Perfusion magnetic resonance imaging in the differentiation between glioma recurrence and pseudoprogression: a systematic review, meta-analysis and meta-regression
Introduction
Glioma is the most common primary intracerebral tumor of the central nervous system (1). According to the 2021 World Health Organization (WHO) classification of tumors of the central nervous system, adult-type diffuse gliomas are divided into astrocytoma, oligodendroglioma and glioblastoma, accounting for approximately 22% of all central nervous system tumors (1,2). Currently, radiotherapy combined with adjuvant temozolomide chemotherapy has become the standard treatment for newly diagnosed glioma in adults (3,4). The higher the tumor grade, the higher the risk of recurrence and death (5). Regular follow-up and early detection of tumor recurrence have important clinical significance (6). According to the recommendations of the Response Assessment in Neuro-Oncology (RANO) Working Group, magnetic resonance imaging (MRI) examination is the main method for follow-up after treatment; however, in conventional MRI, tumor recurrence and pseudoprogression (PsP) have similar imaging manifestations, making them difficult to making them difficult to differentiate (7). Furthermore, the subsequent treatments for tumor recurrence and PsP are completely different (8).
At present, magnetic resonance (MR) perfusion-weighted imaging (PWI) is a hot research topic for many researchers in China and internationally. The most commonly used PWI techniques include dynamic susceptibility contrast MRI (DSC-MRI), dynamic contrast enhanced MRI (DCE-MRI), and arterial spin labeling (ASL). Among these methods, DSC-MRI is usually used to evaluate the distribution of microcirculation, the degree of microvascular proliferation, and blood perfusion (9); DCE-MRI is mainly applied to calculate functional parameters related to tissue flow and leakage of contrast agent from the intravascular space (10); and ASL can noninvasively reflect tissue blood perfusion information without contrast agents (11). The PWI findings of gliomas are shown in Figure 1, and the MRI scan protocols and parameters are shown in Appendix 1 (Table S1). However, the previous meta-analysis (12-14) based on the above studies have involved small sample sizes, limited glioma grading and short time spans, which affected the stability and reliability of the results, and evaluation of the diagnostic value of MR perfusion imaging has remained incomplete. Therefore, this study attempted to perform a meta-analysis of published studies to evaluate the accuracy of MR perfusion studies in the differentiation of glioma recurrence from PsP, which may assist with future clinical treatment selection and improve the prognosis of patients. We present the following article in accordance with the Preferred Reporting Items for Systematic Reviews and Meta-Analyses Diagnostic Test Accuracy (PRISMA-DTA) reporting checklist (15) (available at https://qims.amegroups.com/article/view/10.21037/qims-22-32/rc).
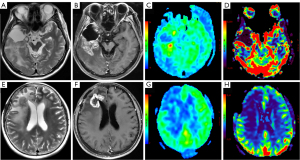
Methods
The meta-analysis was registered on the International Prospective Register of Systematic Reviews (PROSPERO) with the registration number of CRD42022304404.
Literature search strategy
A systematic search in 4 international databases (PubMed, Embase, Web of Science and Cochrane Library) and 4 Chinese local academic databases [China National Knowledge Infrastructure (CNKI), Wanfang Med Online, Sinomed, and Chinese Medical Journal of Database (CMJD)] was performed up to 31 October 2021. The search terms were a combination of Medical Subject Headings (MeSH) terms and text words representing (I) glioma, (II) MR PWI, (III) tumor recurrence, and (IV) PsP. Details of the literature search strategy are provided in Appendix 2. Two reviewers independently screened paper titles, abstracts, and full text. For any difference of opinion that emerged during data extraction, consensus was reached between the two reviewers by discussion or consultation with a third reviewer. Articles that cited the included articles were also checked to see if any studies were omitted after the initial search.
Selection criteria
The inclusion criteria were as follows: (I) clinical studies using MRI perfusion imaging to differentiate between glioma recurrence and PsP, in Chinese or English; (II) studies in which local or whole brain radiotherapy was performed after surgery, and an abnormal enhanced lesion appeared in the operative area; (III) studies in which recurrence was defined as the pathological results of a second operation or combined follow-up examination, and the standard for radiation brain injury was mainly evidence from MRI follow-up; (IV) studies in which diagnostic 2×2 tables could be extracted directly or indirectly.
Studies were excluded if (I) the study type was a case report or review or they were published in the Chinese literature but not included in the Institute of Scientific and Technical Information of China (ISTIC); (II) they contained a sample size ≤30; (III) they included patients aged ≤18 years; (IV) they demonstrated incomplete reporting of essential data, such as a lack of sensitivity and specificity and incomplete and informally published studies.
Data extraction and quality assessment
Data extraction and quality evaluation included the following: (I) basic information: first author, publication year, country, study type, number of cases, age, WHO classification, treatment, and diagnostic criteria; (II) MR information, including MR equipment, field intensity, and perfusion imaging methods and parameters; (III) Data from 2×2 tables of diagnostic tests requiring evaluation, including true positive (TP), true negative (TN), false positive (FP), and false negative (FN) test results. The Quality Assessment of diagnostic Accuracy Studies 2 (QUADAS-2) tool was used to evaluate the quality of the included studies, including the risk of bias and applicability concerns.
Statistical analysis
Statistical analysis was performed using Stata 16.0 (Stata Corp, College Station, TX, USA) and Meta-Disc 1.4 (http://www.hrc.es/investigacion/metadisc_en.htm). The pooled sensitivity, specificity and diagnostic odds ratio (DOR) were calculated and the summary receiver operating characteristic (SROC) was plotted. The Spearman correlation coefficient was used to test the threshold effect. A Fagan plot was drawn to calculate prior probability and posterior probability. Cochran’s Q test was applied to determine whether there was heterogeneity, and I2 was adopted to measure the heterogeneity. If homogeneity among the results was good (Cochran’s Q test P>0.1; I2≤50%), a fixed-effects model was adopted; otherwise, a random-effect model was used along with an attempt to identify the source of heterogeneity through meta-regression and subgroup analysis. A P value of <0.05 was considered to indicate a statistically significant difference. Funnel plots were drawn to determine whether publication bias existed.
Results
Literature search process and study selection
A total of 1,342 studies were preliminarily identified through electronic database searches. After removing duplicate studies, they were assessed for eligibility for inclusion. A total of 40 studies were finally selected, including 27 English studies and 13 Chinese studies. There were 2,217 patients, including 1,341 cases of tumor recurrence and 876 cases of PsP. Of the patients in all of the included studies, 39.5% [95% confidence interval (CI): 0.37 to 0.42] displayed PsP due to treatment effects. A total of 60.5% (95% CI: 0.58 to 0.63) of the patients with progression were diagnosed with true progression. A flow chart of the study selection process is shown in Figure 2.
