Soft tissue sarcoma: correlation of dynamic contrast-enhanced magnetic resonance imaging features with HIF-1α expression and patient outcomes
Introduction
Soft tissue sarcomas (STS) are highly heterogeneous, malignant tumors originating from mesenchymal components (1). Due to the high mortality and recurrence rates, researchers have focused on evaluating the relationship between the internal microenvironmental state and patient clinical outcomes (2-5). Several studies have reported that the hypoxic microenvironment is essential for generating adverse events, such as high recurrence rate and high radiotherapy resistance, in the sarcoma population (6,7). Among them, activation of hypoxia-inducible factor 1-alpha (HIF-1α) is a crucial process in mediating the adaptive cellular response to the hypoxic microenvironment. The prognostic relevance of HIF-1α expression has been demonstrated in various malignant solid tumors, including hepatocellular carcinoma, glioma, and breast cancer (8-10). Recently, high HIF-1α expression in STS has been associated with higher mortality. Nyström et al. reported that the expression of HIF-1α was associated with an independently predicted shorter metastasis-free survival in high-grade STS (6). Several studies of efficacy assessment have also confirmed that high HIF-1α status might lead to higher drug resistance in tumors (11,12). Thus, assessing the hypoxic level and monitoring HIF-1α expression in STS facilitates determination of the prognosis and treatment response.
Accurate HIF-1α profiling relies on the genetic analysis of surgical tissue. However, clinicians cannot preoperatively assess HIF-1α levels in STS patients to predict patient survival. In addition, surgical resection is not a viable treatment for patients with advanced STS. Therefore, it is critical to explore a non-invasive method to assess HIF-1α expression in STS.
Magnetic resonance imaging (MRI) has been widely used for imaging-pathology control studies of malignant tumors due to its high soft-tissue resolution and multi-sequence imaging. Non-contrast conventional MRI sequences are helpful in predicting STS histological information. It has been reported that necrosis, heterogeneity, and peritumoral enhancement of STS are associated with grade III tumors, metastasis-free survival, and overall survival (OS) (13). However, non-contrast MRI has not been reported to predict the oxygenation status of STS. Instead, quantitative functional MRI methods have been used to assess tumor oxygenation status (14-16). Among these methods, quantitative parameters generated by pharmacokinetic modeling with dynamic contrast-enhanced MRI (DCE-MRI) have correlated significantly with tumor hypoxia. However, the reflection of quantitative parameters on HIF-1α expression levels has remained controversial among the different histological types of tumors. Some researchers have reported positive correlations between HIF-1α and DCE perfusion parameters in cervical and ovarian cancer, while other studies have arrived at opposing conclusions (17-20). In studies of STS, it has been confirmed that quantitative DCE-MRI parameters can distinguish between benign and malignant lesions and the histopathological grade of tumors (21-23). This implies that DCE-MRI has the potential to reflect the microstructure of complex types of STS by the level of perfusion.
This study aimed to analyze the relationship between DCE-MRI features and HIF-1α expression in STS and explore the optimal threshold for quantitative parameters to diagnose the hypoxic tumor state. Additionally, the effect of different oxygenation states on patient survival was verified. We hypothesized that there might be positive correlations between quantitative DCE-MRI parameters and that HIF-1α expression and MRI features associated with high HIF-1α expression might be associated with poor prognosis. We present the following article in accordance with the STARD reporting checklist (available at https://qims.amegroups.com/article/view/10.21037/qims-22-75/rc).
Methods
This prospective study was conducted in accordance with the Declaration of Helsinki (as revised in 2013). The study was approved by the Health Sciences Institutional Review Board (HIRB) of Huashan Hospital, Fudan University, and informed consent was provided by all individual participants.
Study population
From January 2018 to January 2019, 150 patients with suspected soft tissue tumors were recruited among patients attending Huashan Hospital, Fudan University for treatment. All patients were recruited using the convenience sampling method. All participants underwent 3.0 Tesla (3.0T) MRI, including conventional MRI protocol and DCE-MRI sequences. The histopathological diagnosis based on the examination of resected surgical specimens was in accordance with the World Health Organization (WHO) Soft Tissue Tumor Classification (2020 version) (24). The following exclusion criteria were used in this study: (I) benign or intermediate soft tissue tumors; (II) MRI images with severe artifacts; (III) patients who underwent only puncture biopsy; (IV) history of previous treatment; and (V) interval between MRI examination and surgery of more than 2 weeks.
MRI examination protocol
All MRI examinations were performed using a 3.0T MRI scanner (Verio; Siemens Healthcare, Erlangen, Germany). The appropriate coil and body position were selected according to the site and size of the tumor. Conventional MRI protocols included axial and coronal fast spin-echo T1-weighted image [repetition time (TR)/echo time (TE) =445–883/13–19 ms; slice thickness =3–7 mm] and fat suppression T2-weighted image (T2WI) (TR/TE =3,002–5,428/70–82 ms, slice thickness =3–7 mm) in the axial and sagittal planes.
