Dark blood T2-weighted imaging of the human heart with AI-assisted compressed sensing: a patient cohort study
Introduction
Dark blood T2-weighted (DB-T2W) imaging with fat saturation is routinely used for cardiac morphological evaluation, which is valuable for the diagnosis of myocardial edema (1-3). The 2-dimensional (2D) dual inversion recovery fast spin echo sequence is conventionally used for cardiac DB-T2W morphological imaging (4), which acquires k-space data in multi-shot acquisition across multiple cardiac cycles. However, this technique is sensitive to arrhythmia, tachycardia, and cardiac and respiratory motion, which can lead to myocardial signal loss, reduced contrast, and motion artifacts (5). To resolve these limitations, parallel imaging (PI) can be used to decrease scanning time to 11 heartbeats per slice or improve the spatial resolution with high acceleration factors (6-8); however, this can be degraded by noise amplification and undersampling artifacts and leads to lower image quality and a decreased signal-to-noise ratio (SNR) (9).
Alternatively, compressed sensing (CS) facilitates rapid acquisition without perceptible deterioration of the SNR by performing a nonlinear iterative reconstruction (10-12). This has been used in a wide variety of biomedical research and clinical applications, including DB-T2W imaging (13). In the reconstruction, image information is recovered from undersampled k-space data by exploiting the sparsity in an appropriate transform domain. Combined with the techniques of PI and half Fourier (14-16), CS reconstruction can even achieve higher acceleration with a shorter scan time. However, insufficient sparseness may lead to noise-like aliasing artifacts with a very high acceleration factor.
In recent years, a convolutional neural network has been widely used in the field of artificial intelligence (AI) to reconstruct images with high quality, especially at a highly accelerated condition (17-21). Subsequently, a novel magnetic resonance (MR) acceleration solution, called AI-assisted compressed sensing (ACS) (22), was proposed to further leverage the individual benefits of AI and CS and was approved by the US Food and Drug Administration (FDA) (23). The main architecture of ACS reconstruction is an integration of half Fourier, PI, CS, and AI in an iterative reconstruction procedure and has been validated in numerous applications, such as liver imaging (24) and kidney imaging (25). The feasibility of the DB-T2W imaging with the ACS method has also been reported to validate in previous abstracts (26-28). However, the effect of DB-T2W imaging with ACS remains to be explored.
In this study, we evaluated the effects of ACS on the image quality of single-shot (SS) and multi-shot (MS) DB-T2W imaging of edema by comparing it with conventional MS imaging with PI.
Theory
ACS reconstruction
The main architecture of ACS reconstruction is an integration of half Fourier, PI, CS, and AI in an iterative reconstruction procedure. The framework of this reconstruction is shown in Figure 1. Specifically, the AI module (Figure 1A) is trained using the ResNet network as reported previously (24), which can solve the problem of gradient disappearance while improving the performance and convergence of the network. The outputs of the AI module are then added into the iterative reconstruction as a new regularization term. Since its output is integrated into the iterative procedure, the uncertainty of the AI module is constrained by both mathematical constraints from the half Fourier, PI, and CS methods, as well as data consistency constraints from the original undersampled k-space. A diagram of the inline reconstruction is displayed in Figure 1B. Therefore, the ACS is defined as follows:
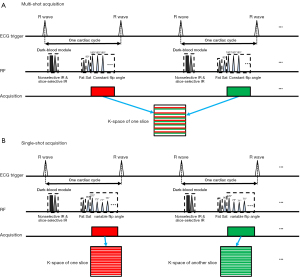
x denotes the image to be reconstructed, E denotes the production of Fourier encoding with a binary k-space sampling mask, y represents the acquired k-space data, denotes the sparse transform (e.g., wavelet or total variation), and xAI denotes the reconstructed image of the trained AI module, with the under-sampled k-space as input.
Methods
DB-T2W sequence with MS acquisition
As shown in Figure 1A, a dual inversion recovery with MS fast spin echo readout acquisition (4) was implemented with ACS reconstruction to speed up the examination. An echo train length of 25 was applied in MS acquisition with ACS (MS-ACS) and with PI (MS-PI), but the MS-ACS method reduced the breath-holding time of 4 heartbeats for each slice due to the higher acceleration of ACS.
