18F-FDG PET/CT radiomic analysis for classifying and predicting microvascular invasion in hepatocellular carcinoma and intrahepatic cholangiocarcinoma
Introduction
Liver cancer is a high-risk malignancy which 5-year survival rate is only 10% (1). Hepatocellular carcinoma (HCC) and intrahepatic cholangiocarcinoma (ICC) account for over 95% of primary liver cancer and have significant differences in clinical treatment and prognosis (2-4). Even in those cases when a radical resection is feasible, the probability of intrahepatic recurrent cancer and extrahepatic metastases is still very high (5). HCC and ICC tend to invade vascular structures. Macrovascular invasion (3) refers to tumor invasion of larger vessels, and the most common is the portal vein tumor thrombus (PVTT), while microvascular invasion (MVI) refers to the presence of tumor cells within the portal or hepatic venous system (6). The diagnostic gold standard of MVI positive in histopathology is defined as 5 or more tumor clusters visible within the peritumoral vascular (usually covered by endothelium) only on microscopy (7). Previous studies have identified MVI as a major risk factor for early recurrence after liver resection (8-10). However, lacking effective early diagnostic strategies, and with highly heterogeneous in clinical features and histological morphology (11,12), liver cancer is difficult to distinguish from HCC and ICC as well as identify MVI status before surgery. Biopsy is a solution with invasive examination, yet it increases the risk of metastasis and cannot provide the whole status of tumors (13). Therefore, there is an urgent need for a non-invasive quantitative evaluation method in vivo clinically, which can accurately distinguish pathological subtype and reflect the biological characteristics of the whole tumor before surgery.
Radiomics, served as a quantitative high-throughput analysis method for mining medical images with high dimensional extractable data, has attracted increasing attention in recent years (14-16). Positron emission tomography/computed tomography (PET/CT)-based radiomics combined with medical imaging and molecular imaging could potentially be used to investigate predictive or prognostic biomarkers for tumor diagnosis, treatment, efficacy evaluation and prognosis prediction before surgery (17,18). Some studies (19-21) have shown that PET/CT radiomics applications have obtained encouraging results, for instance, in differentiating benign and malignant tumors, and identifying tumor stages.
Contrast-enhanced ultrasound (CEUS) is commonly used in the differentiation of HCC and ICC (22). Besides, researchers have been making great efforts to find more precise ways to predict MVI status before surgery. MRI is widely used for detection the presence of MVI in ICC and HCC (23,24). Compared to CEUS and MRI, PET/CT scans noninvasively reflect tumor metabolism and molecular level changes in vivo and monitor tumor biological characteristics (25). Cassim et al. stated that most HCC tumor cells were hypermetabolic activity stemming from an increased metabolic plasticity, which can be identified by PET/CT (26). Findlay et al. recently reported that FDG accumulation correlated with the degree of ICC differentiation (27). Hence, PET/CT-based radiomics are expected to have great potential for predicting HCC and ICC type and MVI status with the advantages of high sensitivity, high specificity, repeatability.
Encouraged by the aforementioned promising applications, we attempted to explore 18F-FDG PET/CT imaging’s potential capability in auxiliary diagnosis of its additional application in HCC and ICC classification, as well as detection in MVI presence before surgery. It is efficient and convenient for patients to obtain a comprehensive quantification assessment of liver tumors after a single preoperative 18F-FDG PET/CT examination. Our aim of this article is to build a feasible and robust machine learning model with radiomics biomarkers and clinical characteristics that may provide preoperative prediction of HCC and ICC classification and MVI status in patients with primary liver cancer by using 18F-FDG PET/CT images. We present the following article in accordance with the TRIPOD reporting checklist (available at https://qims.amegroups.com/article/view/10.21037/qims-21-1167/rc).
Methods
Patients
The study was carried out in compliance with the International Guidelines for Human Research Protection of the Declaration of Helsinki (as revised in 2013) and International Conference on Harmonization in Good Clinical Practical (ICH-GCP). This retrospective study was approved by the Ethics Committee of Fudan University Shanghai Cancer Center and individual consent for this retrospective analysis was waived. We collected clinicopathological indicators and PET/CT images of 112 patients (58 females and 54 males) with liver cancer who underwent 18F-FDG PET/CT scan between January 2016 and December 2019 at Fudan University Shanghai Cancer Centre (Shanghai, China).
Inclusion criteria were as follows: (I) pathological diagnosis of either HCC or ICC confirmed by partial hepatectomy of primary liver lesion; (II) validation of 18F-FDG PET/CT scan images within two weeks before surgery; (III) with normal hematologic, renal, and hepatic function; (IV) complete clinical characteristics and pathology immunohistochemistry results. Exclusion criteria included: (I) metastatic liver tumor; (II) preoperative PET/CT showed portal vein tumor thrombosis (PVTT); (III) incomplete clinical characteristics and pathology immunohistochemistry results, including only performed liver biopsy; (IV) blood glucose levels over 7.78 mmol/L or with abnormal laboratory indexes.
Preoperative tumor staging followed the Barcelona Clinic Liver Cancer (BCLC) criteria revised by American Association for the Study of Liver Diseases (AASLD) in 2010. Postoperative pathological classification and the presence of MVI and number of satellite node were confirmed by two experienced pathologists. MVI positive is defined as 5 or more tumor clusters visible within the peritumoral vascular (usually covered by endothelium) only on microscopy. The selected serum alpha-fetoprotein (AFP), carbohydrate antigen 19-9 (CA19-9) levels were measured within one week before surgery. The threshold value of serum AFP and CA19-9 level was 20 ng/mL and 37 U/mL respectively.