We performed DCE-MRI using an acquisition with a volumetric interpolated breath-hold examination (VIBE) sequence based on a three-dimensional (3D), T1-weighted, fast-spoiled gradient-echo technique. The parameters were as follows: flip angle =3°, 6°, 9°, and 12°; layer thickness =3–7 mm; layer spacing =0 mm; TR =5.0 ms; TE =1.7 ms; field of view (FOV) and matrix size were adjusted according to the lesion size. Three cycles were pre-scanned before injecting contrast material to calculate the baseline T1 maps. From the fourth cycle, dynamic enhancement scans were performed using a high-pressure syringe injection of gadoterate meglumine (Dotarem®; Guerbet, Villepinte, France) at a rate of 2.5 mL/s and a dose of 0.1 mmol/kg, followed by a 20 mL physiological saline flush delivered at a flow rate of 2 mL/s. A total of 40 cycles were scanned for 8 s each. The total acquisition time was 320 s.
MR image analysis
Two readers with 12 and 23 respective years of experience in musculoskeletal radiology who were blinded to the patients’ clinical information recorded the following tumor signs: (I) pain; (II) location, defined as the depth of the tumor relative to the superficial fascia; (III) maximum tumor diameter, defined as the maximum distance of the tumor measurable in each imaging plane; (IV) growth rate, classified as slow growth or rapid growth in a short period of time; (V) peritumoral capsule sign, defined as the capsule of the tumor itself and/or a pseudo-capsule with low peritumor signal; (VI) T2WI tumor heterogeneity, with homogeneity or less than 50% heterogeneity classified as slight heterogeneity and more than 50% heterogeneity classified as high heterogeneity; (VII) peritumoral edema, defined as a fluid-like, high signal in the peritumoral area on T2WI that is clearly distinguishable from the tumor entity; (VIII) peritumoral enhancement, defined as contrast enhancement beyond the weighted tumor border on T1-weighted imaging after gadolinium chelate injection; (IX) necrosis, defined as a high signal on T2WI without enhancement on DCE-MRI images after gadolinium chelate injection, categorized as less than 50% necrotic area and more than 50% necrotic area; (X) configuration, categorized as multilobulated and non-multilobulated tumors; (XI) tail-like pattern, defined as thick tendon membrane enhancement around the tumor in T1-weighted imaging after gadolinium chelate injection; (XII) bone invasion, categorized as absent and present; and (XIII) vessel/nerve invasion and/or encasement.
For data extraction, DCE-MRI images were transferred to the Siemens imaging workstation (Syngo v. B17; Siemens Healthineers, Forchheim, Germany). The Tissue 4D software was used for data post-processing. The extended Tofts linear hemodynamic model and the population-averaged arterial input function were chosen to estimate quantitative parameters. For each lesion, the region of interest (ROI) was drawn as large as possible on the highest perfusion area of the Ktrans map (with red representing presumed hyperperfusion areas and yellow or blue representing relatively low-perfusion areas) on the slice depicting the tumor’s largest diameter. Areas of cystic degeneration, necrosis, and calcification were actively avoided. Finally, time-signal intensity curve (TIC) types and Ktrans, Kep, and Ve values were obtained separately for each lesion. The TIC types were classified into four types. Type I was flat, with a curve alignment similar to that of the muscle tissue curve and without a significant upward trend; type II was slow-rising, with a continuous rise in the curve and no clear peak; type III was a fast-rising and then flat type, in which the signal intensity rose significantly in the early stage of strengthening and then remained relatively stable; and type IV was a fast-rising and fast-decreasing type, in which the signal intensity rose significantly at the early stage of reinforcement and reached its peak rapidly, and then decreased rapidly. The Ktrans represents the volume transfer constant; Kep represents the flux rate constant between the extravascular, extracellular space and plasma; and Ve represents the volume of the extravascular, extracellular space per unit volume of tissue.
Pathological and immunohistochemical evaluation
Histopathological, histological grade diagnosis, and immunohistochemical staining analysis of each lesion were reviewed by pathologists with more than 10 years of experience who were blinded to participant MRI and clinical information. Formalin-fixed and paraffin-embedded tissues were stained with hematoxylin and eosin for histopathological observation and analyzed by immunohistochemistry using antibodies against HIF-1α [1:300, phosphate-buffered saline (PBS)]. Five FOVs were randomly selected for each section, and 200 cells were counted in each FOV. After that, the level of expression of HIF-1α was scored according to the percentage of positive cells: 0 points, 0–25%; 1 point, 26–50%; 2 points, 51–75%; 3 points, 76–99%; and 4 points, 100%. Staining intensity was scored as: 0 points, no staining; 1 point, light yellow; 2 points, dark yellow; and 3 points, dark brown. A tumor was allocated to the high-expression group only when HIF-1α positive cells were more than 50% and staining intensity was ≥2 points; otherwise, it was allocated to the low-expression group.
Postoperative follow-up
Routine follow-up included clinical physical examination and chest computed tomography (CT; with a 1 mm thick reconstruction). This was done every 3 months for 2 years and then every 6 months for 1 year. The local assessment was performed below the MRI examination in case of abnormal findings. The OS was defined as the time from the initial pathological diagnosis to death.
