Metabolic phenotypes, serum tumor markers, and histopathological subtypes in predicting bone metastasis: analysis of 695 patients with lung cancer in China
Introduction
Lung cancer remains one of the most frequent malignancies and the leading cause of cancer-related deaths worldwide despite significant improvements in diagnosis and treatment strategies (1,2). In 2022, there were approximately 236,740 new lung cancer diagnoses and 130,180 lung cancer-related deaths in the United States (1), with corresponding numbers in China of 870,982 and 766,898, respectively (2). The high mortality rate is primarily due to the advanced stage of the lung cancer at the time of diagnosis, including the occurrence of multisite metastasis (e.g., lymph node metastasis, organ metastasis, and, most importantly, bone metastasis (BM)] (3,4). Clinically, approximately 20–30% of patients with non-small cell lung cancer (NSCLC) have BM at diagnosis, and a further 30–40% develop BM during the course of the disease (3,4). Patients with BM often have poor quality of life due to severe bone pain, hypercalcemia, and pathological fractures (5). Therefore, early diagnosis or prediction of the occurrence and development of BM can help clinicians initiate timely and appropriate treatment or preventive measures, thereby improving the quality of life of patients and possibly improving their prognosis.
Several clinical modalities have been used to evaluate BM in patients with lung cancer. Positron emission tomography/computed tomography (PET/CT), a molecular imaging technique with 18F-2-fluoro-2-deoxyglucose (FDG), has been widely used in the diagnosis and pretreatment staging of lung cancer (6-8). In terms of BM detection, the sensitivity of 18F-FDG PET/CT is higher than that of conventional CT and 99mTc-methylene diphosphonate (MDP) bone scans in both NSCLC (9) and small cell lung cancer (SCLC) (10). The maximum standard uptake value (SUVmax), a semiquantitative parameter on PET/CT, has been proven to be a promising metabolic indicator for predicting the risk of FDG-avid BM in various tumors (11,12). However, although the detection of BM by 18F-FDG PET/CT has high sensitivity, the development of BM is an ongoing process, and there is still no effective way to predict which patients with lung cancer are likely to develop BM based on imaging.
Carcinoembryonic antigen (CEA), an oncofetal protein, is often overexpressed in different types of carcinomas (e.g., lung cancer, colorectal cancer, gastric cancer, pancreatic cancer) (13-15). Serum CEA concentrations have been shown to be associated with the development of brain metastasis in patients with advanced NSCLC (16). In addition, studies assessing the use of the serum tumor markers carbohydrate antigen (CA) 50, CA125, squamous cell carcinoma-related antigen (SCCA), cytokeratin 19 fragment (CYFRA21-1), neuron-specific enolase (NSE), and ferritin to predict the prognosis of patients with lung cancer showed that high concentrations of these serum tumor markers at baseline were associated with poor prognosis (17,18). Moreover, other studies investigated correlations between serum tumor markers (CEA, CA125, CYFRA21-1, SCCA, and NSE) and histopathological subtypes of lung cancer and showed that tumor markers play an auxiliary role in the histological diagnosis of patients with NSCLC (19). The poor prognosis of patients with lung cancer has been shown to be associated with BM and the high concentrations of these serum tumor markers. However, whether the concentrations of serum tumor markers contribute to the occurrence of BM and how they are correlated remain poorly understood.
In the present study, we speculated that high concentrations of serum tumor markers and high metabolic activity of primary and/or metastatic lesions may be risk factors for BM in patients with lung cancer and that combining these factors would achieve a higher efficiency in predicting BM than would each factor alone. Thus, we investigated the associations between metabolic phenotypes, serum tumor markers, and histopathological subtypes in patients with and without BM, and established corresponding models to predict the possibility of BM in patients with lung cancer. We present the following article in accordance with the STARD reporting checklist (available at https://qims.amegroups.com/article/view/10.21037/qims-22-741/rc).
Methods
Patients
The study was performed in accordance with the International Guidelines for Human Research Protection of the Declaration of Helsinki (as revised in 2013) and the International Conference on Harmonization in Good Clinical Practical (ICH-GCP). This study was approved by the Institutional Review Board of Hwa Mei Hospital, University of Chinese Academy of Sciences (protocol No. YJ-NBEY-KY202108401). Because this study was a retrospective study, the need for written informed consent was waived.
In this study, we analyzed 1,104 consecutive patients who had been initially diagnosed as having lung cancer with 18F-FDG PET/CT at Hwa Mei Hospital, University of Chinese Academy of Sciences (Ningbo, China) between September 2019 and March 2022. To be eligible for inclusion in this study, patients had to meet the following 3 criteria: (I) histopathology confirmed lung cancer [e.g., SCLC, squamous cell carcinoma (SCC), adenocarcinoma (ADC), and not otherwise specified (NOS)]; (II) no treatment before 18F-FDG PET/CT scans; and (III) pretreatment detection of at least 1 of serum tumor markers CEA (normal ≤5.00 ng/mL), CA50 (normal <25.00 U/mL), CA125 (normal ≤16.00 U/mL), CA72-4 (normal <10.00 U/mL), NSE (normal <20.00 ng/mL), SCCA (normal <1.50 ng/mL), CYFRA21-1 (normal <3.30 ng/mL), or ferritin (normal 10.0–291.0 ng/mL). After application of the inclusion criteria, 695 patients were enrolled in this study (Figure 1). The clinical characteristics of the patients in the study are summarized in Table 1 and included age, sex, smoking status, clinical tumor-node-metastasis (TNM) stage, histopathological subtypes, and distant metastatic area. Never-smokers were strictly defined as patients who had smoked <100 cigarettes in their lifetime (20).