PET/CT imaging acquisition and reconstruction parameters
18F-FDG was produced by an RDS Eclipse ST medical cyclotron (Siemens Healthiness, Knoxville, TN, USA) and an Explore FDG4 synthesis module.18F-FDG radiochemical purity was >95%. Blood glucose levels of all patients were less than 7.78 mmol/L. Patients fasted for at least 6 hours prior to injection. After intravenous administration of 18F-FDG (3.7 MBq/kg), all patients laid in a bed for one hour and imaged by a Biograph 16HR PET/CT scanner (Siemens Medical Systems, Erlangen, Germany). First, an unenhanced low-dose whole-body CT scan was performed from head to the top of the thighs (tube voltage, 120 kV; tube current, 80–250 mA; rotation time, 0.5 s; helical pitch 3.6; slice thickness, 5 mm; matrix, 512×512). Images were performed for attenuation correction. Then, whole-body PET scan was acquired over the same extent at three minutes per bed position for a total of 6–7 bed positions. PET data were reconstructed using iterative protocols with gaussian-filter iterative method (iterations, 4; subsets, 8). The PET and CT images were imported into the Siemens workstation for analysis.
Volume of interest (VOI) segmentation and image delineation
To provide an accurate segmentation, the VOI of primary liver tumors was first semi-automatically delineated using the GrowCut algorithm (28) implemented on 3D Slicer (https://www.slicer.org) based on PET standardized-uptake-value (SUV) data, which shows high reproducibility. For the instances where SUV data of tumors were similar with adjacent structures, LLC model (29) and an improved edge detector were used to separate the tumor from the background and highlight the regions with weak boundaries. All results were corrected by manual adjustment and validated independently by two senior nuclear medical physicians to ensure reproducibility and reliability. All masks were reshaped to the same pixel spacing as original PET-CT images and checked based on PET-CT fusion image on 3D Slicer. Besides, pixel value of PET image was replaced by SUVbw to eliminate the effects of patients’ absorption differences. Conventional PET metrics were also considered as radiomics features. On the 18F-FDG-PET, the SUVmax (standardized uptake value of the highest-uptake voxel within a VOI) and MTV (metabolic tumor volume) were automatically calculated on the Siemens workstation. TLG was calculated as follows: TLG = MTV×SUVmean.
Radiomics feature selection and machine learning
The workflow of radiomic analysis by machine learning method is shown in Figure 1, which consists of four key steps. At first, we obtain discriminative radiomics features from VOIs by using reproducible feature selection method; then the supervised machine learning classifier was constructed by random forest algorithm, which contributed to two tasks: HCC and ICC classification and MVI prediction; besides, we analysed potential correlations between radiomics and clinical features as well as each feature’s contribution to model’s results. Especially, for MVI prediction task, we divided all patients into HCC group and ICC group before feature selection step, then trained the MVIs identification models for each group separately.

Totally 1,815 radiomics features including 918 CT features and 897 PET features were extracted for each patient. PyRadiomics (version 3.0), an open-source Python software package was used to pre-process image and extract features, which is compliant with the Imaging biomarker standardization initiative as well. The matrix size of CT was 512×512 with the voxel size 1.0×1.0×3.3 mm3. The matrix size of PET was 128×128 with the voxel size 5.5×5.5×3.3 mm3. The images were discretized with a fixed bin size of 40 HU and 30 of SUV, and the mask images were resampled to the same pixel spacing as PET and CT images. From this package, we applied three filters for each PET and CT image before extraction: original channel, Laplacian of Gaussian (LoG) channel and wavelet channel. The extracted features reflected tumors’ traits including intensity distribution, morphological characteristic, and texture pattern. The intensity feature is a first-order feature, which includes the maximum, mean, and average absolute deviation of the voxel values. The shape feature includes tumors’ geometry properties such as edges and angles. Texture feature is a second-order feature and is used to express tumor’s heterogeneity by the distribution of some common matrix, i.e., Gray Level Co-occurrence Matrix (GLCM), Gray Level Size Zone Matrix (GLSZM), Gray Level Run Length Matrix (GLRLM), and Gray Level Dependence Matrix (GLDM). High-order feature includes first-order features, second-order features and texture features from LoG and wavelet images, which aimed to reduce noises and obtain the subtle information from image at different frequency domains (30).
At feature selection part (Figure 2), we aimed to build a reproducible feature set. Take features’ high dimensionality into consideration, we first eliminated statistical insignificant by Wilcoxon test (P<0.05 was considered significant). Then we used uni-variable random forest feature selection to choose relevant features. Key features with tree importance greater than 0.001 were included. To further keep feature set discriminative, we use partwise Pearson Correlation matrix. We first identified pairs of related features (|r| ≥0.7 for PET and CT features), then the feature with higher prediction ability (higher AUC using random forest classifier) will be included. Next, we utilize Sequential Forward Floating Algorithm (31-33) to recursively find optimal feature combinations and avoid overfitting. We perform same steps for both HCC and ICC classification task and MVI prediction task in feature selection.
Modelling and validation
The model was evaluated with cross validation and independent validation to achieve robustness and stability (Figure 3). For HCC and ICC classification task (127 patients in total), they were randomly split into training (100 out of 127) and validation (27 out of 127) cohort. For MVI prediction in HCC (76 patients in total), there were 60 patients for training and 16 patients for validation; for MVI prediction in ICC (51 patients in total), there were 40 patients for training and 11 patients for validation. The proportion of positive and negative samples in the training and test sets is roughly the same as the proportion in the original dataset.
