A software program for automated compressive vertebral fracture detection on elderly women’s lateral chest radiograph: Ofeye 1.0
Introduction
The bone composition of the spine, which is predominantly trabecular bone, is more prone to the thinning and microarchitectural changes associated with osteoporosis than regions of the hip that are richer in cortical bone. Assessment of vertebral fracture status, in addition to BMD (bone mineral density), provides relevant clinical information to aid in predicting fracture risk in postmenopausal women (1-6). Siris et al. (1) reported that at any given BMD T-score, the risk of incident vertebral, non-vertebral, and any fracture depended heavily on prevalent radiographic osteoporotic vertebral fracture (OVF) status (1). Johansson et al. (2) reported that, in older women and after adjustment for clinical risk factors and BMD, grade-1 OVFs identified on lateral spine imaging with dual-energy x-ray absorptiometry (DXA) are associated with incident major osteoporotic fractures. Though BMD has been commonly used in decision-making for the diagnosis of osteoporosis, it is associated with many inherent limitations (7,8). How to define cutpoint T-scores for differential ethnic groups remains controversial (9). In addition, osteoporotic fracture commonly occurs in subjects not in the category of BMD-defined osteoporosis, and thus would not be selected for pharmacological therapy based on BMD score alone (10-14). On the other hand, OVF is a highly prevalent clinical endpoint (15). OVF can be considered as a “gateway” to other more serious fractures, such as hip fractures.
Many guidelines suggest women with age ≥60 or ≥65 years take osteoporosis screening (16-22). Many fractures and associated complications, including secondary fractures and mortality, can be prevented by routine osteoporosis screening in older people and timely treatment initiation in at-risk individuals. A number of medications have demonstrated that they can reduce fracture risk, both vertebral and nonvertebral, including hip fractures (23-28). Nonpharmacologic approaches to manage osteoporosis, including the combination of weight-bearing and resistance training, adequate calcium and vitamin D intake, and physical activity, can positively affect bone mass. Coupled with preventing falls and limiting modifiable risk factors, such as smoking and alcohol use, these measures can help reduce a person’s risk for osteoporotic fractures (29,30). Early detection of an OVF can lead to further investigation and appropriate management that decreases the risk of future fractures (31). However, osteoporosis screening is still not commonly taken by individuals. In clinical practice, OVF remains under-recognized and undertreated. Because the OVF damage is limited to the anterior vertebral column in most cases, the fracture is usually stable and not associated with neurologic impairment. It is estimated that 3/4 of OVFs are clinically silent. Moreover, OVFs are often unrecognized on chest radiographs, especially when the radiographs were not ordered primarily for skeletal conditions. This underreporting can be due to a lack of awareness by radiologists of the clinical implications of incidental OVFs, and also some radiologists tend to focus their report only on the clinical indication of the X-ray examination, thus tend to not perform an accurate analysis of the spine while reading chest radiograph when the clinical indication is pulmonary or heart diseases (32). Even in back pain patients when the spine is the focus of investigation, OVF can also be missed (33). In our recent retrospective analysis of 105 female cases (mean: 72 years, range: 55–93 years) in a tertiary hospital in China, among the patients with OVF, the false negative reporting rate was 23.9% (17/71, counting cases), though with missed cases being all minimal or mild grades in vertebral height loss. Moreover, in 25 cases with multiple OVFs, in addition to those OVFs reported, one additional OVF was missed in 8 cases, more than one additional OVF were missed in 15 cases, and one additional vertebra with endplate and/or cortex fracture (ECF) was missed in two cases. Multiple and more severe grades of OVFs are associated with an even greater fracture risk (34-36). A precise reporting of the number of OVFs in each patient is also highly relevant in clinical practice.
