A systematic review of the modelling of patient arrivals in emergency departments
Introduction
Accident and emergency departments (AEDs) play an important role in the urgent assessment and treatment of illness and injury. Acting as a buffer and pending zone for other specialties, AEDs play an essential gatekeeping role for undifferentiated patients. Figures from NHS England reveal the pressure on hospital AEDs in the UK. Only 92.6% patients were seen within four hours, which cannot reach the normal service level. A similar situation is common in other countries and territories. Given the challenges of an ageing population, AED manpower shortages, and fluctuations in patient numbers (for instance due to an infectious disease outbreak or winter surge in arrivals), it is essential to ensure a high quality and reliable AED operation and provide efficient services. To solve this problem, large volume of researches (1,2) have been carried out to determine optimal staffing levels and scheduling in AEDs, with the purpose of maximising resource utilisation and patient satisfaction. Prediction of AED patient arrivals is important for decision-making across the whole AED system and is a prerequisite for staff rostering and scheduling, whether using a simulation-based approach (3) or a mathematical programming approach (4).
A previous study (5) focused on techniques for forecasting AED visits and was intended to provide basic modelling guidelines to practitioners. Recently, healthcare information systems have enabled access to accurate information about AED patient arrivals. To respond to the opportunities big data brings to healthcare, many AEDs now recruit health data analytics specialists with strong statistical training to analyse their institutional data. More sophisticated approaches (such as machine learning, black box, and hybrid methods) have had proven success in obtaining more powerful model predictions. Model performance should be evaluated to determine the robustness of the various methods of demand prediction and an in-depth systematic review of patient arrivals modelling is needed. This study reviews AED demand prediction studies and analyses and compares the different uses of prediction models, factor selection and model performance. We summarise research gaps and provide suggestions for improvement for future AED modellers. We present the following article in accordance with the PRISMA reporting checklist (available at https://qims.amegroups.com/article/view/10.21037/qims-22-268/rc) (6).
Methods
Search strategy and data extraction
Online literature searches were performed in late October 2021. Three major electronic bibliographic databases (Medline, ScienceDirect and Scopus) were used. For each database, we conducted an initial search with the following search keywords: ‘forecast OR predict’ AND ‘daily attendances OR patient volumes OR patient visits’ AND ‘emergency room OR emergency department’ AND ‘time series OR regression’. The publication date range was from 2004 to 2021. Based on the title and abstract or full text, a two-step screening process was carried out according to the inclusion and exclusion criteria described in the next section.
Study selection
For papers to be eligible for inclusion in this review, the reported study’s main objective had to be predicting the volume of patient arrivals to an AED or department serving a similar clinical function. We considered studies that used mathematical or statistical modelling approaches and some other nonlinear approaches.
A two-step screening process was adopted to select eligible papers for the final analysis and the Endnote reference management software (version No. X8.1) was used to conduct the entire study selection and duplicates exclusion (see Table 1). The initial screening was based on the titles and abstracts of all the selected papers. These papers were evaluated based on the first set of exclusion criteria. We excluded papers concerning cost estimation or other topics not directly related to patient arrivals. We also excluded papers aiming to provide decision-making solutions for clinical operations, including scheduling or optimisation. Pathological studies in AED settings were also eliminated. Papers duplicated in different databases were omitted.
Table 1
Inclusion criteria | Exclusion criteria |
---|---|
Years considered: 2004–2021 Language: English Publication status: published with formal issue or volume number Main objective: volume of patient arrivals prediction in AED Methodology: mathematical or statistical or machine learning-based modelling approaches |
First step: • Papers concerning cost estimation or other topics not directly related to patient arrivals • Papers focusing on decision-making solutions for clinical operations • Papers conducting statistical analysis of a certain disease • Pathological studies in AED settings |
Second step: • Papers with objective of measuring or optimizing AED operational factors • Simulation-based approaches • Papers concerning patient admission prediction • Papers aiming to quantify AED crowding levels |
AED, accident and emergency department.
The full texts of the remaining articles were then evaluated based on a second set of exclusion criteria. We eliminated studies that aimed to measure or optimize AED operational factors, for instance, mean waiting time and staffing level. Simulations based on prediction or queuing models were also excluded. We excluded papers that focused on forecasting patient admissions based on patient arrivals and studies aiming to quantify AED crowding levels without taking patient volume into consideration. Figure 1 shows the full literature search procedure.
Results
A total of 1,677 papers that fulfilled the initial searching criteria was obtained from the three databases. Based on the first exclusion criteria, 1,603 articles were eliminated. The remaining 74 full text articles were evaluated based on the second exclusion criteria. Finally, 35 articles were selected for full review.
Forecasting methods
In this section, we provide an evaluation and comparison of modelling methods based on the selected papers. Table 2 provides an overview of the research goals, methods and settings of the studies. Note that various methods may be used in a single study; if applicable, more than one method is listed. Based on the reviewed articles, we categorised AED patient arrivals modelling methods into four main classes: regression, time series, artificial intelligence and time series regression (see Table 3).
