Validation of hemodynamic stress calculation in coronary computed tomography angiography versus intravascular ultrasound
Introduction
Coronary artery disease (CAD) is a major cause of morbidity and mortality worldwide. Explorations in computational fluid dynamics (CFD) suggested that mechanical forces, especially wall shear stress (WSS) and axial plaque stress (APS), promote plaque growth and vulnerable phenotype transformation, resulting in a higher risk of plaque erosion and rupture(1-4). Clinical studies found corresponding results that WSS and APS determination provided enhanced identification and prediction of major adverse cardiac events (MACEs) (5-8).
The geometry of coronary arteries has a great impact on the forces against vessel walls, including the normal wall and the plaques. Therefore, an accurate three-dimensional (3D) construction of coronary geometry appears to be essential for further CFD calculations. However, CFD has not been routinely used in clinical practice. On the other hand, most coronary artery mechanical forces are commonly derived and calculated from the combination of coronary angiograms (CAGs) and intravascular ultrasound/intravascular coronary angiography (IVUS/ICA) (9), which is time-consuming and requires invasive operations. Furthermore, IVUS/ICA requires highly skilled clinicians and involves a high dose of radiation.
Parallel to the development of CFD, advances in coronary computed tomography angiography (CCTA) allowed higher resolution, reduced artifacts and an accurate demonstration of the coronary artery tree, enabling the application of CFD in a cost-effective and noninvasive way (8,10-12). However, to our knowledge, few studies have examined the agreement and correlation between the indices measured by IVUS and those measured by CCTA (13). The purpose of our study was to establish a reliable mechanical force calculation methodology derived from CCTA and further investigate the agreement in CFD calculations between CCTA and the reference standard. We present the following article in accordance with the STARD reporting checklist (available at https://qims.amegroups.com/article/view/10.21037/qims-22-832/rc).
Methods
Study sample
The present study was a retrospective, single-site study. We consecutively collected the patients with unstable angina who underwent both CCTA and IVUS/ICA at an interval of less than 7 days for diagnosis or therapeutic operation at the Chinese PLA General Hospital within the year of 2021. Patients with a history of myocardial infarction or previous coronary revascularization of the IVUS/ICA examined artery were excluded. Patients who were found to have complete occlusion in the IVUS/ICA examined artery either were also excluded. Those with incomplete baseline data or of poor image quality for CFD analysis were excluded from further analysis. Thirteen patients (14 arteries) were eventually included in the cohort. Patients’ 10-year overall atherosclerotic cardiovascular disease (ASCVD) risk were classified as high risk (≥10%), intermediate risk (5−9%), and low risk (<5%) separately based on clinical disease status, lipoprotein cholesterol levels and other traditional risk factors in accordance with the 2016 guidelines for the management of dyslipidemia in Chinese adults (14). This study was approved by the Ethics Committee of Chinese PLA General Hospital (No. S2020-255-01) and was in accordance with the guidelines of the Declaration of Helsinki (as revised in 2013). Individual consent for this retrospective analysis was waived.
Imaging modalities
CCTA data acquisition
Multidetector CCTA scans were performed on a dual-source CT scanner (Somatom Definition Flash CT, Siemens Medical Solutions, Forchreim, Germany) using prospective electrocardiographic gating. All images were acquired at end-diastole and were analysed using a dedicated workstation (Syngo.via, Siemens) by two level III-trained experts using a 17-segment coronary artery tree model. Patients with a heart rate of 65 beats per minute or higher prior to CT received 50 mg of metoprolol orally 1 hour before the examination, unless contraindicated. All patients received a 0.5 mg dose of nitroglycerin immediately prior to scanning, unless contraindicated (15). The scan parameters were as follows: 2 mm × 64 mm × 0.6 mm section collimation, 280 ms rotation time, 100 or 120 kVp tube voltage (depending on body habitus), and 290–560 mAs tube current (depending on body habitus). A 4-point Likert scales were applied to assess the image quality as previously described (16), and arteries with a Likert score under 3 were excluded.
IVUS/ICA data acquisition
All interventional procedures were done by an experienced interventional team with >15 years’ experience. Image acquisition with IVUS was performed after the administration of intracoronary nitroglycerin with a phased-array 40-MHz Sion blue Catheter and iLab system (Boston Scientific/Scimed, USA). Automated motorized pullback (1 mm/s) was performed, and IVUS images were continuously acquired up to the guide catheter in the aorta. IVUS and AX images were conducted in synchronization, and end-diastolic frames were used to reconstruct the vessel geometry (17,18).
3D reconstruction of the coronary tree and regions of interest
An in-house code was applied to identify the lumen borders and semi-automatically segment the full coronary tree into point cloud data using grayscale threshold and a region-growing algorithm in CCTA (Figure 1). Corrections were made when necessary by two experienced readers to ensure accurate segmentation. Disagreements between two readers were resolved by consensus reading. Using erosion and dilation, the coronary tree point cloud was segmented from the aorta. 3D centerline was generated using the in-house code based on the scikit-image package in Python.