The characteristics of the included studies are shown in Table 1. Of the 40 studies, 30 were retrospective studies, 5 were prospective studies (24,28,35,41,42), and 5 were not described (43,46,47,52,53). Tumor types were classified into glioma (WHO grade II–IV) (31,35,37,40,44,46,52), high-grade glioma (WHO grade III–IV) (27,33,34,36,42,43,45,47-51,54,55) and glioblastoma (WHO grade IV) (16-26,28-30,32,38,39,41,53). All glioma grades were based on the WHO classification prior to 2021. As the “gold standard” for diagnosis, 28 studies used pathological diagnosis combined with follow-up, 6 studies used pathological diagnosis (20,26,33,35,55), and 6 studies were confirmed by follow-up alone (23,24,36,39,41,44). Among the 40 studies, DSC-MRI was used in 28 studies to distinguish glioma recurrence from PsP, most of which used relative cerebral blood volume (rCBV) as the best parameter (approximately 50%), and the other parameters included relative peak height (rPH), relative cerebral blood flow (rCBF), and 90% normalized cerebral blood volume (nCBV). The DCE-MRI technique was performed in 14 studies (20-22,25,30,32,36,38,39,41,42,44,45,51), most of which used transfer constant (Ktrans) as the best parameter; ASL was applied in 12 studies (19,24,29,31,35,36,40,43,48,49,53,55), and rCBF was the most commonly used parameter. For the quality assessment of the literature, the QUADAS-2 tool showed low-risk bias and good clinical applicability. The risk of bias and applicability concerns graph of the included studies is shown in Figure 3. The methodology for quality assessment in this study was consistent with that of the previous meta-analysis (12), which included the risk of bias and applicability concerns. There was potential introduction of bias in patient selection, index test, reference standard, and flow and timing.
Table 1
First author | Year | Nation | Study design | Cases | Age (y) | WHO grade | PWI | Best parameter | Field strength | Reference standard | Follow-up interval | TP | FP | TN | FN |
---|---|---|---|---|---|---|---|---|---|---|---|---|---|---|---|
Baek (16) | 2012 | Korea | R | 79 | 50.6 [19–83] | IV | DSC-MRI | nCBV | 3.0-T Philips | Both | Within 4 w | 36 | 4 | 33 | 6 |
Barajas (17) | 2009 | USA | R | 66 | 54.2 | IV | DSC-MRI | rPH | 1.5-T GE | Both | 1.7–50.2 m | 41 | 4 | 16 | 5 |
Cha (18) | 2014 | Korea | R | 35 | 49 [24–70] | IV | DSC-MRI | Mean rCBV | 3.0-T Philips | Both | Within 6 m | 9 | 4 | 20 | 2 |
Choi (19) | 2013 | Korea | R | 62 | 49.3 [22–79] | IV | DSC-MRI | nCBV | 3.0-T Philips | Both | Within 4 w | 28 | 9 | 19 | 6 |
ASL | ASLmaps | 27 | 10 | 18 | 7 | ||||||||||
Chung (20) | 2013 | Korea | R | 57 | 52.1 [25–69] | IV | DCE-MRI | mAUCR | 3.0-T Philips | Path | 39.6 w | 30 | 3 | 22 | 2 |
Elshafeey (21) | 2019 | USA | R | 98 | UR | IV | DCE-MRI | Ktrans | 1.5-T and 3.0-T UR |
Both | ≤24 m | 70 | 2 | 20 | 6 |
DSC-MRI | rCBV | 70 | 0 | 22 | 6 | ||||||||||
Heo (22) | 2015 | Korea | R | 45 | 53.9 [27–73] | IV | DCE-MRI | 90th% IAUC | 3.0-T Philips | Both | 14 w | 16 | 7 | 18 | 4 |
Hu (23) | 2011 | USA | R | 31 | UR | IV | DSC-MRI | nCBV | UR | Follow up | Every 2–3 m | 13 | 1 | 15 | 2 |
Jovanovic (24) | 2017 | Serbia | P | 31 | 49±13.84 | IV | ASL | CBF | 3.0-T Siemens | Follow up | >3 m | 18 | 1 | 10 | 2 |
DSC-MRI | nCBV | 20 | 0 | 11 | 0 | ||||||||||
Kim (25) | 2014 | Korea | R | 169 | 52.2 [25–69] | IV | DSC-MRI | 90th% nCBV | 3.0-T Philips | Both | 46.5 w | 73 | 4 | 78 | 14 |
DCE-MRI | 30th% IAUC | 79 | 11 | 71 | 8 | ||||||||||
Kim (26) | 2014 | Korea | R | 51 | 51.5 [25–72] | IV | DSC-MRI | 90th% nCBV | 3.0-T Philips | Path | 46.5 w | 26 | 1 | 19 | 5 |
Kim (27) | 2017 | Korea | R | 51 | 52.9±11.6 | III–IV | DSC-MRI | 90th% nCBV | 3.0-T GE and Siemens | Both | ≥6 m | 28 | 5 | 14 | 4 |
Kong (28) | 2011 | Korea | P | 59 | 25–74 | IV | DSC-MRI | rCBV | 3.0-T Philips | Both | ≥3–4 m | 27 | 6 | 20 | 6 |
Manning (29) | 2020 | USA | R | 32 | 56±13 | IV | ASL | nCBF | 3.0-T GE | Both | ≥6 m | 23 | 1 | 6 | 2 |
DSC-MRI | nrCBF | 22 | 1 | 6 | 3 | ||||||||||
Nael (30) | 2018 | USA | R | 46 | 32–78 | IV | DSC-MRI | rCBV | 3.0-T Siemens | Both | 9–13 m | 27 | 1 | 11 | 7 |
DCE-MRI | Ktrans | 23 | 2 | 10 | 11 | ||||||||||
Ozsunar (31) | 2010 | Turkey | R | 32 | 42±11 | II–IV | DSC-MRI | CBV | 1.5-T GE | Both | 1 d–4 w | 19 | 3 | 7 | 3 |
ASL | ASLmaps | 18 | 1 | 11 | 3 | ||||||||||
Park (32) | 2015 | Korea | R | 54 | 49.1±10.5 | IV | DSC-MRI | 90th% nCBV | UR | Both | Within 12 w | 18 | 5 | 26 | 5 |
DCE-MRI | 90th% IAUC | 19 | 6 | 25 | 4 | ||||||||||
Prager (33) | 2015 | USA | R | 68 | 54.9 [22.6–79.4] | III–IV | DSC-MRI | rCBV | 1.5-T and 3.0-T GE | Path | 6.1 m (0.4–40.4 m) |
50 | 2 | 8 | 8 |
Qiao (34) | 2019 | China | R | 42 | UR | III–IV | DSC-MRI | rCBVmean | 3.0-T Siemens | Both | Interval >3 m | 22 | 2 | 7 | 11 |
Razek (35) | 2018 | Egypt | P | 42 | UR | II–IV | ASL | CBF | 1.5-T Philips | Path | 11 m | 23 | 1 | 17 | 1 |
Seeger (36) | 2013 | Germany | R | 40 | 53.6 | III–IV | DSC-MRI | CBV | 1.5-T Siemens | Follow up | 10 m (6–15 m) | 19 | 4 | 13 | 4 |