DB-T2W sequence with SS acquisition
As shown in Figure 1B, SS acquisition with the ACS method (SS-ACS) was designed to shorten the scan time of DB-T2W imaging to acquire full left ventricle (LV) coverage in a single breath-hold. In this method, a total of 49 phase encoding lines were acquired for each slice. To suppress the myocardial inhomogeneity and blurring due to the long acquisition, a variable flip angle strategy for refocusing pulses was designed as described previously (29) and was optimized according to the myocardial T1 and T2 relaxation times (T1 =1,200 ms and T2 =40 ms). Additionally, 1 R-R interval was skipped between each slice to allow for signal recovery between the inversion pulses.
Patient population
This study was conducted in accordance with the Declaration of Helsinki (as revised in 2013) and was approved by the institutional ethics board of Tongji Hospital, Tongji Medical College, Huazhong University of Science and Technology. Informed consent was obtained from all patients. From August to December 2021, 33 patients with clinical indications including cardiomyopathy, cardiac valve disease, myocarditis, and other cardiovascular diseases were prospectively recruited.
Image acquisition
All cardiac MR (CMR) scans were performed on a 3T scanner (uMR 790, United Imaging Healthcare, Shanghai, China) with a dedicated 24-channel cardiac coil. Before the scan, all patients were told to hold their breath after exhalation. The MS-PI protocol was performed before the other protocols, and the proposed MS-ACS and SS-ACS protocols were subsequently carried out. Nine short-axis slices were acquired for each protocol to cover the entire left ventricle (LV) from the mitral valve to the LV apex. The scanning parameters for all protocols are listed in Table 1.
Table 1
Scanning parameters | MS-PI | MS-ACS | SS-ACS |
---|---|---|---|
FOV, mm2 | 400×320 | 400×320 | 400×320 |
Voxel size, mm2 | 1.95×1.56 | 1.95×1.56 | 1.95×1.56 |
Slice thickness, mm | 8.0 | 8.0 | 8.0 |
TE, ms | 69.68 | 63.44 | 82.8 |
Refocus flip angle | 180° | 180° | Variable (70°~160°) |
Acceleration method | PI | ACS | ACS |
Acceleration factor | 2 | 3 | 3 |
Shot number | Multi-shot | Multi-shot | Single-shot |
Acquisition slices | 9 | 9 | 9 |
Scan time per slice | 11 heartbeats | 7 heartbeats | – |
Total scan time | 9×11 heartbeats | 9×7 heartbeats | 19 heartbeats |
Breath-hold, number | 9 | 9 | 1 |
CMR, cardiac magnetic resonance; MS-PI, multi-shot acquisition with parallel imaging; MS-ACS, multi-shot acquisition with artificial intelligence-assisted compressed sensing; SS-ACS, single-shot acquisition with artificial intelligence-assisted compressed sensing; FOV, field of view; TE, echo time; PI, parallel imaging; ACS, artificial intelligence-assisted compressed sensing.
In addition, patients with heart failure were additionally distinguished to validate the feasibility of these protocols in patients that failed to perform the long breath-holding. Therefore, cardiac function was measured from images acquired from the continuous short-axial balanced steady-state free precession pulse (bSSFP) sequence (30) (echo time =1.32 ms, repetition time =2.84 ms, flip angle =55°, matrix =208×208, field of view =320×320). Patients with an LV ejection fraction (LVEF) <50% (31) and the clinical indication of heart failure were considered and included in the heart failure cohort.
Image analysis
The myocardium and blood pool regions of interest (ROIs) in the MS-PI, MS-ACS, and SS-ACS were contoured on a workstation by 2 CMR technicians (X.Y. and Y.L.). The myocardial signal intensity (SI) was measured at the septum. Blood signals were measured at the center of the blood pool in the left ventricle away from the papillary muscles and chordae tendineae. SI was obtained using the mean signal of the ROI, and noise was obtained by the standard deviation (SD) of the myocardium or blood pool.
For patients with myocardial edema, the edematous region areas were evaluated using commercial software cvi42, v. 5.3 (Circle Cardiovascular Imaging, Calgary, AB, Canada) according to the so-called 2SD method (32). The LV endocardium and epicardium were delineated at all slices, and the remote normal myocardium ROI was selected to calculate the mean and SD values. Next, myocardium with a signal intensity >2 SD were automatically detected as an edematous region. The total LV mass and edema myocardium mass were calculated, and the extent of edema was defined by the ratio of edema mass and LV mass (%LVM).
