Deep learning-based fully automated differential diagnosis of eyelid basal cell and sebaceous carcinoma using whole slide images
Introduction
Eyelid malignancies can cause blindness, disfigurement, metastasis and even death; they reduce patients’ quality of life and can negatively affect mental health (1-3). Among eyelid malignancies, eyelid basal cell carcinoma (BCC) and sebaceous carcinoma (SC) are the two most prevalent, accounting for 41.82% and 41.55% of all eyelid malignancies in China, respectively (4). For eyelid BCC, metastasis and mortality are extremely rare, at 0.0028% and 0.5%, respectively (5,6). In contrast, for eyelid SC, metastasis is much higher, with lymph node metastasis and distant metastasis rates of 21% and 6%, respectively (7,8), leading to a disease-specific mortality of 7.5% (9). It was demonstrated that periocular SCs have a higher mortality than nonperiocular SCs (10). Furthermore, two groups have investigated the intraepithelial growth pattern of eyelid SC and established that patients with pagetoid intraepithelial neoplasia encountered higher risk for metastasis and tumour-related mortality (11,12). For patients with eyelid BCC and SC, mainstream management involves surgery, including Mohs micrographic surgery, frozen-section control marginal surgery, and wide local excision. For patients with eyelid SC, adjuvant radiotherapy should be considered in cases with extraocular extension, perineural invasion, or positive nodal basins. It was suggested that electrochemotherapy and cryosurgery can serve as therapeutic methods for some eyelid BCCs (13,14). After surgery, patients with eyelid BCC are usually followed up every year for at least 5 years. In contrast, more frequent follow-ups are recommended for eyelid SC patients, who are routinely followed up at one, three, and 6 months after surgery and every 6 months thereafter, for at least 5 years (15,16). Hence, accurate differentiation of eyelid BCC and SC is important for the choice of adjuvant therapies and follow-up regimens.
Eyelid BCC and SC usually present as a solitary substantial nodule on the eyelid, with a typical loss of cilia. Both diseases, and especially advanced lesions, cause ulceration. The appearance of the lesions sometimes provides insufficient clues for the differential diagnosis of eyelid BCC and SC. Therefore, the use of haematoxylin and eosin (H&E)-stained sections combined with immunohistochemistry (IHC) staining remains the gold standard to confirm diagnosis. However, because both BCC and SC are derived from the pilosebaceous unit, they sometimes resemble one other, especially when there is poor tissue differentiation. Further, when eyelid BCC foci present sebaceous differentiation, differentiating between eyelid SC and BCC becomes difficult (17). Although the consensus is that senior pathologists can differentiate these two tumours based on H&E sections alone, real-world statistics are rather disappointing. Doxnas et al. reported that 27.5% (11/40) of eyelid SCs were mistakenly diagnosed as BCCs (18). In another study from South Carolina in America, only 277 (42.8%) out of 1,333 SCs were diagnosed correctly initially (19). When differential diagnosis using H&E-stained sections is difficult, additional pathological tests (such as those based on fat and immunohistochemistry staining) should be conducted (8,20). However, the fat staining test requires fresh unprocessed tissue, which is sometimes unavailable in clinical practice (21). Further, although IHC-based panel identification of androgen receptor, epithelial membrane antigen, berEP4, and adipophilin can suggest the diagnosis of eyelid SC, other eyelid carcinomas can also express these markers, increasing the false positive rate. These adjuvant staining tests require specific techniques and reagents that are expensive and time-consuming. There is therefore a pressing clinical need for aided diagnostic methods, based on H&E-stained sections, to improve pathologists’ diagnostic accuracy in terms of differentiating between eyelid SC and BCC.