40 | 53.6 | III–IV | DCE-MRI | Ktrans | 14 | 3 | 14 | 9 | |||||||
26 | 53.6 | III–IV | ASL | rCBF | 8 | 2 | 10 | 6 | |||||||
Steidl (37) | 2021 | Germany | R | 104 | 52 [20–78] | II–IV | DSC-MRI | rCBV | 1.5-T Philips and 3.0-T Siemens | Both | WHO III–IV 6 m, WHO II 12 m | 45 | 0 | 21 | 38 |
Suh (38) | 2013 | Korea | R | 79 | 51.2 [25–69] | IV | DCE-MRI | mAUCRH | 3.0-T Philips | Both | Within 5 w | 39 | 6 | 30 | 4 |
Thomas (39) | 2015 | USA | R | 37 | 37–87 | IV | DCE-MRI | 90th% nVp | 1.5- and 3.0-T GE | Follow up | UR | 22 | 2 | 11 | 2 |
Wang (40) | 2018 | China | R | 69 | 41.6 [18–77] | II–IV | ASL | nCBF | 3.0-T GE | Both | Every 2–3 m/17 m (6–96 m) | 24 | 3 | 31 | 11 |
69 | 41.6 [18–77] | II–IV | DSC-MRI | nrCBF | 3.0-T GE | Both | Every 2–3 m/17 m (6–96 m) | 26 | 3 | 31 | 9 | ||||
Yun (41) | 2015 | Korea | P | 33 | 54.6 [28–82] | IV | DCE-MRI | 5th% Ve | 3.0-T Siemens | Follow up | UR | 13 | 2 | 14 | 4 |
Zakhari (42) | 2019 | Canada | P | 65 | 54.1 [50.9–57.3] | III–IV | DSC-MRI | CBV | 3.0-T Siemens | Both | 1–3 m | 24 | 6 | 22 | 13 |
DCE-MRI | Ktrans | 19 | 9 | 19 | 18 | ||||||||||
Hu (43) | 2019 | China | UR | 32 | 47 [28–69] | III–IV | ASL | rCBF | 3.0-T Siemens | Both | 11 m (6–26 m) | 16 | 2 | 5 | 9 |
Qian (44) | 2016 | China | R | 32 | 52.2±9.1 | II–IV | DCE-MRI | Ktrans | 3.0-T GE | Follow up | ≥6 m | 13 | 2 | 12 | 5 |
Ren (45) | 2019 | China | R | 32 | 48.0±14.1 | III–IV | DCE-MRI | Ktrans | UR | Both | ≥6 m | 21 | 1 | 9 | 1 |
Sha (46) | 2013 | China | UR | 52 | 50.4±18.8 | II–IV | DSC-MRI | rCBVmax | 3.0-T Siemens | Both | 2–4 m | 21 | 0 | 22 | 9 |
Shan (47) | 2020 | China | UR | 32 | 49.4 | III–IV | DSC-MRI | rCBV | 3.0-T Siemens | Both | ≥6 m | 16 | 1 | 9 | 6 |
Shi (48) | 2020 | China | R | 40 | 55±11 | III–IV | ASL | rCBF | 3.0-T GE | Both | ≥6 m | 17 | 2 | 18 | 3 |
DSC-MRI | rCBV | 18 | 6 | 14 | 2 | ||||||||||
Wang (49) | 2016 | China | R | 36 | 50 [19–70] | III–IV | ASL | rCBF | 1.5-T GE | Both | ≥10 m | 6 | 5 | 23 | 2 |
Wang (50) | 2017 | China | R | 56 | 56.4 [14.5–67.8] | III–IV | DSC-MRI | rCBV | 3.0-T Siemens | Both | UR | 25 | 0 | 26 | 5 |
Xie (51) | 2019 | China | R | 86 | 45.2±5.6 | III–IV | DCE-MRI | Ktrans | UR | Both | UR | 40 | 7 | 31 | 8 |
Xing (52) | 2016 | China | UR | 54 | 47 [13–74] | II–IV | DSC-MRI | rCBV | 3.0-T Siemens | Both | ≥6 m | 26 | 1 | 19 | 8 |
Xu (53) | 2018 | China | UR | 31 | UR | IV | ASL | CBF | 3.0-T Siemens | Both | ≥6 m | 11 | 9 | 8 | 3 |
DSC-MRI | rCBV | 9 | 11 | 6 | 5 | ||||||||||
Yin (54) | 2015 | China | R | 96 | 43 [24–55] | III–IV | DSC-MRI | rCBV | UR | Both | 2 m–2 y | 67 | 18 | 6 | 5 |
Zhang (55) | 2019 | China | R | 58 | 58 [18–65] | III–IV | ASL | rCBF | 3.0-T GE | Path | UR | 29 | 0 | 20 | 9 |
R, retrospective; P, prospective; UR, unreported; y, years; WHO, World Health Organization; PWI, perfusion-weighted imaging; DSC-MRI, dynamic susceptibility contrast magnetic resonance imaging; ASL, arterial spin labeling; DCE-MRI, dynamic contrast enhanced magnetic resonance imaging; CBV, cerebral blood volume; nCBV, normalized CBV; rPH, relative peak height; rCBV, relative CBV; AUC, area under the curve; mAUCR, mean AUC ratio; Ktrans, transfer constant; IAUC, initial AUC; CBF, cerebral blood flow; nCBF, normalized CBF; nrCBF, normalized relative CBF; rCBF, relative CBF; mAUCRH, mean AUC RH; Vp, volumetric plasma volume; nVp, normalized Vp; Ve, volume fraction of extracellular extravascular space; Path, pathology; d, days; w, weeks; m, months; TP, true positive; TN, true negative; FP, false positive; FN, false negative.
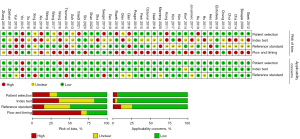
Results of the meta-analysis of the DSC-MRI studies
A total of 28 studies were included in the meta-analysis, including 21 English studies and 7 Chinese studies. The threshold effect test results showed that Spearman’s correlation coefficient was −0.3 (P=0.09). After drawing the SROC diagram, no obvious “shoulder-arm shape” emerged, indicating that there was no heterogeneity caused by the threshold effect in this study. The results of the forest plots showed that the Q test of sensitivity was P<0.01 with I2=68.33%, and the Q test of specificity was P<0.01 with I2=81.00%, indicating that there was significant heterogeneity among the included studies. Therefore, a random-effects model was used to analyze the pooled sensitivity and specificity in the DSC-MRI studies. The results showed that the pooled sensitivity, specificity, positive likelihood ratio (PLR), negative likelihood ratio (NLR), and DOR were 0.82 (95% CI: 0.78 to 0.86), 0.87 (95% CI: 0.80 to 0.92), 6.5 (95% CI: 4.1 to 10.3), 0.20 (95% CI: 0.17 to 0.25) and 32 (95% CI: 18 to 55), respectively. The area under the curve (AUC) was 0.89 (95% CI: 0.86 to 0.92). Fagan plots displayed a prior probability of 0.5 and a posterior probability of 0.87 and 0.17 for the PLR and NLR, respectively. The above results for the DSC-MRI studies are shown in Table 2 and Figures 4-6.
