Early prediction of acute pancreatitis severity based on changes in pancreatic and peripancreatic computed tomography radiomics nomogram
Introduction
Acute pancreatitis (AP) is one of the most common gastrointestinal emergencies (1). According to the 2012 revised Atlanta Classification, AP can be categorized as mild acute pancreatitis (MAP), moderately severe acute pancreatitis (MSAP), and severe acute pancreatitis (SAP) (2). With a mortality rate reaching 30%, SAP has a poor prognosis (3). Therefore, early prediction of the severity of AP can be of great use for guiding treatment, improving patient outcomes, preventing the development of SAP, and ultimately saving patients’ lives.
Currently, several scoring systems are available for AP severity classification. Common clinical scoring systems include Ranson Criteria (4), the Acute Physiology and Chronic Health Evaluation II (APACHE II) (5), and the Bedside Index of Severity in Acute Pancreatitis (BISAP) (6), among others. Although these traditional scoring systems are able to predict SAP, the calculations involve cumbersome and time-consuming processes (7). For example, APACHE II requires a total of 16 tests to predict the severity, and many need to be measured in an intensive care unit (ICU) setting rather than a typical clinical setting. To predict SAP, Ranson scoring requires continuous collection of data over at least 48 hours starting from the admission of patients, causing a delay in the evaluation of the disease (8) and an increase in hospitalization costs for patients.
Radiomics extracts quantitative imaging features from digital images in a high-throughput manner and can reflect changes in the human body at the tissue, cellular, and gene level (9,10). A large amount of information that cannot be observed with the naked eye can be acquired in a noninvasive manner, providing support for clinical decision-making. This paper aimed to study the potential differences among AP patients of varying severity through radiomics features and establish a radiomics model to accurately assess the severity of AP at an early stage. In addition, a nomogram based on the radiomics features of pancreatic and peripancreatic regions was proposed to provide a reference for precision medicine. We present the following article in accordance with the TRIPOD reporting checklist (available at https://qims.amegroups.com/article/view/10.21037/qims-22-821/rc).
Methods
Data collection
We conducted a retrospective analysis of 1,408 patients with AP from January 1, 2016, to June 30, 2022, at the Affiliated Hospital of North Sichuan Medical College. This study was conducted in accordance with the Declaration of Helsinki (as revised in 2013) and was approved by the institutional ethics board of the Affiliated Hospital of North Sichuan Medical College. Patient informed consent of all cases was waived owing to the retrospective nature and absence of harm for all patients. The 2012 revised Atlanta Classification was referred to for AP diagnostic criteria and severity classification. A total of 215 first-episode patients with AP were enrolled and divided into 2 groups: the nonsevere group (64 MAP and 94 MSAP patients) and severe group (57 SAP patients). A total of 141 patients enrolled between January 1, 2016, and July 30, 2021, were assigned to the training and validation cohorts, including 113 nonsevere patients and 28 severe patients. The 74 patients enrolled from August 1, 2021, to June 30, 2022, were used as the test cohort, including 45 nonsevere patients and 29 severe patients.
The inclusion criteria were the following: (I) first-episode AP patients, (II) enhanced abdominal computed tomography (CT) examination, and (III) a time interval from admission to enhanced CT examination not exceeding 2 days. The exclusion criteria were the following: (I) electronic medical record with incomplete medical history and/or present illness history, (II) transferred patients, (III) previous hepatic cirrhosis and malignant tumor, (IV) major pelvic and/or abdominal surgery within 1 month, (V) current infectious disease(s), and (VI) poor quality of enhanced-CT images. The flowchart of patient recruitment is shown in Figure 1.
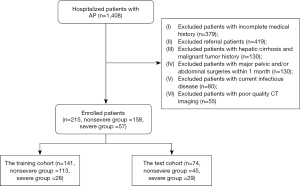
CT imaging collection
All patients with AP underwent abdominal enhanced CT examination with Siemens SOMATOM Force CT (2 sets of data acquisition modes, 2×96 detector rows). The scanning parameters were as follows: tube voltage, 120 kV; tube current, 200 mAs; field of view (FOV), 30 cm × 30 cm; matrix, 512×512; voxel size, 1×1×5; scanning slice thickness, 5 mm; interslice interval, 5 mm; and pitch, 0.6–1. To perform the scanning, 1.5 mL/kg of nonionic contrast agent was injected through the cubital vein at a flow rate of 3.5–4 mL/s, and the portal venous phase scanning timing was 65–70 s after the injection. Images were retrieved retrospectively from the Picture Archiving and Communication System (PACS).