The feature selection procedure and random forest classifier were built on the entire training cohort, at which the hyper parameters were assured as well. To check models’ performances, 20 times of stratified 10-fold cross-validations were performed on training cohort. To access machine learning models’ performances, we used receiver operating characteristic curve (9), sensitivity, specificity, positive predictive value (PPV), and negative predictive value (NPV) in the independent cohort. Statistical analyses were performed with ‘Scipy 1.3.0’, and ‘math’ packages in Python 3.6.8 programming language and environment.
Results
Clinical characteristics of patients
Patients’ characters with split details are shown in Tables 1,2. Totally 112 (55±28 years old) patients were included. The clinical indicators include AFP, CA19-9, age, tumor size, stage, tumor amount, and number of satellite nodes.
Table 1
Demographic and clinical characteristics | Total | HCC/ICC | P value |
---|---|---|---|
Number of patients | 127 | 76/51 | – |
Age (years), median (range) | 55±28 | 54±28/61±25 | 0.211 |
Gender | 0.194 | ||
Male | 79 | 58/21 | |
Female | 33 | 11/22 | |
AFP (ng/mL) | 0.029 | ||
≥20 | 39 | 37/2 | |
<20 | 73 | 33/40 | |
CA19-9 (U/mL) | 0.802 | ||
≤37 | 74 | 52/22 | |
>37 | 38 | 18/20 | |
Tumor size (mm) | 0.001 | ||
3 | 21 | 16/5 | |
5 | 34 | 23/11 | |
10 | 38 | 17/21 | |
>10 | 21 | 14/7 | |
Tumor stage | 0.001 | ||
A | 33 | 25/8 | |
B | 46 | 32/14 | |
C | 33 | 13/20 | |
Tumor amount | 0.112 | ||
Multiple | 12 | 10/2 | |
Single | 100 | 60/40 | |
Satellite node | 0.154 | ||
None | 81 | 44/37 | |
1–3 | 41 | 26/15 |
AFP, alpha-fetoprotein; CA19-9, carbohydrate antigen 19-9; HCC, hepatocellular carcinoma; ICC, intrahepatic cholangiocarcinoma.
Table 2
Demographic and clinical characteristics | Total | MVI present/MVI absent | P value |
---|---|---|---|
Number of patients | 127 | 77/50 | – |
Age (years), median (range) | 55±28 | 55±28/56±27 | 0.486 |
Gender | 0.347 | ||
Male | 79 | 48/31 | |
Female | 33 | 16/17 | |
AFP (ng/mL) | 0.1 | ||
≥20 | 39 | 21/18 | |
<20 | 73 | 43/30 | |
CA19-9 (U/mL) | 0.399 | ||
≤37 | 74 | 43/31 | |
>37 | 38 | 21/17 | |
Tumor size (mm) | 0.0009 | ||
3 | 21 | 9/12 | |
5 | 34 | 16/18 | |
10 | 38 | 25/13 | |
>10 | 21 | 14/7 | |
Tumor stage | 0.090 | ||
A | 33 | 11/22 | |
B | 46 | 30/16 | |
C | 33 | 23/10 | |
Tumor amount | 0.031 | ||
Multiple | 12 | 10/2 | |
Single | 100 | 54/46 | |
Satellite node | 0.359 | ||
None | 81 | 32/49 | |
1–3 | 41 | 32/9 |
AFP, alpha-fetoprotein; CA19-9, carbohydrate antigen 19-9; MVI, microvascular invasion.
Radiomics features and performance of predictions
The most predictive feature combinations selected by feature engineering and corresponding explanation are shown in Table 3.
Table 3
Task | Feature name | Feature explanation |
---|---|---|
Classify HCC and ICC | Wavelet-LHL_Median_ct | The median gray level intensity within the VOI. A higher value means higher density in the image |
Wavelet-HHL_Variance_pet | Variance is the mean of the squared distances of each intensity value from the mean value. This is a measure of the spread of the distribution about the mean | |
Formula: [1] | ||
Log-sigma-3-0-mm-3D_shortrunhighgraylevelemphasis_pet | SRHGLE measures the joint distribution of shorter run lengths with higher gray-level values | |
Formula: [2] | ||
MVI: HCC | Log-sigma-3-0-mm-3D_Range_pet | The range of gray values in the VOI |
Formula: [3] | ||
Wavelet-HHH_totalenergy_pet | Total energy is the value of energy feature scaled by the volume of the voxel in cubic mm | |
Formula: [4] | ||
Wavelet-LLH_Entropy_pet | Here, 𝜖 is an arbitrarily small positive number (≈2.2×10−16≈2.2×10−16). Entropy specifies the uncertainty/randomness in the image values. It measures the average amount of information required to encode the image values. | |
Formula: [5] | ||
MVI: ICC | Wavelet-HLL_Minimum_pet | 𝐗 be a set of 𝑁𝑝 voxels included in the VOI |
Formula: [6] | ||
Wavelet-HLL_totalenergy_pet | The same as wavelet-HHH_TotalEnergy_pet | |
Formula: [7] |
HCC, hepatocellular carcinoma; ICC, intrahepatic cholangiocarcinoma; LHL, low, high, and low frequency; VOI, volume of interest; SRHGLE, short run high gray level emphasis; MVI, microvascular invasion; HHH, HHH, high, high, and high frequency; LLH, low, low, and high frequency; HLL, high, low, and low frequency.