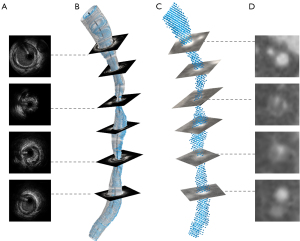
The lumen borders and centerline were manually identified in 2 end-diastolic frames selected from CAG, which was then used to recreate the arterial 3D centerline. The lumen borders from IVUS were manually outlined and positioned perpendicularly to the 3D centerline. The orientation of the IVUS frames was adjusted using the sequential triangulation method to find the best-fit absolute orientation of the reconstructed lumen (19). The individual lumen contours were then connected forming the 3D lumen surface (20). The 3D reconstruction model was output using an in-house code. The AX images were also used to ensure the general shape of the vessel and the location of branches from IVUS matched that of CCTA. Anatomic landmarks such as side branches and bifurcations were used to match the longitudinal and circumferential location of the plaque in CCTA and IVUS/ICA (Figure 1). The side branches (>1.5 mm) were segmented in the CCTA, scaled to the IVUS lumen contours and fused with the IVSU/ICA models to ensure the consistency of the flow of the inlet and outlets between these two modalities.
The myocardial mass was output using in-house code through CCTA images. Segmentation was achieved using Geomagic Studio 11 (Geomagic, Inc., Research Triangle Park, NC, USA). Each 3D-reconstruction was converted to a tetrahedral mesh with a 5-layer prism layer at the boundary using the open-source software FreeCAD (https://www.freecadweb.org/), with a maximum element size of 0.1–0.2 mm in the stenotic lumen and of 0.35–0.5 mm in the reference lumen. Mesh independence test at similar scale was conducted in a previous study validating the feasibility of WSS simulation using CCTA images (21).
The regions of interest were the vessel segments between the side branches proximal and distal to the lesion or the 10-mm segments proximal and distal to the lesion when the distance between the side branches and the lesion was longer than 10 mm. Alongside the centerline, slices were made every 1 mm, and the magnitude of lumen area, velocity, pressure, mean WSS and mean APS were assessed on each slice. The same region of interest was analyzed for each modality.
CFD and analysis of hemodynamic stresses
Mathematical model
Blood was assumed to be an incompressible Newtonian fluid as the blood flow was assumed to be constant, adiabatic and laminar. And the no-slip boundary condition was applied at the vessel wall (1). Then, coronary flow and pressure were computed by solving the Navier-Stokes equations numerically (22):
where ρ is the blood density equal to 1,060 kg/m3; µ is the velocity vector; p is the pressure function; ui is the velocity component; µ is the blood viscosity, which is equal to 0.0035 Pa·s; and xi is the coordinate (23).
Boundary conditions and CFD
To obtain the velocity vector u by solving the Navier-Stokes equations, we need to set the pressure function.
The inlet boundary pressure was set equal to the mean aortic pressure:
The pressure on the outlet of the coronary artery was calculated using the coupled lumped-parameter model (24,25):
where Poutlet,i is the pressure on the i-th outlet, Qoutlet,iis the flow on the i-th outlet, Ri is the resistance on the i-th outlet and Pv is the venous pressure.
Then, the resting coronary flow on each outlet was computed according to the allometric scaling law using the myocardial mass:
where Qtotal is the total flow, Mmyo is the myocardial mass and a and b are constants. For the main artery with n side branches, the flow in each side branch is proportional to the diameter of the side branches to the power of 3 (26), which can be expressed as
where Qi is the flow on i-th side branch, di is the diameter of the i-th side branch and Qm is the flow of the main artery. After recursive uses of Murray’s law to allocate the flow, we can get the flow on the outlet using (2). The pressure on side branches was calculated under a simulated hyperemic condition. Thus, the velocity vector u can be solved using the finite element method.
Calculation of hemodynamic stresses
The aforementioned hemodynamic stresses were evaluated along the coronary centerline for each patient. The traction against the vessel wall () can be decomposed into a normal component () and a tangential component (). Another way to decompose the traction is based on the centerline of the vessel, introducing , which can be computed by the projection of onto the centerline. The values of WSS and APS were computed as follows:
and
All the calculation was performed using OpenFOAM 9 (OpenFOAM Foundation, Ltd., London, UK). The simpleFOAM solver was used to solve the steady-state laminar Navier-Stokes equations and the simple algorithm was implemented for pressure-velocity coupling. The criteria of convergence were chosen as 10–5 for the normalized continuity, velocity, and pressure residuals. And an example of WSS distribution in 3D models and 2D map derived from IVUS/ICA and CCTA is presented in Figure 2.
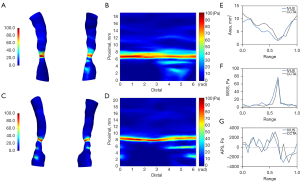
Calculation of calcium arc
The arc of plaques with an attenuation of more than 350 Hounsfield units (HU) were measured on the lumen every 1 mm, and the maximal arc of calcium deposits was identified (27). Mild and severe calcification were defined as calcium deposits with an arc of <90° and ≥90°, respectively. Vessels of interest were stratified into a mild calcified subgroup and a severe calcified subgroup according to the calcium arc.