Table 2
PWI | Studies | Cases | Se (95% CI) | Sp (95% CI) | PLR (95% CI) | NLR (95% CI) | DOR [95% CI] | AUC (95% CI) |
---|---|---|---|---|---|---|---|---|
DSC-MRI | 28 | 1,645 | 0.82 (0.78–0.86) | 0.87 (0.80–0.92) | 6.5 (4.1–10.3) | 0.20 (0.17–0.25) | 32 [18–55] | 0.89 (0.86–0.92) |
DCE-MRI | 14 | 873 | 0.83 (0.76–0.89) | 0.83 (0.78–0.87) | 4.9 (3.6–6.6) | 0.20 (0.13–0.30) | 24 [12–47] | 0.88 (0.85–0.91) |
ASL | 12 | 492 | 0.80 (0.73–0.86) | 0.86 (0.76–0.92) | 5.7 (3.1–10.3) | 0.23 (0.16–0.33) | 24 [10–57] | 0.88 (0.85–0.91) |
PWI, perfusion-weighted imaging; PsP, pseudoprogression; DSC-MRI, dynamic susceptibility contrast magnetic resonance imaging; DCE-MRI, dynamic contrast enhanced magnetic resonance imaging; ASL, arterial spin labeling; Se, sensitivity; CI, confidence interval; Sp, specificity; PLR, positive likelihood ratio; NLR, negative likelihood ratio; DOR, diagnostic odds ratio; AUC, area under the curve.
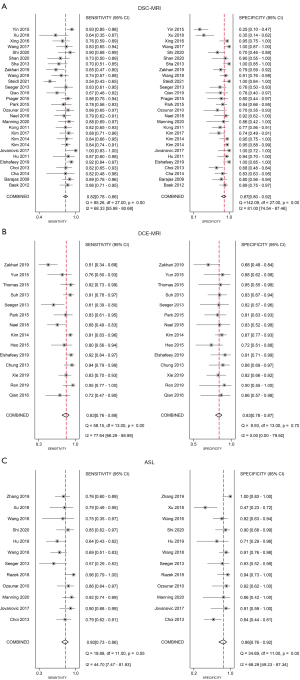
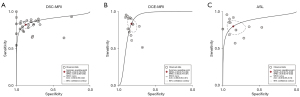
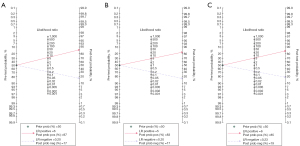
Results of the meta-analysis of the DCE-MRI studies
A total of 14 studies were included, including 11 English studies and 3 Chinese studies. The threshold effect test results showed that Spearman’s correlation coefficient was 1.0 (P=1.00). After drawing the SROC diagram, no obvious “shoulder-arm shape” emerged, indicating that there was no heterogeneity caused by the threshold effect in this study. The results of forest plots showed that the Q test of sensitivity was P<0.01 with I2=77.64%, and the Q test of specificity was P>0.1 with I2=0.00%, indicating that there was significant heterogeneity in the sensitivity among the included studies. Therefore, a random-effects model was used to analyze the pooled sensitivity and specificity in the DCE-MRI studies. The results showed that the pooled sensitivity, specificity, PLR, NLR, and DOR were 0.83 (95% CI: 0.76 to 0.89), 0.83 (95% CI: 0.78 to 0.87), 4.9 (95% CI: 3.6 to 6.6), 0.20 (95% CI: 0.13 to 0.30) and 24 (95% CI: 12 to 47), respectively. The AUC was 0.88 (95% CI 0.85 to 0.91). Fagan plots showed a prior probability of 0.5 and a posterior probability of 0.83 and 0.17 for PLR and NLR, respectively. The above results for the DCE studies are shown in Table 2 and Figures 4-6.
Results of the meta-analysis of the ASL studies
A total of 12 studies were included, including 7 English studies and 5 Chinese studies. The threshold effect test results showed that Spearman’s correlation coefficient was 0.4 (P=0.16). After drawing the SROC diagram, no obvious “shoulder-arm shape” emerged, indicating that there was no heterogeneity caused by the threshold effect in this study. The results of forest plots showed that the Q test of sensitivity was P>0.1 with I2=44.70%, and the Q test of specificity was P<0.01 with I2=68.29%, indicating that there was significant heterogeneity in the sensitivity among the included studies. Therefore, a random-effects model was used to analyze the pooled sensitivity and specificity in the ASL studies. The results showed that the pooled sensitivity, specificity, PLR, NLR, and DOR were 0.80 (95% CI: 0.73 to 0.86), 0.86 (95% CI: 0.76 to 0.92), 5.7 (95% CI: 3.1 to 10.3), 0.23 (95% CI: 0.16 to 0.33) and 24 (95% CI: 10 to 57), respectively. The AUC was 0.88 (95% CI: 0.85 to 0.91). Fagan plots showed a prior probability of 0.5 and a posterior probability of 0.85 and 0.19 for PLR and NLR, respectively. The above results for the ASL studies are shown in Table 2 and Figures 4-6.
Meta regression analysis and subgroup analysis
Univariate regression analysis was applied to identify the sources of study heterogeneity, including study type, tumor type, diagnostic criteria, field strength, and MRI parameter. The results demonstrated that tumor type was the main factor leading to the heterogeneity of the sensitivity in the DSC-MRI studies and specificity in the DSC-MRI studies, and the difference was statistically significant. In the DCE-MRI studies, study type was the main reason leading to the heterogeneity of the sensitivity. The results of the meta-regression analysis are shown in Appendix 3 (Table S2).
Subgroup analysis further clarified the impact of the above factors on the heterogeneity of the results. In the DSC-MRI studies, in which 14 studies were conducted on WHO grade IV gliomas, the pooled sensitivity and specificity were 0.85 and 0.88, respectively, and the AUC was 0.90. Nine studies were conducted on WHO grade III–IV gliomas, with pooled sensitivity and specificity of 0.79 and 0.87, respectively, and an AUC of 0.87. The remaining 5 studies involved WHO grade II–IV gliomas with pooled sensitivity and specificity of 0.82 and 0.87, respectively, and an AUC of 0.87. In the DCE-MRI studies, 12 studies were retrospective studies, with pooled sensitivity and specificity of 0.86 and 0.84, respectively, and an AUC of 0.89. Two studies were prospective, with pooled sensitivity and specificity of 0.60 and 0.75, respectively. In the studies of ASL, 3 involved WHO grade II–IV gliomas, and their pooled sensitivity and specificity were 0.81 and 0.92, respectively, with an AUC of 0.98. Five studies involved WHO grade III–IV gliomas, with pooled sensitivity and specificity values of 0.72 and 0.88, respectively, and an AUC of 0.84. Four studies focused on WHO grade IV gliomas. The pooled sensitivity and specificity were 0.85 and 0.67, respectively, with an AUC of 0.95. See Appendix 4 (Table S3).
Publication bias
The Deeks’ funnel plot showed that the data were symmetrically distributed (DSC-MRI: P=0.216; DCE-MRI: P=0.381; ASL: P=0.735), suggesting no significant publication bias, as shown in Figure 7. In view of the possibility of publication bias as expected in the studies from local China databases, we performed subgroup analyses and drew funnel plots to determine whether publication bias existed. The results showed that there was no publication bias in DSC-MRI, DCE-MRI, and ASL studies from Chinese and English databases (P>0.05) [Appendix 5 (Table S4, Figures S1-S3)].