Statistical analysis
The software SPSS 28.0 (IBM Corp., Armonk, NY, USA) was used for all statistical analyses. Interobserver agreements between the two observers for conventional and DCE-MRI features were assessed using the intraclass correlation coefficient (ICC) scale: ICC <0.50, poor agreement; 0.50–0.75, moderate agreement; 0.76–0.90, good agreement; and 0.91–1.00, excellent agreement. Pearson and Spearman analyses were used to determine the correlation between the DCE-MRI parameters and HIF-1α-positive cell expression. Differences in MRI features between high- and low-expression groups were assessed. An independent samples t-test was used for continuous normal distribution variables. The chi-square test and Mann-Whitney U-test were used for categorical and continuous non-normal distribution variables. Receiver operating characteristic (ROC) curves were used for analyzing statistically significant DCE-MRI parameters. The cut-off value, area under the curve (AUC), sensitivity, specificity, accuracy, positive predictive value (PPV), and negative predictive value (NPV) were obtained, respectively. Cox proportional hazard models were used to explore the univariate association between OS and MRI features. Survival curves were plotted using the Kaplan-Meier method. A P value of 0.05 was considered statistically significant.
Results
Figure 1 illustrates the flow chart of the participant recruitment process. Ultimately, 71 patients were included in the final analysis, including 40 males (mean age 58 years; range, 35 to 77 years) and 31 females (mean age 61 years; range, 29 to 82 years). There was only one lesion in each participant. Of the 71 lesions included in this study, 34 were in the low-expression group, and 37 were in the high-expression group. A total of 21 lesions were located in the thigh, 11 in the calf, 8 in the knee, 9 in the shoulder, 12 in the forearm, 6 in the upper arm, and 4 in the back. The expression of HIF-1α-positive cells ranged from 10% to 90%. Among the 71 included patients, 23 (32.4%) had grade I STS, 33 (46.5%) had grade II STS, and 15 (21.1%) had grade III STS. The expression of HIF-1α exhibited a moderately positive correlation with histological grade (r=0.512, P=0.012). The distribution of histological types in the two groups is demonstrated in Table S1.
Interobserver agreement
The interobserver agreement between the two readers for conventional MRI features ranged from good to excellent (Table 1). The ICC values ranged from 0.815 to 0.927. The percentage coefficient of variation (CV%) ranged from 3.4% to 8.6%. Measurement of DCE-MRI features showed the ICC ranging from 0.927 to 0.934 and the CV% ranging from 3.4% to 4.8%.
Table 1
MRI features | ICC (95% CI) | CVs (95% CI) (%) |
---|---|---|
Location | 0.918 (0.835 to 0.946) | 5.1 (2.6 to 7.9) |
Maximum tumor diameter | 0.924 (0.874 to 0.961) | 4.6 (2.2 to 7.8) |
Envelope | 0.854 (0.772 to 0.913) | 6.6 (3.4 to 9.1) |
T2WI tumor heterogeneity | 0.843 (0.724 to 0.895) | 6.9 (3.2 to 9.5) |
Peritumoral edema | 0.891 (0.821 to 0.953) | 5.7 (2.8 to 8.1) |
Peritumoral enhancement | 0.905 (0.732 to 0.932) | 5.3 (2.5 to 7.8) |
Necrosis | 0.815 (0.751 to 0.887) | 8.6 (4.0 to 11.3) |
Configuration | 0.921 (0.882 to 0.951) | 7.5 (3.4 to 9.1) |
Tail-like pattern | 0.927 (0.851 to 0.981) | 4.4 (2.4 to 7.3) |
Bone invasion | 0.843 (0.738 to 0.916) | 6.8 (3.1 to 9.6) |
Vessel invasion and/or encasement | 0.826 (0.782 to 0.843) | 8.1 (4.2 to 10.6) |
Nerve invasion and/or encasement | 0.842 (0.775 to 0.914) | 7.1 (3.9 to 9.5) |
TIC curve types | 0.927 (0.891 to 0.962) | 4.3 (1.9 to 7.2) |
Ktrans | 0.921 (0.834 to 0.965) | 4.8 (2.1 to 7.5) |
Kep | 0.934 (0.892 to 0.987) | 3.4 (2.1 to 6.7) |
Ve | 0.931 (0.861 to 0.971) | 3.7 (1.8 to 6.6) |
MRI, magnetic resonance imaging; ICC, intraclass correlation coefficient; CVs, coefficients of variation; CI, confidence interval; T2WI, T2-weighted image; TIC, time-signal intensity curve; Ktrans, the volume transfer constant; Kep, the flux rate constant between the extravascular, extracellular space and plasma; Ve, the volume of the extravascular, extracellular space per unit volume of tissue.
Univariate analysis of MRI features in HIF-1α high- and low-expression groups
Table 2 shows the univariate analysis of the differences in MRI features between the HIF-1α high- and low-expression groups. With respect to conventional MRI features, T2WI tumor heterogeneity, peritumor enhancement, necrotic area, and configuration were significantly different between the groups (P<0.05). Specifically, features of high T2WI heterogeneity, peritumoral enhancement, a necrotic area of 50%, and multilobulated tumors were more prone to occur in the HIF-1α high-expression group.