The whole slide scanning technique enables H&E-stained sections to be digitized as whole slide images (WSIs), making it possible to develop computer-aided diagnostic methods. Due to the rapid development and wide application of deep learning (DL) in medical image field (22,23), there have been remarkable breakthroughs in computer-aided diagnostic methods based on WSI (24-27). Jain et al. developed an Inception v3-based classification method to predict the tumour mutational burden using WSIs at different resolutions (×5, ×10, and ×20 magnification) (28). Iizuka et al. combined the Inception v3 with the long short-term memory (LSTM) network, to classify WSI into adenocarcinoma, adenoma, and non-neoplastic tumours (29). Wei et al. presented a ResNet-based classification method to identify regions of neoplastic cells and aggregated those results to infer predominant and minor histologic patterns for any WSI (30). More recently, Wang et al. proposed a visual geometry group network (VGG)-based classification method to identify eyelid malignant melanoma using WSIs (31). To our knowledge, this is the only study on the DL-based computer-aided diagnosis of eyelid tumours using WSIs, but it merely classifies benign and malignant types. Eyelid BCC and SC account for most of the malignant eyelid carcinomas, the treatment and prognosis of which vary widely, and a more refined classification solely based on H&E-stained slides is desired in clinical scenarios.
To meet the clinical need for a refined classification method based solely on H&E-stained sections, we propose a DL-based differential diagnostic method to classify eyelid BCC and SC using WSIs in two stages, namely patch prediction and WSI differentiation. In patch prediction stage, the method generates a DL model based on DenseNet-161 architecture, and in WSI differentiation stage, it constructs an integration module based on patch voting strategy. Our proposed method implements a fully automated differential diagnosis procedure of eyelid BCC and SC and generate a localization map for identifying important tumour region in WSIs. This might greatly improve differentiation accuracy and efficiency, and also reduce the workload of pathologists. We present the following article in accordance with the TRIPOD reporting checklist (available at https://qims.amegroups.com/article/view/10.21037/qims-22-98/rc).
Methods
This single-centre retrospective cohort study was conducted in compliance with the Declaration of Helsinki principles (as revised in 2013) and was approved by the ethics committee of Shanghai Ninth People’s Hospital, Shanghai Jiao Tong University School of Medicine (No. SH9H-2019-T185-2). Informed consent was waived due to the retrospective nature of the medical record review.
Image acquisition and processing
We included patients with eyelid SC and BCC who were diagnosed and treated at the Shanghai Ninth People’s Hospital from 2017 to 2019. Diagnosis was established by two senior pathologists based on H&E sections and IHC sections, following the eighth edition of the American Joint Committee on Cancer staging system for eyelid carcinoma. We used 116 H&E-stained sections from 116 eyelid BCC patients, and 180 H&E-stained sections from 129 eyelid SC patients. We digitized the H&E-stained sections as WSIs, using the Aperio ScanScope XT system (Leica Biosystems, Wetzlar, Germany) at ×40 magnification, and stored the images in SVS format. To delineate the tumour regions in each WSI, we used medical image processing software, CaseViewer v. 2.0 (https://www.3dhistech.com/). An experienced pathologist having eight years of experience in dermatological pathology first outlined the tumour regions, and then these outlines were confirmed by another experienced pathologist, who has 15 years of experience in dermatological pathology. When the interpretation differed, a final consensus was reached by group discussion.
To develop and assess the DL-based differential diagnostic method, we randomly divided the WSIs into a training set and a testing set (Table 1). By tiling each WSI on a grid at 40× magnification, 224×224 pixels’ patches were generated from tumour regions using the OpenSlide library (32). All these patches shared the same type (BCC or SC) as the WSI from which they were extracted, and those patches comprising <60% tissue were discarded (33). The colours of these remaining patches were normalized via a colour correction method named structure-preserving colour normalization (34).
Table 1
Eyelid carcinoma type | Training set | Testing set | Total |
---|---|---|---|
BCC | 93 | 23 | 116 |
SC | 144 | 36 | 180 |
Total | 237 | 59 | 296 |
WSI, whole slide image; BCC, basal cell carcinoma; SC, sebaceous carcinoma.