Table 2
Paper ID | Research goal | Method | Setting |
---|---|---|---|
Tai et al., [2007] (7) | To evaluate the effects of change in ambient temperature on AED patient visits | Regression | Five-year data from the AED of National Taiwan University Hospital, China |
Jones et al., [2008] (8) | To explore and evaluate the use of several statistical forecasting methods to predict daily AED patient volumes at three hospital AEDs | Regression, time series, artificial intelligence | Daily patient arrivals at three hospital AEDs were collected for 3 years in Salt Lake City, USA |
McCarthy et al., [2008] (9) | To develop a methodology for predicting demand for AED services by characterising AED arrivals | Regression | One year’s AED arrival data from an academic AED, USA |
Boyle et al., [2008] (10) | To analyse patient admission data and carry out forecasts using regression techniques | Regression, time series | Five years’ AED admissions data from two hospitals in Australia |
Schweigler et al., [2009] (11) | To investigate whether models using time series methods can generate accurate short-term forecasts of AED bed occupancy | Time series | One year’s hourly AED bed occupancy values were collected from three tertiary care hospitals, USA |
Sun et al., [2009] (12) | To predict total patient attendance and patient volume for each of the three acuity categories | Time series | Three-year data at the AED of an acute care regional general hospital in Singapore |
Kam et al., [2010] (13) | To develop and evaluate time series models to predict the daily number of patients visiting the AED | Time series | Data were collected from the information system database of an AED in Korea |
Wargon et al., [2010] (14) | To investigate whether mathematical models using calendar variables could identify the determinants of AED census in geographically close AEDs and to assess the performance of long-term forecasts | Regression | Four years’ daily visits to four AEDs at academic hospitals in the Paris area, France |
Chen et al., [2011] (15) | To analyse meteorological, clinical and economic factors in terms of their effects on monthly AED revenue and visitor volume | Time series | Four years’ monthly data collected from the AED of a regional teaching hospital in Taiwan, China |
Xu et al., [2011] (16) | To investigate several contributing factors to AED visits, and various time series methods of modelling AED visits with different triage categories and mode of arrival | Regression, time series | One year’s administrative data retrieved from the clinical management system of an AED in Hong Kong, China |
Aladag et al., [2012] (17) | To examine different activation functions to obtain more accurate out sample predictions when the number of patients is being forecasted | Artificial intelligence | Four year’s monthly observations of outpatient visits to a health centre at Hacettepe University, Turkey |
Boyle et al., [2012] (18) | To develop and validate models to predict AED presentations and hospital admissions for time and day of the year | Time series | Five years’ historical data from two dissimilar hospitals in Australia |
Moustris et al., [2012] (19) | To forecast childhood asthma admissions using different ANN models | Artificial intelligence | Weekly number of childhood asthma admissions during a four-year period in the greater Athens area, Greece |
Xu et al., [2013] (20) | To select covariables by a data-driven method and propose a novel attempt for using an artificial neural network (ANN) to model patient arrivals | Artificial intelligence | One year’s patient arrivals data from an AED in Hong Kong, China |
Marcilio et al., [2013] (21) | To develop models to forecast the daily number of patients seeking AED care in a general hospital and to compare the models in terms of forecasting accuracy | Regression, time series, time series regression | Three years’ total daily patient visits to an AED in Sao Paulo, Brazil |
Bergs et al., [2014] (22) | To evaluate an automatic forecasting algorithm that predicts the number of monthly AED visits one year ahead | Time series | The number of monthly visiting patients for a 6-year period from four hospitals in Belgium |
Kadri et al., [2014] (23) | To develop models for forecasting daily attendances at an AED | Time series | One year’s daily patient attendances at the paediatric ED in the Lille regional hospital centre, France |
Menke et al., [2014] (24) | To develop an ANN method and to test it retrospectively to determine if it can predict AED volume | Artificial intelligence | Two year’s data from an inner-city tertiary care hospital, USA |
Ekström et al., [2015] (25) | To investigate whether Web site visits to a regional medical Web site, the Stockholm Health Care Guide, a proxy for the general public’s concern of their health, could be used to predict the ED attendance for the coming day. | Regression | The period of August 13, 2011, to August 12, 2012 from the Stockholm County Council administrative database |
Afilal et al., [2016] (26) | To predict long and short term daily attendance based on time-series forecasting models | Time series | Date from the first January 2010 to the 31st December 2014 in Troyes Hospital Center |
Xu et al., [2016] (27) | To combine ARIMA and linear regression (LR) models and develop a hybrid ARIMA–LR approach to forecast ED patient visits in two EDs in China | Time series, regression | We extract daily ED visits in both hospitals from January 1, 2012 to December 31, 2013 |
Lin et al., [2017] (28) | To conduct comparisons between current waiting times and potential reduction of waiting times by optimizing the doctor rosters | Time series | The period of historical Patient Count is from 1st January 2015 to 29th April 2016 |
Carvalho-Silva et al., [2018] (29) | To decreases the cancellations of planned admissions and optimizes the beds allocation to the real demand. | Time series | Data for ED arrivals in 2 years (2012–2013) from Braga Hospital, in Portugal |
Jiang et al., [2018] (30) | To explore an integrated framework with high accuracy for predicting AED patient flow under different triage levels, by combining a novel feature selection process with deep neural networks. | Artificial intelligence | Administrative data was obtained from the A&ED of a flagship hospital from 1st July 2009 to 31th March 2011 in Hong Kong SAR, China |
Khaldi et al., [2018] (31) | To combine Artificial Neural Networks with a signal decomposition technique named Ensemble Empirical Mode decomposition, to make one-ahead forecasting of patients arrivals to ED | Artificial intelligence | Seven years’ weekly date collected from 2010 to 2016 in the University Hospital Hassan II of Fez city of Morocco |
Ho et al., [2019] (32) | To predict emergency department (ED) patient volume in hospitals using publicly available Google Trends search data. | Regression | The visit records of actual ED visits in Singapore General Hospital from January 2006 to February 2019 |
Whitt and Zhang, (2019] (33) | To forecast future daily arrival totals and predicting hourly occupancy levels in real time. | Time series regression | Data from the large 1000-bed Rambam Hospital in Haifa, Israel, from 2004–2007 |
Jilani et al., [2019] (34) | To create a tool that accurately predicts attendance at emergency departments to support optimal planning of human and physical resources | Time series Artificial intelligence |
Four years’ historical attendance data between Jan-2011 – December-2015 from four hospitals |
Almeida et al., [2020] (35) | To identify and analyze temporal periodicities of a self-referred pediatric ED (PED), correlate them with meteorological and calendar variables and build a robust forecasting model. | Time series regression |
An 8-year data set (2010-2017) of the daily number of admissions to the PED of a public hospital in Lisbon |
Choudhury et al., [2020] (36) | To help improve emergency department management by predicting the arrival of future patients. |
Time series | Data from January 2014 to August 2017 was retrieved from a hospital in Iowa |
Harrou et al., [2020] (37) | To propose an effective method to forecast daily and hourly visits at an ED using Variational AutoEncoder (VAE) algorithm | Artificial intelligence | The data consist of a number of visits at PED of Lille collected from January 2011 to November 2013 every hour |
Yousefi et al., [2020] (38) | To investigate the factors affecting daily demand in an emergency department (ED) and to provide a forecasting tool in a public hospital for horizons of up to seven days | Artificial intelligence | Data was collected in a period of 1,095 consecutive days, from January 2014 to November 2016 at Risoleta Tolentino Neves Hospital |
Fralick et al., [2021] (39) | Using ensembling approach for predicting emergency department volumes rather than focusing on one specific type of statistical model | Time series regression | Date from St Michael’s Hospital, Mount Sinai Hospital, Michael Garron Hospital during 2016-10-01 to 2019-05-31 |
Rema et al., [2021] (40) | To predict patient flow and provide a quantitative approach to make decisions related to resource planning and deployment by hospital administrators | Time series | Data was collected from a hospital located in a popular residential area in Bengaluru city from September to November |
Sudarshan, (2021] (41) | To help the hospital management in early planning and avoiding of overcrowding | Artificial intelligence | May 2015 to October 2017, January 2019 to December 2019 |
AED, accident and emergency department; ANN, artificial neural networks.