We compare five categories of features: (I) clinical characteristic only; (II) optimal CT features only; (III) optimal PET features only; (IV) optimal PET and CT features combination; (V) best PET, CT, and clinical characteristic combination that selected by feature engineering. Figure 4 shows the results of model performances in testing cohort in five categories. There were 2 PET features and one CT features that achieved the most prognostic value when working with HCC and ICC classification task (AUC =0.86). As to MVI prediction tasks, three PET features and tumor stage shown great ability in HCC group (AUC =0.88), meanwhile two PET features and CA19-9 performed well in ICC group (AUC =0.90).
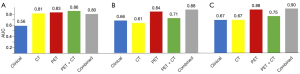
For HCC and ICC classification task, PET features show an AUC of 0.83. CT features also showed valuable information (AUC =0.81), enhancing the results of PET-CT features combination (AUC =0.86). But clinical features failed to give useful information (AUC =0.56), which was worse than the results of combination as well (AUC =0.80).
On the other hand, the results of MVI prediction tasks show that PET and clinical features outperform than CT features. For HCC in MVI task, three PET features plus one clinical feature (tumor stage) gave model highest AUC at 0.88. PET features only can achieve AUC of 0.84, but CT features have AUC of 0.61. Since CT features’ AUC is much lower than PET’s, they not only fail to give useful information, but worsening the results of PET-CT features (AUC =0.71).
For ICC in MVI task, two PET features and CA19-9 can achieve AUC of 0.90. PET features also gave impressive AUC of 0.88. Meanwhile clinical features and CT features have AUC of 0.67 and 0.66 respectively, which are unable to give results in high accuracy, worsening the results of combinations. Table 4 shows detailed performances in optimal feature category for three tasks.
Table 4
Task | AUC | Accuracy | Sensitivity | Specificity | NPV | PPV |
---|---|---|---|---|---|---|
HCC and ICC classification | 0.86 | 0.82 | 0.78 | 0.88 | 0.91 | 0.88 |
MVI (HCC) | 0.88 | 0.78 | 0.88 | 0.60 | 0.80 | 0.60 |
MVI (ICC) | 0.90 | 0.77 | 0.75 | 0.80 | 0.75 | 0.80 |
AUC, area under the curve; NPV, negative predictive value; PPV, positive predictive value; HCC, hepatocellular carcinoma; ICC, intrahepatic cholangiocarcinoma; MVI, microvascular invasion.
We also analysed the radiomics features’ category. As it illustrated in Figures 5,6, for all three tasks, PET features outperformed than CT features because only one CT feature was included. Besides, due to the spatial resolution of PET/CT is relatively low, and it has less advantages in defining the tumor boundaries all selected radiomics features were intensity as well as texture feature, which means shape features failed to give predictive information for both tasks. In Figure 7, we show four representative patient examples.
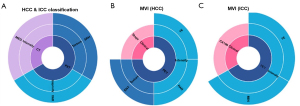
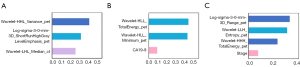
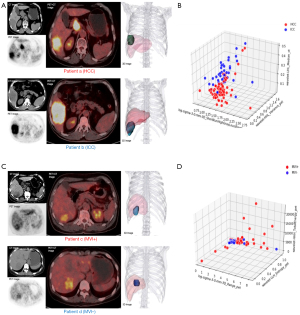
Correlation with clinical and conventional PET features
We also applied Pearson correlation matrix to discovery potential relationships between radiomics features and clinical as well as conventional PET features. Figure 8 shows the results of Pearson partwise correlations with four conventional PET features and six clinical features. P<0.05 was considered significant. We found that for all three tasks, the selected feature combinations had significant relationships with metabolic indicators, tumor size and tumor stage.
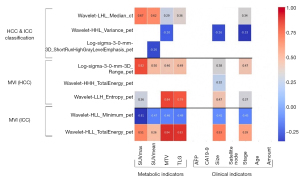
Discussion
HCC and ICC are two common subtypes in primary liver cancer with distinctive prognosis (34). The metastasis and recurrence are the two major obstacles to improve the prognosis of liver cancer patients. More importantly, MVI status is an indicator of tumor’s aggressiveness and an independent risk factor of metastasis and recurrence (14). Hence, to provide precise information and appropriate treatment, the prediction of HCC and ICC classification and MVI statues before surgery is crucial. CEUS is commonly used in HCC and ICC differentiation and achieves an AUC of 0.92 (22), and MRI for MVI detection can achieve an AUC of 0.86 for HCC (23), an AUC of 0.81 for ICC (25). Though the specialized medical imaging could give relatively high accuracy in detection, it is a great burden for patients to do many examinations. Encouraged by PET’s promising applications, we aimed to explore whether 18F-FDG PET/CT imaging could provide a potential possibility for playing an auxiliary diagnosis and additional contribution for HCC and ICC classification and MVI before surgery, so that patients can obtain the comprehensive quantification of tumor phenotypes after a single preoperative 18F-FDG PET/CT examination for guiding oncologists or surgeons to establish a personalized therapeutic strategy. Generally, there are two findings in our study: we constructed a prediction model for HCC and ICC classification and MVI statues in primary liver cancer based on 18F-FDG PET/CT radiomics features and clinical factors. Moreover, we found that PET features had an impressive prediction capability in HCC and ICC classification and MVI, which outperformed than CT and clinical characteristics.