Region of interest (ROI) delineation and feature extraction
The ROI consisted of 2 parts: label 1 for the entire pancreas (including the necrotic area and excluding blood vessels and bile ducts) and label 2 for the large regions of peripancreatic exudation and effusion. Without knowledge of the patient clinical outcome, manual delineation layer by layer based on the three-dimensional (3D) Slicer (https://www.slicer.org/) platform was performed by 2 abdominal radiologists with 8 and 10 years of clinical experience, respectively. PyRadiomics, an open-source software package in Python (version 3.9.6), was used to automatically extract radiomics features on the Jupyter notebook platform. A collection of 3,040 features (label 1: 1,520; label 2: 1,520) were obtained based on filtering transformations, including common morphological features, first-order gray histogram features, second-order and higher-order texture features, and other features.
Intraobserver reliability and interobserver agreement
Two radiologists (Dr. B Xiao with 12 years’ experience and Dr. Yang Li with 8 years’ experience in abdominal imaging) delineated the ROIs on 30 randomly selected images and extracted radiomics features to assess the intraobserver reliability and interobserver agreement of feature extraction. For the former, observer 1 delineated the ROI twice and extracted radiomics features twice using the same method within a week; for the latter, observer 2 delineated the ROI only once, and the results were compared with the features extracted by observer 1 the first time. Intraobserver reliability and interobserver agreement were assessed using intraclass correlation coefficients (ICCs). To evaluate intraobserver reliability, we calculated the ICC estimates and corresponding 95% confidence interval (CI) using a single-measurement, absolute-agreement, 2-way mixed effects model. For interobserver agreement, we calculated the ICC estimates and corresponding 95% CI using a single-measurement, absolute-agreement, 2-way random effects model. An ICC value above 0.7 indicated good agreement (11). If good agreement was achieved, observer 1 would delineate the remaining samples.
Feature process and selection
The feature data were discretized and encoded by the method of equal distant binning and equal frequency binning. The light gradient boosting machine (LightGBM) algorithm was used for feature selection to obtain the top-ranked features to improve the accuracy of the model and reduce computational cost. LightGBM is both a tree modeling algorithm and an embedded feature selection algorithm, and it can generate information gained for each feature during modeling or record the total number of splits of the feature in the whole model, providing an importance score of the feature. After sorting by feature importance scores, we built the model by gradually increasing the number of features in a decreasing importance manner to acquire the best subcohort of radiomics features.
Logistic regression (LR) model construction
The LR model was established based on the best radiomics features, and 10-fold cross-validation was used to train the model. The model was then applied to the test cohort. The model’s prediction performance was evaluated according to the area under the receiver operating characteristic (ROC) curve (AUC) of the sensitivity, specificity, and accuracy.
Nomogram plotting
A nomogram was plotted based on the best radiomics features, and the top 3 features with the highest feature importance scores were displayed individually. The remaining parts were displayed with a linear combination of the feature multiplied by the corresponding nonzero coefficients. A flowchart of the radiomics model construction is shown in Figure 2.
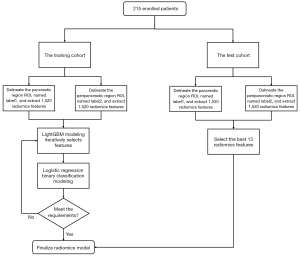
Statistical analysis
Statistical analysis was performed using SPSS version 26.0 (IBM Corp.). Analysis of patient age was performed using t-test. The distribution of patient gender, type of AP, and occurrence rates of multiple organ dysfunction syndrome (MODS), ICU, and mortality were all statistically analyzed using the chi-squared test.
Results
Demographic and clinical characteristics
A total of 215 patients with AP were enrolled in this study, including 158 patients with nonsevere AP and 57 patients with severe AP. Among all patients with AP, the distribution of AP type and occurrence rates of MODS and ICU were significantly different between the nonsevere and severe groups for both the training and test cohorts. The age of the patients (P=0.021) and mortality (P<0.001) were significantly different between the 2 groups in the training cohort. The sex of the patients was not significantly different between the 2 groups in both the training cohort (P=0.579) and the test cohort (P=0.609). The baseline clinical data of the patients are shown in Table 1.