Table 3
Category | Type | Number of studies | References |
---|---|---|---|
Regression (causal) | Multiple linear regression (MLR) | 8 | Jones, 2008 (8); Tai, 2007 (7); Xu, 2013 (20); Wargon, 2010 (14); Boyle, 2012 (18); Boyle, 2008 (10); Andreas, 2015 (25); Andrew, 2019 (32) |
Poisson regression | 2 | McCarthy, 2008 (9); Marcilio, 2013 (21) | |
Quadratic | 1 | Boyle, 2008 (10) | |
Nonlinear least square regression | 1 | Xu, 2013 (20) | |
Time series | Autoregressive integrated moving average (ARIMA) | 13 | Boyle, 2008 (10); Sun, 2009 (12); Jones, 2008 (8); Schweihler, 2009 (11); Xu, 2011 (16); Boyle, 2012 (18); Marcilio, 2013 (21); Kadri, 2014 (23); Afilal, 2016 (26); Carvalho-Silva, 2018 (29); Avishek, 2020 (36); Tahseen, 2019 (34); W.D. Lin, 2017 (28) |
Exponential smoothing (ES) | 5 | Jones, 2008 (8); Xu, 2011 (16); Boyle, 2012 (18); Bergs, 2014 (22); Rema, 2021 (40) | |
Other smoothing methods | 3 | Schweihler,2009 (11); Boyle, 2008 (10); Boyle, 2012 (18) | |
Artificial intelligence | Artificial neural networks (ANN) | 11 | Jones, 2008 (8); Aladag, 2012 (17); Xu, 2013 (20); Moustris, 2012 (19); Menke, 2014 (24); Fouzi, 2020 (37); Jiang, 2018 (30); Tahseen, 2019 (34); Khaldi, 2018 (31); Sudarshan, 2021 (41); Yousefi, 2020 (38) |
Time series regression | Generalised estimating equations (GEE) | 1 | Marcilio, 2013 (21) |
Multiple linear regression (MLR) with time series terms | 6 | Xu, 2011 (16); Jones, 2008 (8); Almeida, 2020 (35); Fralick, 2021 (39);Whitt, 2019 (33); Xu, 2016 (27) | |
Generalized additive model | 1 | Fralick, 2021 (39) |
Regression methods
Regression-based models assume that predicted outcomes can be explained by the interaction of predictor variables. Regression is a statistical method for estimating cause-and-effect relationships between a dependent variable and one or more independent variables. Regression-based models have been widely used for prediction and forecasting AED patient visits (5). Well-known regression-based methods include the generalised linear model, multiple linear regression (MLR), Poisson regression and the quadratic model. Twelve of the reviewed articles used regression methods to forecast AED patient visits. Of these, eight studies used MLR and four used other types of causal model. Two studies used the Poisson regression model, which is a log-linear model that assumes that the response variable (i.e., number of arrivals) follows a Poisson distribution. Marcilio et al. (21) regarded this method as a class of time series regression method and compared the forecast results with other time series regression models.
Time series methods
An important forecasting approach is time series analysis. A time series is defined as a collection of measurements obtained through repeated observations over time. Time series patient arrivals data are often captured in modern AED healthcare information systems. Time series analysis often takes account of the fact that data points over time may exhibit certain internal trends or patterns. Time series methods predict future outcome values based on historical values that incorporate the combined effects of long-term trends, cyclical changes and random effects (5). These methods are especially good at long-term forecasting. Typical time series models include moving average, exponential smoothing (ES) and autoregressive moving average (ARMA). Time series modelling was used in 21 of the reviewed studies, including 13 autoregressive integrated moving average (ARIMA) models, 5 ES models and 3 using other smoothing methods. An ARIMA model is a generalised form of an autoregressive moving average model, taking non-stationary data into account. Seasonal autoregressive integrated moving average (SARIMA) models expand the basic ARIMA model by adding seasonal autoregressive terms and are useful when modelling AED patient visits. Most of the reviewed time series models were univariate, which has been deemed useful for forecasting monthly health data (10). However, Kam et al. (13) recently performed a multivariate SARIMA analysis that included explanatory variables in the SARIMA model. Calendar and meteorological variables were included in a model of patient visits and the desired model accuracy was achieved.