Raman et al. (35) described that radiomics features using computed tomography texture analysis can detect different liver lesion types and normal liver tissue. Our results showed that PET and CT radiomics features achieved AUC of 0.86 (compared to 0.92 in CEUS) and a specificity of 0.88 for HCC and ICC classification, which has potential for informative reference. We also found that factors related to tumor intensity and texture were the most important components in predicting histological classification. This is partly in agreement with the findings of Wu et al. (36). Specifically, tumor intensity and texture features can reflect subtle information from PET/CT images. For instance, median and variance represented tumor area’s degree of heterogeneity. Short Run High Gray Level Emphasis, as a texture feature, revealed joint distribution of dark small areas in VOIs. PET/CT radiomics provides molecular-based image features and intratumoral heterogeneity (37), which could be an effective diagnostic tool in histological classification for primary liver cancers. This finding contributes to to the evaluation of differentiation between HCC and ICC, especially in cases that differentiation using conventional medical imaging methods is difficult.
Previous studies (38,39) validated that MVI worsened the prognosis of liver cancer. Emerging studies have focused on the relationships of contrast-enhanced CT features and MVI status (37,40). But prediction model of MVI based PET/CT radiomics features has never been reported. In our study, three PET features integrating tumor clinical stage in HCC and two PET features integrating one clinical factor in ICC were selected for MVI prediction. The compound (PET, CT, and clinical characteristic) radiomics predictors can identify more than 0.77 of the MVI− positive cases with the AUC of 0.88–0.90 (compared to 0.86 and 0.81 in MRI). Our model exhibited better performance with the MRI model in both HCC and ICC. Besides, PET features were more important than clinical features, and intensity features seemed to perform better than texture features. One possible interpretation is that tumor intensity and texture features implied a range of discrete tumor activity and intratumor heterogeneity. Another is that the spatial resolution of PET/CT is relatively low, and it has less advantages in defining the tumor boundaries. The greater values of these factors, the higher probability of MVI. This finding is consistent with the previous report (41) that the radiomics signature, nonsmoothed tumor margin, hypoattenuating halos and internal arteries were significantly associated with MVI status. We also found a positive association between some higher-order PET radiomics features (range, total energy, total energy) and the 18F-FDG uptake activity (SUVmax, SUVmean, TLG) of the lesion. This part of results is important since it may be an indicator of disease extent and tumor staging, especially in cases where evaluation using conventional clinical imaging methods might have been overlooked.
Notably, our model exhibited equivalent or superior performance with the CEUS and MRI model in HCC and ICC classification and MVI prediction. Therefore, 18F-FDG PET/CT imaging would contribute to its key role in evaluating and staging tumors and potential value in differentiating ICC and HCC and detecting MVI before surgery, which could help to provide an earlier indication of liver cancer to select a more appropriate treatment and relieve the medical burden of patients. Interestingly, our model revealed that PET features had dominant predictive power in HCC and ICC classification and MVI, which outperformed than CT and clinical characteristics. In this study only one CT feature was selected by in HCC and ICC classification task. The reasons may be as follows: CT scanning in PET/CT is unenhanced low-dose CT, which only provides limited information and is not capable for sufficient tumor detection or distinction. While PET reflects the metabolic activity of a whole tumor (42). Though the importance of features in unenhanced low-dose CT has been validated in many studied (43,44), such as HCC surveillance analysis (45), esophageal cancer (46) and lymphoma (47), the value of CT features on HCC and ICC differentiation still requires a larger sample for further validation. Further, CT features were not included in the MVI model. One potential explanation is that MVI detection is relevant to find out the presence of tumor cells in inside portal or hepatic venous systems (6), and most hepatic cancer cells were hypermetabolic activity stemming from an increased metabolic plasticity, which can be identified by PET/CT scan, especially in PET images. Hence unenhanced low-dose CT is insufficient in MVI detection in this study.
Our study has some limitations. Firstly, most clinical characteristics of patients cannot contribute to the accuracy of the predictive model. It might be attributed to the small sample and a potential selection bias in this single-center retrospective study. Multicenter and larger clinical studies are necessary to be designed for validating our radiomics model. Nonetheless, our findings are still reasonable and important. According to Cochran’s formula (48): if it’s assumed 50% of patients are positive in 95% confidence level and 5% margin error, the ideal sample size is 382. Besides, machine learning models require around 50 patients for algorithm’s training and validation to avoiding overfitting. Further, for PET/CT studies, Chalkidou et al. (49) found that for one radiomic feature, 10 to 15 patients are the minimum requirement. For three different tasks, though the idea sample size of 382 wasn’t achieved, our feature selection model reduced the number of features to 3 (out of 127 patients), 4 (out of 76 patients) and 2 (out of 51 patients), which suggests that our results are relatively valid with the minimum false detection rate. In addition, prognosis information with histologic MVI were not collected to investigate the predictive effectiveness of the model. The prediction model based on 18F-PET/CT radiomics features that widely used for liver cancer will be continually explored in future studies.
Conclusions
18F-FDG PET/CT radiomics features integrating clinical factors have potential in HCC and ICC classification and MVI prediction, while PET features have dominant predictive power in model performance. Patients may obtain the comprehensive quantification of tumor phenotypes after a single preoperative 18F-FDG PET/CT examination for guiding oncologists or surgeons to establish a personalized therapeutic strategy.