Development of the DL-based differential diagnostic method
We developed the diagnostic method according to the protocol shown in Figure 1. The method can be divided into patch prediction and WSI differentiation stages. In patch prediction stage, a DL model was built based on DenseNet-161, using a patch as input, and generating the patch-level probability for each carcinoma type. In WSI differentiation stage, an integration module was constructed using the average-probability strategy.
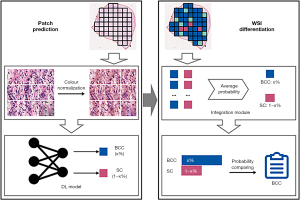
DL model for patch prediction
The DL model for patch prediction was built based on DenseNet-161 architecture (35), in which all neural network layers were directly connected with each other in a feed-forward fashion: for each layer, feature-maps of all preceding layers were used as inputs, and their own feature-maps were used as inputs into all subsequent layers. Based on this architecture, the network was fully trained to classify eyelid BCC and SC at the patch level.
Integration module for WSI differentiation
We used the DL model to predict the probability of each carcinoma type for each patch in the tumour region. To differentiate between carcinoma types, we constructed an integration module that uses the average-probability aggregation strategy (36) to average the predicted probabilities for each patch and carcinoma type and assigns the type with the larger probability. We used this approach to obtain the carcinoma type for each WSI.
Implementation details
We implemented the DenseNet-161 architecture using the PyTorch library (37). To achieve rapid convergence, we used a pre-trained DenseNet-161 [initialized on an ImageNet dataset (https://www.image-net.org)] and fine-tuned it on our data via back propagation, using an NVIDIA GTX 2080Ti graphics processing unit (38). We used cross entropy as the loss function to measure the difference between the predicted probabilities and the true class probabilities. We used Adam (39) optimization (basic learning rate of 1×10−5, batch size 16, and using 400 epochs) to train the weights.
Diagnostic interpretation and visualization
To increase the interpretability of our proposed method’s diagnostic predictions (40), we generated a localization map for identifying the regions, which contributed most significantly to the model’s predictions. First, we greyscaled the WSI, and then used blue and red to represent the predicted BCC and SC, respectively, with darker colour indicating higher confidence. Finally, we overlayed these coloured patches onto corresponding patch from the tumour region in each greyscaled WSI.
Observer study
We compared the method’s performance with that of three pathologists: two junior pathologists who recently completed a three-year standardization training program for residents in pathology, and a senior pathologist who had more than 10 years’ experience in dermatological pathology. The three pathologists were blinded to the diagnostic results and clinical data when they evaluated carcinoma type of the WSIs in the testing set. In order to verify the clinical utility of the proposed method, we then provided the pathologists with the diagnostic results (a predicted carcinoma-type probability and a localization map) obtained via the proposed method; the pathologists then re-evaluated the carcinoma type of those WSIs.
Statistical analysis
We conducted the statistical analyses using R v. 3.5.1 (https://www.r-project.org/). To evaluate carcinoma classification performance; we compared the differential diagnosis accuracy and F1-scores of the method and the pathologists. F1BCC and F1SC indicate, respectively, the F1-scores for BBC and SC identification. We applied the proportion test to assess differences in classification performance. We considered P<0.05 significant.
Results
Differential classification performance
We evaluated the method using the testing set; the corresponding confusion matrix (Table 2) reveals that only one BCC WSI was identified as SC, and no SC WSI was identified as BCC. In contrast, the junior and senior pathologists all made misdiagnoses. In particular, one of the junior pathologists incorrectly identified half of the SC WSIs. The method’s classification performance significantly differed (P<0.05) from that of the pathologists (Table 3): the method achieved substantially higher classification accuracy (0.983), compared to 0.644 and 0.729 for the two junior pathologists and 0.831 for the senior pathologist. The method achieved a higher F1-score than the pathologists for both BCC (0.978 vs. 0.656 and 0.637 for the two junior pathologists and 0.792 for the senior pathologist) and SC (0.973 vs. 0.632 and 0.784 for the two junior pathologists and 0.833 for the senior pathologist).