Artificial intelligence methods
Artificial intelligence methods usually involve processing multiple tasks in an attempt to imitate intelligent human behaviour. Typically organised in layers with a number of interconnected nodes, artificial neural networks (ANNs) have widely been used to approximate unknown functions from a number of inputs. Usually called ‘black box’ methods, ANNs extract hidden information from the contributing variables to provide explanatory insight into the subject of interest. ANNs are presented as systems of interconnected ‘neurons’ which can compute values from inputs, and are widely used in machine learning and pattern recognition applications. The method was applied in 11 recent studies in which a three-stage method comprising identification of contributing variables, ANN development and relative importance computation was proposed to model patient arrivals (20). In recent years, ANN-based models for modelling patient arrival become more flexible with deeper layers and more complicated frameworks. For example, Sudarshan et al. employed a recurrent neural network with long short-term memory method in their forecast model, which obtained best performance in their comparative experiments (41).
Time series regression methods
Time series regression models take account of properties of both regression methods and time series methods. Unlike traditional regression methods that consider cross-sectional data, trends over time (such as seasonality) are often used to describe the behaviour of a time series. Historical trends and causal relationships are presented in one single model. Eight of the reviewed studies fell into this category. Jones et al. and Xu et al. (8,16) used time series explanatory variables and auto-correlated error terms to develop an MLR to model patient visits. Generalised estimating equations (GEE) are widely used for time series analysis. Marcilio et al. (21) developed GEE that considered proximate day patient visits as the autocorrelation term of dependence. Fralick et al. introduced a generalized additive model in their forecast method, and the output of generalized additive model was embedded in the final random forest model (39).
Selection of covariables
In this section, the selected covariables are systematically reviewed to demonstrate how AED patient arrival factors were selected for modelling patient visits. The variables in the reviewed studies can be categorised into calendar-based factors, meteorological factors and other related factors. Table 4 summarises the different factors used in each reviewed study. Calendar-based factors were widely used; 25 of 35 studies chose calendar-based factors, including time and date (such as hour of the day, day of the week or month of the year), public holiday, and before or after a public holiday, for their predictions. Calendar variables are well established as significantly correlated with patient arrivals. Marcilio et al. (21) conclude that adding information about ambient temperature in their models does not improve forecasting accuracy. They also find that that weekly seasonality was more dominant than monthly seasonality on daily AED patient visit variation. Ekström et al.’s study (25) shows that accurate predictions of ED visits can be made only using calendar data combined with Web site visits data, in which the model achieves competitive accuracy over studies based on regression models including only weather and calendar variables or time series models. Xu et al. (27) suggest the raw data of holiday factors might contain outliers and cause the inaccuracy. To eliminate these noises, the study adds a smoothing procedure to smooth patient visits in holidays.
Table 4
Study | Calendar-based | Meteorological | Other | ||||||||||||
---|---|---|---|---|---|---|---|---|---|---|---|---|---|---|---|
Date type | HD | Before /after HD | T | ΔT | H | P | Wind speed | AC | Extreme weather warning | Triage level | Influenza level | SI | |||
Tai et al., [2007] (7) | ▲ | ▲ | ▲ | ▲ | |||||||||||
Jones et al., [2008] (8) | ▲ | ▲ | ▲ | ▲ | |||||||||||
McCarthy et al., [2008] (9) | ▲ | ▲ | ▲ | ▲ | ▲ | ▲ | |||||||||
Boyle et al., [2008] (10) | ▲ | ||||||||||||||
Schweigler et al., [2009] (11) | ▲ | ||||||||||||||
Sun et al., [2009] (12) | ▲ | ▲ | ▲ | ▲ | ▲ | ▲ | |||||||||
Kam et al., [2010] (13) | ▲ | ▲ | ▲ | ▲ | ▲ | ▲ | ▲ | ||||||||
Wargon et al., [2010] (14) | ▲ | ▲ | ▲ | ||||||||||||
Chen et al., [2011] (15) | ▲ | ▲ | ▲ | ▲ | ▲ | ||||||||||
Xu et al., [2011] (16) | ▲ | ▲ | ▲ | ▲ | ▲ | ||||||||||
Aladag et al., [2012] (17) | |||||||||||||||
Boyle et al., [2012] (18) | ▲ | ▲ | ▲ | ||||||||||||
Moustris et al., [2012] (19) | ▲ | ▲ | ▲ | ▲ | |||||||||||
Xu et al., [2013] (20) | ▲ | ▲ | ▲ | ▲ | ▲ | ▲ | |||||||||
Marcilio et al., [2013] (21) | ▲ | ▲ | ▲ | ▲ | ▲ | ||||||||||
Bergs et al., [2014] (22) | |||||||||||||||
Kadri et al., [2014] (23) | ▲ | ||||||||||||||
Menke et al., [2014] (24) | ▲ | ▲ | ▲ | ||||||||||||
Ekström et al., [2015] (25) | ▲ | ▲ | |||||||||||||
Afilal et al., [2016] (26) | |||||||||||||||
Xu et al., [2016] (27) | ▲ | ▲ | ▲ | ▲ | ▲ | ||||||||||
Lin et al., [2017] (28) | ▲ | ▲ | |||||||||||||
Carvalho-Silva et al., [2018] (29) | |||||||||||||||
Jiang et al., [2018] (30) | ▲ | ▲ | ▲ | ▲ | ▲ | ▲ | ▲ | ▲ | ▲ | ||||||
Khaldi et al., [2018] (31) | |||||||||||||||
Ho, al. (2019] (32) | ▲ | ||||||||||||||
Whitt, Zhang, (2019] (33) | ▲ | ▲ | ▲ | ▲ | ▲ | ▲ | |||||||||
Jilani et al., [2019] (34) | ▲ | ||||||||||||||
Almeida et al., [2020] (35) | ▲ | ▲ | ▲ | ▲ | ▲ | ||||||||||
Choudhury et al., [2020] (36) | ▲ | ||||||||||||||
Harrou et al., [2020] (37) | ▲ | ||||||||||||||
Yousefi et al., [2020] (38) | ▲ | ▲ | ▲ | ▲ | |||||||||||
Fralick et al., [2021] (39) | ▲ | ▲ | ▲ | ▲ | ▲ | ▲ | |||||||||
Rema et al., [2021] (40) | |||||||||||||||
Sudarshan et al., [2021] (41) | ▲ | ▲ | ▲ |
HD, holiday; T, temperature; ΔT, change of temperature; H, humidity; P, precipitation; AC, air quality condition; SI, socioeconomic indicators.