Acknowledgments
Funding: This work was partially supported by the National Natural Science Foundation of China (Nos. 81771861, 81471708); 2018 Shanghai Scientific and Technological Innovation Program (No. 18410711200).
Footnote
Reporting Checklist: The authors have completed the TRIPOD reporting checklist. Available at https://qims.amegroups.com/article/view/10.21037/qims-21-1167/rc
Conflicts of Interest: All authors have completed the ICMJE uniform disclosure form (available at https://qims.amegroups.com/article/view/10.21037/qims-21-1167/coif). The authors report that this work was partially supported by the National Natural Science Foundation of China (Nos. 81771861, 81471708); 2018 Shanghai Scientific and Technological Innovation Program (No. 18410711200). The authors have no other conflicts of interest to declare.
Ethical Statement: The authors are accountable for all aspects of the work in ensuring that questions related to the accuracy or integrity of any part of the work are appropriately investigated and resolved. The study was carried out in compliance with the International Guidelines for Human Research Protection of the Declaration of Helsinki (as revised in 2013) and International Conference on Harmonization in Good Clinical Practical (ICH-GCP). This retrospective study was approved by the Ethics Committee of Fudan University Shanghai Cancer Center and individual consent for this retrospective analysis was waived.
Open Access Statement: This is an Open Access article distributed in accordance with the Creative Commons Attribution-NonCommercial-NoDerivs 4.0 International License (CC BY-NC-ND 4.0), which permits the non-commercial replication and distribution of the article with the strict proviso that no changes or edits are made and the original work is properly cited (including links to both the formal publication through the relevant DOI and the license). See: https://creativecommons.org/licenses/by-nc-nd/4.0/.
References
- Bray F, Ferlay J, Soerjomataram I, Siegel RL, Torre LA, Jemal A. Global cancer statistics 2018: GLOBOCAN estimates of incidence and mortality worldwide for 36 cancers in 185 countries. CA Cancer J Clin 2018;68:394-424. [Crossref] [PubMed]
- Rizvi S, Gores GJ. Emerging molecular therapeutic targets for cholangiocarcinoma. J Hepatol 2017;67:632-44. [Crossref] [PubMed]
- Mavros MN, Economopoulos KP, Alexiou VG, Pawlik TM. Treatment and Prognosis for Patients With Intrahepatic Cholangiocarcinoma: Systematic Review and Meta-analysis. JAMA Surg 2014;149:565-74. [Crossref] [PubMed]
- Armengol C, Sarrias MR, Sala M. Hepatocellular carcinoma: Present and future. Med Clin (Barc) 2018;150:390-7. [Crossref] [PubMed]
- Tabrizian P, Jibara G, Shrager B, Schwartz M, Roayaie S. Recurrence of hepatocellular cancer after resection: patterns, treatments, and prognosis. Ann Surg 2015;261:947-55. [Crossref] [PubMed]
- Sumie S, Kuromatsu R, Okuda K, Ando E, Takata A, Fukushima N, Watanabe Y, Kojiro M, Sata M. Microvascular invasion in patients with hepatocellular carcinoma and its predictable clinicopathological factors. Ann Surg Oncol 2008;15:1375-82. [Crossref] [PubMed]
- Rodríguez-Perálvarez M, Luong TV, Andreana L, Meyer T, Dhillon AP, Burroughs AK. A systematic review of microvascular invasion in hepatocellular carcinoma: diagnostic and prognostic variability. Ann Surg Oncol 2013;20:325-39. [Crossref] [PubMed]
- Lim KC, Chow PK, Allen JC, Chia GS, Lim M, Cheow PC, Chung AY, Ooi LL, Tan SB. Microvascular invasion is a better predictor of tumor recurrence and overall survival following surgical resection for hepatocellular carcinoma compared to the Milan criteria. Ann Surg 2011;254:108-13. [Crossref] [PubMed]
- Renzulli M, Buonfiglioli F, Conti F, Brocchi S, Serio I, Foschi FG, Caraceni P, Mazzella G, Verucchi G, Golfieri R, Andreone P, Brillanti S. Imaging features of microvascular invasion in hepatocellular carcinoma developed after direct-acting antiviral therapy in HCV-related cirrhosis. Eur Radiol 2018;28:506-13. [Crossref] [PubMed]
- Tang Z, Liu WR, Zhou PY, Ding ZB, Jiang XF, Wang H, Tian MX, Tao CY, Fang Y, Qu WF, Dai Z, Qiu SJ, Zhou J, Fan J, Shi YH. Prognostic Value and Predication Model of Microvascular Invasion in Patients with Intrahepatic Cholangiocarcinoma. J Cancer 2019;10:5575-84. [Crossref] [PubMed]
- Hiley C, de Bruin EC, McGranahan N, Swanton C. Deciphering intratumor heterogeneity and temporal acquisition of driver events to refine precision medicine. Genome Biol 2014;15:453. [Crossref] [PubMed]
- Lu LC, Hsu CH, Hsu C, Cheng AL. Tumor Heterogeneity in Hepatocellular Carcinoma: Facing the Challenges. Liver Cancer 2016;5:128-38. [Crossref] [PubMed]