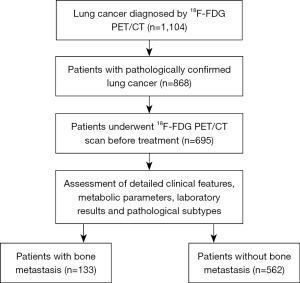
Table 1
Characteristics | Number |
---|---|
Age (years) | |
Mean ± SD | 66.39±9.11 |
Range | 29–86 |
Sex, n (%) | |
Male | 478 (68.8) |
Female | 217 (31.2) |
Smoking status, n (%) | |
Never smokers | 378 (54.4) |
Smokers | 317 (45.6) |
Clinical TNM stage, n (%) | |
I | 238 (34.24) |
II | 82 (11.80) |
III | 150 (21.58) |
IV | 225 (32.37) |
Histopathological subtype, n (%) | |
ADC | 416 (59.86) |
SCC | 188 (27.05) |
SCLC | 61 (8.78) |
NOS | 30 (4.32) |
Distant metastasis, n (%) | |
Bone | 133 (19.14) |
Lung | 54 (7.77) |
Liver | 45 (6.47) |
Brain | 26 (3.74) |
Adrenal | 39 (5.61) |
Others | 37 (5.32) |
SD, standard deviation; TNM, tumor-node-metastasis; ADC, adenocarcinoma; SCC, squamous cell carcinoma; SCLC, small cell lung cancer; NOS, not otherwise specified.
PET/CT scan technique
PET/CT scans were performed on a GE Discovery 710 PET scanner (GE Healthcare, Chicago, IL, USA). All patients fasted for ≥6 h before PET/CT examination. Blood glucose concentrations were tested and confirmed to be <7.0 mmol/L before intravenous injection of 5.2–7.4 MBq/kg of 18F-FDG, with the PET/CT scan performed 45–60 min after 18F-FDG administration. A low-dose CT scan was performed using the following parameters: 140 kV, 10 mA, 0.5 s rotation time, and 40 mm collimation. Then, a PET scan was conducted in 3-dimensional mode from the skull base to the upper thigh at 2.5 min per bed position, and the CT data of the iterative algorithm were used for reconstruction. PET, CT, and fusion PET/CT images in the transverse, sagittal, and coronal planes were obtained on the Xeleris Workstation (GE Healthcare) for evaluation.
Analysis of PET/CT imaging
All PET and CT images were evaluated consistently by 2 senior nuclear physicians (MJ and QG; both with >10 years experience) who were familiar with the clinical data. Abnormal 18F-FDG uptake within the lesion was defined as metabolic activity that was greater than that of the surrounding background; the uptake intensity of 18F-FDG was quantified by calculating the SUVmax. Two-dimensional regions of interest (ROIs) were manually drawn at the edges of the tumor lesions and placed in the region of the tumor with the highest 18F-FDG uptake. SUVmax is defined as the peak SUV on the pixel with the highest count in the ROI and can be calculated as follows:
Where RCROI is the concentration of radioactivity in the ROI (MBq/g), dose is the dose of 18F-FDG injected (MBq), and the BW is the patient’s total body weight (g). According to visual qualitative analysis, when the metabolic activity of the lymph nodes was higher than that of the background mediastinal blood pool, metastatic lymph nodes were considered to be present (21).
Evaluation of lung cancer with BM
All patients underwent baseline 18F-FDG PET/CT scans, and BMs were assessed. Bone lesions were classified into 3 groups: (I) obvious normal or benign; (II) obvious BM on PET and CT images; and (III) equivocal (i.e., PET and CT images could not be categorized definitively into the first 2 categories, requiring additional imaging procedures). In case of differences between the 2 nuclear physicians (MJ and QG), the following criteria were used to enable a consensus to be reached: (I) confirmation by histopathology; (II) confirmation by conventional anatomic imaging during follow-up (e.g., X-ray, CT, and magnetic resonance); (III) confirmation by follow-up PET/CT scans or whole-body bone scan on 99mTc-MDP single photon emission CT/CT; and (IV) classification of no BM for patients without evidence of BM during clinical or imaging feature-based follow-up.