Meteorological factors included maximum and minimum daily temperature, humidity, wind speed, air quality and general weather conditions. It is yet to be explained how these factors influence patient arrivals and to what extent. Sixteen of the 35 studies considered meteorological factors. Three of the studies concluded that some meteorological factors (i.e., temperature) are not significantly related to patient arrivals whereas others showed that meteorological factors influence total patient arrivals or patient arrivals of a certain triage level. Temperature is the most frequently used meteorological factor. Sun et al. (12) reported that increasing temperature lead to a remarkable increase in patient arrivals at a specific triage level but did not have the same effect on the total volume of patient arrivals. Kam et al. (13) also showed that there is a positive correlation between average daily temperature and patient arrivals. However, Marcilio et al. (21) reported a lag effect of temperature on patient arrivals, and the authors found that estimated effects of calendar variables did not change after controlling for the current temperature. Tai et al. (7) provided more details on the effect of temperature, and demonstrated that the change in temperature, rather than the absolute temperature, is a more sensitive marker for total AED patient arrivals. They also analysed the combined effect of absolute temperature and change in temperature on patient arrivals at different triage levels.
Apart from temperature, other meteorological factors, such as rainfall, wind speed and air quality, were also widely considered. Sun et al. (12) concluded that poor air quality significantly influenced patient arrivals positively or negatively depending on triage level. Xu et al. (16) analysed the influence of extreme weather (for instance, a yellow warning of rainstorms, and cold or hot weather warnings), and showed its remarkable effect on reducing patient arrivals.
Other variables included triage level, influenza indicators (16,20,21,30) and socioeconomic indicators (15,39). These factors are relatively uncommon in the models but were shown to be closely related to the volume of patient arrivals. Several studies developed multiple prediction models based on triage level. Overall, triage-based models perform well. It is notable that patients at the same triage level are less heterogeneous in terms of their arrival pattern and their underlying reasons for seeking a consultation, which implies a more homogeneous trend and seasonality in patient arrivals. Some special social event also plays a significant role in AED patient flow modelling. Yousefi et al. found that soccer match days have significant impacts on the number of daily patients’ visitors (38).
Prediction accuracy
This section details the accuracy of various forecasting models. A number of criteria are used to assess prediction model performance, including the percentage of variability (R2), the mean absolute percentage error (MAPE) and the root mean square error (RMSE). Thirteen of the reviewed articles reported model performance based on MAPE, which is defined as the percentage mean absolute difference between predicted values and measured values. Lower MAPE values indicate better model performance. As a single study may include a number of MAPE values based on different forecasting methods, for those studies that included more than one evaluation dataset for a single method, only the lowest MAPE is included in this meta-analysis. We stratified the reported performance by type of forecasting model and forecast time intervals.
Figures 2,3 compare the performance of the reviewed models using MAPE. Totally 34 of the 37 sets of data achieved an MAPE of less than 10%, and 13 of them achieved an MAPE of less than 5%. Monthly predictions generally performed better than daily and hourly predictions (except for one study with an MAPE of 12.39%). This finding generally agrees with Boyle et al.’s study (18) that shorter forecast time intervals increase prediction difficulty. Among the models with a monthly forecasting horizon, the MLR model developed by Boyle et al. (10) achieved the lowest MAPE (1.79%). Other simple smoothing models also achieved excellent MAPEs (around 2%) (10,18). All the monthly prediction models apart from one achieved an MAPE of less than 10%. The daily forecast models all achieved an MAPE of less than 12%. The eight ARIMA models reported MAPE values between 2.3% and 11.8% and among which (28) achieved the lowest MAPE of 2.3%. The MAPEs of the regression (causal) models ranged from 4.8–9.20%. The best performing model with daily prediction intervals was an ANN-based model with an MAPE of 2.63%. By comparing these two figures, we noticed that ANN-based model became more popular after year 2014, which indicates the rapid development of artificial intelligence-based algorithms in recent years. However, we cannot conclude that any prediction model consistently outperforms the others.
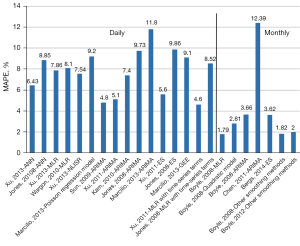
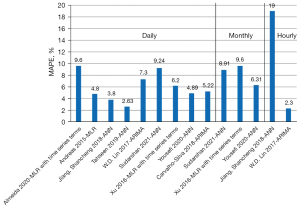
Discussion
This paper reviews the relevant literature on forecasting AED patient visits, in terms of modelling approach, choice of covariables, and prediction accuracy, according to the PRISMA guidelines. There are four major model categories: regression, time series, artificial intelligence and time series regression. Based on the results displayed in Figures 2,3, it is not clear if any method outperforms the others. The various models use different data sources (i.e., data are acquired from different AEDs in different countries), all AEDs are inherently different and different covariables may have different effects on patient arrivals. Certain factors may play a key role in one AED but not others (9). For instance, estimates of a single meteorological factor could vary widely across different geographical regions and even the commonly used calendar-based variables can influence forecasts to a different degree in different AEDs (14). When modelling patient arrivals, it is essential to understand the actual AED situation and carefully select relevant dominating factors and the most suitable modelling method. Local calibration is also important to ensure good estimates are obtained.
The various methods have different strengths, weaknesses and limitations in practice. Clinical statisticians should be aware of the properties of the different methods when applying them to the real AED context. For instance, cause-and-effect prediction models have the advantage of evaluating or describing the effect of a range of independent variables on the output (dependent) variable. Jones et al. (8) included both temporal and climatic variables in one MLR model and also examined the interaction of independent variables. The adaptive GLM method is able to re-compute/update regression coefficients for each forecasting interval. However, cause-and-effect based models often need large amounts of data to guarantee accurate estimates (21), so are rarely practical for short-term predictions (11). Other issues, including factor selection, overfitting, and multicollinearity, should also be considered.