Table 2
Characteristics | Low expression | High expression | P value |
---|---|---|---|
Gender | 0.686 | ||
Male | 41 (14/34) | 46 (17/37) | |
Female | 59 (20/34) | 54 (20/37) | |
Age | 0.242 | ||
<50 years old | 32 (11/34) | 51 (17/37) | |
≥50 years old | 68 (23/34) | 49 (20/37) | |
Pain | 0.877 | ||
Absent | 56 (19/34) | 54 (20/37) | |
Present | 44 (15/34) | 46 (17/37) | |
Location | 0.306 | ||
Deep | 50 (17/34) | 41 (15/37) | |
Superficial | 35 (12/34) | 38 (14/37) | |
Deep and superficial | 15 (5/34) | 21 (8/37) | |
Maximum tumor diameter | 0.219 | ||
<5 cm | 41 (14/34) | 19 (7/37) | |
5–10 cm | 32 (11/34) | 49 (18/37) | |
>10 cm | 27 (9/34) | 32 (12/37) | |
Growth rate | 0.580 | ||
Slow | 47 (16/34) | 41 (15/37) | |
Fast | 53 (18/34) | 59 (22/37) | |
Peritumoral capsule sign | 0.451 | ||
Absent | 71 (24/34) | 78 (29/37) | |
Present | 29 (10/34) | 22 (8/37) | |
T2WI tumor heterogeneity | 0.044* | ||
Slightly heterogeneous | 62 (21/34) | 38 (14/37) | |
Highly heterogeneous | 38 (13/34) | 62 (23/37) | |
Peritumoral edema | 0.542 | ||
Absent | 44 (15/34) | 51 (19/37) | |
Present | 56 (19/34) | 49 (18/37) | |
Peritumoral enhancement | 0.024* | ||
Absent | 65 (22/34) | 38 (14/37) | |
Present | 35 (12/34) | 62 (23/37) | |
Necrosis | 0.002* | ||
<50% necrosis | 85 (29/34) | 41 (15/37) | |
≥50% necrosis | 15 (5/34) | 59 (22/37) | |
Configuration | 0.032* | ||
Non-multilobulated | 74 (25/34) | 43 (16/37) | |
Multilobulated | 26 (9/34) | 57 (21/37) | |
Tail-like pattern | 0.649 | ||
Absent | 65 (22/34) | 59 (22/37) | |
Present | 35 (12/34) | 41 (15/37) | |
Bone invasion | 0.260 | ||
Absent | 79 (27/34) | 68 (25/37) | |
Present | 21 (7/34) | 32 (12/37) | |
Vessel invasion and/or encasement | 0.627 | ||
Absent | 59 (20/34) | 57 (21/37) | |
Present | 41 (14/34) | 43 (16/37) | |
Nerve invasion and/or encasement | 0.078 | ||
Absent | 56 (19/34) | 76 (28/37) | |
Present | 44 (15/34) | 24 (9/37) | |
TIC curve types | 0.188 | ||
I | 15 (5/34) | 8 (3/37) | |
II | 35 (12/34) | 27 (10/37) | |
III | 35 (12/34) | 38 (14/37) | |
IV | 15 (5/34) | 27 (10/37) | |
Ktrans(min−1), mean ± SD | 0.210±0.058 | 0.311±0.091 | <0.001* |
Kep(min−1), mean ± SD | 0.444±0.300 | 0.896±0.656 | 0.001* |
Ve (min−1), mean ± SD | 0.528±0.247 | 0.560±0.253 | 0.596 |
Data are percentages, with the ratio between the number of patients with a given feature and the number of patients with analyzable features in parentheses, if not otherwise specified. *, statistically significant at P value <0.05. MRI, magnetic resonance imaging; T2WI, T2-weighted image; TIC, time-signal intensity curve; HIF-1α, hypoxia-inducible factor 1-alpha; Ktrans, the volume transfer constant; Kep, the flux rate constant between the extravascular, extracellular space and plasma; Ve, the volume of the extravascular, extracellular space per unit volume of tissue; SD, standard deviation.
Regarding DCE-MRI parameters, the high-expression group showed significantly higher Ktrans and Kep values than the low-expression group. No significant differences in TIC curve types and Ve values were observed between the low- and high-expression groups. Figures 2,3 show typical examples of DCE-MRI maps that illustrate these trends in the low- and high-expression groups. The ROC analysis (Table 3, Figure 4) indicated high specificity (93.9%) of the Ktrans value in predicting HIF-1α high-expression; however, the Kep value provided the best performance in diagnostic sensitivity (84.2%).
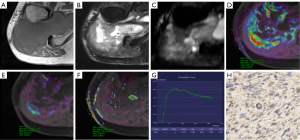
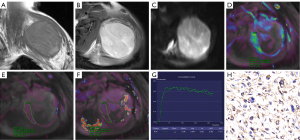
Table 3
Parameters | AUC (95% CI) | Cut-off value | Sensitivity | Specificity | Accuracy | PPV | NPV |
---|---|---|---|---|---|---|---|
Ktrans | 0.823 (0.726 to 0.921) | 0.262 | 60.5% | 93.9% | 76.1% | 92.0% | 67.4% |
Kep | 0.776 (0.664 to 0.887) | 0.455 | 84.2% | 66.7% | 76.1% | 74.4% | 78.6% |
ROC, receiver operating characteristic; AUC, area under the curve; CI, confidence interval; PPV, positive predictive value; NPV, negative predictive value; Ktrans, the volume transfer constant; Kep, the flux rate constant between the extravascular, extracellular space and plasma.