Statistical analysis
Demographic patient data are presented using descriptive statistics. Clinical characteristics, including sex (male vs. female), smoking status (never-smokers vs. smokers), and histopathological subtypes (ADC, SCC, SCLC, and NOS), were compared between patients with and without BM using Fisher exact test and the chi-squared test. Quantitative data are presented as the mean ± SD. Serum concentrations of tumor markers are presented as the median with interquartile range (IQR). The significance of differences in continuous variables [e.g., SUVmax of the primary tumor (pSUVmax), lymph node (nSUVmax), and distant metastasis (mSUVmax)] and serum tumor marker concentration were compared between patients with and without BM using Mann-Whitney tests.
Receiver operating characteristic (ROC) curves were constructed using parameters or factors that were significantly different between patients with and without BM. The area under the ROC curve (AUC) was calculated to evaluate the predicted value for an established criterion. Multiple logistic regression analysis was used to establish a model to predict the risk of BM in patients with lung cancer. The Hosmer-Lemeshow test was used to evaluate the fitting effect of the model.
In all analyses, a 2-sided P value <0.05 was considered statistically significant. GraphPad Prism 9.0 (GraphPad Software, San Diego, CA, USA) was used for all statistical analyses and to draw graphs.
Results
Patient characteristics
Patient characteristics stratified according to the presence of BM are summarized in Table 2. All patients underwent baseline 18F-FDG PET/CT scans. Of the 695 patients in this study, 359 (51.65%) had lymph node metastasis and 218 (31.37%) had distant metastasis; 133 (19.14%) patients had BM and 562 (80.86%) did not. Of the 695 patients, 672 (96.69%) were tested for serum CEA, 668 (96.12%) were tested for ferritin and CA125, 607 (87.34%) were tested for CA50 and CA72-4, and 615 (88.49%) were tested for NSE, CYFRA21-1, and SCCA.
Table 2
Characteristics | With BM (n=133) | Without BM (n=562) | P value |
---|---|---|---|
Age (years) | 0.760 | ||
Mean ± SD | 66.17±9.57 | 66.44±9.01 | |
Range | 29–86 | 29–86 | |
Sex, n (%) | 0.999 | ||
Male | 92 (69.2) | 386 (68.7) | |
Female | 41 (30.8) | 176 (31.3) | |
Smoking status, n (%) | 0.772 | ||
Never smokers | 74 (55.6) | 304 (54.1) | |
Smokers | 59 (44.4) | 258 (45.9) | |
Serum tumor markers | |||
CEA (ng/mL) | 7.54 [2.05–56.72] | 2.60 [1.44–5.14] | <0.001 |
Ferritin (ng/mL) | 256.2 [170.7–456.4] | 199.6 [108.4–347.7] | <0.001 |
CA50 (U/mL) | 12.69 [6.82–30.04] | 9.22 [5.53–14.66] | <0.001 |
CA125 (U/mL) | 34.55 [12.70–108.9] | 10.70 [6.53–20.48] | <0.001 |
CA72-4 (U/mL) | 1.93 [1.00–4.57] | 1.86 [1.00–4.22] | 0.547 |
NSE (ng/mL) | 11.60 [8.69–21.94] | 8.27 [5.88–11.64] | <0.001 |
SCCA (ng/mL) | 0.62 [0.48–1.04] | 0.73 [0.47–1.34] | 0.151 |
CYFRA21-1 (ng/mL) | 5.23 [3.00–14.38] | 3.03 [1.87–5.12] | <0.001 |
Histopathological subtype, n (%) | <0.001 | ||
ADC | 93 (69.92) | 323 (57.47) | |
SCC | 13 (9.77) | 175 (31.14) | |
SCLC | 22 (16.54) | 39 (6.94) | |
NOS | 5 (3.76) | 25 (4.45) | |
Metabolic phenotype | |||
pSUVmax | 12.53 [8.42–15.23] | 10.30 [6.81–14.86] | 0.002 |
nSUVmax | 10.17 [7.51–13.98] | 8.74 [5.68–12.28] | 0.001 |
mSUVmax | 11.60 [8.00–14.77] | 6.86 [4.08–10.56] | <0.001 |
Lymph node metastasis, n (%) | <0.001 | ||
Yes | 121 (90.98) | 237 (42.17) | |
No | 12 (9.02) | 325 (57.83) | |
Lung metastasis, n (%) | <0.001 | ||
Yes | 34 (25.56) | 20 (3.56) | |
No | 99 (74.44) | 542 (96.44) | |
Liver metastasis, n (%) | <0.001 | ||
Yes | 35 (26.31) | 10 (17.80) | |
No | 98 (73.69) | 552 (82.20) | |
Brain metastasis, n (%) | <0.001 | ||
Yes | 17 (12.78) | 9 (1.60) | |
No | 116 (87.22) | 553 (98.40) | |
Adrenal metastasis, n (%) | <0.001 | ||
Yes | 27 (20.30) | 12 (2.14) | |
No | 106 (79.70) | 550 (97.86) |
Unless indicated otherwise, data are given as the median [interquartile range] or n (%). BM, bone metastasis; SD, standard deviation; CEA, carcinoembryonic antigen; CA, carbohydrate antigen; NSE, neuron-specific enolase; SCCA, squamous cell carcinoma-related antigen; CYFRA21-1, cytokeratin 19 fragment; ADC, adenocarcinoma; SCC, squamous cell carcinoma; SCLC, small cell lung cancer; NOS, not otherwise specified; pSUVmax, maximum standardized uptake value of primary tumor; nSUVmax, maximum standardized uptake value of metastatic lymph node; mSUVmax, maximum standardized uptake value of distant metastasis.