Patient arrivals data can be ordered temporally for time series analysis. Time series models have proven success in capturing short-term patterns in historical data. Bergs et al. (22) used extended range exponential smoothing methods within a state-space framework that subsumes all exponential smoothing models. Seasonal ARMA models with external inputs and their variants (including ARX, ARIMAX, and MAX) are better able to capture the lag effect of interactive variables (8,10,12,13). Schweigler et al. (11) presented a SARIMA model with a high degree of accuracy. Box et al. (42) proposed a systematic method for acquiring appropriate p, d, and q values by analysing autocorrelation and partial autocorrelation functions.
Most time series approaches, such as the simple smoothing method (18), exponential smoothing (16), and SARIMA (21), only compare values of a single series at different points in time. Traditionally, time series models do not consider causal relationships. Incorporating time series terms into regression models could be useful when modelling AED arrivals as it would take account of the effects of seasonality and other contributing factors. For instance, patient arrivals can be approximated by a Poisson process. McCarthy, et al. (9) developed Poisson regression models to predict future patient arrivals based on three different types of independent variable (temporal, individual and climatic). Xu, et al. (16) established an MLR model with ARIMA terms for the residuals.
The use of artificial neural networks (ANNs), which are inspired by biological neutral networks, is a machine learning approach that uses systems of interconnected and interacting ‘neurons’. It is considered a nonlinear statistical data modelling tool for revealing complex relationships between inputs and outputs (8,20,24,30,41) evaluated the performance of ANN models in which both calendar and meteorological variables are included in the input layer. Some deep learning methods, such as deep neural networks (DNN), have received attention in other disciplines. Recently, the potential of big data analytics in healthcare has opened up the possibility of using more advanced and accurate approaches and algorithms for patient demand forecasts.
Calendar-based factors were common used among the reviewed studies. Patient arrivals show evident seasonal patterns, with significant fluctuations in the volume of patient arrivals across different days of the week (21) and months of the year (10). Some researches (8,9,13,14,21) found that the pattern of patient arrivals changed near holidays. It is difficult to draw a universal conclusion about whether a meteorological factor applies to all AEDs because climate is a regional variable and it can also produce different effects depending on the attitude of patients (13). For some diseases that are caused by a sudden change of climate, adding factors describing change in temperature to the model rather than its absolute value can optimise prediction accuracy (7). Few studies considered factors other than meteorological and calendar-based factors. Only one approach (15) explored socioeconomic indicators, finding that stock index fluctuations influenced patient arrivals. Although it is difficult and controversial to generalise this finding, it is worth exploring other independent variables that might be related to patient arrival patterns in real AED situations.
Several potential research directions are suggested. Data pre-processing has been regarded as an essential statistical means of understanding problems being investigated and potential contributing factors, such as trend, seasonality, cyclic oscillation, and so on. In most ANN-based studies pre-processing is omitted (17,24), which undermines the stability of the predictions. To address this issue, one ANN study (20) plotted a relational diagram of input and output variables based on Spearman’s rank correlation, and calculated the relative importance of input variables using four different methods. Few of the reviewed studies combined outcomes from more than one model to make predictions. Hibon et al. (43) studied the performance and reliability of 14 prediction methods, including combining models and individual models and concluded that it is more reliable in practice to combine forecasts than to select only from an individual forecasting model. Fralick et al. (39) assembled a generalized additive model, an auto regressive integrated moving average with Fourier transform, a feed forward neural network, a Holt Winters model, an exponential smoothing state space model, and a seasonal and trend decomposition using loess forecasting model in one framework, and the final output is generated by a random forest model (39). In fact, a growing number of combining prediction models have been proposed in recent years, and they have usually resulted in a good predictions, especially for time series exhibiting both linear and nonlinear patterns. For instance, Zhang et al. (44) combined ARIMA and ANN models using three well-known datasets and found that the performance was better than either of the individual models. Chen et al. (45) proposed a combination model incorporating a SARIMA model and support vector machines (SVM) for predicting production values in the manufacturing industry. Zhou et al. (46) used a combined grey and Box-Jenkins ARMA model to forecast the gyro drift in navigational systems. It is worth exploring the possibilities of using multiple models to predict the volume of patient arrivals in AED.
Risk of bias
As respect to the review level, we choose Medline, ScienceDirect and Scopus database to conduct paper extraction, which may cover the most studies focusing on patient arrival prediction in AED. We only include publish journal papers with formal issue or volume number, and grey literature (e.g., proceedings, dissertations and theses) or unpublished papers (e.g., pre-print articles in arXiv archive) are not considered in the literature search process. Another possible bias of completeness is that the key word “patient flow” is not included in the search string, this might bring risk of excluding a few target papers in which only “patient flow” is used to describe the meaning of patient visits. However, if we added this key word in the search string, numbers of irrelevant papers, which are aimed at quantifying AED crowding levels or optimizing the service level of AED, are included in the initial search result. These data of noises need to be excluded via full text examination (i.e., the second step of exclusion), which cannot be achieved with limited reviewers.
As respect to the study and outcome level, the MAPE of different predictive models shown in Figures 2,3 cannot reflect their absolute performance or property on this specific task. As is indicated in Prediction accuracy, different studies conduct numeric experiments on different datasets, and most datasets are not publicly available. It is impossible to judge the advantage and weakness of these predictive models with results shown in Figures 2,3, but the results can reflect a rough interval of MAPE achieved by various models in this task. In addition, studies using other metrics except MAPE are not included in Figures 2,3, and those studies reporting more than one results for a single method, only the lowest MAPE is included in this meta-analysis.