Table 2
Eyelid carcinoma type | DL-based fully automated differential diagnostic method | Pathologist: first junior/second junior/senior | |||
---|---|---|---|---|---|
BCC | SC | BCC | SC | ||
BCC | 22 | 1 | 20/14/19 | 3/9/4 | |
SC | 0 | 36 | 18/7/6 | 18/29/30 |
DL, deep learning; BCC, basal cell carcinoma; SC, sebaceous carcinoma.
Table 3
Diagnostic approach | BCC and SC classification accuracy | P valuea | F1BCCb | F1SC |
---|---|---|---|---|
DL-based fully automated differential diagnostic method | 0.983 | – | 0.978 | 0.973 |
First junior pathologist | 0.644 | <0.05 | 0.656 | 0.632 |
Second junior pathologist | 0.729 | <0.05 | 0.637 | 0.784 |
Senior pathologist | 0.831 | <0.05 | 0.792 | 0.833 |
a, the P values are for the comparisons between the performance of each pathologist and the automated method and are based on the proportion test; b, F1BCC and F1SC: F1-scores for the identification of BCC and SC, respectively. DL, deep learning; BCC, basal cell carcinoma; SC, sebaceous carcinoma.
Visualization of the DL model
We visualized the indicative regions for the model’s carcinoma type predictions (Figure 2A,2B: BCC; Figure 2C,2D: SC). Figure 2A is the BCC WSI (a1–3: higher magnification view of A). Figure 2B visualizes the indicative regions in A (b1–3: higher magnification view of B, corresponding to a1–3). Figure 2C is the WSI (c1–4: higher magnification view of C). Figure 2D visualizes the indicative regions in C (d1–4: higher magnification view of D, corresponding to c1–4).
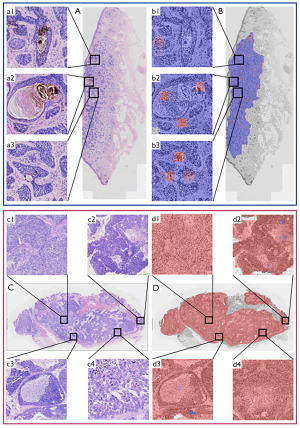
Clinical utility of DL-based differential diagnostic assistance
Auxiliary use of the method significantly improved the accuracy of pathologists, with an improvement of 28.8% and 15.2% for the two junior pathologists and 11.8% for the senior pathologist (P<0.05; Table 4). F1BCC was improved by 26.1% and 22.0% for the junior pathologists and 14.5% for the senior pathologist; F1SC was improved by 31.1% and 11.4% for the junior pathologists and 12.4% for the senior pathologist.
Table 4
Pathologists | BCC and SC classification accuracy (+difference) | P valuea | F1BCCb (+difference) | F1SC (+difference) |
---|---|---|---|---|
First junior | 0.932 (+28.8%) | <0.05 | 0.917 (+26.1%) | 0.943 (+31.1%) |
Second junior | 0.881 (+15.2%) | <0.05 | 0.857 (+22.0%) | 0.898 (+11.4%) |
Senior | 0.949 (+11.8%) | <0.05 | 0.937 (+14.5%) | 0.957 (+12.4%) |
a, the P values for each pathologist are for the comparisons of their performance with and without the help of the automated method and are based on the proportion test; b, F1BCC and F1SC: F1-scores for the identification of BCC and SC, respectively. DL, deep learning; BCC, basal cell carcinoma; SC, sebaceous carcinoma.
Discussion
Early and accurate diagnosis is of importance for treatment-related decisions (41). A method to improve differential diagnosis of eyelid BCC and SC by clinical pathologists is required. Here, we propose a novel DL-based fully automated differential diagnostic method, which can accurately classify eyelid BCC and SC using WSIs. Moreover, our proposed method generates a localization map to help pathologists focus on important regions in WSI, potentially improving their diagnostic accuracy and efficiency.