In addition, the SNR, contrast ratio (CR), and contrast-to-noise ratio (CNR) were calculated to compare the images of the different protocols, and were defined as follows:
In the qualitative evaluation, the images were blindly reviewed by 3 radiologists (L.H., L.R., and Z.Y.), all of whom had more than 5 years of experience in cardiovascular imaging. The overall image quality, visual quality of blood nulling, free wall of the right ventricle (RV), free wall of the LV, and the interventricular septum were evaluated using a 5-point ordinal Likert scale (33) as follows: 1, nondiagnostic; 2, poor; 3, fair; 4, good; and 5, excellent. In addition, motion-related artifacts and signal dropout in the LV free wall were evaluated in the heart failure cohort.
Statistical analysis
Statistical analysis was performed using SPSS (version 23.0; IBM Corp., Armonk, NY, USA). Continuous data are described as the mean ± SD or median and interquartile range, and categorical data are presented as the frequency and percentage. Normality was evaluated using the Kolmogorov-Smirnov test, and homogeneity of variance was assessed using Levene test. Before analysis, the ordinal Likert scores from the 3 radiologists and quantitative parameters calculated by 2 observers were averaged. Analysis of variance or the Friedman test was used to assess the differences between the ordinal Likert scores and quantitative parameters among MS-PI, MS-ACS, and SS-ACS. The differences in qualitative scores and quantitative values between the 3 protocols pairwise were respectively evaluated by paired t-tests or paired Wilcoxon signed-rank test. Kendall W was used to assess the interobserver agreement of the scoring data. The intraclass correlation coefficient (ICC) was used to assess the interobserver agreement between SNRmyocardium, CRblood,myocardium, CNRblood,myocardium, CRedema,myocardium, and CNRedema,myocardium. The agreement between the edema extents obtained from MS-PI, MS-ACS, and SS-ACS was compared using Bland-Altman analysis. P<0.05 was considered statistically significant.
Results
All 33 patients successfully underwent the scans with both the conventional approach and the 2 proposed protocols. Among these, 9 patients (8 males 89%; aged 45.7±14.0 years) were classified as patients with heart failure (LVEF 23.7%±8.9%) and added into the heart failure cohort, including 8 (89%) cases of dilated cardiomyopathy and 1 (11%) case of hypertension heart disease. Moreover, 11 (33%) patients had myocardial edema, including myocardial infarction (n=5), hypertrophic cardiomyopathy (n=4), and myocarditis (n=2). The characteristics of the included patients are listed in Table 2.
Table 2
Clinical characteristics | Value |
---|---|
Sex, male | 26 (79%) |
Age, years | 40.5±14.8 |
Height, cm | 169.7±7.3 |
Weight, kg | 73.8±15.4 |
BSA, m2 | 1.83±0.22 |
Heart rate, bpm | 69.6±13.9 |
Clinical diagnosis | |
Pulmonary hypertension | 1 |
Cardiomyopathy | 15 |
Hypertensive heart disease | 2 |
Myocardial infarction | 5 |
Valvular heart diseases | 2 |
Myocarditis | 2 |
Other diseases | 6 |
The results are shown as the number (%) or mean ± SD, as specified. Continuous data presented as mean ± SD. BSA, body surface area; SD, standard deviation.
The acquisition time per slice of MS-ACS was shorter than that of MS-PI (6.1±1.0 vs. 9.7±1.7 s; P<0.001). In addition, the entire acquisition time of SS-ACS was only about 17 s in a single breath-hold, while the other approaches were longer than 55 s with 9 breath-holds. In the 9 patients with heart failure, the breath-holding time for 1 slice was significantly shortened using MS-ACS compared to MS-PI (5.5±1.0 vs. 8.8±1.5 s; P<0.001), and the total acquisition time for 9 slices was shortened by 36% (50.0±9.1 vs. 79.6±13.5 s; P<0.001).
The quantitative and qualitative results are listed in Table 3. The SNRmyocardium, CRblood,myocardium, and CNRblood,myocardium of SS-ACS were significantly higher than those of MS-PI (all P values <0.001) and MS-ACS (all P values ˂0.05). In addition, the SNRmyocardium and CNRblood,myocardium of MS-ACS were statistically significant higher than those of MS-PI (both P values <0.001), whereas the CRblood,myocardium was not (P=0.143). The heart failure cohort exhibited better SNRmyocardium (67.6±33.3 vs. 50.0±19.0; P=0.038) and CNRblood,myocardium (77.9±29.2 vs. 47.8±19.0; P=0.038) with MS-ACS compared to with MS-PI. Overall image quality, blood nulling, LV free wall visibility, and septum visibility showed significant differences between the MS-PI, MS-ACS, and SS-ACS protocols (all P values ˂0.05), while RV wall visibility did not (P=0.399). Moreover, overall image quality and LV free wall visibility in all protocols using ACS reconstruction were significantly higher than those using PI reconstruction. In addition, the blood nulling and septum visibility of SS-ACS were significantly improved compared with those of MS-PI (both P values <0.001). None of the ordinal Likert scores were significantly different between the 2 ACS methods (all P values ˃0.05). The scores of the 3 cardiovascular radiologists showed good interobserver agreement (all P values <0.05), as shown in Table 4, and the results of SNRmyocardium, CRblood,myocardium, and CNRblood,myocardium measured by the 2 radiographers were also in a good agreement (all P values <0.05).