BM risk factors in patients with lung cancer
In terms of 18F-FDG metabolic activity, pSUVmax, nSUVmax, and mSUVmax were higher in patients with BM (12.63±5.28, 11.01±4.92, and 12.30±5.90, respectively) than in those without (11.13±6.52, 9.62±5.46 and 8.09±5.71, respectively; Figure 2). Moreover, the incidence rates of lymph node metastasis, lung metastasis, liver metastasis, brain metastasis, and adrenal metastasis were all significantly higher in patients with than without BM (Table 2).
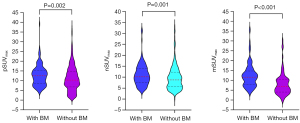
The median (IQR) concentrations of the 8 serum tumor markers (CEA, ferritin, CA50, CA125, CA72-4, NSE, SCCA, and CYFRA21-1) are presented in Table 2. Concentrations of CEA, ferritin, CA50, CA125, NSE, and CYFRA21-1 were significantly higher in patients with BM than without BM (all P values <0.001; Figure 3). There were no significant differences in serum CA72-4 and SCCA concentrations between patients with and without BM (P=0.547 and P=0.151, respectively).
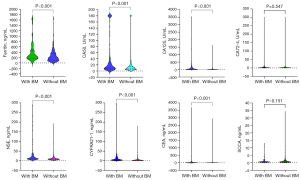
With regard to histopathological subtypes (Table 2), the incidence of ADC and SCLC was significantly higher among patients with BM (69.62% and 16.54%, respectively) than among those without BM (57.47% and 6.94%, respectively). However, the incidence of SCC was significantly lower among patients with than without BM (9.77% vs. 31.14%, respectively).
Prediction of BM in patients with lung cancer
Values of pSUVmax, nSUVmax, and mSUVmax were significantly correlated with BM in patients with lung cancer, with corresponding AUCs of 0.586, 0.603, and 0.741, respectively. In addition, the AUC of the combination of pSUVmax, nSUVmax, and mSUVmax was 0.737 (95% CI: 0.644–0.829), with a sensitivity of 76.77% and a specificity of 83.33% (Figure 4A).
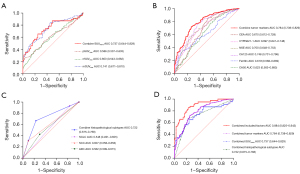
Of the serum tumor markers, high serum CEA, CA50, CA125, NSE, ferritin, and CYFRA21-1 concentrations were significantly correlated with BM in patients with lung cancer, with corresponding AUCs of 0.670, 0.623, 0.748, 0.700, 0.619, and 0.697, respectively. The combination of these 6 serum tumor markers resulted in a higher AUC (0.784; 95% CI: 0.738–0.829), with a sensitivity of 70.00% and a specificity of 82.81% (Figure 4B).
Histopathologically, a high incidence of ADC and SCLC and a low incidence of SCC were significantly correlated with BM in patients with lung cancer, with corresponding AUCs of 0.562, 0.548, and 0.607, respectively. The combination of these 3 factors resulted in an AUC of 0.722 (95% CI: 0.675–0.768) for predicting BM in patients with lung cancer (Figure 4C).
When all these factors (i.e., metabolic parameters, significant serum tumor markers, and histopathological subtypes) were combined, the AUC was significantly higher (0.884; 95% CI: 0.825–0.943; Figure 4D). Moreover, we established a model using multiple logistic regression to predict the risk of BM in patients with lung cancer as follows:
The sensitivity and specificity of this model were 87.72% and 72.97%, respectively. The Hosmer-Lemeshow P=0.239, indicating that the model fit well.
Representative images from patients with and without BM showing the association between 18F-FDG uptake and serum tumor markers are presented in Figure 5.
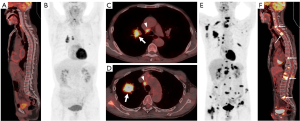
Discussion
We performed a retrospective analysis using 18F-FDG PET/CT, serum tumor markers, and histopathological subtypes to evaluate the risk factors for BM and predict BM in patients with lung cancer. Our results demonstrated that high pSUVmax, nSUVmax, and mSUVmax favor the presence of BM in patients with lung cancer and that serum tumor markers (CEA, CA50, CA125, NSE, ferritin, and CYFRA21-1) and histopathological subtypes (ADC, SCC, and SCLC) are important factors for predicting BM in these patients.