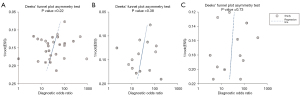
Discussion
In the process of postoperative radiation therapy for glioma, it is easy to cause brain damage (56). As it usually occurs weeks to months after radiotherapy (8,57), PsP is difficult to distinguish from tumor recurrence (58). Histopathological diagnosis is the gold standard for differentiating between the two; however, it is difficult for patients to undergo biopsy or a second operation before receiving the final diagnosis. At present, some MRI imaging methods are used to determine whether there is tumor progression, such as diffusion weighted imaging (DWI), PWI, and MR spectrum imaging and so on. Of these, PWI is one of the most reliable imaging techniques (24,29). In this study, three MRI perfusion imaging techniques (DSC-MRI, DCE-MRI, and ASL) were included to systematically evaluate and statistically analyze studies focused on differentiating between tumor recurrence and PsP. The incidence of PsP found in our study was comparable with what is known from the literature, 39.5% in the included studies versus 37% in a previous meta-analysis by Abbasi et al. (59).
The results demonstrated that all three perfusion imaging methods displayed a high pooled diagnostic performance. Among the three, DSC-MRI performed the best, with a pooled sensitivity and specificity of 0.82 and 0.87, respectively, a DOR of 24, and an area under the SROC curve of 0.89. However, heterogeneity analysis indicated obvious heterogeneity among the studies. The threshold effect test combined with the SROC curve results suggested that heterogeneity was not caused by the threshold effect; hence, meta regression analysis and subgroup analysis were applied to find the source of heterogeneity. After analysis, the sensitivity of the tumor type group was significantly higher than that of the other groups. Further subgroup analysis showed that the sensitivity of WHO grade IV glioma patients was significantly higher than that of WHO grades III–IV and WHO grades II–IV glioma patients, with sensitivities of 0.85, 0.79, and 0.72, respectively.
The DSC-MRI is a functional MR imaging technique that reflects the distribution of tissue microvessels and blood perfusion. Due to the increased expression of vascular endothelial growth factor, high neovascular density, and immature vascular structure in the recurrence area of grade IV glioma, the recurrence area shows hyperperfusion, while the area of PsP displays hypoperfusion due to vascular endothelial cell apoptosis (60). For lower-grade gliomas (such as WHO grade II), there was no significant difference in changes after radiotherapy due to relatively little neovascularization. The results in our study were similar to those of the previous meta-analysis by Wang et al. (61), suggesting that DSC-MRI is the perfusion imaging technique with the highest accuracy for differentiating glioma recurrence from PsP; however, the results of the subgroup analysis were different. In the study by Wang et al., field strength and tumor type specificity were the sources of heterogeneity among DSC-MRI studies. The reason for the difference may be related to the exclusion of small sample (≤30 participants) studies as well as the inclusion of only adult glioma patients in our study, and the addition of Chinese glioma-related studies.
Compared with traditional DSC-MRI, DCE-MRI has higher spatial resolution, which not only provides tumor perfusion information, but also reflects vascular permeability (62). However, DCE needs to select an appropriate pharmacokinetic model, and the parameters obtained are relatively complex and diverse, leading to relatively few studies having focused on this technique and less frequent clinical application compared with DSC-MRI. Despite its limitations, our study shows that DCE also displays a high accuracy for differentiating recurrent glioma from PsP, with a sensitivity and specificity of 0.83 and 0.83, respectively. As a complete noncontrast agent perfusion imaging technology, ASL applies water molecules in endogenous arterial blood as tracers, which are not affected by the integrity of the blood-brain barrier and are able to more truly reflect tissue perfusion (63). Our results are similar to the meta-analysis results of Du et al. (14), demonstrating that ASL has high sensitivity and specificity in distinguishing glioma recurrence and PsP, with a sensitivity and specificity of 0.80 and 0.86 respectively.
The studies included in this meta-analysis were based on the 2016 or earlier WHO classification. In 2021, the WHO classification was updated, emphasizing the important role of genetics in the development and subsequent treatment of glioma (2). For adult gliomas, changes in glioblastoma have greater clinical significance. It has been verified that IDH-mutant and IDH-wildtype have distinct biological behaviors and prognosis (64-66). In the new classification, glioblastoma represents only IDH-wildtype glioma. Alternatively, tumors that contain one or more of three genes [TERT promoter mutation, EGFR gene amplification, or copy number changes on chromosome 7/10 (+7/−10)] into the classification of glioblastoma (2). These changes contribute to a more homogeneous study population in clinical trials. Other molecular alterations, such as CDKN2A/B homozygous deletion in IDH-mutant gliomas, tends to predict worse prognosis (67,68).
There were some limitations to this study. First, the inclusion criteria of this study did not entirely depend on histopathological diagnosis, and differences in follow-up time and diagnostic criteria may have caused bias in the study results. Second, this study included WHO grade II–IV tumors according to the WHO Classification of central nervous system tumors prior to 2021. Although most of the tumors were WHO grade IV tumors, the results of the analysis may have been biased by treatment differences due to different tumor grades. In addition, most of the included studies were retrospective studies, MRI perfusion imaging parameters were more complicated, and the selection of parameters and threshold values lacked uniform standards, which may have aggravated the heterogeneity of the studies. Finally, in the quality assessment of the included studies, it was found that some of the studies did not report blinding in detail, and there may have been risk bias in measurements and subsequent results.
To sum up, our meta-analysis demonstrated that DSC-MRI, DCE-MRI, and ASL, as advanced MR perfusion imaging techniques, could accurately differentiate postoperative glioma recurrence from PsP. Among them, DSC-MRI had a higher diagnostic performance than the other two techniques. Therefore, MRI perfusion imaging could be used as a feasible and quantitative examination method for postoperative follow-up after radiotherapy and chemotherapy, providing strong evidence to support the subsequent clinical treatment.
Acknowledgments
Funding: This study was supported by China Postdoctoral Science Foundation (No. 2020T130779).
Footnote
Reporting Checklist: The authors have completed the PRISMA-DTA reporting checklist. Available at https://qims.amegroups.com/article/view/10.21037/qims-22-32/rc
Conflicts of Interest: All authors have completed the ICMJE uniform disclosure form (available at https://qims.amegroups.com/article/view/10.21037/qims-22-32/coif). Yulin Wang reports that this study was supported by China Postdoctoral Science Foundation (No. 2020T130779). The other authors have no conflicts of interest to declare.
Ethical Statement: The authors are accountable for all aspects of the work in ensuring that questions related to the accuracy or integrity of any part of the work are appropriately investigated and resolved.
Open Access Statement: This is an Open Access article distributed in accordance with the Creative Commons Attribution-NonCommercial-NoDerivs 4.0 International License (CC BY-NC-ND 4.0), which permits the non-commercial replication and distribution of the article with the strict proviso that no changes or edits are made and the original work is properly cited (including links to both the formal publication through the relevant DOI and the license). See: https://creativecommons.org/licenses/by-nc-nd/4.0/.