Table 3
Quantitative parameters/qualitative scores | MS-PI | MS-ACS | SS-ACS | P value# |
---|---|---|---|---|
Total acquisition time, s | 87.5±16.3 | 55.2±9.2* | 17.0±3.1*/& | <0.001 |
SNRmyocardium | 65.2±28.1 | 80.7±30.6* | 109.9±37.5*/& | <0.001 |
CRblood,myocardium | 14.7±5.8 | 15.6±5.0 | 18.0±5.7*/& | 0.043 |
CNRblood,myocardium | 62.8±27.9 | 77.9±30.2 * | 95.7±32.8*/& | <0.001 |
CRedema,myocardium† | 1.40±0.28 | 1.37±0.27 | 1.33±0.23 | 0.833 |
CNRedema,myocardium‡ | 20.0±17.5 | 27.3±24.2 | 29.7±29.7 | 0.624 |
Overall image quality | 3.9±0.6 | 4.1±0.4* | 4.0±0.4* | 0.011 |
Blood nulling | 4.6±0.6 | 4.7±0.5 | 4.8±0.5* | <0.001 |
RV wall visibility | 3.8±0.5 | 3.9±0.5 | 3.8±0.6 | 0.399 |
LV wall visibility | 3.9±0.6 | 4.4±0.5* | 4.3±0.5* | <0.001 |
Septum visibility | 4.4±0.6 | 4.5±0.5 | 4.6±0.5* | 0.034 |
Data are presented as the mean ± SD. †, CRedema,myocardium was counted across 11 patients with myocardial edema; ‡, CNRedema,myocardium was counted across 11 patients with myocardial edema; #, P value was calculated by analysis of variance or the Friedman test among the 3 DB-T2W protocols; *, P<0.05 of the MS-ACS or SS-ACS methods, as compared to the MS-PI method; &, P<0.05 between the MS-ACS and SS-ACS methods. MS-PI, multi-shot acquisition with parallel imaging; MS-ACS, multi-shot acquisition with artificial intelligence-assisted compressed sensing; SS-ACS, single-shot acquisition with artificial intelligence-assisted compressed sensing; SNR, signal-to-noise ratio; CNR, contrast-to-noise ratio; CR, contrast ratio; RV, right ventricle; LV, left ventricle.
Table 4
Qualitative scores/quantitative parameters | MS-PI | MS-ACS | SS-ACS |
---|---|---|---|
Overall image quality | 0.735 | 0.767 | 0.614 |
Blood nulling | 0.718 | 0.715 | 0.729 |
RV wall visibility | 0.639 | 0.637 | 0.708 |
LV wall visibility | 0.668 | 0.762 | 0.659 |
Septum visibility | 0.669 | 0.693 | 0.738 |
SNRmyocardium | 0.773 | 0.771 | 0.763 |
CRblood,myocardium | 0.924 | 0.812 | 0.946 |
CNRblood,myocardium | 0.807 | 0.783 | 0.781 |
CRedema,myocardium† | 0.927 | 0.960 | 0.961 |
CNRedema,myocardium‡ | 0.816 | 0.833 | 0.883 |
†, CRedema,myocardium was counted across 11 patients with myocardial edema; ‡, CNRedema,myocardium was counted across 11 patients with myocardial edema. MS-PI, multi-shot acquisition with parallel imaging; MS-ACS, multi-shot acquisition with artificial intelligence-assisted compressed sensing; SS-ACS, single-shot acquisition with artificial intelligence-assisted compressed sensing; SNR, signal-to-noise ratio; CNR, contrast-to-noise ratio; CR, contrast ratio; RV, right ventricle; LV, left ventricle.