The TNM staging system plays a critical role in the choice of treatment strategy and prognosis evaluation for patients with lung cancer (22). BM indicates an advanced stage of the disease, and the quality of life and outcomes of these patients are significantly worse than for patients without BM (3,5,23,24). To date, the detection of BM in patients with lung cancer using anatomical and functional imaging modalities (e.g., 18F-FDG PET/CT) has been highly accurate (9,10). Studies have shown that BM can be identified at the time of initial diagnosis in 20–30% of patients, but up to 30–40% of patients develop BM in the subsequent disease course (3-5). Thus, it is of considerable importance to identify patients who are likely to develop BM so that clinicians can initiate timely, preventive measures. Lung cancer patients with high metabolic activity on PET/CT tend to have poor survival (25-28). Therefore, in this study, we compared differences in metabolic parameters (e.g., pSUVmax, nSUVmax, and mSUVmax) between patients with and without BM. These comparisons revealed that pSUVmax, nSUVmax, and mSUVmax are significantly higher in patients with than without BM, suggesting that patients with high metabolic activity are prone to developing BM. We further conducted multiple logistic regression analysis to evaluate the ability of PET/CT based on these 3 parameters to predict the probability of BM in patients with lung cancer. The AUC for this analysis was 0.737, indicating moderate predictive power.
In clinical practice, the serum tumor markers CEA, CA50, CA125, CA72-4, ferritin, NSE, SCCA, and CYFRA21-1 are routinely measured in patients with lung cancer or suspected lung cancer before and/or after treatment. Several studies have demonstrated the significant role of these tumor markers in the diagnosis, evaluation of treatment response, and assessment of prognosis for patients with lung cancer (14,17-19,29,30). Molina et al. showed that after combining the serum tumor markers CEA, CYFRA21-1, NSE, SCCA, CA153, and gastrin-releasing peptide precursor, the diagnostic sensitivity and specificity for lung cancer rose to 88.5% and 82%, respectively (29). High serum concentrations of CYFRA21-1 have been shown to be associated with poor prognosis in patients with NSCLC (31). Ayan et al. assessed the correlations between serum CEA and osteopontin (OPN) concentrations and 18F-FDG uptake in patients with lung cancer and BM and did not find any significant correlations; however, the increase in CEA and OPN concentrations may be a risk factor in patients with lung cancer and BM (32). Thus, differences in concentrations of serum tumor markers between lung cancer patients with and without BM needs to be clarified to identify more closely related risk factors. In this present study, we found that serum CEA, CA50, CA125, ferritin, NSE, and CYFRA21-1 concentrations were higher in patients with than without BM, but there was no significant difference between the 2 groups in serum CA72-4 and SCCA concentrations. Therefore, we also performed multiple logistic regression analysis to evaluate the role of these 6 serum tumor markers in predicting the probability of BM in patients with lung cancer. Our results yielded an AUC of 0.784, showing moderate predictive power.
The prognosis of different histopathological subtypes varies greatly, and patients with SCLC usually have a poor prognosis (33). Moreover, the clinical TNM stage is another important prognostic factor for patients with lung cancer. Patients with BM, regardless of histopathological subtype, often have adverse outcomes (34,35). Thus, early diagnosis and/or prediction of BM can play a central role in the management of patients with lung cancer. In the present study, the proportion of ADC and SCLC was significantly higher among patients with than without BM, but the opposite was true for SCC. Subsequently, we performed multiple logistic regression analysis to evaluate the role of histopathological subtype in predicting the probability of BM. This analysis yielded an AUC of 0.722, which was higher than the predictive power of individual histopathological subtypes.
Various models have been proposed to predict BM in patients with lung cancer using serological molecular bone markers (36,37). Zhou et al. established a model combining the biomarkers chemokine receptor type 4, bone sialoprotein, OPN, and bone morphogenetic protein-4 to predict BM in resected patients with stage III NSCLC, achieving a sensitivity and specificity of 71% and 70%, respectively (36). In addition, a serological molecular model based on parathyroid hormone-related peptide, osteoprotegerin, and the bone resorptive markers carboxyterminal telopeptide of type I collagen (β-CTX) and procollagen type I N-terminal propeptide (tP1NP) was established for early diagnosis and to monitor the progress of BM in patients with lung cancer, achieving a sensitivity and specificity of 85.8% and 89.7%, respectively (37). However, these above molecular bone markers are not routinely checked in patients with lung cancer. With the development of molecular imaging, 18F-FDG PET/CT is often used for diagnosis, staging or restaging, and monitoring the treatment response of lung cancer (38,39). The significance of PET/CT metabolic parameters, concentrations of serum tumor markers, and histopathological subtypes in the diagnosis and/or prediction of BM in patients with lung cancer has been studied. However, to our knowledge, the risk stratification of patients with or without BM based on metabolic parameters, serum tumor markers, and histopathological subtypes has not been reported. The results of the present study demonstrate a significant role for these factors in distinguishing between patients with and without BM, with an AUC 0.884 for predicting BM in patients with lung cancer; thus, this model could be used in clinical practice for the early identification of and intervention in patients at high risk of BM.
Although the results of this study are interesting, there are some limitations that need to be acknowledged. First, this was a retrospective study, and the number of patients enrolled, especially those with BM, was relatively low. Second, the 8 serum tumor markers were not tested in all patients. Third, due to the small number of patients, this study did not evaluate the correlation between BM and other distant metastatic organs, including the lung, liver, brain, and adrenal gland. Finally, there was no validation model in our study, and further research is needed to confirm our results.