References
- Ostrom QT, Cioffi G, Waite K, Kruchko C, Barnholtz-Sloan JS. CBTRUS Statistical Report: Primary Brain and Other Central Nervous System Tumors Diagnosed in the United States in 2014-2018. Neuro Oncol 2021;23:iii1-iii105. [Crossref] [PubMed]
- Louis DN, Perry A, Wesseling P, Brat DJ, Cree IA, Figarella-Branger D, Hawkins C, Ng HK, Pfister SM, Reifenberger G, Soffietti R, von Deimling A, Ellison DW. The 2021 WHO Classification of Tumors of the Central Nervous System: a summary. Neuro Oncol 2021;23:1231-51. [Crossref] [PubMed]
- Stupp R, Mason WP, van den Bent MJ, Weller M, Fisher B, Taphoorn MJ, et al. Radiotherapy plus concomitant and adjuvant temozolomide for glioblastoma. N Engl J Med 2005;352:987-96. [Crossref] [PubMed]
- Stupp R, Hegi ME, Mason WP, van den Bent MJ, Taphoorn MJ, Janzer RC, et al. Effects of radiotherapy with concomitant and adjuvant temozolomide versus radiotherapy alone on survival in glioblastoma in a randomised phase III study: 5-year analysis of the EORTC-NCIC trial. Lancet Oncol 2009;10:459-66. [Crossref] [PubMed]
- Weller M, van den Bent M, Preusser M, Le Rhun E, Tonn JC, Minniti G, et al. EANO guidelines on the diagnosis and treatment of diffuse gliomas of adulthood. Nat Rev Clin Oncol 2021;18:170-86. [Crossref] [PubMed]
- Chukwueke UN, Wen PY. Use of the Response Assessment in Neuro-Oncology (RANO) criteria in clinical trials and clinical practice. CNS Oncol 2019;8:CNS28. [Crossref] [PubMed]
- Stockham AL, Tievsky AL, Koyfman SA, Reddy CA, Suh JH, Vogelbaum MA, Barnett GH, Chao ST. Conventional MRI does not reliably distinguish radiation necrosis from tumor recurrence after stereotactic radiosurgery. J Neurooncol 2012;109:149-58. [Crossref] [PubMed]
- Radbruch A, Fladt J, Kickingereder P, Wiestler B, Nowosielski M, Bäumer P, Schlemmer HP, Wick A, Heiland S, Wick W, Bendszus M. Pseudoprogression in patients with glioblastoma: clinical relevance despite low incidence. Neuro Oncol 2015;17:151-9. [Crossref] [PubMed]
- Carpenter TK, Armitage PA, Bastin ME, Wardlaw JM. DSC perfusion MRI-Quantification and reduction of systematic errors arising in areas of reduced cerebral blood flow. Magn Reson Med 2006;55:1342-9. [Crossref] [PubMed]
- Sourbron S, Ingrisch M, Siefert A, Reiser M, Herrmann K. Quantification of cerebral blood flow, cerebral blood volume, and blood-brain-barrier leakage with DCE-MRI. Magn Reson Med 2009;62:205-17. [Crossref] [PubMed]
- Duyn JH, van Gelderen P, Talagala L, Koretsky A, de Zwart JA. Technological advances in MRI measurement of brain perfusion. J Magn Reson Imaging 2005;22:751-3. [Crossref] [PubMed]
- van Dijken BRJ, van Laar PJ, Holtman GA, van der Hoorn A. Diagnostic accuracy of magnetic resonance imaging techniques for treatment response evaluation in patients with high-grade glioma, a systematic review and meta-analysis. Eur Radiol 2017;27:4129-44. [Crossref] [PubMed]
- Patel P, Baradaran H, Delgado D, Askin G, Christos P, John Tsiouris A, Gupta A. MR perfusion-weighted imaging in the evaluation of high-grade gliomas after treatment: a systematic review and meta-analysis. Neuro Oncol 2017;19:118-27. [Crossref] [PubMed]
- Du YH, Guo ZW, Wang T, Jiang Y, Mu QW. Diagnostic value of arterial spin labeling in differentiating glioma recurrence from radiation-induced brain injury: a Meta-analysis. Chinese Journal of Practical Nervous Diseases 2021;24:966-71.
- Liberati A, Altman DG, Tetzlaff J, Mulrow C, Gøtzsche PC, Ioannidis JP, Clarke M, Devereaux PJ, Kleijnen J, Moher D. The PRISMA statement for reporting systematic reviews and meta-analyses of studies that evaluate health care interventions: explanation and elaboration. J Clin Epidemiol 2009;62:e1-34. [Crossref] [PubMed]
- Baek HJ, Kim HS, Kim N, Choi YJ, Kim YJ. Percent change of perfusion skewness and kurtosis: a potential imaging biomarker for early treatment response in patients with newly diagnosed glioblastomas. Radiology 2012;264:834-43. [Crossref] [PubMed]
- Barajas RF Jr, Chang JS, Segal MR, Parsa AT, McDermott MW, Berger MS, Cha S. Differentiation of recurrent glioblastoma multiforme from radiation necrosis after external beam radiation therapy with dynamic susceptibility-weighted contrast-enhanced perfusion MR imaging. Radiology 2009;253:486-96. [Crossref] [PubMed]
- Cha J, Kim ST, Kim HJ, Kim BJ, Kim YK, Lee JY, Jeon P, Kim KH, Kong DS, Nam DH. Differentiation of tumor progression from pseudoprogression in patients with posttreatment glioblastoma using multiparametric histogram analysis. AJNR Am J Neuroradiol 2014;35:1309-17. [Crossref] [PubMed]
- Choi YJ, Kim HS, Jahng GH, Kim SJ, Suh DC. Pseudoprogression in patients with glioblastoma: added value of arterial spin labeling to dynamic susceptibility contrast perfusion MR imaging. Acta Radiol 2013;54:448-54. [Crossref] [PubMed]
- Chung WJ, Kim HS, Kim N, Choi CG, Kim SJ. Recurrent glioblastoma: optimum area under the curve method derived from dynamic contrast-enhanced T1-weighted perfusion MR imaging. Radiology 2013;269:561-8. [Crossref] [PubMed]
- Elshafeey N, Kotrotsou A, Hassan A, Elshafei N, Hassan I, Ahmed S, Abrol S, Agarwal A, El Salek K, Bergamaschi S, Acharya J, Moron FE, Law M, Fuller GN, Huse JT, Zinn PO, Colen RR. Multicenter study demonstrates radiomic features derived from magnetic resonance perfusion images identify pseudoprogression in glioblastoma. Nat Commun 2019;10:3170. [Crossref] [PubMed]
- Heo YJ, Kim HS, Park JE, Choi CG, Kim SJ. Uninterpretable Dynamic Susceptibility Contrast-Enhanced Perfusion MR Images in Patients with Post-Treatment Glioblastomas: Cross-Validation of Alternative Imaging Options. PLoS One 2015;10:e0136380. [Crossref] [PubMed]
- Hu X, Wong KK, Young GS, Guo L, Wong ST. Support vector machine multiparametric MRI identification of pseudoprogression from tumor recurrence in patients with resected glioblastoma. J Magn Reson Imaging 2011;33:296-305. [Crossref] [PubMed]