In the heart failure cohort, the images from a total of 4 (44%) patients showed obvious artifacts under MS-PI, while no artifact was seen on the MS-ACS images. Figure 2 illustrates an example of artifact comparison from the heart failure cohort in which an obvious artifact was observed on the MS-PI image, whereas no artifact was seen on the MS-ACS image.
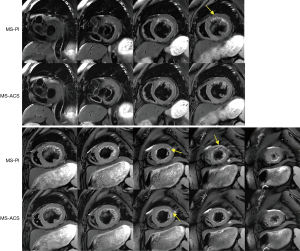
A comparison of the MS-PI and SS-ACS images is shown in Figure 3, in which multiple breath-holds were performed during the MS acquisition and a single breath-hold was performed in the SS acquisition. Fewer motion-related artifacts in the myocardium and a better dark blood effect were observed in the SS-ACS images, especially at slices adjacent to the apex.
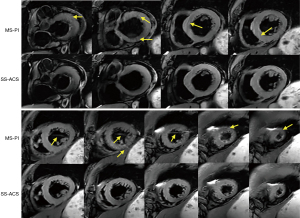
Figure 4 displays the edema comparison of MS-PI and SS-ACS in a patient in whom edema was shown with a similar location and size under both sequences. The Bland-Altman plots of edema extent among the different techniques are shown in Figure 5. The edema extents of SS-ACS was in good agreement with that of MS-PI (bias 0.35%; 95% confidence interval: −9.7% to 10.4%), while those of SS-ACS and MS-PI exhibited no significant difference (P=0.859). Furthermore, the edema extent of MS-ACS showed good agreement with that of MS-PI (bias −0.03%, 95% confidence interval: −6.8% to 6.8%), while those of MS-ACS and MS-PI displayed no significant difference (P=0.979).
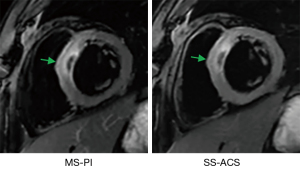
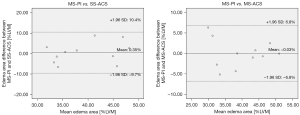
Discussion
In this study, an ACS technique was implemented to accelerate DB-T2W myocardial imaging. Two acquisition modes of ACS, MS-ACS and SS-ACS, were used to compare to conventional DB-T2W imaging with the PI method. The breath-holding time of MS-ACS method was 4 heartbeats shorter than that of MS-PI method for each slice, and 36 heartbeats of scan time could be reduced with the acquisition of 9 slices. The total scan time of SS-ACS was reduced to 17 s and the acquisition could be implemented within a single breath-hold, while the total scan time of the MS acquisition was longer than 55 s for 9 slices, and multiple breath-holds were needed during the acquisition. Meanwhile, the image quality of DB-T2W imaging with ACS was comparable or even superior to that using the PI method.
Fast spin echo-based DB-T2W imaging is routinely used for cardiac morphological evaluation but is severely impaired by signal loss due to the long echo train length (4). Alternatively, T2-prepared SSFP approaches have developed rapidly without the limitation of signal loss due to the fast acquisition of gradient echo (34,35). However, the signal of SSFP is proportional to (36), which reduces the signal change of different tissue. Moreover, the SSFP is sensitive to field inhomogeneity (37), which may lead to a banding artifact, especially at higher magnetic fields. To some extent, the fast spin echo-based methods are preferable for DB-T2W imaging because they are insensitive to field inhomogeneity and have higher SNRs and CNRs (5). In addition, the signal loss caused by the long echo train length of the fast spin echo could be largely eliminated with the integration of the ACS technique for its super-fast acquisition.
The ACS technique in this application uses the advantages of AI and CS reconstruction. To guarantee generalizability, the network was trained by millions of fully sampled bits of data, including various image indications, scan conditions, body habiti, and anatomies from phantoms and volunteers in a clinical setting (24). In addition, to guarantee the reliability of the reconstruction, the output of the network was regarded as a reference image of the additional regularization term in the CS reconstruction (38). Previous studies reported that the ACS in DB-T2W imaging significantly shortened the breath-holding time of the patients and improved the image quality by reducing motion artifacts for patients (26-28). In this study, it took 7 heartbeats per slice using the MS-ACS approach and 11 heartbeats per slice using MS-PI protocol, which improved patient cooperation and thus reduced respiratory motion artifacts and avoided myocardial signal loss. In the heart failure cohort, the MS-ACS also took 7 heartbeats per slice, which shortened the acquisition time.