Conclusions
In summary, this study investigated whether PET/CT parameters, 8 conventional serum tumor markers, and histopathological subtypes can be used to predict BM in patients with lung cancer. We identified significant associations between metabolic phenotypes (i.e., pSUVmax, nSUVmax, and mSUVmax), serum tumor markers (i.e., CEA, CA50, CA125, NSE, ferritin, and CYFRA21-1), and histopathological subtype (i.e., ADC, SCC, and SCLC) in patients with and without BM. Our combined model had an AUC of 0.884, which was significantly higher than that of each of the individual prediction models. However, further prospective studies are needed to verify our results.
Acknowledgments
Funding: This work was supported by the Ningbo Public Service Technology Foundation, China (grant No. 2021S176), the Exploration Project of Natural the Science Foundation of Zhejiang Province (grant No. LTGY23H180004), the Research Foundation of Hwa Mei Hospital at the University of Chinese Academy of Sciences (grant No. 2022HMKY27), the Ningbo Clinical Research Center for Medical Imaging (grant No. 2021L003), and the Provincial and Municipal Co-construction Key Discipline for Medical Imaging (grant No. 2022-S02).
Footnote
Reporting Checklist: The authors have completed the STARD reporting checklist. Available at https://qims.amegroups.com/article/view/10.21037/qims-22-741/rc
Conflicts of Interest: All authors have completed the ICMJE uniform disclosure form (available at https://qims.amegroups.com/article/view/10.21037/qims-22-741/coif). The authors have no conflicts of interest to declare.
Ethical Statement:
Open Access Statement: This is an Open Access article distributed in accordance with the Creative Commons Attribution-NonCommercial-NoDerivs 4.0 International License (CC BY-NC-ND 4.0), which permits the non-commercial replication and distribution of the article with the strict proviso that no changes or edits are made and the original work is properly cited (including links to both the formal publication through the relevant DOI and the license). See: https://creativecommons.org/licenses/by-nc-nd/4.0/.
References
- Siegel RL, Miller KD, Fuchs HE, Jemal A. Cancer statistics, 2022. CA Cancer J Clin 2022;72:7-33. [Crossref] [PubMed]
- Xia C, Dong X, Li H, Cao M, Sun D, He S, Yang F, Yan X, Zhang S, Li N, Chen W. Cancer statistics in China and United States, 2022: profiles, trends, and determinants. Chin Med J (Engl) 2022;135:584-90. [Crossref] [PubMed]
- Santini D, Barni S, Intagliata S, Falcone A, Ferraù F, Galetta D, et al. Natural History of Non-Small-Cell Lung Cancer with Bone Metastases. Sci Rep 2015;5:18670. [Crossref] [PubMed]
- Kuchuk M, Addison CL, Clemons M, Kuchuk I, Wheatley-Price P. Incidence and consequences of bone metastases in lung cancer patients. J Bone Oncol 2013;2:22-9. [Crossref] [PubMed]
- Coleman RE. Metastatic bone disease: clinical features, pathophysiology and treatment strategies. Cancer Treat Rev 2001;27:165-76. [Crossref] [PubMed]
- Jiang M, Zhang X, Chen Y, Chen P, Guo X, Ma L, Gao Q, Mei W, Zhang J, Zheng J. A Review of the Correlation Between Epidermal Growth Factor Receptor Mutation Status and (18)F-FDG Metabolic Activity in Non-Small Cell Lung Cancer. Front Oncol 2022;12:780186. [Crossref] [PubMed]
- Kirchner J, Sawicki LM, Nensa F, Schaarschmidt BM, Reis H, Ingenwerth M, Bogner S, Aigner C, Buchbender C, Umutlu L, Antoch G, Herrmann K, Heusch P. Prospective comparison of (18)F-FDG PET/MRI and (18)F-FDG PET/CT for thoracic staging of non-small cell lung cancer. Eur J Nucl Med Mol Imaging 2019;46:437-45. [Crossref] [PubMed]
- Niu R, Shao X, Shao X, Jiang Z, Wang J, Wang Y. Establishment and verification of a prediction model based on clinical characteristics and positron emission tomography/computed tomography (PET/CT) parameters for distinguishing malignant from benign ground-glass nodules. Quant Imaging Med Surg 2021;11:1710-22. [Crossref] [PubMed]
- Liu N, Ma L, Zhou W, Pang Q, Hu M, Shi F, Fu Z, Li M, Yang G, Yu J. Bone metastasis in patients with non-small cell lung cancer: the diagnostic role of F-18 FDG PET/CT. Eur J Radiol 2010;74:231-5. [Crossref] [PubMed]