Statistical analysis
Continuous data were presented as mean ± standard deviation or median with interquartile range. The comparison between two groups was performed using Student t-test or Mann-Whitney U test. Categorical data were presented as percentages and compared using the Chi-square test or Fisher’s exact test as appropriate. Correlations were assessed using Pearson’s or Spearman’s correlation when appropriate. IVUS/ICA image processing of 3 randomly selected vessels was conducted by 2 researchers, and reproducibility was evaluated using the intraclass correlation coefficient (ICC). Bland-Altman analysis was used to show the discrepancies between variables from CCTA and IVUS/ICA. Statistical analysis was performed by using Statistics 22.0 (IBM, Chicago, IL, USA), and a two-sided P value of ≤0.05 was considered significant.
Results
Baseline data
The baseline demographics and clinical characteristics of all enrolled patients are described in Table 1. The study population consisted of 13 patients (mean age: 61.0±9.9; male: 84.6%), among whom 9 had a history of hypertension, 4 had a history of diabetes and the majority of the patients received anti-platelet (84.6%) and statin (76.9%) therapy. At the CCTA baseline examination, ASCVD risk stratification identified patients at high risk (15.4%), intermediate risk (61.5%) and low risk (23.1%), respectively. 3D reconstruction and hemodynamic calculation were successfully performed in all 14 arteries. The mean length of the reconstructed vessels was 26.07±9.56 mm, ranging from 18.4 to 53.4 mm. Among the included arteries, 12 were moderately calcified, and 2 were severely calcified.
Table 1
Characteristic | Value (N=13) |
---|---|
Age, years | 61.0±9.9 |
Male, n (%) | 11 (84.6) |
Body mass index, kg/m2 | 26.2±2.6 |
Mean pressure, mmHg | 92.2±10.9 |
Clinical risk factors | |
Hypertension | 9 (69.2) |
Current smoking | 5 (38.5) |
Diabetes mellitus | 4 (30.8) |
Dyslipidemia | 6 (46.2) |
ASCVD risk stratification | |
High risk | 2 (15.4) |
Intermediate risk | 8 (61.5) |
Low risk | 3 (23.1) |
Medication | |
Aspirin | 10 (76.9) |
Clopidogrel | 6 (46.2) |
Ticagrelor | 1 (7.7) |
Statin | 10 (76.9) |
Data are presented as mean ± standard deviation or n (%). ASCVD, atherosclerotic cardiovascular disease.
Reproducibility of IVUS simulation
Intraobserver and interobserver reproducibility for hemodynamic quantities on IVUS/ICA assessment were evaluated in 3 randomly selected vessels. Good to excellent correlation and ICCs were observed in all the parameters evaluated for both intra- and interobserver agreement (Table 2). Intraobserver agreement and interobserver agreement for WSS were ICCs of 0.926 and 0.928 (P<0.001 for both) and 0.884 and 0.910 for APS, respectively. In the Bland-Altman analysis, the mean differences in WSS (with the 95% limits of agreement) were 0.1625 (−1.819 to 2.144) and 0.1580 (−1.676 to 1.992) for intraobserver and interobserver measurements, respectively.
Table 2
Parameter | Interobserver agreement | Intraobserver agreement | |||
---|---|---|---|---|---|
Pearson r | ICC (95% CI) | Pearson r | ICC (95% CI) | ||
Area (mm2) | 0.980 | 0.993 (0.990–0.996) | 0.982 | 0.991 (0.987–0.994) | |
Velocity (cm/s) | 0.992 | 0.994 (0.990–0.996) | 0.989 | 0.992 (0.988–0.995) | |
Re | 0.991 | 0.990 (0,985–0.994) | 0.990 | 0.988 (0.981–0.992) | |
Pressure (Pa) | 0.970 | 0.988 (0.982–0.992) | 0.937 | 0.979 (0.969–0.986) | |
WSS (Pa) | 0.936 | 0.928 (0.888–0.953) | 0.920 | 0.926 (0.890–0.950) | |
APS (Pa) | 0.802 | 0.910 (0.861–0.942) | 0.748 | 0.884 (0.827–0.922) |
Data are presented as mean ± standard deviation. For all the variables, the Pearson’s correlation coefficient r was significant (P<0.01). CFD, computational fluid dynamics; IVUS intravascular ultrasound; ICA, intravascular coronary angiography; ICC, intraclass correlation coefficient; CI, confidence interval; Re, Reynolds number; WSS, wall shear stress; APS, axial plaque stress.
Statistical analysis of all slice-level parameters
As shown in Table 3, the percent diameter stenosis (41.2%±15.1% vs. 27.6%±13.8%, P=0.004) and the percent area stenosis (50.2%±16.2% vs. 36.4%±19.4%, P=0.004) were larger than those derived from IVUS/ICA, while the MLA (5.81±2.24 vs. 6.72±2.04 mm2, P=0.126) demonstrated no significant difference between CT and IVUS. Among the slice-level parameters (Table 4), lumen area (10.23±3.62 vs. 9.49±2.71 mm2, P<0.001) measured in CCTA was significantly larger than the value calculated in IVUS, echoing the results from Table 3, as the percent area stenosis was calculated by the function: area stenosis% = (1 − MLA/reference lumen area) ×100%. CCTA demonstrated a lower blood velocity (38.08±15.69 vs. 38.41±14.25 cm/s, P=0.053), a higher Reynolds number (399.40±153.28 vs. 398.18±135.69, P<0.001), and a lower pressure (85.94±3.43 vs. 86.99±1.80, P<0.001) than IVUS. Notwithstanding, no significant difference was observed in WSS (6.57±6.18 vs. 6.04±5.50 Pa, P=0.560) or APS (19.14±1,150.91 vs. 7.25±887.38 Pa, P=0.770) between CCTA and IVUS. Bland–Altman analysis of WSS and APS is presented in Figure 3.