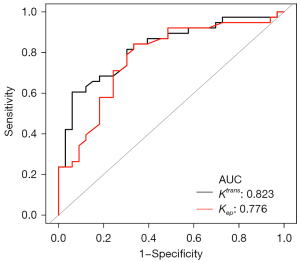
The correlation coefficient analysis revealed that the Ktrans value showed the most significant positive correlation with HIF-1α expression (r=0.705, P<0.001). There was a moderate positive correlation between the Kep value and HIF-1α expression (r=0.543, P<0.001), yet no correlation was found between the Ve value and HIF-1α expression (r=0.123, P=0.308).
Multivariate Cox proportional analysis of OS
Participants were followed up for 6–36 months. There were 48 survivors and 23 deaths. The 3-year survival probability for this series was 0.68 for OS. The variables that differed significantly between the HIF-1α high- and low-expression groups were included in a multifactorial Cox regression analysis (Table 4). In the final model, features of necrosis greater than 50%, multilobulation, and Ktrans values greater than 0.262 min−1 were strongly associated with a higher risk of death. Survival analyses are summarized in Figure 5.
Table 4
MRI features | HR (95% CI) | P value |
---|---|---|
T2WI tumor heterogeneity | ||
Slightly heterogeneous | 1.00 (referent) | |
Highly heterogeneous | 0.92 (0.56 to 1.52) | 0.757 |
Peritumoral enhancement | ||
Absent | 1.00 (referent) | |
Present | 1.26 (0.77 to 2.41) | 0.301 |
Necrosis | ||
<50% necrosis | 1.00 (referent) | 0.008* |
≥50% necrosis | 2.45 (1.38 to 4.35) | |
Configuration | ||
Non-multilobulated | 1.00 (referent) | |
Multilobulated | 1.98 (1.01 to 3.90) | 0.009* |
Ktrans | ||
Ktrans<0.262 min−1 | 1.00 (referent) | |
Ktrans ≥0.262 min−1 | 4.19 (1.79 to 9.84) | 0.006* |
Kep | ||
Kep <0.455 min−1 | 1.00 (referent) | |
Kep ≥0.455 min−1 | 1.33 (0.92 to 1.85) | 0.082 |
*, statistically significant at P value <0.05. MRI, magnetic resonance imaging; T2WI, T2-weighted image; HR, high risk; CI, confidence interval; Ktrans, the volume transfer constant; Kep, the flux rate constant between the extravascular, extracellular space and plasma.
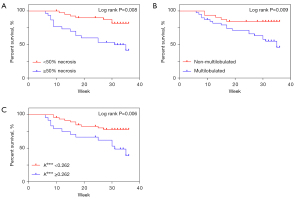
Discussion
This study initially revealed the feasibility of conventional MRI features and DCE-MRI parameters in assessing HIF-1α expression. On this basis, we performed a short-term survival analysis of the study population. The findings suggest that MRI morphological features and quantitative parameters help predict high HIF-1α expression and may significantly predict prognosis in patients with STS.
Although various functional MRIs have been developed to evaluate the histological expression of solid tumors, conventional MRI sequences are still critical for radiologists. Several studies have illustrated that morphologic MRI characteristics may help predict pathological outcomes of STS. Zhao et al. reported that peritumoral enhancement was an independent predictor of high-grade STS (25). Crombé et al. pointed out that MRI features, including necrosis, heterogeneity, and peritumoral enhancement of soft-tissue sarcomas, were associated with grade III tumors, metastasis-free survival, and OS (13). In this study, the univariate results showed that the HIF-1α high-expression group was more likely to have a rapid infiltrative growth pattern with peritumoral enhancement, multilobulated necrotic areas, and T2WI heterogeneity. Subsequent multifactorial analysis showed that more than 50% necrosis and multilobulation were good predictors of high mortality risk in patients. Necrosis, as a vital marker of rapid growth in high-grade malignancies, has been widely reported in studies related to STSs. Indeed, HIF-1α reflects the oxygenation level of the tissue. For highly malignant sarcomas, the faster the growth rate, the higher the need for oxygen. Necrosis is bound to occur when tumor neovascularization cannot meet its demand. The more extensive the area of necrosis, the higher the level of oxygen deprivation of the tumor will be. This malignant cycle explains why extensive necrosis occurs in sarcomas with high HIF-1α expression. The configuration of STS has been reported several times in recent years. Higher grade (G2/3) STS display a polycyclic/multilobulated structure, and this configuration may help predict tumor recurrence (26,27). In the present study, the multilobulated structure was associated with high HIF-1α expression and might be a potential predictor of poor patient prognosis. Indeed, the multilobulated structure reflects an infiltrative tumor growth pattern, and such tumors are often shown to have a high histological grade. We also verified that HIF-1α expression gradually increased with higher histological grade.
The DCE-MRI quantitative analysis introduces a pharmacokinetic model to monitor the interpenetration process between the contrast agent in the intravascular and extracellular extravascular space (EES), which allows a more accurate and visual description of microcirculatory information such as tumor tissue perfusion and capillary permeability (21). The Ktrans reflects the outflow rate of the contrast agent from plasma into EES, determined by the effects of plasma blood flow, permeability, and capillary surface area. Conversely, Kep represents the outflow rate of the contrast agent from the EES back into the plasma. The correlation of DCE-MRI perfusion parameters with HIF-1α expression has been performed in various tumors. The results show that both Ktrans and Kep values positively correlate with HIF-1α expression, which is consistent with the findings of Awasthi and Xie in their studies of gliomas (15,19). However, it is essential to note that several studies have suggested opposite conclusions. In a study of cervical cancer, HIF-1α was negatively correlated with Ktrans or Kep (17). Lindgren also concluded that high DCE-MRI perfusion parameters imply a low hypoxic state within ovarian cancer (18).