Our fully automated diagnosis method for eyelid carcinoma classification improved the accuracy and efficiency of clinical diagnosis. For the differential diagnosis of eyelid BCC and SC, the accuracy of pathologists has been reported to be 72.5% and much lower for junior pathologists (18), consistent with our findings (Table 2). Our method achieved an accuracy of 0.983, beyond that of the pathologists (Table 3). The senior pathologist achieved higher F1 scores than the junior pathologists, for both carcinoma types, verifying that clinicopathological diagnosis depends on experience (Table 3). Notably, our method outperformed the senior pathologist.
The method generates a localization map of signature regions and visualizes the prediction results in different colours to help pathologists interpret the results and recognize typical tumour signatures. We observed tumour pigmentation (Figure 2, a1), horn pearl formation (Figure 2, a2), and palisading of basophilic tumours islands (Figure 2, a3) (all marked in blue in Figure 2B). The WSIs revealed the presence of multi-nodal tumour foci (Figure 2, c1), vacuolated cytoplasm (Figure 2, c2), polygonal basaloid cells (Figure 2, c3), and comedo-type necrosis (Figure 2, c4) (these cells are all marked in red in Figure 2D). These observations demonstrated that the indicative regions were consistent with the characteristic regions of the histological patterns used for diagnosing eyelid carcinomas, indicating the potential clinical utility and reliability of our method.
The localization map of signature regions, and the carcinoma diagnosis provided by the method, significantly improved the classification accuracy of the junior and senior pathologists (Table 4), demonstrating the method’s assistive value. The increase in accuracy was greater for the junior pathologists, indicating this method can help junior pathologists to achieve the diagnostic level of senior pathologists. Our proposed method could serve as a preliminary step in the mass processing of eyelid pathology slides in clinics or primary hospitals, without the participation of specialized pathologists. Patients can then be referred to experienced ocular oncologists. Further, this digitized-image diagnostic method could facilitate collaborative consultation and communication. Notably, our proposed method is data-driven, which means that as long as we take enough WSIs as input for training a DL model, this method will implement fully automated differential diagnosis for eyelid carcinoma based on paraffin sections or frozen sections. Especially the diagnosis based on frozen sections can guide adjuvant therapies during operation, which will offer optimized solutions earlier for improving patients’ prognosis compared to postoperative.
However, this study had several limitations. First, this was a single-hospital study and all WSIs were scanned via only a same scanner. Then, the generalizability of our proposed method needs to be externally evaluated on more WSIs obtained from different WSI scanners at multiple hospitals. Second, the adjacent regions that may provide abundant information about the tumour microenvironment were not included in this study. However, they may also be useful in the differential diagnosis of eyelid carcinomas. We are developing an automated annotation procedure, which will implement automatic annotation for tumour regions and their adjacent regions, to further simplify the diagnostic process and offer more accurate diagnostic result. Finally, the current process of each WSI using our proposed method was single threaded, which resulted in hours for obtaining the diagnostic result of a WSI. In the future, we will use multi-thread processing and distributed computing to reduce the processing time for a WSI to a few minutes, which can meet the requirement of the clinic practice.
Conclusions
We propose a novel DL-based fully automated differential diagnostic method, which can accurately classify eyelid carcinomas using WSIs, and may assist pathologists in diagnosing eyelid carcinomas more accurately. If validated in prospective studies, our proposed method may serve as a useful approach for helping clinicians to optimize therapeutic plans for eyelid carcinoma patients.
Acknowledgments
Funding: This work was supported by the National Natural Science Foundation of China (Nos. 81802702, 81570884, 81871439); the Science and Technology Commission of Shanghai (Nos. 20DZ2270800, 19JC1410202); the Innovative research team of high-level local universities in Shanghai (No. SHSMU-ZDCX20210902); the Key Research and Development Program of Shandong Province (No. 2021SFGC0104); the Key Research and Development Program of Jiangsu Province (No. BE2021663); the Jiangsu Province Engineering Research Center of Diagnosis and Treatment of Children’s Malignant Tumour; the Shandong Province Natural Science Foundation (No. ZR2020QF019).