Table 3
Parameter | IVUS/ICA | CCTA | P |
---|---|---|---|
Minimal lumen diameter, mm | 2.60±0.48 | 2.11±0.47 | 0.004 |
Percent diameter stenosis, % | 27.6±13.8 | 41.2±15.1 | 0.004 |
Minimal lumen area, mm2 | 6.72±2.04 | 5.81±2.24 | 0.126 |
Percent area stenosis, % | 36.4±19.4 | 50.2±16.2 | 0.004 |
Data are presented as mean ± standard deviation. CCTA, coronary computed tomography angiograms; IVUS intravascular ultrasound; ICA, invasive coronary angiography.
Table 4
Parameter | IVUS | CCTA | P | Pearson r | ICC (95% CI) |
---|---|---|---|---|---|
Area (mm2) | 9.49±2.71 | 10.23±3.62 | <0.001 | 0.621 | 0.747 (0.692–0.792) |
Velocity (cm/s) | 38.41±14.25 | 38.08±15.69 | 0.053 | 0.665 | 0.796 (0.752–0.833) |
Re | 398.18±135.69 | 399.40±153.28 | <0.001 | 0.686 | 0.810 (0.768–0.844) |
Pressure (Pa) | 86.99±1.80 | 85.94±3.43 | <0.001 | 0.506 | 0.589 (0.499–0.662) |
WSS (Pa) | 6.04±5.50 | 6.57±6.18 | 0.560 | 0.628 | 0.769 (0.718–0.810) |
APS (Pa) | 7.25±887.38 | 19.14±1,150.91 | 0.770 | 0.212 | 0.341 (0.197–0.458) |
Data are presented as mean ± standard deviation. For all the variables, the Pearson’s correlation coefficient r was significant (P<0.01). CCTA, coronary computed tomography angiograms; IVUS intravascular ultrasound; ICA, invasive coronary angiography; ICC, intraclass correlation coefficient; CI, confidence interval; Re, Reynolds number; WSS, wall shear stress; APS, axial plaque stress.
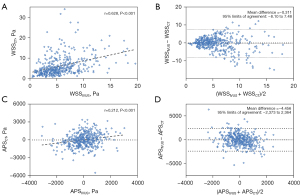
ICC evaluation was used to further assess the correlation between CT and IVUS, where good correlation was found for velocity [ICC: 0.796, 95% CI: 0.752–0.833], Reynolds number [ICC: 0.810, 95% CI: 0.768–0.844] and WSS [ICC: 0.769, 95% CI: 0.718–0.810], while the ICC of APS was 0.341 [95% CI: 0.197–0.458], indicating relatively poor correlation (Table 4).
Impact of calcification on CCTA simulation
In the mildly calcified vessels, good or moderate agreement was found between CCTA and IVUS/ICA in area, velocity, Reynolds number, pressure, WSS and APS, with an ICC of 0.750, 0.810, 0.795, 0.750, 0.800 and 0.415 (Figure 4). For severely calcified vessels, similar results were observed in area (0.793), velocity (0.772) and Reynolds number (0.798), while a significantly lower agreement was found in pressure (0.386), WSS (0.479) and APS (0.230).
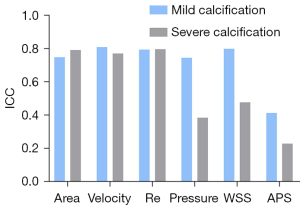
Discussion
The present retrospective study was designed to examine the accuracy of CCTA compared with the reference standard IVUS/ICA. Our study demonstrated the following:
- CCTA presented an underestimation of intravascular area and an overestimation of stenosis severity in comparison to IVUS/ICA;
- Parameters derived from CCTA had moderate to good correlation with IVUS/ICA parameters;
- Calcification had a negative impact on CCTA performance in CFD calculation. Explorations have been made to allow CFD based on CCTA to calculate forces against the vessel wall.
As CCTA becomes the most commonly used modality in clinical CAD severity evaluation and risk prediction, there comes a high demand for more than the accuracy of morphology but also the functional evaluation and hemodynamic calculation. Previous studies have shown a wide variety of agreements between CCTA and invasive parameters (13,15,16,28,29). However, no agreement was found on the degree of the over- or underestimation of lesion severity in CCTA and IVUS/ICA parameters.