The discrepancy between the mechanisms of hypoxia regulation in different histological types of tumors should be considered when interpreting the differences between the results of various studies. It has been reported that HIF-1α expression in cervical and ovarian cancers is mainly induced by oxygen-dependent mechanisms (28,29). The more severe the tumor hypoxia due to reduced intra-tumor perfusion, the higher the HIF-1α expression. In contrast, in STS, the induction pathway of HIF-1α is a non-oxygen-dependent pathway (30). The expression of HIF-1α was mainly generated by the induction of oncogenic signals in tumor cells, which implies that HIF-1α expression was not entirely affected by the hypoxic environment (31). Therefore, our microscopic pathological observation of a diffuse distribution of HIF-1α-positive cells throughout the sections is consistent with the findings of Shintani et al. (32). However, it is known that HIF-1α induces the expression of downstream vascular-related factors such as VEGF, which means that the high expression of HIF-1α leads to more tumor neovascularization. Therefore, the overall level of microcirculation in the tumor is elevated. The exchange of contrast agents between the vascular lumen and the EES becomes faster and more frequent, explaining why the increased expression of HIF-1α leads to higher Ktrans and Kep values. Survival analysis showed that Ktrans values showed a more reliable quantitative predictor of OS in STS patients than the morphological features of conventional MRI [hazard ratio (HR) =4.19], which is an essential addition to Ktrans values in STS studies.
The Ve is a direct estimate of leakage volume used for the distribution of contrast agent in the extravascular space, reflecting the volume of EES. A higher Ve value indicates a more extensive area of tumor necrosis. In this study, Ve values were not significantly different between the high- and low-expression groups, which is inconsistent with previous studies (18,33). The EES reflected by the Ve value is easily affected by cell density, microvascular permeability, necrosis, cystic degeneration, and extracellular matrix. The Ve value is also affected by edema around the tumor tissue and might be inaccurate. However, the diffusion-weighted imaging (DWI) technique may assess extracellular extravascular volume noninvasively by the diffusion limitation of water molecules. The application of DWI techniques in the assessment of HIF-1α expression in tissues has been reported (16,34,35). High HIF-1α expression may promote glucose uptake and metabolism and enhance the proliferation of sarcoma cells, leading to increased cell number and volume, limiting the diffusion of water molecules (36). This implies that DWI techniques and DCE-MRI may complement each other by providing heterogeneous information about microstructures in a hypoxic microenvironment.
Some limitations should be considered when interpreting the results. First, this study involved a small sample and was conducted at a single institution. The differences in the biological behavior of different histological types of STS may have impacted the final results. Second, the standardized image acquisition protocol in DCE-MRI imaging of STS remains uncertain. Further determination of the optimal DCE-MRI scanning protocol for STS is needed in the future. Third, the selection and processing of the ROI in this study were singular. Although this approach is conventional, texture analysis, deep learning, and other means of acquisition may provide more in-depth information for image processing.
Conclusions
This study demonstrated a statistically significant correlation between MRI features and HIF-1α expression in STS, based on the DCE-MRI examination. Significant differences were found between multiple MRI morphological features and quantitative parameters between the high- and low-expression groups. Moreover, the results of short-term survival analysis showed that a greater than 50% necrotic area, multilobulation, and and Ktrans values over 0.262 min−1 were potential predictors of poor prognosis in STS patients. This result suggests the potential of conventional MRI combined with DCE-MRI analysis as a reliable imaging biomarker for predicting STS oxygenation levels. It may offer a method of patient prognosis, however further follow-up studies with large samples are needed to verify its significance as an independent prognostic predictor.
Acknowledgments
Funding: This work was supported by National Natural Science Foundation of China (No. 82171911 and No. 82102013).
Footnote
Reporting Checklist: The authors have completed the STARD reporting checklist. Available at https://qims.amegroups.com/article/view/10.21037/qims-22-75/rc
Conflicts of Interest: All authors have completed the ICMJE uniform disclosure form (available at https://qims.amegroups.com/article/view/10.21037/qims-22-75/coif). QL and BX are employees of Siemens Healthcare. The other authors have no conflicts of interest to declare.
Ethical Statement: The authors are accountable for all aspects of the work in ensuring that questions related to the accuracy or integrity of any part of the work are appropriately investigated and resolved. The study was conducted in accordance with the Declaration of Helsinki (as revised in 2013). The study was approved by the Health Sciences Institutional Review Board (HIRB) of Huashan Hospital, Fudan University, and informed consent was provided by all individual participants.
Open Access Statement: This is an Open Access article distributed in accordance with the Creative Commons Attribution-NonCommercial-NoDerivs 4.0 International License (CC BY-NC-ND 4.0), which permits the non-commercial replication and distribution of the article with the strict proviso that no changes or edits are made and the original work is properly cited (including links to both the formal publication through the relevant DOI and the license). See: https://creativecommons.org/licenses/by-nc-nd/4.0/.