- Sherman M, Bruix J. Biopsy for liver cancer: how to balance research needs with evidence-based clinical practice. Hepatology 2015;61:433-6. [Crossref] [PubMed]
- Aerts HJ. The Potential of Radiomic-Based Phenotyping in Precision Medicine: A Review. JAMA Oncol 2016;2:1636-42. [Crossref] [PubMed]
- Larue RT, Defraene G, De Ruysscher D, Lambin P, van Elmpt W. Quantitative radiomics studies for tissue characterization: a review of technology and methodological procedures. Br J Radiol 2017;90:20160665. [Crossref] [PubMed]
- Ochiai T, Ikoma H, Okamoto K, Kokuba Y, Sonoyama T, Otsuji E. Clinicopathologic features and risk factors for extrahepatic recurrences of hepatocellular carcinoma after curative resection. World J Surg 2012;36:136-43. [Crossref] [PubMed]
- Hatt M, Tixier F, Visvikis D, Cheze Le Rest C. Radiomics in PET/CT: More Than Meets the Eye? J Nucl Med 2017;58:365-6. [Crossref] [PubMed]
- Antunovic L, De Sanctis R, Cozzi L, Kirienko M, Sagona A, Torrisi R, Tinterri C, Santoro A, Chiti A, Zelic R, Sollini M. PET/CT radiomics in breast cancer: promising tool for prediction of pathological response to neoadjuvant chemotherapy. Eur J Nucl Med Mol Imaging 2019;46:1468-77. [Crossref] [PubMed]
- Liu Q, Sun D, Li N, Kim J, Feng D, Huang G, Wang L, Song S. Predicting EGFR mutation subtypes in lung adenocarcinoma using 18F-FDG PET/CT radiomic features. Transl Lung Cancer Res 2020;9:549-62. [Crossref] [PubMed]
- Li P, Wang X, Xu C, Liu C, Zheng C, Fulham MJ, Feng D, Wang L, Song S, Huang G. 18F-FDG PET/CT radiomic predictors of pathologic complete response (pCR) to neoadjuvant chemotherapy in breast cancer patients. Eur J Nucl Med Mol Imaging 2020;47:1116-26. [Crossref] [PubMed]
- Kirienko M, Cozzi L, Antunovic L, Lozza L, Fogliata A, Voulaz E, Rossi A, Chiti A, Sollini M. Prediction of disease-free survival by the PET/CT radiomic signature in non-small cell lung cancer patients undergoing surgery. Eur J Nucl Med Mol Imaging 2018;45:207-17. [Crossref] [PubMed]
- Chen LD, Ruan SM, Liang JY, Yang Z, Shen SL, Huang Y, Li W, Wang Z, Xie XY, Lu MD, Kuang M, Wang W. Differentiation of intrahepatic cholangiocarcinoma from hepatocellular carcinoma in high-risk patients: A predictive model using contrast-enhanced ultrasound. World J Gastroenterol 2018;24:3786-98. [Crossref] [PubMed]
- Qian X, Lu X, Ma X, Zhang Y, Zhou C, Wang F, Shi Y, Zeng M. A Multi-Parametric Radiomics Nomogram for Preoperative Prediction of Microvascular Invasion Status in Intrahepatic Cholangiocarcinoma. Front Oncol 2022;12:838701. [Crossref] [PubMed]
- Nebbia G, Zhang Q, Arefan D, Zhao X, Wu S. Pre-operative Microvascular Invasion Prediction Using Multi-parametric Liver MRI Radiomics. J Digit Imaging 2020;33:1376-86. [Crossref] [PubMed]
- Na SJ, Oh JK, Hyun SH, Lee JW, Hong IK, Song BI, Kim TS, Eo JS, Lee SW, Yoo IR, Chung YA, Yun M. 18F-FDG PET/CT Can Predict Survival of Advanced Hepatocellular Carcinoma Patients: A Multicenter Retrospective Cohort Study. J Nucl Med 2017;58:730-6. [Crossref] [PubMed]
- Cassim S, Raymond VA, Dehbidi-Assadzadeh L, Lapierre P, Bilodeau M. Metabolic reprogramming enables hepatocarcinoma cells to efficiently adapt and survive to a nutrient-restricted microenvironment. Cell Cycle 2018;17:903-16. [Crossref] [PubMed]
- Findlay JM, Antonowicz S, Segaran A, El Kafsi J, Zhang A, Bradley KM, Gillies RS, Maynard ND, Middleton MR. Routinely staging gastric cancer with 18F-FDG PET-CT detects additional metastases and predicts early recurrence and death after surgery. Eur Radiol 2019;29:2490-8. [Crossref] [PubMed]
- Zhu L, Kolesov I, Gao Y, Kikinis R, Tannenbaum A. An effective interactive medical image segmentation method using fast grow cut. MICCAI workshop on interactive medical image computing 2014. Available online: https://nac.spl.harvard.edu/publications/effective-interactive-medical-image-segmentation-method-using-fast-growcut
- Li C, Wang X, Eberl S, Fulham M, Yin Y, Chen J, Feng DD. A likelihood and local constraint level set model for liver tumor segmentation from CT volumes. IEEE Trans Biomed Eng 2013;60:2967-77. [Crossref] [PubMed]
- van Griethuysen JJM, Fedorov A, Parmar C, Hosny A, Aucoin N, Narayan V, Beets-Tan RGH, Fillion-Robin JC, Pieper S, Aerts HJWL. Computational Radiomics System to Decode the Radiographic Phenotype. Cancer Res 2017;77:e104-7. [Crossref] [PubMed]
- Ferri FJ, Pudil P, Hatef M, Kittler J. Comparative study of techniques for large-scale feature selection. Machine Intelligence and Pattern Recognition. Elsevier, 1994:403-13.