Conclusions
Healthcare information systems give access to accurate information about AED patient arrivals. In light of the opportunities presented by big data and healthcare analytics, this study systemically reviewed AED demand prediction studies. We analysed and compared the use of different prediction models, selection of factors and model performance. We find that the use of artificial intelligence-based model has risen in recent years, from the view of predictive model selection. The calendar-based factors are most commonly used compared with other types of dependent variables. However, one general finding is that models need to be locally derived given the variation across countries and health systems with no clear consistent predictors. Although it is impossible to judge the advantage and weakness of involved predictive models with results obtained from different dataset, ANN-based machine learning models have shown great promise with high model capacity and flexibility. We conducted this systematic literature review and present our methods, results and discussion in accordance with the PRISMA statement.
Acknowledgments
Funding: This work was supported in part by the National Nature Science and Foundation of China under Grant No. 71801031 and 52105079, the Guangdong Basic and Applied Basic Research Foundation of China project “Research on dermatosis automatic diagnosis system based on multi-type of medical image” under Grant No. 2019A1515011962.
Footnote
Reporting Checklist: The authors have completed the PRISMA reporting checklist. Available at https://qims.amegroups.com/article/view/10.21037/qims-22-268/rc.
Conflicts of Interest: All authors have completed the ICMJE uniform disclosure form (available at https://qims.amegroups.com/article/view/10.21037/qims-22-268/coif). The authors have no conflicts of interest to declare.
Ethical Statement: The authors are accountable for all aspects of the work in ensuring that questions related to the accuracy or integrity of any part of the work are appropriately investigated and resolved.
Open Access Statement: This is an Open Access article distributed in accordance with the Creative Commons Attribution-NonCommercial-NoDerivs 4.0 International License (CC BY-NC-ND 4.0), which permits the non-commercial replication and distribution of the article with the strict proviso that no changes or edits are made and the original work is properly cited (including links to both the formal publication through the relevant DOI and the license). See: https://creativecommons.org/licenses/by-nc-nd/4.0/.
References
- Komarudin, Guerry MA, De Feyter T, Berghe GV. The roster quality staffing problem - A methodology for improving the roster quality by modifying the personnel structure. European Journal of Operational Research 2013;230:551-62. [Crossref]
- Wise S, Fry M, Duffield C, Roche M, Buchanan J. Ratios and nurse staffing: the vexed case of emergency departments. Australas Emerg Nurs J 2015;18:49-55. [Crossref] [PubMed]
- Sinreich D, Jabali O, Dellaert NP. Reducing emergency department waiting times by adjusting work shifts considering patient visits to multiple care providers. Iie Transactions 2012;44:163-80. [Crossref]
- Erdem E, Qu X, Shi J. Rescheduling of elective patients upon the arrival of emergency patients. Decision Support Systems 2012;54:551-63. [Crossref]
- Wargon M, Guidet B, Hoang TD, Hejblum G. A systematic review of models for forecasting the number of emergency department visits. Emerg Med J 2009;26:395-9. [Crossref] [PubMed]
- Moher D, Liberati A, Tetzlaff J, Altman DG. PRISMA Group. Preferred reporting items for systematic reviews and meta-analyses: the PRISMA statement. PLoS Med 2009;6:e1000097. [Crossref] [PubMed]
- Tai CC, Lee CC, Shih CL, Chen SC. Effects of ambient temperature on volume, specialty composition and triage levels of emergency department visits. Emerg Med J 2007;24:641-4. [Crossref] [PubMed]
- Jones SS, Thomas A, Evans RS, Welch SJ, Haug PJ, Snow GL. Forecasting daily patient volumes in the emergency department. Acad Emerg Med 2008;15:159-70. [Crossref] [PubMed]
- McCarthy ML, Zeger SL, Ding R, Aronsky D, Hoot NR, Kelen GD. The challenge of predicting demand for emergency department services. Acad Emerg Med 2008;15:337-46. [Crossref] [PubMed]
- Boyle J, Wallis M, Jessup M, Crilly J, Lind J, Miller P, Fitzgerald G. Regression forecasting of patient admission data. Annu Int Conf IEEE Eng Med Biol Soc 2008;2008:3819-22. [PubMed]
- Schweigler LM, Desmond JS, McCarthy ML, Bukowski KJ, Ionides EL, Younger JG. Forecasting models of emergency department crowding. Acad Emerg Med 2009;16:301-8. [Crossref] [PubMed]
- Sun Y, Heng BH, Seow YT, Seow E. Forecasting daily attendances at an emergency department to aid resource planning. BMC Emerg Med 2009;9:1. [Crossref] [PubMed]
- Kam HJ, Sung JO, Park RW. Prediction of Daily Patient Numbers for a Regional Emergency Medical Center using Time Series Analysis. Healthc Inform Res 2010;16:158-65. [Crossref] [PubMed]
- Wargon M, Casalino E, Guidet B. From model to forecasting: a multicenter study in emergency departments. Acad Emerg Med 2010;17:970-8. [Crossref] [PubMed]
- Chen CF, Ho WH, Chou HY, Yang SM, Chen IT, Shi HY. Long-term prediction of emergency department revenue and visitor volume using autoregressive integrated moving average model. Comput Math Methods Med 2011;2011:395690. [Crossref] [PubMed]
- Xu M, Wong TC, Chin KS, Wong SY, Tsui KL, Ieee, editors. Modeling Patient Visits to Accident and Emergency Department in Hong Kong. IEEE International Conference on Industrial Engineering and Engineering Management (IEEM); 2011. 2011 Dec 06-09; Singapore, Singapore, 2011.
- Aladag CH, Aladag S. Forecasting the number of outpatient visits with different activation functions. Advances in Time Series Forecasting, 2012. p: 26-33.