Comparison between CCTA- and IVUS-derived morphology measurement
According to previous studies, hemodynamic parameters are highly sensitive to morphology. Our study is consistent with previous studies that compared the minimal lumen area and found an exaggeration of CCTA area stenosis% compared with IVUS/ICA. However, the CCTA mean lumen area was found to be larger than the IVUS/ICA mean lumen area, which was supported by Park et al. (15) and Gauss et al. (28), but was different from the reports by Doh et al. (29). These contradictory results may be due to discrepancies in the lumen delineation method, lesion characteristics, stenosis severity, lesion location side branch exclusion in IVUS and small sample size (30,31). In our study, the delineation of IVUS was manually managed to ensure the accuracy and precision of the lumen morphology. Thus, the inter-/intraoperator agreement was measured to examine the reproducibility and robustness of IVUS/ICA, which showed excellent consistency in both conditions. However, the lumen from CCTA was recognized with in-house code using a hierarchy strategy, which tends to draw the lumen as near circular. Moreover, the lower resolution, effect of scattering, and blooming effect resulting from calcification may contribute to the discrepancy between CCTA and IVUS/ICA measurements. Another point that is worth mentioning is that our study included a high proportion of arteries with diffuse plaques, with an average length of 21.5 mm, and 79% of the lesions had a length of more than 1 cm, increasing the difficulty in reference selection.
Comparison between CCTA- and IVUS-derived hemodynamic stresses
Only a small number of studies have examined the discrepancy in hemodynamic stresses. In the present study, the WSS and APS of CCTA were numerically greater than those of IVUS, although no significant difference was found between these two modalities. WSSCCTA showed an excellent correlation with WSSIVUS. Bulant et al. (26) described the overestimation of WSS as well in their study, where 11 patients (16 arteries) were included and sample-wide and vessel-wide comparisons through CCTA and IVUS/ICA were analyzed. The researchers also verified an equivalence in terms of fractional flow reserve (FFR) (differences smaller than 1%) and time-averaged WSS (differences smaller than 4%) by comparing steady-state and pulsatile simulations for both imaging modalities. Eslami et al. (13) showed detailed agreement of segment-averaged as well as arc-averaged between the two imaging modalities using standard CCTA and IVUS/optical coherence tomography (OCT) and found a higher value of WSS in CCTA but with a similar pattern and relatively steady discrepancy along the centerline, which is consistent with our result.
To our knowledge, the present study is the first to examine the agreement of APS between CCTA and IVUS; however, we have not yet reached an ideal result. The reasons may be as follows: (I) APS has a large-scale unit and can also be a positive or negative value, which means that calculating the average APS across the slice is easily subject to fluctuating value. (II) As deduced from Eq. [1], the APS is highly sensitive to the morphological differences between models derived from the modalities, which is associated not only with the change in lumen area but also with the smoothness of the vessel wall, requiring a higher accuracy and consistency of model construction. More extensive reconstruction and improved image quality are essential for further APS analysis.
Impact of calcification
Due to blooming effect calcification, especially severe calcification, a larger volume with higher density may mislead readers in the identification of the lumen boundary and result in an underestimation of lumen area. Studies found that this impact was posed not only from an anatomic aspect but also on the functional assessment. Multiple CCTA-based methods, such as CT-FFR and the perivascular fat attenuation index, have been created to improve the risk prediction of CAD, and similar difficulties have been encountered in the face of severe calcification (32-34). In the present study, larger calcifications showed a negative influence on the agreement between CCTA and IVUS measurements. With the development of CT of higher resolution and the emergence of new processing methods, this problematic situation may be improved in the near future.
Clinical impact of hemodynamic stresses derived from CCTA
Although not as commonly used as intravascular modalities, a few studies based on CCTA revealed the association between hemodynamic stresses and vulnerable plaque characteristics and a higher risk of adverse cardiac events. High WSS was found to be strongly related to vulnerable OCT findings, such as lipid tissue with a thin fibrous cap (LTFC) and a higher lipid index (3). The Emerald trial (35) indicated that high WSS and high APS, together with ΔCT-FFR and CT-FFR, can provide incremental value over anatomical parameters and adverse plaque characteristics in the assessment of acute cardiac syndrome (ACS). The team reasserted the point in another study (36) that high WSS and high APS can optimize risk stratification compared with using impaired CT-FFR alone for ACS.
One distinct advantage of CCTA over intravascular modalities is its facility for comprehensively evaluating the global coronary tree and serially monitoring plaque development, which are essential for clinical risk assessment and therapy decision-making. Moreover, CCTA is a potential tool for the clinical paradigm shift into a “one-stop shop”, which offers a collection of functional assessments such as CT-FFR, hemodynamic stresses and risk scores other than anatomical analysis. In the meantime, CCTA is cost-effective and time-saving in comparison to its counterparts. Despite its current limitation in time and spatial resolution and the relatively complex procedures of CFD calculation, hemodynamic stress derived from CCTA still sheds light on more personalized risk stratification of adverse cardiac events. Newly emerged technologies such as photon-counting CT with prime spatial resolution and processing power based on artificial intelligent may facilitate CFD calculation in clinical practice.
Limitations
There are several limitations to be acknowledged. First, we included both the left anterior descending coronary artery and right coronary artery without further stratification due to a relatively small sample size. Discrepancies in the number of side branches, movement through cardiac cycles and vessel curvature may have an additional impact on the forces. Another limitation is that segmentation procedures involved manual processing, which might be solved with the development of relevant tools. Finally, it should be mentioned that steady-state simulation was applied in this study, which means that the changes throughout the cardiac cycle were not recorded in the research. Although it was proven to be a satisfactory alternative for pulsatile simulation, we must admit that information loss might exist as a source of error.