Footnote
Reporting Checklist: The authors have completed the TRIPOD reporting checklist. Available at https://qims.amegroups.com/article/view/10.21037/qims-22-98/rc
Conflicts of Interest: All authors have completed the ICMJE uniform disclosure form (available at https://qims.amegroups.com/article/view/10.21037/qims-22-98/coif). XG is a technical consultant of Jinan Guoke Medical Engineering and Technology Development Co., Ltd. The other authors have no conflicts of interest to declare.
Ethical Statement: The authors are accountable for all aspects of the work in ensuring that questions related to the accuracy or integrity of any part of the work are appropriately investigated and resolved. This study was conducted in compliance with the Declaration of Helsinki principles (as revised in 2013) and was approved by the ethics committee of Shanghai Ninth People’s Hospital, Shanghai Jiao Tong University School of Medicine (No. SH9H-2019-T185-2). Informed consent was waived due to the retrospective nature of the medical record review.
Open Access Statement: This is an Open Access article distributed in accordance with the Creative Commons Attribution-NonCommercial-NoDerivs 4.0 International License (CC BY-NC-ND 4.0), which permits the non-commercial replication and distribution of the article with the strict proviso that no changes or edits are made and the original work is properly cited (including links to both the formal publication through the relevant DOI and the license). See: https://creativecommons.org/licenses/by-nc-nd/4.0/.
References
- Huang YY, Liang WY, Tsai CC, Kao SC, Yu WK, Kau HC, Liu CJ. Comparison of the Clinical Characteristics and Outcome of Benign and Malignant Eyelid Tumors: An Analysis of 4521 Eyelid Tumors in a Tertiary Medical Center. Biomed Res Int 2015;2015:453091. [Crossref] [PubMed]
- McGrath LA, Currie ZI, Mudhar HS, Tan JHY, Salvi SM. Management of recurrent sebaceous gland carcinoma. Eye (Lond) 2020;34:1685-92. [Crossref] [PubMed]
- Freitag SK, Aakalu VK, Tao JP, Wladis EJ, Foster JA, Sobel RK, Yen MT. Sentinel Lymph Node Biopsy for Eyelid and Conjunctival Malignancy: A Report by the American Academy of Ophthalmology. Ophthalmology 2020;127:1757-65. [Crossref] [PubMed]
- Chen RJ, Xiao YQ. Clinical and pathological analysis of 2734 cases of eyelid neoplasms. Zhonghua Yan Ke Za Zhi 2008;44:143-6. [PubMed]
- von Domarus H, Stevens PJ. Metastatic basal cell carcinoma. Report of five cases and review of 170 cases in the literature. J Am Acad Dermatol 1984;10:1043-60. [Crossref] [PubMed]
- Kim DP, Kus KJB, Ruiz E. Basal Cell Carcinoma Review. Hematol Oncol Clin North Am 2019;33:13-24. [Crossref] [PubMed]
- Sa HS, Rubin ML, Xu S, Ning J, Tetzlaff M, Sagiv O, Kandl TJ, Esmaeli B. Prognostic factors for local recurrence, metastasis and survival for sebaceous carcinoma of the eyelid: observations in 100 patients. Br J Ophthalmol 2019;103:980-4. [Crossref] [PubMed]
- Shields JA, Demirci H, Marr BP, Eagle RC Jr, Shields CL. Sebaceous carcinoma of the ocular region: a review. Surv Ophthalmol 2005;50:103-22. [Crossref] [PubMed]
- Zhou C, Wu F, Chai P, Shi Y, Ye J, Shi X, Tan J, Ding Y, Luo Y, Esmaeli B, Jia R, Fan X. Mohs micrographic surgery for eyelid sebaceous carcinoma: A multicenter cohort of 360 patients. J Am Acad Dermatol 2019;80:1608-1617.e1. [Crossref] [PubMed]