References
- Clark MA, Fisher C, Judson I, Thomas JM. Soft-tissue sarcomas in adults. N Engl J Med 2005;353:701-11. [Crossref] [PubMed]
- Merry E, Thway K, Jones RL, Huang PH. Predictive and prognostic transcriptomic biomarkers in soft tissue sarcomas. NPJ Precis Oncol 2021;5:17. [Crossref] [PubMed]
- Lugano R, Ramachandran M, Dimberg A. Tumor angiogenesis: causes, consequences, challenges and opportunities. Cell Mol Life Sci 2020;77:1745-70. [Crossref] [PubMed]
- Hoos A, Stojadinovic A, Mastorides S, Urist MJ, Polsky D, Di Como CJ, Brennan MF, Cordon-Cardo C. High Ki-67 proliferative index predicts disease specific survival in patients with high-risk soft tissue sarcomas. Cancer 2001;92:869-74. [Crossref] [PubMed]
- Boutin RD, Katz JR, Chaudhari AJ, Yabes JG, Hirschbein JS, Nakache YP, Seibert JA, Lamba R, Fananapazir G, Canter RJ, Lenchik L. Association of adipose tissue and skeletal muscle metrics with overall survival and postoperative complications in soft tissue sarcoma patients: an opportunistic study using computed tomography. Quant Imaging Med Surg 2020;10:1580-9. [Crossref] [PubMed]
- Nyström H, Jönsson M, Werner-Hartman L, Nilbert M, Carneiro A. Hypoxia-inducible factor 1α predicts recurrence in high-grade soft tissue sarcoma of extremities and trunk wall. J Clin Pathol 2017;70:879-85. [Crossref] [PubMed]
- Zhang M, Qiu Q, Li Z, Sachdeva M, Min H, Cardona DM, DeLaney TF, Han T, Ma Y, Luo L, Ilkayeva OR, Lui K, Nichols AG, Newgard CB, Kastan MB, Rathmell JC, Dewhirst MW, Kirsch DG. HIF-1 Alpha Regulates the Response of Primary Sarcomas to Radiation Therapy through a Cell Autonomous Mechanism. Radiat Res 2015;183:594-609. [Crossref] [PubMed]
- Semenza GL. Targeting HIF-1 for cancer therapy. Nat Rev Cancer 2003;3:721-32. [Crossref] [PubMed]
- Reszec J, Hermanowicz A, Rutkowski R, Bernaczyk P, Mariak Z, Chyczewski L. Evaluation of mast cells and hypoxia inducible factor-1 expression in meningiomas of various grades in correlation with peritumoral brain edema. J Neurooncol 2013;115:119-25. [Crossref] [PubMed]
- Shamis SAK, McMillan DC, Edwards J. The relationship between hypoxia-inducible factor 1α (HIF-1α) and patient survival in breast cancer: Systematic review and meta-analysis. Crit Rev Oncol Hematol 2021;159:103231. [Crossref] [PubMed]
- Masoud GN, Li W. HIF-1α pathway: role, regulation and intervention for cancer therapy. Acta Pharm Sin B 2015;5:378-89. [Crossref] [PubMed]
- Yoon C, Lee HJ, Park DJ, Lee YJ, Tap WD, Eisinger-Mathason TS, Hart CP, Choy E, Simon MC, Yoon SS. Hypoxia-activated chemotherapeutic TH-302 enhances the effects of VEGF-A inhibition and radiation on sarcomas. Br J Cancer 2015;113:46-56. [Crossref] [PubMed]
- Crombé A, Marcellin PJ, Buy X, Stoeckle E, Brouste V, Italiano A, Le Loarer F, Kind M. Soft-Tissue Sarcomas: Assessment of MRI Features Correlating with Histologic Grade and Patient Outcome. Radiology 2019;291:710-21. [Crossref] [PubMed]
- Liang J, Cheng Q, Huang J, Ma M, Zhang D, Lei X, Xiao Z, Zhang D, Shi C, Luo L. Monitoring tumour microenvironment changes during anti-angiogenesis therapy using functional MRI. Angiogenesis 2019;22:457-70. [Crossref] [PubMed]
- Awasthi R, Rathore RK, Soni P, Sahoo P, Awasthi A, Husain N, Behari S, Singh RK, Pandey CM, Gupta RK. Discriminant analysis to classify glioma grading using dynamic contrast-enhanced MRI and immunohistochemical markers. Neuroradiology 2012;54:205-13. [Crossref] [PubMed]
- Li X, Yang L, Wang Q, Tao J, Pan Z, Wang S. Soft tissue sarcomas: IVIM and DKI correlate with the expression of HIF-1α on direct comparison of MRI and pathological slices. Eur Radiol 2021;31:4669-79. [Crossref] [PubMed]
- Li X, Wu S, Li D, Yu T, Zhu H, Song Y, Meng L, Fan H, Xie L. Intravoxel Incoherent Motion Combined With Dynamic Contrast-Enhanced Perfusion MRI of Early Cervical Carcinoma: Correlations Between Multimodal Parameters and HIF-1α Expression. J Magn Reson Imaging 2019;50:918-29. [Crossref] [PubMed]
- Lindgren A, Anttila M, Rautiainen S, Arponen O, Hämäläinen K, Könönen M, Vanninen R, Sallinen H. Dynamic contrast-enhanced perfusion parameters in ovarian cancer: Good accuracy in identifying high HIF-1α expression. PLoS One 2019;14:e0221340. [Crossref] [PubMed]