Conclusions
Our study found that CCTA can serve as a satisfactory alternative for the reference standard, IVUS/ICA, in morphology simulation and hemodynamic stress calculation, especially in the calculation of WSS. More intensive work is still required to improve the accuracy of APS calculation.
Acknowledgments
We acknowledge Zhihong Lin, Yunxia Lu and Xingli Liu (Hangzhou Shengshi Technology) for technical support.
This work was partially presented at the 33rd Great Wall International Congress of Cardiology Asian Heart Society Congress and the 10th Annual Meeting of the Society of Cardiovascular Computed Tomography China IRC in 2022.
Funding: This work was supported by grants from National Key R&D Program of China (No. 2016YFC1300304 to YC and No. 2021YFC2500505 to JY), Beijing NOVA Program (No. Z181100006218055 to JY), and Medical big data program of PLAGH (No. 2019MBD-035 to JY).
Footnote
Reporting Checklist: The authors have completed the STARD reporting checklist. Available at https://qims.amegroups.com/article/view/10.21037/qims-22-832/rc
Conflicts of Interest: All authors have completed the ICMJE uniform disclosure form (available at https://qims.amegroups.com/article/view/10.21037/qims-22-832/coif). JY reports support from National Key R&D Program of China (No. 2021YFC2500505), Beijing NOVA Program (No. Z181100006218055), and medical big data program of PLAGH (No. 2019MBD-035). YC reports support from National Key R&D Program of China (No. 2016YFC1300304). The other authors have no conflicts of interest to declare.
Ethical Statement: The authors are accountable for all aspects of the work in ensuring that questions related to the accuracy or integrity of any part of the work are appropriately investigated and resolved. The study was conducted in accordance with the Declaration of Helsinki (as revised in 2013). The study was approved by the Ethics Committee of the Chinese PLA General Hospital and individual consent for this retrospective analysis was waived.
Open Access Statement: This is an Open Access article distributed in accordance with the Creative Commons Attribution-NonCommercial-NoDerivs 4.0 International License (CC BY-NC-ND 4.0), which permits the non-commercial replication and distribution of the article with the strict proviso that no changes or edits are made and the original work is properly cited (including links to both the formal publication through the relevant DOI and the license). See: https://creativecommons.org/licenses/by-nc-nd/4.0/.
References
- Kumar A, Thompson EW, Lefieux A, Molony DS, Davis EL, Chand N, et al. High Coronary Shear Stress in Patients With Coronary Artery Disease Predicts Myocardial Infarction. J Am Coll Cardiol 2018;72:1926-35. [Crossref] [PubMed]
- Shishikura D, Sidharta SL, Honda S, Takata K, Kim SW, Andrews J, Montarello N, Delacroix S, Baillie T, Worthley MI, Psaltis PJ, Nicholls SJ. The relationship between segmental wall shear stress and lipid core plaque derived from near-infrared spectroscopy. Atherosclerosis 2018;275:68-73. [Crossref] [PubMed]
- Toba T, Otake H, Choi G, Kim HJ, Onishi H, Sugizaki Y, Takeshige R, Nagasawa A, Nagano Y, Tsukiyama Y, Yanaka K, Yamamoto H, Kawamori H, Mori S, Kawata M, Taylor CA, Hirata KI. Wall Shear Stress and Plaque Vulnerability: Computational Fluid Dynamics Analysis Derived From cCTA and OCT. JACC Cardiovasc Imaging 2021;14:315-7. [Crossref] [PubMed]
- Kok AM, Molony DS, Timmins LH, Ko YA, Boersma E, Eshtehardi P, Wentzel JJ, Samady H. The influence of multidirectional shear stress on plaque progression and composition changes in human coronary arteries. EuroIntervention 2019;15:692-9. [Crossref] [PubMed]
- Kogo T, Hiro T, Kitano D, Takayama T, Fukamachi D, Morikawa T, Sudo M, Okumura Y. Macrophage accumulation within coronary arterial wall in diabetic patients with acute coronary syndrome: a study with in-vivo intravascular imaging modalities. Cardiovasc Diabetol 2020;19:135. [Crossref] [PubMed]
- Timmins LH, Molony DS, Eshtehardi P, McDaniel MC, Oshinski JN, Giddens DP, Samady H. Oscillatory wall shear stress is a dominant flow characteristic affecting lesion progression patterns and plaque vulnerability in patients with coronary artery disease. J R Soc Interface 2017;14:20160972. [Crossref] [PubMed]