- Lee JW, Lee SM, Lee HS, Kim YH, Bae WK. Comparison of diagnostic ability between (99m)Tc-MDP bone scan and (18)F-FDG PET/CT for bone metastasis in patients with small cell lung cancer. Ann Nucl Med 2012;26:627-33. [Crossref] [PubMed]
- Yao G, Zhou Y, Gu Y, Wang Z, Yang M, Sun J, Luo Q, Zhao H. A Retrospective Study of predicting risk of Metastasis among FDG-avid Bone Lesions in (18)F-FDG PET/CT. J Cancer 2020;11:4989-95. [Crossref] [PubMed]
- Li TC, Wang LL, Liu BL, Hong JJ, Xu NN, Tang K, Zheng XW. Association between bone marrow fluorodeoxyglucose uptake and recurrence after curative surgical resection in patients with T1-2N0M0 lung adenocarcinoma: a retrospective cohort study. Quant Imaging Med Surg 2020;10:2285-96. [Crossref] [PubMed]
- Kamel F, Eltarhoni K, Nisar P, Soloviev M. Colorectal Cancer Diagnosis: The Obstacles We Face in Determining a Non-Invasive Test and Current Advances in Biomarker Detection. Cancers (Basel) 2022;14:1889. [Crossref] [PubMed]
- Shibata C, Nakano T, Yasumoto A, Mitamura A, Sawada K, Ogawa H, Miura T, Ise I, Takami K, Yamamoto K, Katayose Y. Comparison of CEA and CA19-9 as a predictive factor for recurrence after curative gastrectomy in gastric cancer. BMC Surg 2022;22:213. [Crossref] [PubMed]
- Chai X, Yinwang E, Wang Z, Wang Z, Xue Y, Li B, Zhou H, Zhang W, Wang S, Zhang Y, Li H, Mou H, Sun L, Qu H, Wang F, Zhang Z, Chen T, Ye Z. Predictive and Prognostic Biomarkers for Lung Cancer Bone Metastasis and Their Therapeutic Value. Front Oncol 2021;11:692788. [Crossref] [PubMed]
- Arrieta O, Saavedra-Perez D, Kuri R, Aviles-Salas A, Martinez L, Mendoza-Posada D, Castillo P, Astorga A, Guzman E, De la Garza J. Brain metastasis development and poor survival associated with carcinoembryonic antigen (CEA) level in advanced non-small cell lung cancer: a prospective analysis. BMC Cancer 2009;9:119. [Crossref] [PubMed]
- Cedrés S, Nuñez I, Longo M, Martinez P, Checa E, Torrejón D, Felip E. Serum tumor markers CEA, CYFRA21-1, and CA-125 are associated with worse prognosis in advanced non-small-cell lung cancer (NSCLC). Clin Lung Cancer 2011;12:172-9. [Crossref] [PubMed]
- Yu D, Du K, Liu T, Chen G. Prognostic value of tumor markers, NSE, CA125 and SCC, in operable NSCLC Patients. Int J Mol Sci 2013;14:11145-56. [Crossref] [PubMed]
- Molina R, Filella X, Augé JM, Fuentes R, Bover I, Rifa J, Moreno V, Canals E, Viñolas N, Marquez A, Barreiro E, Borras J, Viladiu P. Tumor markers (CEA, CA 125, CYFRA 21-1, SCC and NSE) in patients with non-small cell lung cancer as an aid in histological diagnosis and prognosis. Comparison with the main clinical and pathological prognostic factors. Tumour Biol 2003;24:209-18. [Crossref] [PubMed]
- Kawaguchi T, Takada M, Kubo A, Matsumura A, Fukai S, Tamura A, Saito R, Kawahara M, Maruyama Y. Gender, histology, and time of diagnosis are important factors for prognosis: analysis of 1499 never-smokers with advanced non-small cell lung cancer in Japan. J Thorac Oncol 2010;5:1011-7. [Crossref] [PubMed]
- Lee EY, Khong PL, Lee VH, Qian W, Yu X, Wong MP. Metabolic phenotype of stage IV lung adenocarcinoma: relationship with epidermal growth factor receptor mutation. Clin Nucl Med 2015;40:e190-5. [Crossref] [PubMed]
- Feng SH, Yang ST. The new 8th TNM staging system of lung cancer and its potential imaging interpretation pitfalls and limitations with CT image demonstrations. Diagn Interv Radiol 2019;25:270-9. [Crossref] [PubMed]
- Raschka T, Weiss S, Reiter A, Barg A, Schlickewei C, Frosch KH, Priemel M. Outcomes and prognostic factors after surgery for bone metastases in the extremities and pelvis: A retrospective analysis of 140 patients. J Bone Oncol 2022;34:100427. [Crossref] [PubMed]
- Del Conte A, De Carlo E, Bertoli E, Stanzione B, Revelant A, Bertola M, Spina M, Bearz A. Bone Metastasis and Immune Checkpoint Inhibitors in Non-Small Cell Lung Cancer (NSCLC): Microenvironment and Possible Clinical Implications. Int J Mol Sci 2022;23:6832. [Crossref] [PubMed]