Table 1
Characteristics | Training cohort (n=141) | Test cohort (n=74) | |||||||
---|---|---|---|---|---|---|---|---|---|
Nonsevere AP (n=113) | Severe AP (n=28) | Statistical value | P value | Nonsevere AP (n=45) | Severe AP (n=29) | Statistical value | P value | ||
Age (years) | 49.81±15.39 | 57.64±17.02 | t=2.34 | 0.021 | 55.13±18.735 | 55.13±18.735 | t=0.27 | 0.979 | |
Sex (male) | 71 (62.83) | 16 (57.14) | χ2=0.31 | 0.579 | 26 (57.78) | 15 (51.72) | χ2=0.262 | 0.609 | |
Etiology | χ2=1.03 | 0.793 | χ2=7.655 | 0.022 | |||||
Biliary | 26 (23.01) | 8 (28.57) | 18 (40.00) | 14 (48.28) | |||||
Hyperlipemia | 41 (36.28) | 7 (25.00) | 8 (17.78) | 11 (37.93) | |||||
Alcoholic | 32 (28.32) | 8 (28.57) | 14 (31.11) | 2 (6.90) | |||||
Trauma | 1 (0.88) | 1 (3.57) | 2 (4.44) | 0 (0) | |||||
Others | 13 (11.50) | 4 (14.29) | 3 (6.67) | 2 (6.90) | |||||
MODS | 0 (0) | 28 (100.00) | χ2=141 | <0.001 | 0 (0) | 29 (100.00) | χ2=74 | <0.001 | |
ICU | 1 (0.88) | 7 (25.00) | χ2=24.38 | <0.001 | 1 (2.22) | 5 (17.24) | χ2=5.339 | <0.001 | |
Type of AP | χ2=19.96 | <0.001 | <0.001 | ||||||
Acute edematous pancreatitis | 101 (89.38) | 18 (64.29) | 44 (97.78) | 14 (48.28) | |||||
Acute necrotizing pancreatitis | 12 (10.62) | 10 (35.71) | 1 (2.22) | 15 (51.72) | |||||
Mortality | 0 (0) | 3 (10.71) | χ2=12.37 | <0.001 | 0 (0) | 1 (3.45) | χ2=1.573 | 0.210 |
Data are presented as mean ± standard deviation or number (%). P<0.05 indicates statistical significance. MODS, multiple organ dysfunction syndrome; ICU, intensive care unit; AP, acute pancreatitis.
Intraobserver reliability and interobserver agreement
For label 1, the mean ICC for intraobserver reliability was 0.932 (95% CI: 0.814–0.976) while the interobserver agreement was 0.881 (95% CI: 0.702–0.951). For label 2, the mean ICC for intraobserver agreement was 0.892 (95% CI: 0.716–0.962) while the interobserver agreement was 0.845 (95% CI: 0.598–0.945). The intraobserver and interobserver ICC values for all extracted radiomics features were greater than 0.7, and therefore all 3,040 radiomics features were included in the follow-up study.
Feature process and selection
In the LightGBM algorithm, the AUC values were recorded as the number of features increased and the optimal radiomics features were screened out. When the number of features reached 13, the AUC remained stable at 94.75%; that is, further increase in feature numbers no longer brought a rise in the AUC value but made the model more complicated. Therefore, 13 optimal radiomics features were used for model construction.
Model validation and nomogram plotting
Good performance of the LR model was observed in both the training and test cohorts (Figure 3A). The AUC was 0.992 (95% CI: 0.963–0.996) and 0.894 (95% CI: 0.789–0.966) in the training cohort and test cohort, respectively, the sensitivity was 0.862 (25/29; 95% CI: 0.674–0.954), the specificity was 0.800 (36/45; 95% CI: 0.649–0.899), and the accuracy was 0.824 (95% CI: 0.720–0.919). Visualization of the LR model incorporating the radiomics feature predictors is presented as a nomogram (Figure 3B). When the total score was greater than 124 points, SAP was predicted.
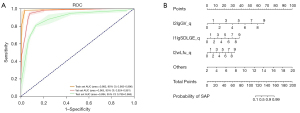
Feature interpretability
We found 13 features which could be used as risk factors for predicting the severity of AP, including label2_logarithm_glrlm_GrayLevelVariance_qcut (l2lgGV-q) and label1_log-sigma-2-0-mm-3D_gldm_SmallDependenceLowGrayLevelEmphasis_qcut (l1lgSDLGE-q). Feature importance was measured by Shapley Additive Explanations (SHAP) value (Figure 4).