- Liszewski W, Amon G, Blanchette D, Maher IA. Survival and demographic differences of periocular and nonperiocular sebaceous carcinomas. J Am Acad Dermatol 2020;83:224-7. [Crossref] [PubMed]
- Kaliki S, Morawala A, Dharap RS, Mohamed A. Pagetoid tumour spread in periocular sebaceous gland carcinoma: a comparative analysis in 130 patients. Eye (Lond) 2021;35:2864-70. [Crossref] [PubMed]
- Zhou C, Chai P, Xia W, Li J, Jia R, Fan X. Intraepithelial growth pattern for eyelid sebaceous carcinoma: a cohort of 214 patients from a single institution. Br J Ophthalmol 2021; [Crossref] [PubMed]
- Finskas O, Zaar O, Svedberg K. Cryosurgery of Periocular Moderately Aggressive Basal Cell Carcinoma. Acta Derm Venereol 2020;100:adv00336. [Crossref] [PubMed]
- Schmelter M, Scheffka D, Kaune KM, Zutt M. Electrochemotherapy as a curative therapeutic approach for basal cell carcinoma of the eyelids. J Dtsch Dermatol Ges 2022;20:690-3. [Crossref] [PubMed]
- Orr CK, Yazdanie F, Shinder R. Current review of sebaceous cell carcinoma. Curr Opin Ophthalmol 2018;29:445-50. [Crossref] [PubMed]
- Weesie F, Naus NC, Vasilic D, Hollestein LM, van den Bos RR, Wakkee M. Recurrence of periocular basal cell carcinoma and squamous cell carcinoma after Mohs micrographic surgery: a retrospective cohort study. Br J Dermatol 2019;180:1176-82. [Crossref] [PubMed]
- Asadi-Amoli F, Khoshnevis F, Haeri H, Jahanzad I, Pazira R, Shahsiah R. Comparative examination of androgen receptor reactivity for differential diagnosis of sebaceous carcinoma from squamous cell and basal cell carcinoma. Am J Clin Pathol 2010;134:22-6. [Crossref] [PubMed]
- Doxanas MT, Green WR. Sebaceous gland carcinoma. Review of 40 cases. Arch Ophthalmol 1984;102:245-9. [Crossref] [PubMed]
- Desiato VM, Byun YJ, Nguyen SA, Thiers BH, Day TA. Sebaceous Carcinoma of the Eyelid: A Systematic Review and Meta-Analysis. Dermatol Surg 2021;47:104-10. [Crossref] [PubMed]
- Sinard JH. Immunohistochemical distinction of ocular sebaceous carcinoma from basal cell and squamous cell carcinoma. Arch Ophthalmol 1999;117:776-83. [Crossref] [PubMed]
- Muthusamy K, Halbert G, Roberts F. Immunohistochemical staining for adipophilin, perilipin and TIP47. J Clin Pathol 2006;59:1166-70. [Crossref] [PubMed]
- Xiao H, Teng X, Liu C, Li T, Ren G, Yang R, Shen D, Cai J. A review of deep learning-based three-dimensional medical image registration methods. Quant Imaging Med Surg 2021;11:4895-916. [Crossref] [PubMed]
- Lin H, Xiao H, Dong L, Teo KB, Zou W, Cai J, Li T. Deep learning for automatic target volume segmentation in radiation therapy: a review. Quant Imaging Med Surg 2021;11:4847-58. [Crossref] [PubMed]
- Campanella G, Hanna MG, Geneslaw L, Miraflor A, Werneck Krauss Silva V, Busam KJ, Brogi E, Reuter VE, Klimstra DS, Fuchs TJ. Clinical-grade computational pathology using weakly supervised deep learning on whole slide images. Nat Med 2019;25:1301-9. [Crossref] [PubMed]
- Ehteshami Bejnordi B, Mullooly M, Pfeiffer RM, Fan S, Vacek PM, Weaver DL, Herschorn S, Brinton LA, van Ginneken B, Karssemeijer N, Beck AH, Gierach GL, van der Laak JAWM, Sherman ME. Using deep convolutional neural networks to identify and classify tumor-associated stroma in diagnostic breast biopsies. Mod Pathol 2018;31:1502-12. [Crossref] [PubMed]