- van Baardwijk A, Dooms C, van Suylen RJ, Verbeken E, Hochstenbag M, Dehing-Oberije C, Rupa D, Pastorekova S, Stroobants S, Buell U, Lambin P, Vansteenkiste J, De Ruysscher D. The maximum uptake of (18)F-deoxyglucose on positron emission tomography scan correlates with survival, hypoxia inducible factor-1alpha and GLUT-1 in non-small cell lung cancer. Eur J Cancer 2007;43:1392-8. [Crossref] [PubMed]
- Pellegrino S, Fonti R, Pulcrano A, Del Vecchio S. PET-Based Volumetric Biomarkers for Risk Stratification of Non-Small Cell Lung Cancer Patients. Diagnostics (Basel) 2021;11:210. [Crossref] [PubMed]
- Sharma A, Mohan A, Bhalla AS, Sharma MC, Vishnubhatla S, Das CJ, Pandey AK, Sekhar Bal C, Patel CD, Sharma P, Agarwal KK, Kumar R. Role of Various Metabolic Parameters Derived From Baseline 18F-FDG PET/CT as Prognostic Markers in Non-Small Cell Lung Cancer Patients Undergoing Platinum-Based Chemotherapy. Clin Nucl Med 2018;43:e8-e17. [Crossref] [PubMed]
- Dooms C, van Baardwijk A, Verbeken E, van Suylen RJ, Stroobants S, De Ruysscher D, Vansteenkiste J. Association between 18F-fluoro-2-deoxy-D-glucose uptake values and tumor vitality: prognostic value of positron emission tomography in early-stage non-small cell lung cancer. J Thorac Oncol 2009;4:822-8. [Crossref] [PubMed]
- Molina R, Marrades RM, Augé JM, Escudero JM, Viñolas N, Reguart N, Ramirez J, Filella X, Molins L, Agustí A. Assessment of a Combined Panel of Six Serum Tumor Markers for Lung Cancer. Am J Respir Crit Care Med 2016;193:427-37. [Crossref] [PubMed]
- Zhang Z, Yuan F, Chen R, Li Y, Ma J, Yan X, Wang L, Zhang F, Tao H, Guo D, Huang Z, Zhang S, Li X, Zhi X, Ge X, Hu Y, Wang J. Dynamics of Serum Tumor Markers Can Serve as a Prognostic Biomarker for Chinese Advanced Non-small Cell Lung Cancer Patients Treated With Immune Checkpoint Inhibitors. Front Immunol 2020;11:1173. [Crossref] [PubMed]
- Xu Y, Xu L, Qiu M, Wang J, Zhou Q, Xu L, Wang J, Yin R. Prognostic value of serum cytokeratin 19 fragments (Cyfra 21-1) in patients with non-small cell lung cancer. Sci Rep 2015;5:9444. [Crossref] [PubMed]
- Ayan AK, Erdemci B, Orsal E, Bayraktutan Z, Akpinar E, Topcu A, Turkeli M, Seven B. Is there any correlation between levels of serum ostepontin, CEA, and FDG uptake in lung cancer patients with bone metastasis? Rev Esp Med Nucl Imagen Mol 2016;35:102-6. [Crossref] [PubMed]
- Stokes M, Berfeld N, Gayle A, Descoteaux A, Rohrmoser O, Franks A. A systematic literature review of real-world treatment outcomes of small cell lung cancer. Medicine (Baltimore) 2022;101:e29783. [Crossref] [PubMed]
- Ulas A, Bilici A, Durnali A, Tokluoglu S, Akinci S, Silay K, Oksuzoglu B, Alkis N. Risk factors for skeletal-related events (SREs) and factors affecting SRE-free survival for nonsmall cell lung cancer patients with bone metastases. Tumour Biol 2016;37:1131-40. [Crossref] [PubMed]
- Kang EJ, Lee SY, Kim HJ, Min KH, Hur GY, Shim JJ, Kang KH, Oh SC, Seo JH, Lee SY, Kim JS. Prognostic Factors and Skeletal-Related Events in Patients with Small Cell Lung Cancer with Bone Metastases at the Time of Diagnosis. Oncology 2016;90:103-11. [Crossref] [PubMed]
- Zhou Z, Chen ZW, Yang XH, Shen L, Ai XH, Lu S, Luo QQ. Establishment of a biomarker model for predicting bone metastasis in resected stage III non-small cell lung cancer. J Exp Clin Cancer Res 2012;31:34. [Crossref] [PubMed]
- Teng X, Wei L, Han L, Min D, Du Y. Establishment of a serological molecular model for the early diagnosis and progression monitoring of bone metastasis in lung cancer. BMC Cancer 2020;20:562. [Crossref] [PubMed]
- Kandathil A, Subramaniam RM. FDG PET/CT for Primary Staging of Lung Cancer and Mesothelioma. Semin Nucl Med 2022;52:650-61. [Crossref] [PubMed]
- Han J, Song Q, Guo F, Du R, Fang H, Kang J, Lu Z. Evaluation of response to stereotactic body radiation therapy for nonsmall cell lung cancer: PET response criteria in solid tumors versus response evaluation criteria in solid tumors. Nucl Med Commun 2022;43:717-24. [Crossref] [PubMed]