- Choi G, Lee JM, Kim HJ, Park JB, Sankaran S, Otake H, Doh JH, Nam CW, Shin ES, Taylor CA, Koo BK. Coronary Artery Axial Plaque Stress and its Relationship With Lesion Geometry: Application of Computational Fluid Dynamics to Coronary CT Angiography. JACC Cardiovasc Imaging 2015;8:1156-66. [Crossref] [PubMed]
- Kalykakis GE, Antonopoulos AS, Pitsargiotis T, Siogkas P, Exarchos T, Kafouris P, Sakelarios A, Liga R, Tzifa A, Giannopoulos A, Scholte AJHA, Kaufmann PA, Parodi O, Knuuti J, Fotiadis DI, Neglia D, Anagnostopoulos CD. Relationship of Endothelial Shear Stress with Plaque Features with Coronary CT Angiography and Vasodilating Capability with PET. Radiology 2021;300:549-56. [Crossref] [PubMed]
- Jiang J, Feng L, Li C, Xia Y, He J, Leng X, Dong L, Hu X, Wang J, Xiang J. Fractional flow reserve for coronary stenosis assessment derived from fusion of intravascular ultrasound and X-ray angiography. Quant Imaging Med Surg 2021;11:4543-55. [Crossref] [PubMed]
- Newby DE, Adamson PD, Berry C, Boon NA, Dweck MR, Flather M, Forbes J, Hunter A, Lewis S, MacLean S, Mills NL, Norrie J, Roditi G, Shah ASV, Timmis AD, van Beek EJR, Williams MC, Coronary CT. Angiography and 5-Year Risk of Myocardial Infarction. N Engl J Med 2018;379:924-33. [Crossref] [PubMed]
- Tufaro V, Safi H, Torii R, Koo BK, Kitslaar P, Ramasamy A, Mathur A, Jones DA, Bajaj R, Erdoğan E, Lansky A, Zhang J, Konstantinou K, Little CD, Rakhit R, Karamasis GV, Baumbach A, Bourantas CV. Wall shear stress estimated by 3D-QCA can predict cardiovascular events in lesions with borderline negative fractional flow reserve. Atherosclerosis 2021;322:24-30. [Crossref] [PubMed]
- Cong M, Zhao H, Dai S, Chen C, Xu X, Qiu J, Qin S. Transient numerical simulation of the right coronary artery originating from the left sinus and the effect of its acute take-off angle on hemodynamics. Quant Imaging Med Surg 2021;11:2062-75. [Crossref] [PubMed]
- Eslami P, Hartman EMJ, Albaghadai M, Karady J, Jin Z, Thondapu V, Cefalo NV, Lu MT, Coskun A, Stone PH, Marsden A, Hoffmann U, Wentzel JJ. Validation of Wall Shear Stress Assessment in Non-invasive Coronary CTA versus Invasive Imaging: A Patient-Specific Computational Study. Ann Biomed Eng 2021;49:1151-68. [Crossref] [PubMed]
- 2016 Chinese guidelines for the management of dyslipidemia in adults. J Geriatr Cardiol 2018;15:1-29. [PubMed]
- Park HB, Lee BK, Shin S, Heo R, Arsanjani R, Kitslaar PH, Broersen A, Dijkstra J, Ahn SG, Min JK, Chang HJ, Hong MK, Jang Y, Chung N. Clinical Feasibility of 3D Automated Coronary Atherosclerotic Plaque Quantification Algorithm on Coronary Computed Tomography Angiography: Comparison with Intravascular Ultrasound. Eur Radiol 2015;25:3073-83. [Crossref] [PubMed]
- Nakanishi R, Sankaran S, Grady L, Malpeso J, Yousfi R, Osawa K, Ceponiene I, Nazarat N, Rahmani S, Kissel K, Jayawardena E, Dailing C, Zarins C, Koo BK, Min JK, Taylor CA, Budoff MJ. Automated estimation of image quality for coronary computed tomographic angiography using machine learning. Eur Radiol 2018;28:4018-26. [Crossref] [PubMed]
- Costopoulos C, Timmins LH, Huang Y, Hung OY, Molony DS, Brown AJ, Davis EL, Teng Z, Gillard JH, Samady H, Bennett MR. Impact of combined plaque structural stress and wall shear stress on coronary plaque progression, regression, and changes in composition. Eur Heart J 2019;40:1411-22. [Crossref] [PubMed]
- Samady H, Eshtehardi P, McDaniel MC, Suo J, Dhawan SS, Maynard C, Timmins LH, Quyyumi AA, Giddens DP. Coronary artery wall shear stress is associated with progression and transformation of atherosclerotic plaque and arterial remodeling in patients with coronary artery disease. Circulation 2011;124:779-88. [Crossref] [PubMed]
- Wahle A, Prause PM, DeJong SC, Sonka M. Geometrically correct 3-D reconstruction of intravascular ultrasound images by fusion with biplane angiography--methods and validation. IEEE Trans Med Imaging 1999;18:686-99. [Crossref] [PubMed]
- Chatzizisis YS, Toutouzas K, Giannopoulos AA, Riga M, Antoniadis AP, Fujinom Y, Mitsouras D, Koutkias VG, Cheimariotis G, Doulaverakis C, Tsampoulatidis I, Chouvarda I, Kompatsiaris I, Nakamura S, Rybicki FJ, Maglaveras N, Tousoulis D, Giannoglou GD. Association of global and local low endothelial shear stress with high-risk plaque using intracoronary 3D optical coherence tomography: Introduction of 'shear stress score'. Eur Heart J Cardiovasc Imaging 2017;18:888-97. [Crossref] [PubMed]
- Lu J, Yu J, Shi H. Feasibility Study of Computational Fluid Dynamics Simulation of Coronary Computed Tomography Angiography Based on Dual-Source Computed Tomography. J Clin Med Res 2017;9:40-5. [Crossref] [PubMed]