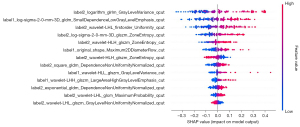
Model input variables are ranked in descending order of feature importance/value. Each line represents a feature, and the abscissa is the SHAP value. Points to the right of the line of neutral contribution resulted in a higher score; points to the left of this line resulted in a lower score. Red is indicative of a high feature value, while blue is indicative of a low feature value. cut, equal width binning; qcut, equal frequency binning; LR, logistic regression; SHAP, Shapley Additive Explanations.
Among the features above, l1lgSDLGE-q was the most important feature in ROI label 1. The acquiring process included performing Laplacian of Gaussian (LOG) filtering on the image first with a 2-mm filter width (sigma), extracting the ROI in 3D, performing gray-level dependence matrix (GLDM) transformation, and calculating the small dependence low-gray level emphasis (SDLGLE). The larger the SDLGLE value was, the smaller the dependency between the adjacent pixels in the low-gray level area was, indicating a more uneven texture in the ROI (Figure 5A). The SDLGLE formula was as follows:
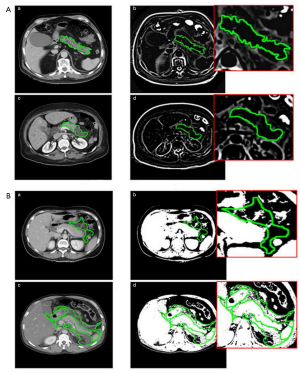
l2lgGV-q was the most important feature in the ROI label 2. The acquiring process included performing logarithmic filtering on the image to obtain the logarithm of the absolute intensity plus 1 of the image and then performing gray-level run length matrix (GLRLM) transformation to quantify the gray level of the image and calculating the gray level variance (GLVAR). The larger the Frlm.gl.var value was, the greater the average gray level change was in the ROI (Figure 5B). The Frlm.gl.var formula was as follows:
Discussion
The early identification of patients with SAP is of great significance for clinical treatment guidelines. Based on portal venous phase enhanced-CT images, this paper used a machine learning algorithm to establish a radiomics model using features extracted by delineating the ROI in the pancreatic and peripancreatic regions. The model was then displayed in a nomogram. This study identified 13 radiomics features as risk factors for the early prediction of AP severity. The AUC of the radiomics model was 0.992 and 0.894 in the training cohort and test cohort, respectively. The nomogram based on these features suggested that when the total score was greater than 124 points, SAP would be predicted.
Many studies have explored different aspects of AP using medical imaging. Jiang et al. (12) reported that patients with early-phase AP frequently had vascular anomalies on magnetic resonance imaging (MRI), such as splenic vein phlebitis and splenic artery arteritis, which may serve as additional markers for pancreatitis severity. According to Peng et al. (13), pleural effusion and pulmonary consolidation are associated with AP severity. Additionally, the pleural effusion volume and pulmonary consolidation lobes can predict severe AP and organ failure in advance. Tasu et al. (14) found that pancreatic enhancement less than 30 Hounsfield units (HU) in portal phase CT-enhanced images was an accurate and repeatable threshold for the diagnosis of necrotizing pancreatitis (NP) and could correctly distinguish nonsevere AP from severe AP. Roussey et al. (15) revealed that liver spontaneous attenuation (LSA) reflecting the degree of steatosis on CT images was related to the severity of AP, and that the median LSA in images of patients with severe AP was lower than that of patients with nonsevere AP. Liao et al. (16) analyzed the correlation between readmission and reintervention by determining the pancreatic necrosis volume (PNV) and confirmed that PNV was a useful tool for quantifying pancreatic necrosis and was strongly associated with readmission and reintervention. Gupta et al. (17) found that the site and size of extrapancreatic necrosis (EPN) were associated with severity as well as various clinical outcomes of patients with AP. By using radiomics, we hoped to uncover information that differed from traditional imaging findings and capture the characteristics of tissues and lesions more comprehensively, thus providing a more in-depth understanding of the disease. In addition, in order to improve the readability of the radiomics machine learning model, we also plotted a nomogram based on radiomics features, which could calculate the scores of important features and judge the severity of AP more intuitively. The nomogram showed that in the ROIs label 1 and label 2, features l1lgSDLGE_q and l2lgGV_q were both positively correlated with the severity of AP, indicating that the larger the feature value was, the higher the probability of SAP diagnosis was. When SAP occurs, the pancreatic tissue becomes inflamed, congested, edematous, and even necrotic, resulting in a large variance in the internal components of the pancreas, which can be essentially interpreted as an uneven texture. In the peripancreatic region, due to the mixture of a large amount of exudate and adipose tissue, the average gray level varies greatly in this area.