Additionally, the application of ACS makes it possible to acquire whole-heart images during a single breath hold in DB-T2W imaging by cooperating with an SS acquisition, which shortens the scan time considerably, simplifies the scanning workflow, and lessens the breath-holding burden of patients. Moreover, the SS-ACS solution could substantially reduce the motion artifacts induced by variant R-R intervals through SS acquisition with 1 slice per cardiac cycle. In other words, respiratory-triggered free-breathing acquisition is an alternate method of obtaining DB-T2W imaging for patients who cannot tolerate the breath-hold.
Limitations
In this study, MS DB-T2W imaging with PI was considered a reference for technique comparison because it is hard to collect fully sampled cardiac imaging data as a gold standard. Moreover, the current study lacked technical comparison with other CS or AI methods. Although a total of 33 cardiovascular patients is sufficient to validate the proposed methods, a larger sample size of different cardiac diseases is warranted for the statistical comparisons of various cardiac diseases. In addition, validation across multiple sites is also warranted to eliminate the potential selection bias. Although the SS acquisition was applied to minimize the effects of cardiac motion due to the variant R-R intervals that occur in the MS acquisition, the image quality of the DB-T2W imaging using the ACS method could have been impaired by the serious arrhythmias or extremely high heart rates of the patients.
Conclusions
The ACS method for DB-T2W imaging significantly reduced the acquisition time of the MS scheme to 7 heartbeats per slice and of the SS scheme to 19 heartbeats for 9 slices. It further provided better image quality compared to the conventional PI method. Moreover, DB-T2W imaging was implemented to cover the whole heart with 9 slices in a single breath-hold via the application of SS acquisition combined with ACS, which could help to reduce the breath-holding burden of patients and improve diagnostic efficiency.
Acknowledgments
Funding: This work was partially supported by the National Natural Science Foundation of China (No. 81873889) and the Youth Fund of Hubei Science and Technology Plan (No. 2021CFB060).
Footnote
Conflicts of Interest: All authors have completed the ICMJE uniform disclosure form (available at https://qims.amegroups.com/article/view/10.21037/qims-22-607/coif). Shiyu Zhang and Shuheng Zhang are employed by United Imaging Healthcare. JX is employed by United Imaging Healthcare America, Inc. The other authors have no conflicts of interest to declare.
Ethical Statement: The authors are accountable for all aspects of the work in ensuring that questions related to the accuracy or integrity of any part of the work are appropriately investigated and resolved. The study was conducted in accordance with the Declaration of Helsinki (as revised in 2013) and was approved by the institutional ethics board of Tongji Hospital, Tongji Medical College, Huazhong University of Science and Technology. Informed consent was obtained from all of the patients.
Open Access Statement: This is an Open Access article distributed in accordance with the Creative Commons Attribution-NonCommercial-NoDerivs 4.0 International License (CC BY-NC-ND 4.0), which permits the non-commercial replication and distribution of the article with the strict proviso that no changes or edits are made and the original work is properly cited (including links to both the formal publication through the relevant DOI and the license). See: https://creativecommons.org/licenses/by-nc-nd/4.0/.
References
- Cocker MS, Shea SM, Strohm O, Green J, Abdel-Aty H, Friedrich MG. A new approach towards improved visualization of myocardial edema using T2-weighted imaging: a cardiovascular magnetic resonance (CMR) study. J Magn Reson Imaging 2011;34:286-92. [Crossref] [PubMed]
- Hu C, Huber S, Latif SR, Santacana-Laffitte G, Mojibian HR, Baldassarre LA, Peters DC. Reverse double inversion-recovery: Improving motion robustness of cardiac T(2) -weighted dark-blood turbo spin-echo sequence. J Magn Reson Imaging 2018;47:1498-508. [Crossref] [PubMed]