- Xie Q, Wu J, Du Z, Di N, Yan R, Pang H, Jin T, Zhang H, Wu Y, Zhang Y, Yao Z, Feng X. DCE-MRI in Human Gliomas: A Surrogate for Assessment of Invasive Hypoxia Marker HIF-1A Based on MRI-Neuronavigation Stereotactic Biopsies. Acad Radiol 2019;26:179-87. [Crossref] [PubMed]
- Borren A, Groenendaal G, van der Groep P, Moman MR, Boeken Kruger AE, van der Heide UA, Jonges TN, van Diest PJ, van Vulpen M, Philippens ME. Expression of hypoxia-inducible factor-1α and -2α in whole-mount prostate histology: relation with dynamic contrast-enhanced MRI and Gleason score. Oncol Rep 2013;29:2249-54. [Crossref] [PubMed]
- Choi YJ, Lee IS, Song YS, Kim JI, Choi KU, Song JW. Diagnostic performance of diffusion-weighted (DWI) and dynamic contrast-enhanced (DCE) MRI for the differentiation of benign from malignant soft-tissue tumors. J Magn Reson Imaging 2019;50:798-809. [Crossref] [PubMed]
- Li X, Wang Q, Dou Y, Zhang Y, Tao J, Yang L, Wang S. Soft tissue sarcoma: can dynamic contrast-enhanced (DCE) MRI be used to predict the histological grade? Skeletal Radiol 2020;49:1829-38. [Crossref] [PubMed]
- Park H, Kim SH, Kim JY. Dynamic contrast-enhanced magnetic resonance imaging for risk stratification in patients with prostate cancer. Quant Imaging Med Surg 2022;12:742-51. [Crossref] [PubMed]
- Bansal A, Goyal S, Goyal A, Jana M. WHO classification of soft tissue tumours 2020: An update and simplified approach for radiologists. Eur J Radiol 2021;143:109937. [Crossref] [PubMed]
- Zhao F, Ahlawat S, Farahani SJ, Weber KL, Montgomery EA, Carrino JA, Fayad LM. Can MR imaging be used to predict tumor grade in soft-tissue sarcoma? Radiology 2014;272:192-201. [Crossref] [PubMed]
- Sedaghat S, Ravesh MS, Sedaghat M, Meschede J, Jansen O, Both M. Does the primary soft-tissue sarcoma configuration predict configuration of recurrent tumors on magnetic resonance imaging? Acta Radiol 2022;63:642-51. [Crossref] [PubMed]
- Sedaghat S, Salehi Ravesh M, Sedaghat M, Both M, Jansen O. Configuration of soft-tissue sarcoma on MRI correlates with grade of malignancy. Radiol Oncol 2021;55:158-63. [Crossref] [PubMed]
- Bossler F, Hoppe-Seyler K, Hoppe-Seyler F. PI3K/AKT/mTOR Signaling Regulates the Virus/Host Cell Crosstalk in HPV-Positive Cervical Cancer Cells. Int J Mol Sci 2019;20:2188. [Crossref] [PubMed]
- Jiang H, Feng Y. Hypoxia-inducible factor 1alpha (HIF-1alpha) correlated with tumor growth and apoptosis in ovarian cancer. Int J Gynecol Cancer 2006;16:405-12. [Crossref] [PubMed]
- Kim JI, Choi KU, Lee IS, Choi YJ, Kim WT, Shin DH, Kim K, Lee JH, Kim JY, Sol MY. Expression of hypoxic markers and their prognostic significance in soft tissue sarcoma. Oncol Lett 2015;9:1699-706. [Crossref] [PubMed]
- Keith B, Johnson RS, Simon MC. HIF1α and HIF2α: sibling rivalry in hypoxic tumour growth and progression. Nat Rev Cancer 2011;12:9-22. [Crossref] [PubMed]
- Shintani K, Matsumine A, Kusuzaki K, Matsubara T, Satonaka H, Wakabayashi T, Hoki Y, Uchida A. Expression of hypoxia-inducible factor (HIF)-1alpha as a biomarker of outcome in soft-tissue sarcomas. Virchows Arch 2006;449:673-81. [Crossref] [PubMed]
- Jensen RL, Mumert ML, Gillespie DL, Kinney AY, Schabel MC, Salzman KL. Preoperative dynamic contrast-enhanced MRI correlates with molecular markers of hypoxia and vascularity in specific areas of intratumoral microenvironment and is predictive of patient outcome. Neuro Oncol 2014;16:280-91. [Crossref] [PubMed]
- Huang Z, Xu X, Meng X, Hou Z, Liu F, Hua Q, Liu Q, Xiu J. Correlations between ADC values and molecular markers of Ki-67 and HIF-1α in hepatocellular carcinoma. Eur J Radiol 2015;84:2464-9. [Crossref] [PubMed]
- Ma T, Yang S, Jing H, Cong L, Cao Z, Liu Z, Huang Z. Apparent diffusion coefficients in prostate cancer: correlation with molecular markers Ki-67, HIF-1α and VEGF. NMR Biomed 2018; [Crossref] [PubMed]
- Lee JH, Yoon YC, Seo SW, Choi YL, Kim HS. Soft tissue sarcoma: DWI and DCE-MRI parameters correlate with Ki-67 labeling index. Eur Radiol 2020;30:914-24. [Crossref] [PubMed]