- Jovanovic M, Radenkovic S, Stosic-Opincal T, Lavrnic S, Gavrilovic S, Lazovic-Popovic B, Soldatovic I, Maksimovic R. Differentiation between progression and pseudoprogresion by arterial spin labeling MRI in patients with glioblastoma multiforme. J BUON 2017;22:1061-7. [PubMed]
- Kim HS, Goh MJ, Kim N, Choi CG, Kim SJ, Kim JH. Which combination of MR imaging modalities is best for predicting recurrent glioblastoma? Study of diagnostic accuracy and reproducibility. Radiology 2014;273:831-43. [Crossref] [PubMed]
- Kim HS, Suh CH, Kim N, Choi CG, Kim SJ. Histogram analysis of intravoxel incoherent motion for differentiating recurrent tumor from treatment effect in patients with glioblastoma: initial clinical experience. AJNR Am J Neuroradiol 2014;35:490-7. [Crossref] [PubMed]
- Kim TH, Yun TJ, Park CK, Kim TM, Kim JH, Sohn CH, Won JK, Park SH, Kim IH, Choi SH. Combined use of susceptibility weighted magnetic resonance imaging sequences and dynamic susceptibility contrast perfusion weighted imaging to improve the accuracy of the differential diagnosis of recurrence and radionecrosis in high-grade glioma patients. Oncotarget 2017;8:20340-53. [Crossref] [PubMed]
- Kong DS, Kim ST, Kim EH, Lim DH, Kim WS, Suh YL, Lee JI, Park K, Kim JH, Nam DH. Diagnostic dilemma of pseudoprogression in the treatment of newly diagnosed glioblastomas: the role of assessing relative cerebral blood flow volume and oxygen-6-methylguanine-DNA methyltransferase promoter methylation status. AJNR Am J Neuroradiol 2011;32:382-7. [Crossref] [PubMed]
- Manning P, Daghighi S, Rajaratnam MK, Parthiban S, Bahrami N, Dale AM, Bolar D, Piccioni DE, McDonald CR, Farid N. Differentiation of progressive disease from pseudoprogression using 3D PCASL and DSC perfusion MRI in patients with glioblastoma. J Neurooncol 2020;147:681-90. [Crossref] [PubMed]
- Nael K, Bauer AH, Hormigo A, Lemole M, Germano IM, Puig J, Stea B. Multiparametric MRI for Differentiation of Radiation Necrosis From Recurrent Tumor in Patients With Treated Glioblastoma. AJR Am J Roentgenol 2018;210:18-23. [Crossref] [PubMed]
- Ozsunar Y, Mullins ME, Kwong K, Hochberg FH, Ament C, Schaefer PW, Gonzalez RG, Lev MH. Glioma recurrence versus radiation necrosis? A pilot comparison of arterial spin-labeled, dynamic susceptibility contrast enhanced MRI, and FDG-PET imaging. Acad Radiol 2010;17:282-90. [Crossref] [PubMed]
- Park JE, Kim HS, Goh MJ, Kim SJ, Kim JH. Pseudoprogression in Patients with Glioblastoma: Assessment by Using Volume-weighted Voxel-based Multiparametric Clustering of MR Imaging Data in an Independent Test Set. Radiology 2015;275:792-802. [Crossref] [PubMed]
- Prager AJ, Martinez N, Beal K, Omuro A, Zhang Z, Young RJ. Diffusion and perfusion MRI to differentiate treatment-related changes including pseudoprogression from recurrent tumors in high-grade gliomas with histopathologic evidence. AJNR Am J Neuroradiol 2015;36:877-85. [Crossref] [PubMed]
- Qiao Z, Zhao X, Wang K, Zhang Y, Fan D, Yu T, Shen H, Chen Q, Ai L. Utility of Dynamic Susceptibility Contrast Perfusion-Weighted MR Imaging and 11C-Methionine PET/CT for Differentiation of Tumor Recurrence from Radiation Injury in Patients with High-Grade Gliomas. AJNR Am J Neuroradiol 2019;40:253-9. [Crossref] [PubMed]
- Razek AAKA, El-Serougy L, Abdelsalam M, Gaballa G, Talaat M. Differentiation of residual/recurrent gliomas from postradiation necrosis with arterial spin labeling and diffusion tensor magnetic resonance imaging-derived metrics. Neuroradiology 2018;60:169-77. [Crossref] [PubMed]
- Seeger A, Braun C, Skardelly M, Paulsen F, Schittenhelm J, Ernemann U, Bisdas S. Comparison of three different MR perfusion techniques and MR spectroscopy for multiparametric assessment in distinguishing recurrent high-grade gliomas from stable disease. Acad Radiol 2013;20:1557-65. [Crossref] [PubMed]
- Steidl E, Langen KJ, Hmeidan SA, Polomac N, Filss CP, Galldiks N, Lohmann P, Keil F, Filipski K, Mottaghy FM, Shah NJ, Steinbach JP, Hattingen E, Maurer GD. Sequential implementation of DSC-MR perfusion and dynamic 18FFET PET allows efficient differentiation of glioma progression from treatment-related changes. Eur J Nucl Med Mol Imaging 2021;48:1956-65. [Crossref] [PubMed]
- Suh CH, Kim HS, Choi YJ, Kim N, Kim SJ. Prediction of pseudoprogression in patients with glioblastomas using the initial and final area under the curves ratio derived from dynamic contrast-enhanced T1-weighted perfusion MR imaging. AJNR Am J Neuroradiol 2013;34:2278-86. [Crossref] [PubMed]
- Thomas AA, Arevalo-Perez J, Kaley T, Lyo J, Peck KK, Shi W, Zhang Z, Young RJ. Dynamic contrast enhanced T1 MRI perfusion differentiates pseudoprogression from recurrent glioblastoma. J Neurooncol 2015;125:183-90. [Crossref] [PubMed]
- Wang YL, Chen S, Xiao HF, Li Y, Wang Y, Liu G, Lou X, Ma L. Differentiation between radiation-induced brain injury and glioma recurrence using 3D pCASL and dynamic susceptibility contrast-enhanced perfusion-weighted imaging. Radiother Oncol 2018;129:68-74. [Crossref] [PubMed]
- Yun TJ, Park CK, Kim TM, Lee SH, Kim JH, Sohn CH, Park SH, Kim IH, Choi SH. Glioblastoma treated with concurrent radiation therapy and temozolomide chemotherapy: differentiation of true progression from pseudoprogression with quantitative dynamic contrast-enhanced MR imaging. Radiology 2015;274:830-40. [Crossref] [PubMed]
- Zakhari N, Taccone MS, Torres CH, Chakraborty S, Sinclair J, Woulfe J, Jansen GH, Cron GO, Thornhill RE, McInnes MDF, Nguyen TB. Prospective comparative diagnostic accuracy evaluation of dynamic contrast-enhanced (DCE) vs. dynamic susceptibility contrast (DSC) MR perfusion in differentiating tumor recurrence from radiation necrosis in treated high-grade gliomas. J Magn Reson Imaging 2019;50:573-82. [Crossref] [PubMed]
- Hu LX, Zhu J, Tang N, Zhou L, Li ZH, Gong JS, Li XM. Application of MRI ASL in Identifying Pseudothe Science, Technology & Innovation Commission of Shenzhen Municipality-progression of High Grade Glioma after Treatment. Chinese Journal of CT and MRI 2019;17:4-7.