- Abdel-Aty H, Zagrosek A, Schulz-Menger J, Taylor AJ, Messroghli D, Kumar A, Gross M, Dietz R, Friedrich MG. Delayed enhancement and T2-weighted cardiovascular magnetic resonance imaging differentiate acute from chronic myocardial infarction. Circulation 2004;109:2411-6. [Crossref] [PubMed]
- Simonetti OP, Finn JP, White RD, Laub G, Henry DA. "Black blood" T2-weighted inversion-recovery MR imaging of the heart. Radiology 1996;199:49-57. [Crossref] [PubMed]
- Kellman P, Aletras AH, Mancini C, McVeigh ER, Arai AE. T2-prepared SSFP improves diagnostic confidence in edema imaging in acute myocardial infarction compared to turbo spin echo. Magn Reson Med 2007;57:891-7. [Crossref] [PubMed]
- Pruessmann KP, Weiger M, Scheidegger MB, Boesiger P. SENSE: sensitivity encoding for fast MRI. Magn Reson Med 1999;42:952-62. [Crossref] [PubMed]
- Griswold MA, Jakob PM, Heidemann RM, Nittka M, Jellus V, Wang J, Kiefer B, Haase A. Generalized autocalibrating partially parallel acquisitions (GRAPPA). Magn Reson Med 2002;47:1202-10. [Crossref] [PubMed]
- Tsao J. On the UNFOLD method. Magn Reson Med 2002;47:202-7. [Crossref] [PubMed]
- Deshmane A, Gulani V, Griswold MA, Seiberlich N. Parallel MR imaging. J Magn Reson Imaging 2012;36:55-72. [Crossref] [PubMed]
- Donoho DL. Compressed sensing. IEEE Trans Inform Theory 2006;52:1289-306. [Crossref]
- Lustig M, Donoho D, Pauly JM. Sparse MRI: The application of compressed sensing for rapid MR imaging. Magn Reson Med 2007;58:1182-95. [Crossref] [PubMed]
- Feng L, Benkert T, Block KT, Sodickson DK, Otazo R, Chandarana H. Compressed sensing for body MRI. J Magn Reson Imaging 2017;45:966-87. [Crossref] [PubMed]
- Yuda K, Yoshida T, Furukawa Y, Yoneyama M, Kwon J, Kawauchi N, Peeters JM, Van Cauteren M. Myocardial T2-weighted black-blood imaging with a deep learning constrained compressed SENSE reconstruction. Proceedings of the 29th Annual Meeting of ISMRM 2021:abstr 2667.
- Mann LW, Higgins DM, Peters CN, Cassidy S, Hodson KK, Coombs A, Taylor R, Hollingsworth KG, Accelerating MR. Imaging Liver Steatosis Measurement Using Combined Compressed Sensing and Parallel Imaging: A Quantitative Evaluation. Radiology 2016;278:247-56. [Crossref] [PubMed]
- Feng L, Xu J, Kim D, Axel L, Sodickson D, Otazo R. Combination of compressed sensing, parallel imaging and partial Fourier for highly-accelerated 3D first-pass cardiac perfusion MRI. Proceedings of the 19th Annual Meeting of ISMRM 2011:abstr 4368.
- Otazo R, Kim D, Axel L, Sodickson DK. Combination of compressed sensing and parallel imaging for highly accelerated first-pass cardiac perfusion MRI. Magn Reson Med 2010;64:767-76. [Crossref] [PubMed]
- Pezzotti N, de Weerdt E, Yousefi S, Elmahdy MS, van Gemert J, Schülke C, Doneva M, Nielsen T, Kastryulin S, Lelieveldt BP, van Osch MJ, Staring M. Adaptive-CS-Net: FastMRI with adaptive intelligence. arXiv 2019. arXiv:1912.12259.
- Wang Y, Zhang Y, Wen Z, Tian B, Kao E, Liu X, Xuan W, Ordovas K, Saloner D, Liu J. Deep learning based fully automatic segmentation of the left ventricular endocardium and epicardium from cardiac cine MRI. Quant Imaging Med Surg 2021;11:1600-12. [Crossref] [PubMed]
- Chew PG, Swoboda PP, Ferguson C, Garg P, Cook AL, Ibeggazene S, Brown LAE, Craven TP, Foley JR, Fent GJ, Saunderson CE, Higgins DM, Plein S, Birch KM, Greenwood JP. Feasibility and reproducibility of a cardiovascular magnetic resonance free-breathing, multi-shot, navigated image acquisition technique for ventricular volume quantification during continuous exercise. Quant Imaging Med Surg 2020;10:1837-51. [Crossref] [PubMed]
- Chen EZ, Wang P, Chen X, Chen T, Sun S. Pyramid Convolutional RNN for MRI Image Reconstruction. IEEE Trans Med Imaging 2022;41:2033-47. [Crossref] [PubMed]
- Wang S, Cao G, Wang Y, Liao S, Wang Q, Shi J, Li C, Shen D. Review and prospect: artificial intelligence in advanced medical imaging. Front. Radiol 2021;1:781868. [Crossref]
- Zhai R, Huang X, Zhao Y, Ji M, Lv X, Qian M, Liao S, Li G. Intelligent incorporation of AI with model constraints for MRI acceleration. Proceedings of the 29th Annual Meeting of ISMRM 2021:abstr 1760.
