A. Impact of radiological morphology of clinical T1 renal cell carcinoma on the prediction of upstaging to pathological T3. Jpn J Clin Oncol 2020;50:473-8. [Crossref] [PubMed]
- Renard AS, Nedelcu C, Paisant A, Saulnier P, Le Bigot J, Azzouzi AR, Bigot P, Aubé C. Is multidetector CT-scan able to detect T3a renal tumor before surgery? Scand J Urol 2019;53:350-5. [Crossref] [PubMed]
- Yang X, Sun W, Huang D, Li H, Zhao Y, Li P, Liu Y. Quantitative spectral CT evaluation of kidney tumors with the stretched-exponential nonlinear regression analysis model. Quant Imaging Med Surg 2021;11:676-84. [Crossref] [PubMed]
- Cui EM, Lin F, Li Q, Li RG, Chen XM, Liu ZS, Long WS. Differentiation of renal angiomyolipoma without visible fat from renal cell carcinoma by machine learning based on whole-tumor computed tomography texture features. Acta Radiol 2019;60:1543-52. [Crossref] [PubMed]
- Yu H, Scalera J, Khalid M, Touret AS, Bloch N, Li B, Qureshi MM, Soto JA, Anderson SW. Texture analysis as a radiomic marker for differentiating renal tumors. Abdom Radiol (NY) 2017;42:2470-8. [Crossref] [PubMed]
- Ding J, Xing Z, Jiang Z, Chen J, Pan L, Qiu J, Xing W. CT-based radiomic model predicts high grade of clear cell renal cell carcinoma. Eur J Radiol 2018;103:51-6. [Crossref] [PubMed]
- Wu K, Wu P, Yang K, Li Z, Kong S, Yu L, Zhang E, Liu H, Guo Q, Wu S. A comprehensive texture feature analysis framework of renal cell carcinoma: pathological, prognostic, and genomic evaluation based on CT images. Eur Radiol 2022;32:2255-65. [Crossref] [PubMed]
- Demirjian NL, Varghese BA, Cen SY, Hwang DH, Aron M, Siddiqui I, Fields BKK, Lei X, Yap FY, Rivas M, Reddy SS, Zahoor H, Liu DH, Desai M, Rhie SK, Gill IS, Duddalwar V. CT-based radiomics stratification of tumor grade and TNM stage of clear cell renal cell carcinoma. Eur Radiol 2022;32:2552-63. [Crossref] [PubMed]