- Park JB, Choi G, Chun EJ, Kim HJ, Park J, Jung JH, Lee MH, Otake H, Doh JH, Nam CW, Shin ES, De Bruyne B, Taylor CA, Koo BK. Computational fluid dynamic measures of wall shear stress are related to coronary lesion characteristics. Heart 2016;102:1655-61. [Crossref] [PubMed]
- Blanco PJ, de Queiroz RA, Feijóo RA. A computational approach to generate concurrent arterial networks in vascular territories. Int J Numer Method Biomed Eng 2013;29:601-14. [Crossref] [PubMed]
- Taylor CA, Fonte TA, Min JK. Computational fluid dynamics applied to cardiac computed tomography for noninvasive quantification of fractional flow reserve: scientific basis. J Am Coll Cardiol 2013;61:2233-41. [Crossref] [PubMed]
- Müller LO, Fossan FE, Bråten AT, Jørgensen A, Wiseth R, Hellevik LR. Impact of baseline coronary flow and its distribution on fractional flow reserve prediction. Int J Numer Method Biomed Eng 2021;37:e3246. [Crossref] [PubMed]
- Bulant CA, Blanco PJ, Maso Talou GD, Bezerra CG, Lemos PA, Feijóo RA. A head-to-head comparison between CT- and IVUS-derived coronary blood flow models. J Biomech 2017;51:65-76. [Crossref] [PubMed]
- Di Jiang M, Zhang XL, Liu H, Tang CX, Li JH, Wang YN, et al. The effect of coronary calcification on diagnostic performance of machine learning-based CT-FFR: a Chinese multicenter study. Eur Radiol 2021;31:1482-93. [Crossref] [PubMed]
- Gauss S, Achenbach S, Pflederer T, Schuhbäck A, Daniel WG, Marwan M. Assessment of coronary artery remodelling by dual-source CT: a head-to-head comparison with intravascular ultrasound. Heart 2011;97:991-7. [Crossref] [PubMed]
- Doh JH, Koo BK, Nam CW, Kim JH, Min JK, Nakazato R, Silalahi T, Prawira H, Choi H, Lee SY, Namgung J, Kwon SU, Kwak JJ, Lee WR. Diagnostic value of coronary CT angiography in comparison with invasive coronary angiography and intravascular ultrasound in patients with intermediate coronary artery stenosis: results from the prospective multicentre FIGURE-OUT (Functional Imaging criteria for GUiding REview of invasive coronary angiOgraphy, intravascular Ultrasound, and coronary computed Tomographic angiography) study. Eur Heart J Cardiovasc Imaging 2014;15:870-7. [Crossref] [PubMed]
- Blanco PJ, Bulant CA, Bezerra CG, Maso Talou GD, Pinton FA, Ziemer PGP, Feijóo RA, García-García HM, Lemos PA. Coronary arterial geometry: A comprehensive comparison of two imaging modalities. Int J Numer Method Biomed Eng 2021;37:e3442. [Crossref] [PubMed]
- Tsompou PI, Siogkas PK, Sakellarios AI, Andrikos IO, Kigka VI, Lemos PA, Michalis LK, Fotiadis DI. A comparison of three multimodality coronary 3D reconstruction methods. Annu Int Conf IEEE Eng Med Biol Soc 2019;2019:5812-5. [Crossref] [PubMed]
- Han D, Lin A, Gransar H, Dey D, Berman DS. Influence of Coronary Artery Calcium Score on Computed Tomography-Derived Fractional Flow Reserve: A Meta-Analysis. JACC Cardiovasc Imaging 2021;14:702-3. [Crossref] [PubMed]
- Tesche C, Otani K, De Cecco CN, Coenen A, De Geer J, Kruk M, Kim YH, Albrecht MH, Baumann S, Renker M, Bayer RR, Duguay TM, Litwin SE, Varga-Szemes A, Steinberg DH, Yang DH, Kepka C, Persson A, Nieman K, Schoepf UJ. Influence of Coronary Calcium on Diagnostic Performance of Machine Learning CT-FFR: Results From MACHINE Registry. JACC Cardiovasc Imaging 2020;13:760-70. [Crossref] [PubMed]
- Zhao N, Gao Y, Xu B, Yang W, Song L, Jiang T, Xu L, Hu H, Li L, Chen W, Li D, Zhang F, Fan L, Lu B. Effect of Coronary Calcification Severity on Measurements and Diagnostic Performance of CT-FFR With Computational Fluid Dynamics: Results From CT-FFR CHINA Trial. Front Cardiovasc Med 2022;8:810625. [Crossref] [PubMed]
- Lee JM, Choi G, Koo BK, Hwang D, Park J, Zhang J, et al. Identification of High-Risk Plaques Destined to Cause Acute Coronary Syndrome Using Coronary Computed Tomographic Angiography and Computational Fluid Dynamics. JACC Cardiovasc Imaging 2019;12:1032-43. [Crossref] [PubMed]
- Yang S, Choi G, Zhang J, Lee JM, Hwang D, Doh JH, Nam CW, Shin ES, Cho YS, Choi SY, Chun EJ, Nørgaard BL, Nieman K, Otake H, Penicka M, Bruyne B, Kubo T, Akasaka T, Taylor CA, Koo BK. Association Among Local Hemodynamic Parameters Derived From CT Angiography and Their Comparable Implications in Development of Acute Coronary Syndrome. Front Cardiovasc Med 2021;8:713835. [Crossref] [PubMed]