- Qian HF, Sun SJ, Wu X, Li FQ, Li ZY, Hu CH. Role of dynamic contrast-enhanced MRI in distinguishing pseudoprogression from true progression of glioma. Chinese Journal of General Practice 2016;14:441-4.
- Ren LF, Zhang H, Wang XC, Tan Y. Preliminary study of magnetic resonance dynamic contrast enhancement combined with diffusion weighted imaging to identify high grade glioma recurrence and treatment-related changes. Chin J Magn Reson Imaging 2019;10:655-60.
- Sha L. FAN GG, Cao Q. MR perfusion weighted imaging combined with MR diffusion weighted imaging in differentiating recurrent cerebral gliomas and radiation injury. Journal of China Clinic Medical Imaging 2013;24:841-5.
- Shan MY, Yang GQ, Qin JB, Zhang H. Preliminary study of DSC-MRI and IVIM in differentiating postoperative recurrence and radiation brain injury of high-grade glioma. Chin J Magn Reson Imaging 2020;11:326-31.
- Shi HX, Han L, Ye J, Zhang HY. Application of 3D-ASL and DSC-PWI in differential diagnosis of postoperative recurrence and radiation necrosis of high-grade glioma. Oncoradiology 2020;29:324-9.
- Wang JH, Zhang ZY, Li XM, Cheng J, Jiang YH. Application of MRS and ASL in the diagnosis of pseudoprogression in high-grade glioma. Journal of Medical Imaging 2016;26:1153-6.
- Wang QJ, Wang P, Zhang J, Guo Y, Zheng KH. MR perfusion weighted imaging in discrimination between the recurrence and pseudoprogression in high-grade brain glioma. Beijing Medical Journal 2017;39:492-5.
- Xie LL, Xie C, Tu WB, Gao JH, Cheng XR. The Clinical Value of Dynamic Enhanced Magnetic Resonance Imaging in the Differential Diagnosis of Glioma Recurrence and Radionuclide Injury. Chinese Journal of CT and MRI 2019;17:13-5.
- Xing Z, Zeng Z, She DJ, Liu Y, Huang XY, Xiong ML, Zhang YY, Cao DR. Application of Multimodal Functional MR Imaging of 3.0T in Diagnosing Recurrent Glioma and Radiation Necrosis. Journal of Clinical Radiology 2016;35:1309-13.
- Xu ZS, Chen XL, Wang R, Zhao YL, Lu CY. The value of 3.0 T MRI functional imaging in differential diagnosis of radiation brain injury and recurrence of glioblastoma multiforme. West China Medical Journal 2018;33:727-31.
- Yin DY, Zhao Y. Value of MR spectroscopy and MR perfusion imaging in brain tumor recurrence diagnosis. Journal of Modern Oncology 2015;23:1655-8.
- Zhang J, Wang QJ, Zhang J, Guo Y, Zheng KH, Wang P. MR 3D-ASL in discrimination between high-grade brain glioma recurrence and pseudoprogression. Beijing Medical Journal 2019;41:382-4.
- Wang X, Chen D, Qiu J, Li S, Zheng X. The relationship between the degree of brain edema regression and changes in cognitive function in patients with recurrent glioma treated with bevacizumab and temozolomide. Quant Imaging Med Surg 2021;11:4556-68. [Crossref] [PubMed]
- Wilson CB, Crafts D, Levin V. Brain tumors: criteria of response and definition of recurrence. Natl Cancer Inst Monogr 1977;46:197-203. [PubMed]
- Li C, Gan Y, Chen H, Chen Y, Deng Y, Zhan W, Tan Q, Xie C, Sharma HS, Zhang Z. Advanced multimodal imaging in differentiating glioma recurrence from post-radiotherapy changes. Int Rev Neurobiol 2020;151:281-97. [Crossref] [PubMed]
- Abbasi AW, Westerlaan HE, Holtman GA, Aden KM, van Laar PJ, van der Hoorn A. Incidence of Tumour Progression and Pseudoprogression in High-Grade Gliomas: a Systematic Review and Meta-Analysis. Clin Neuroradiol 2018;28:401-11. [Crossref] [PubMed]
- Soliman HM, ElBeheiry AA, Abdel-Kerim AA, Farhoud AH, Reda MI. Recurrent brain tumor versus radiation necrosis; can dynamic susceptibility contrast (DSC) perfusion magnetic resonance imaging differentiate? Egyptian Journal of Radiology and Nuclear Medicine 2018;49:719-26. [Crossref]
- Wang L, Wei L, Wang J, Li N, Gao Y, Ma H, Qu X, Zhang M. Evaluation of perfusion MRI value for tumor progression assessment after glioma radiotherapy: A systematic review and meta-analysis. Medicine (Baltimore) 2020;99:e23766. [Crossref] [PubMed]
- Li R, Liu JH, Wang YL, Lou H, Lin Ma. Comparative study of three dimensional pseudo-continuous arterial spin labeling and dynamic contrast enhanced MRI parameters in preoperative grading of gliomas. Oncoradiology 2016;25:217-22.
- Liu Y, Chen G, Tang H, Hong L, Peng W, Zhang X. Systematic review and meta-analysis of arterial spin-labeling imaging to distinguish between glioma recurrence and post-treatment radiation effect. Ann Palliat Med 2021;10:12488-97. [Crossref] [PubMed]
- Wen PY, Packer RJ. The 2021 WHO Classification of Tumors of the Central Nervous System: clinical implications. Neuro Oncol 2021;23:1215-7. [Crossref] [PubMed]
- Aldape K, Zadeh G, Mansouri S, Reifenberger G, von Deimling A. Glioblastoma: pathology, molecular mechanisms and markers. Acta Neuropathol 2015;129:829-48. [Crossref] [PubMed]
- Hua T, Zhou W, Zhou Z, Guan Y, Li M. Heterogeneous parameters based on 18F-FET PET imaging can non-invasively predict tumor grade and isocitrate dehydrogenase gene 1 mutation in untreated gliomas. Quant Imaging Med Surg 2021;11:317-27. [Crossref] [PubMed]
- Shirahata M, Ono T, Stichel D, Schrimpf D, Reuss DE, Sahm F, et al. Novel, improved grading system(s) for IDH-mutant astrocytic gliomas. Acta Neuropathol 2018;136:153-66. [Crossref] [PubMed]
- Appay R, Dehais C, Maurage CA, Alentorn A, Carpentier C, Colin C, Ducray F, Escande F, Idbaih A, Kamoun A, Marie Y, Mokhtari K, Tabouret E, Trabelsi N, Uro-Coste E, Delattre JY, Figarella-Branger D. POLA Network. CDKN2A homozygous deletion is a strong adverse prognosis factor in diffuse malignant IDH-mutant gliomas. Neuro Oncol 2019;21:1519-28. [Crossref] [PubMed]