- Sheng RF, Zheng LY, Jin KP, Sun W, Liao S, Zeng MS, Dai YM. Single-breath-hold T2WI liver MRI with deep learning-based reconstruction: A clinical feasibility study in comparison to conventional multi-breath-hold T2WI liver MRI. Magn Reson Imaging 2021;81:75-81. [Crossref] [PubMed]
- Zhao Y, Peng C, Wang S, Liang X, Meng X. The feasibility investigation of AI -assisted compressed sensing in kidney MR imaging: an ultra-fast T2WI imaging technology. BMC Med Imaging 2022;22:119. [Crossref] [PubMed]
- U.S. Food and Drug Administration (FDA). uMR 780. Available online: https://www.accessdata.fda.gov/cdrh_docs/pdf19/K193176.pdf
- Ran L, Huang L, Yan X, Luo Y, Zhang S, Zhang S, Zheng Y, Xu J, Xia L. Clinical assessment of high spatial resolution myocardial T2-weighted dark blood imaging based on deep learning. Proceedings of the 30th Annual Meeting of ISMRM 2022:abstr 6613.
- Yan X, Huang L, Ran L, Luo Y, Zhang S, Bao Y, Zhang S,Ye Y, Xu J, Xia L. Deep learning based whole heart T2-weighted dark blood imaging in a single breath hold. Proceedings of the 30th Annual Meeting of ISMRM 2022:abstr 6572.
- Luo Y, Huang L, Yan X, Ran L, Zhang S, Zhang S, Lv J, Xu Jian, Xia L. Improved image quality using deep learning based T2-weighted dark blood imaging on patients with heart failure. Proceedings of the 30th Annual Meeting of ISMRM 2022:abstr 6507.
- Busse RF, Hariharan H, Vu A, Brittain JH. Fast spin echo sequences with very long echo trains: design of variable refocusing flip angle schedules and generation of clinical T2 contrast. Magn Reson Med 2006;55:1030-7. [Crossref] [PubMed]
- Forbat SM, Sakrana MA, Darasz KH, El-Demerdash F, Underwood SR. Rapid assessment of left ventricular volume by short axis cine MRI. Br J Radiol 1996;69:221-5. [Crossref] [PubMed]
- Wilcox JE, Fang JC, Margulies KB, Mann DL. Heart Failure With Recovered Left Ventricular Ejection Fraction: JACC Scientific Expert Panel. J Am Coll Cardiol 2020;76:719-34. [Crossref] [PubMed]
- Zhu Y, Yang D, Zou L, Chen Y, Liu X, Chung YC T. (2)STIR preparation for single-shot cardiovascular magnetic resonance myocardial edema imaging. J Cardiovasc Magn Reson 2019;21:72. [Crossref] [PubMed]
- Edelman RR, Botelho M, Pursnani A, Giri S, Koktzoglou I. Improved dark blood imaging of the heart using radial balanced steady-state free precession. J Cardiovasc Magn Reson 2016;18:69. [Crossref] [PubMed]
- Liu CY, Bley TA, Wieben O, Brittain JH, Reeder SB. Flow-independent T(2)-prepared inversion recovery black-blood MR imaging. J Magn Reson Imaging 2010;31:248-54. [Crossref] [PubMed]
- Huang TY, Liu YJ, Stemmer A, Poncelet BP. T2 measurement of the human myocardium using a T2-prepared transient-state TrueFISP sequence. Magn Reson Med 2007;57:960-6. [Crossref] [PubMed]
- Huang TY, Huang IJ, Chen CY, Scheffler K, Chung HW, Cheng HC. Are TrueFISP images T2/T1-weighted? Magn Reson Med 2002;48:684-8. [Crossref] [PubMed]
- Nazir MS, Neji R, Speier P, Reid F, Stäb D, Schmidt M, Forman C, Razavi R, Plein S, Ismail TF, Chiribiri A, Roujol S. Simultaneous multi slice (SMS) balanced steady state free precession first-pass myocardial perfusion cardiovascular magnetic resonance with iterative reconstruction at 1.5 T. J Cardiovasc Magn Reson 2018;20:84. [Crossref] [PubMed]
- Wang S, Su Z, Ying L, Peng X, Zhu S, Liang F, Feng D, Liang D. Accelerating magnetic resonance imaging via deep learning. Proc IEEE Int Symp Biomed Imaging 2016;2016:514-7. [Crossref] [PubMed]