- Boyle J, Jessup M, Crilly J, Green D, Lind J, Wallis M, Miller P, Fitzgerald G. Predicting emergency department admissions. Emerg Med J 2012;29:358-65. [Crossref] [PubMed]
- Moustris KP, Douros K, Nastos PT, Larissi IK, Anthracopoulos MB, Paliatsos AG, Priftis KN. Seven-days-ahead forecasting of childhood asthma admissions using artificial neural networks in Athens, Greece. Int J Environ Health Res 2012;22:93-104. [Crossref] [PubMed]
- Xu M, Wong TC, Chin KS. Modeling daily patient arrivals at Emergency Department and quantifying the relative importance of contributing variables using artificial neural network. Decision Support Systems 2013;54:1488-98. [Crossref]
- Marcilio I, Hajat S, Gouveia N. Forecasting daily emergency department visits using calendar variables and ambient temperature readings. Acad Emerg Med 2013;20:769-77. [Crossref] [PubMed]
- Bergs J, Heerinckx P, Verelst S. Knowing what to expect, forecasting monthly emergency department visits: A time-series analysis. Int Emerg Nurs 2014;22:112-5. [Crossref] [PubMed]
- Kadri F, Harrou F, Chaabane S, Tahon C. Time series modelling and forecasting of emergency department overcrowding. J Med Syst 2014;38:107. [Crossref] [PubMed]
- Menke NB, Caputo N, Fraser R, Haber J, Shields C, Menke MN. A retrospective analysis of the utility of an artificial neural network to predict ED volume. Am J Emerg Med 2014;32:614-7. [Crossref] [PubMed]
- Ekström A, Kurland L, Farrokhnia N, Castrén M, Nordberg M. Forecasting emergency department visits using internet data. Ann Emerg Med 2015;65:436-442.e1. [Crossref] [PubMed]
- Afilal M, Yalaoui F, Dugardin F, Amodeo L, Laplanche D, Blua P, editors. Emergency department flow: A new practical patients classification and forecasting daily attendance. 8th IFAC Conference on Manufacturing Modelling, Management and Control (MIM); 2016. 2016 Jun 28-30; Troyes, France, 2016.
- Xu Q, Tsui KL, Jiang W, Guo H. A Hybrid Approach for Forecasting Patient Visits in Emergency Department. Quality and Reliability Engineering International 2016;32:2751-9. [Crossref]
- Lin WD, Chia L, editors. Combined Forecasting of Patient Arrivals and Doctor Rostering Simulation Modelling for Hospital Emergency Department. IEEE International Conference on Industrial Engineering and Engineering Management (IEEE IEEM); 2017 2017 Dec 10-13; Singapore, Singapore, 2017.
- Carvalho-Silva M, Monteiro MTT, de Sa-Soares F, Doria-Nobrega S. Assessment of forecasting models for patients arrival at Emergency Department. Operations Research for Health Care 2018;18:112-8. [Crossref]
- Jiang S, Chin KS, Tsui KL. A universal deep learning approach for modeling the flow of patients under different severities. Comput Methods Programs Biomed 2018;154:191-203. [Crossref] [PubMed]
- Khaldi R, El Afia A, Chiheb R, editors. Forecasting of weekly patient visits to emergency department: real case study. 2nd International Conference on Intelligent Computing in Data Sciences (ICDS); 2018. 2019 Oct 03-05; Fez, Morocco, 2019.
- Ho AFW, To BZYS, Koh JM, Cheong KH. Forecasting Hospital Emergency Department Patient Volume Using Internet Search Data. IEEE Access 2019;7:93387-95.
- Whitt W, Zhang X. Forecasting arrivals and occupancy levels in an emergency department. Operations Research for Health Care 2019;21:1-18. [Crossref]
- Jilani T, Housley G, Figueredo G, Tang PS, Hatton J, Shaw D. Short and Long term predictions of Hospital emergency department attendances. Int J Med Inform 2019;129:167-74. [Crossref] [PubMed]
- Almeida HS, Sousa M, Mascarenhas I, Russo A, Barrento M, Mendes M, Nogueira P, Trigo R. The Dynamics of Patient Visits to a Public Hospital Pediatric Emergency Department: A Time-Series Model. Pediatr Emerg Care 2022;38:e240-5. [Crossref] [PubMed]
- Choudhury A, Urena E. Forecasting hourly emergency department arrival using time series analysis. British Journal of Health Care Management 2020;26:34-43. [Crossref]
- Harrou F, Dairi A, Kadri F, Sun Y. Forecasting emergency department overcrowding: A deep learning framework. Chaos Solitons Fractals 2020;139:110247. [Crossref] [PubMed]
- Yousefi M, Yousefi M, Fathi M, Fogliatto FS. Patient visit forecasting in an emergency department using a deep neural network approach. Kybernetes 2020;49:2335-48. [Crossref]
- Fralick M, Murray J, Mamdani M. Predicting emergency department volumes: A multicenter prospective study. Am J Emerg Med 2021;46:695-7. [Crossref] [PubMed]
- Rema V, Sikdar K, editors. Time Series Modelling and Forecasting of Patient Arrivals at an Emergency Department of a Select Hospital. Recent Trends in Signal and Image Processing; 2021 2021//; Singapore: Springer Singapore.
- Sudarshan VK, Brabrand M, Range TM, Wiil UK. Performance evaluation of Emergency Department patient arrivals forecasting models by including meteorological and calendar information: A comparative study. Comput Biol Med 2021;135:104541. [Crossref] [PubMed]
- Box GEP, Jenkins G. Time series analysis, forecasting and control. Time series analysis, forecasting and control. 1970.
- Hibon M, Evgeniou T. To combine or not to combine: selecting among forecasts and their combinations. International Journal of Forecasting 2005;21:15-24. [Crossref]
- Zhang GP. Time series forecasting using a hybrid ARIMA and neural network model. Neurocomputing 2003;50:159-75. [Crossref]
- Chen KY, Wang CH. A hybrid SARIMA. and support vector machines in forecasting the production values of the machinery industry in Taiwan. Expert Systems with Applications 2007;32:254-64. [Crossref]
- Zhou ZJ, Hu CH. An effective hybrid approach based on grey and ARMA for forecasting gyro drift. Chaos Solitons & Fractals 2008;35:525-9. [Crossref]