- Haralick RM, Shapiro LG. Computer and robot vision. Addison-Wesley Reading, 1992.
- Pudil P, Novovicová J, Kittler J. Floating search methods in feature selection. Pattern Recognit Lett 1994;15:1119-25. [Crossref]
- Zhou M, Wang H, Zeng X, Yin P, Zhu J, Chen W, et al. Mortality, morbidity, and risk factors in China and its provinces, 1990-2017: a systematic analysis for the Global Burden of Disease Study 2017. Lancet 2019;394:1145-58. [Crossref] [PubMed]
- Raman SP, Schroeder JL, Huang P, Chen Y, Coquia SF, Kawamoto S, Fishman EK. Preliminary data using computed tomography texture analysis for the classification of hypervascular liver lesions: generation of a predictive model on the basis of quantitative spatial frequency measurements--a work in progress. J Comput Assist Tomogr 2015;39:383-95. [Crossref] [PubMed]
- Wu M, Tan H, Gao F, Hai J, Ning P, Chen J, Zhu S, Wang M, Dou S, Shi D. Predicting the grade of hepatocellular carcinoma based on non-contrast-enhanced MRI radiomics signature. Eur Radiol 2019;29:2802-11. [Crossref] [PubMed]
- Banerjee S, Wang DS, Kim HJ, Sirlin CB, Chan MG, Korn RL, Rutman AM, Siripongsakun S, Lu D, Imanbayev G, Kuo MD. A computed tomography radiogenomic biomarker predicts microvascular invasion and clinical outcomes in hepatocellular carcinoma. Hepatology 2015;62:792-800. [Crossref] [PubMed]
- Eguchi S, Takatsuki M, Hidaka M, Soyama A, Tomonaga T, Muraoka I, Kanematsu T. Predictor for histological microvascular invasion of hepatocellular carcinoma: a lesson from 229 consecutive cases of curative liver resection. World J Surg 2010;34:1034-8. [Crossref] [PubMed]
- Imamura H, Matsuyama Y, Tanaka E, Ohkubo T, Hasegawa K, Miyagawa S, Sugawara Y, Minagawa M, Takayama T, Kawasaki S, Makuuchi M. Risk factors contributing to early and late phase intrahepatic recurrence of hepatocellular carcinoma after hepatectomy. J Hepatol 2003;38:200-7. [Crossref] [PubMed]
- Ma X, Wei J, Gu D, Zhu Y, Feng B, Liang M, Wang S, Zhao X, Tian J. Preoperative radiomics nomogram for microvascular invasion prediction in hepatocellular carcinoma using contrast-enhanced CT. Eur Radiol 2019;29:3595-605. [Crossref] [PubMed]
- Peng J, Zhang J, Zhang Q, Xu Y, Zhou J, Liu L. A radiomics nomogram for preoperative prediction of microvascular invasion risk in hepatitis B virus-related hepatocellular carcinoma. Diagn Interv Radiol 2018;24:121-7. [Crossref] [PubMed]
- Boellaard R, Delgado-Bolton R, Oyen WJ, Giammarile F, Tatsch K, Eschner W, et al. FDG PET/CT: EANM procedure guidelines for tumour imaging: version 2.0. Eur J Nucl Med Mol Imaging 2015;42:328-54. [Crossref] [PubMed]
- Liu J, Yang X, Li Y, Xu H, He C, Qing H, Ren J, Zhou P. Development and validation of qualitative and quantitative models to predict invasiveness of lung adenocarcinomas manifesting as pure ground-glass nodules based on low-dose computed tomography during lung cancer screening. Quant Imaging Med Surg 2022;12:2917-31. [Crossref] [PubMed]
- Gao Y, Hua M, Lv J, Ma Y, Liu Y, Ren M, Tian Y, Li X, Zhang H. Reproducibility of radiomic features of pulmonary nodules between low-dose CT and conventional-dose CT. Quant Imaging Med Surg 2022;12:2368-77. [Crossref] [PubMed]
- Yoon JH, Lee JM, Lee DH, Joo I, Jeon JH, Ahn SJ, Kim ST, Cho EJ, Lee JH, Yu SJ, Kim YJ, Yoon JH. A Comparison of Biannual Two-Phase Low-Dose Liver CT and US for HCC Surveillance in a Group at High Risk of HCC Development. Liver Cancer 2020;9:503-17. [Crossref] [PubMed]
- Ganeshan B, Miles KA, Babikir S, Shortman R, Afaq A, Ardeshna KM, Groves AM, Kayani I. CT-based texture analysis potentially provides prognostic information complementary to interim fdg-pet for patients with hodgkin's and aggressive non-hodgkin's lymphomas. Eur Radiol 2017;27:1012-20. [Crossref] [PubMed]
- Ganeshan B, Skogen K, Pressney I, Coutroubis D, Miles K. Tumour heterogeneity in oesophageal cancer assessed by CT texture analysis: preliminary evidence of an association with tumour metabolism, stage, and survival. Clin Radiol 2012;67:157-64. [Crossref] [PubMed]
- Woolson RF, Bean JA, Rojas PB. Sample size for case-control studies using Cochran's statistic. Biometrics 1986;42:927-32. [Crossref] [PubMed]
- Chalkidou A, O'Doherty MJ, Marsden PK. False Discovery Rates in PET and CT Studies with Texture Features: A Systematic Review. PLoS One 2015;10:e0124165. [Crossref] [PubMed]