Many supervised machine learning algorithms require a discretized feature space to perform. With feature discretization, the model is more stable, and classification accuracy will be improved while the risk of overfitting will be reduced (18). In this paper, feature processing adopted the discretization methods of equal distance binning and equal frequency binning, and instance labels were not used in the discretization process. These methods are referred to as unsupervised discretization since label information is not used when setting partition boundaries, and so values that are from different categories but strongly associated are likely combined into the same bin, thus preventing the issue of label leakage (19). In some cases, this could contribute to more accurate classification.
The conditions of AP are complex and diverse. In addition to the presence of local pancreatic lesions, the area surrounding the pancreas can also be implicated. Compared with the study of Lin et al. (20), our ROI delineation included the pancreas and the peripancreatic region, and thus the analysis of extracted features might have reflected the changes in AP severity more comprehensively and accurately. When SAP develops, various enzymes in the pancreatic juice are activated, and extensive edema, hemorrhage, necrosis, and the accumulation of a large amount of exudate can be seen in the pancreas and peripancreatic tissues. Therefore, in addition to the changes of the pancreas on CT images, there may also be manifestations such as single-regional pancreatic fluid retention and multiregional pancreatic fluid retention due to hemorrhage, necrosis, and exudative changes around the pancreas.
This study had certain limitations. This was a single-center, small-sample study, and the proportion of patients with SAP was relatively low due to the strict screening criteria used. As a result, we plan next to conduct a multicenter, large-sample study to verify the robustness and reproducibility of our established radiomics model. In addition, our goal is to combine clinical risk factors closely related to the occurrence of SAP with radiomics features to develop a personalized prediction model, further improve the prediction efficiency, and increase the reliability and applicability for the clinical identification of SAP.
In conclusion, we proposed an efficient and easy-to-use radiomics model based on enhanced CT images, providing a noninvasive and convenient method for the early prediction of severity in patients with AP.
Acknowledgments
Funding: This study was funded by the Bureau of Science & Technology Nanchong City (No. 20SXQT0315 to YZ).
Footnote
Reporting Checklist: The authors have completed the TRIPOD reporting checklist. Available at https://qims.amegroups.com/article/view/10.21037/qims-22-821/rc
Conflicts of Interest: All authors have completed the ICMJE uniform disclosure form (available at https://qims.amegroups.com/article/view/10.21037/qims-22-821/coif). YZ reports funding received from the Bureau of Science & Technology Nanchong City (No. 20SXQT0315). The other authors have no conflicts of interest to declare.
Ethical Statement: The authors are accountable for all aspects of the work in ensuring that questions related to the accuracy or integrity of any part of the work are appropriately investigated and resolved. The study was conducted by the Declaration of Helsinki (as revised in 2013). The study was approved by the institutional ethics board the of Affiliated Hospital of North Sichuan Medical College, and individual consent for this retrospective analysis was waived.
Open Access Statement: This is an Open Access article distributed in accordance with the Creative Commons Attribution-NonCommercial-NoDerivs 4.0 International License (CC BY-NC-ND 4.0), which permits the non-commercial replication and distribution of the article with the strict proviso that no changes or edits are made and the original work is properly cited (including links to both the formal publication through the relevant DOI and the license). See: https://creativecommons.org/licenses/by-nc-nd/4.0/.
References
- Lankisch PG, Apte M, Banks PA. Acute pancreatitis. Lancet 2015;386:85-96. [Crossref] [PubMed]
- Banks PA, Bollen TL, Dervenis C, Gooszen HG, Johnson CD, Sarr MG, Tsiotos GG, Vege SS. Classification of acute pancreatitis--2012: revision of the Atlanta classification and definitions by international consensus. Gut 2013;62:102-11. [Crossref] [PubMed]
- Habtezion A, Gukovskaya AS, Pandol SJ. Acute Pancreatitis: A Multifaceted Set of Organelle and Cellular Interactions. Gastroenterology 2019;156:1941-50. [Crossref] [PubMed]
- Ranson JH, Pasternack BS. Statistical methods for quantifying the severity of clinical acute pancreatitis. J Surg Res 1977;22:79-91. [Crossref] [PubMed]
- Larvin M, McMahon MJ. APACHE-II score for assessment and monitoring of acute pancreatitis. Lancet 1989;2:201-5. [Crossref] [PubMed]
- Wu BU, Johannes RS, Sun X, Tabak Y, Conwell DL, Banks PA. The early prediction of mortality in acute pancreatitis: a large population-based study. Gut 2008;57:1698-703. [Crossref] [PubMed]
- Cho SK, Kim JW, Huh JH, Lee KJ. Atherogenic Index of Plasma Is a Potential Biomarker for Severe Acute Pancreatitis: A Prospective Observational Study. J Clin Med 2020.