- Coudray N, Ocampo PS, Sakellaropoulos T, Narula N, Snuderl M, Fenyö D, Moreira AL, Razavian N, Tsirigos A. Classification and mutation prediction from non-small cell lung cancer histopathology images using deep learning. Nat Med 2018;24:1559-67. [Crossref] [PubMed]
- Bao Y, Zhang J, Zhao X, Zhou H, Chen Y, Jian J, Shi T, Gao X. Deep learning-based fully automated diagnosis of melanocytic lesions by using whole slide images. J Dermatolog Treat 2022; [Crossref] [PubMed]
- Jain MS, Massoud TF. Predicting tumour mutational burden from histopathological images using multiscale deep learning. Nat Mach Intell 2020;2:356-62. [Crossref]
- Iizuka O, Kanavati F, Kato K, Rambeau M, Arihiro K, Tsuneki M. Deep Learning Models for Histopathological Classification of Gastric and Colonic Epithelial Tumours. Sci Rep 2020;10:1504. [Crossref] [PubMed]
- Wei JW, Tafe LJ, Linnik YA, Vaickus LJ, Tomita N, Hassanpour S. Pathologist-level classification of histologic patterns on resected lung adenocarcinoma slides with deep neural networks. Sci Rep 2019;9:3358. [Crossref] [PubMed]
- Wang L, Ding L, Liu Z, Sun L, Chen L, Jia R, Dai X, Cao J, Ye J. Automated identification of malignancy in whole-slide pathological images: identification of eyelid malignant melanoma in gigapixel pathological slides using deep learning. Br J Ophthalmol 2020;104:318-23. [Crossref] [PubMed]
- Goode A, Gilbert B, Harkes J, Jukic D, Satyanarayanan M. OpenSlide: A vendor-neutral software foundation for digital pathology. J Pathol Inform 2013;4:27. [Crossref] [PubMed]
- Mobadersany P, Yousefi S, Amgad M, Gutman DA, Barnholtz-Sloan JS, Velázquez Vega JE, Brat DJ, Cooper LAD. Predicting cancer outcomes from histology and genomics using convolutional networks. Proc Natl Acad Sci U S A 2018;115:E2970-9. [Crossref] [PubMed]
- Anand D, Ramakrishnan G, Sethi A. Fast GPU-Enabled Color Normalization for Digital Pathology. 2019 International Conference on Systems, Signals and Image Processing (IWSSIP); 2019: IEEE.
- Huang G, Liu Z, Van Der Maaten LQ, Weinberger K. Densely connected convolutional networks. Proceedings of the IEEE conference on computer vision and pattern recognition 2017:4700-8.
- Araújo T, Aresta G, Castro E, Rouco J, Aguiar P, Eloy C, Polónia A, Campilho A. Classification of breast cancer histology images using Convolutional Neural Networks. PLoS One 2017;12:e0177544. [Crossref] [PubMed]
- Paszke A, Gross S, Massa F, Lerer A, Bradbury J, Chanan G, et al. PyTorch: An Imperative Style, High-Performance Deep Learning Library. Advances in Neural Information Processing Systems 2019;32.
- Russakovsky O, Deng J, Su H, Krause J, Satheesh S, Ma S, Huang Z, Karpathy A, Khosla A, Bernstein M. Imagenet large scale visual recognition challenge. Int J Comput Vis 2015;115:211-52. [Crossref]
- Kingma DP, Ba J. Adam: A method for stochastic optimization. arXiv preprint arXiv:14126980 2014.
- Zeng F, Liang X, Chen Z. New Roles for Clinicians in the Age of Artificial Intelligence. BIO Integration 2020;1:113-7. [Crossref]
- Goyal M, Knackstedt T, Yan S, Hassanpour S. Artificial intelligence-based image classification methods for diagnosis of skin cancer: Challenges and opportunities. Comput Biol Med 2020;127:104065. [Crossref] [PubMed]