- Di MY, Liu H, Yang ZY, Bonis PA, Tang JL, Lau J. Prediction Models of Mortality in Acute Pancreatitis in Adults: A Systematic Review. Ann Intern Med 2016;165:482-90. [Crossref] [PubMed]
- Lambin P, Rios-Velazquez E, Leijenaar R, Carvalho S, van Stiphout RG, Granton P, Zegers CM, Gillies R, Boellard R, Dekker A, Aerts HJ. Radiomics: extracting more information from medical images using advanced feature analysis. Eur J Cancer 2012;48:441-6. [Crossref] [PubMed]
- Kumar V, Gu Y, Basu S, Berglund A, Eschrich SA, Schabath MB, Forster K, Aerts HJ, Dekker A, Fenstermacher D, Goldgof DB, Hall LO, Lambin P, Balagurunathan Y, Gatenby RA, Gillies RJ. Radiomics: the process and the challenges. Magn Reson Imaging 2012;30:1234-48. [Crossref] [PubMed]
- Koo TK, Li MY. A Guideline of Selecting and Reporting Intraclass Correlation Coefficients for Reliability Research. J Chiropr Med 2016;15:155-63. [Crossref] [PubMed]
- Jiang ZQ, Xiao B, Zhang XM, Xu HB. Early-phase vascular involvement is associated with acute pancreatitis severity: a magnetic resonance imaging study. Quant Imaging Med Surg 2021;11:1909-20. [Crossref] [PubMed]
- Peng R, Zhang L, Zhang ZM, Wang ZQ, Liu GY, Zhang XM. Chest computed tomography semi-quantitative pleural effusion and pulmonary consolidation are early predictors of acute pancreatitis severity. Quant Imaging Med Surg 2020;10:451-63. [Crossref] [PubMed]
- Tasu JP, Guen RL, Rhouma IB, Guerrab A, Beydoun N, Bergougnoux B, Ingrand P, Herpe G. Accuracy of a CT density threshold enhancement to identify pancreatic parenchyma necrosis in acute pancreatitis during the first week. Diagn Interv Imaging 2022;103:266-72. [Crossref] [PubMed]
- Roussey B, Calame P, Revel L, Zver T, Konan A, Piton G, Koch S, Vuitton L, Delabrousse E. Liver spontaneous hypoattenuation on CT is an imaging biomarker of the severity of acute pancreatitis. Diagn Interv Imaging 2022;103:401-7. [Crossref] [PubMed]
- Liao Q, Ding L, Xu X, Yu C, Deng F, Xiong H, He W, Xia L, Zeng X, Lu N, Zhu Y. Pancreatic necrosis volume for predicting readmission and reintervention in acute necrotizing pancreatitis. Eur J Radiol 2022;154:110419. [Crossref] [PubMed]
- Gupta P, Rana P, Bellam BL, Samanta J, Mandavdhare H, Sharma V, Sinha SK, Dutta U, Kochhar R. Site and size of extrapancreatic necrosis are associated with clinical outcomes in patients with acute necrotizing pancreatitis. Pancreatology 2020;20:9-15. [Crossref] [PubMed]
- Ferreira AJ, Figueiredo MAT. An unsupervised approach to feature discretization and selection. Pattern Recognition 2012;45:3048-60. [Crossref]
- Dougherty J, Kohavi R, Sahami M. Supervised and Unsupervised Discretization of Continuous Features BT - Machine Learning Proceedings 1995. Machine Learning Proceedings 1995 1995:194-202.
- Lin Q, Ji YF, Chen Y, Sun H, Yang DD, Chen AL, Chen TW, Zhang XM. Radiomics model of contrast-enhanced MRI for early prediction of acute pancreatitis severity. J Magn Reson Imaging 2020;51:397-406. [Crossref] [PubMed]