Because the missing report for OVF on chest radiographs is widespread, computer aided automated detection of compressive vertebral fracture (CVF) (compressive vertebral fracture) is expected to be highly useful (since the diagnosis of OVF should be suggested by a radiologist or physician, computer reading result is termed as CVF, denoting a compressive morphological change of a vertebra). We developed a software program and validated its performance in this study. This version of the software was developed with the following considerations: (I) the software should have the sensitivity of detecting minimal grade CVFs (<20% vertebral height loss); (II) the software should have a low false positivity rate for CVFs; (III) the software should have a low false negativity rate for moderate and severe CVFs (≥25% vertebral height loss). Since we primarily wanted to develop a software program for opportunistic detection of OVF on chest radiographs not indicated for spine disorders, we took the assumption that it will be troublesome to physicians and patients if our software labels a high proportion of false positive reports. On the other hand, it will be acceptable if some minimal and mild OVFs are missed, and it is still acceptable even if a portion of moderate OVFs is missed. Therefore, the goal of our study was to emphasize CVF detection specificity, rather than sensitivity.
Methods
Radiographs materials and OVF gradings
For model training, we retrieved 3,991 spine radiograph cases and 1,979 chest radiograph cases from 15 centers (Table 1). Testing radiographs (542 chest radiograph cases and 162 spine radiograph cases) were from another four independent clinics which differ from the 15 centers which provided training data (Table 1). All cases were female subjects, and except for the training data 12 which were spine trauma cases, all the remaining cases were post-menopausal women. Image data included DICOM format, hard film scanned Portable Network Graphics (PNG) format, DICOM exported PNG format, and Picture Archiving and Communication System (PACS) downloaded reduced resolution DICOM format. The study was conducted in accordance with the Declaration of Helsinki (as revised in 2013). This retrospective study was approved by our institutional ethics committees, study subject consent was obtained for training data 1, 2, 15, and testing data 3, while patient consent was waived for the other data.
Table 1
Hospital | Total cases | Age | OVF cases | X-ray region | Image format |
---|---|---|---|---|---|
Training data 1 | 1,994 | ≥65 years | 308 | T and L spine | Scanned PNG |
Training data 2 | 1,531 | ≥69 years | 249 | T and L spine | Scanned PNG |
Training data 3 | 761 | ≥65 years | 273 | Chest | DICOM |
Training data 4 | 127 | ≥65 years | 25 | Chest | DICOM |
Training data 5 | 109 | ≥65 years | 32 | Chest | DICOM |
Training data 6 | 99 | ≥65 years | 11 | Chest | DICOM |
Training data 7 | 100 | ≥65 years | 38 | Chest | DICOM |
Training data 8 | 101 | ≥65 years | 36 | Chest | DICOM |
Training data 9 | 146 | ≥65 years | 35 | Chest | DICOM |
Training data 10 | 284 | ≥65 years | 52 | Chest | DICOM |
Training data 11 | 112 | ≥65 years | 27 | Chest | DICOM |
Training data 12 | 31 | No limitation | 31 | Spine* | DICOM |
Training data 13 | 132 | ≥55 years | 58 | Spine** | DICOM |
Training data 14 | 102 | ≥65 years | 34 | Chest | DICOM |
Training data 15 # | 303 | ≥60 years | 176 | T and L spine | Exported PNG |
Training data 16 | 38 | ≥65 years | 19 | Chest | DICOM |
Total for Training | 5,970 | 1404 | |||
Testing data 1 | 144 | ≥65 years | 49 | Chest | DICOM |
Testing data 2 | 164 | ≥65 years | 40 | Chest | DICOM |
Testing data 3 | 162 | ≥85 years | 52 | T and L spine | Scanned PNG |
Testing data 4 ## | 234 | ≥65 years | 74 | Chest | Reduced DICOM |
Total for testing | 704 | 215 |
Training data 1 and data 2 were acquired for MsOS (Hong Kong) study with 4 years apart, and with different acquisition radiography machines applied, thus equivalent to data from two sources. *, spine traumatic fracture patients (lateral and frontal view radiographs available); **, spine radiograph for back pain patients (lateral and frontal view radiographs available); #, Caucasian subjects from Italy, in DICOM exported PNG format. ##, data in PACS downloaded reduced resolution DICOM format. Only lateral view radiographs available for training data 1, 2, and 15, while all chest radiographs have both lateral and frontal views. OVF, osteoporotic vertebral fracture; scanned PNG, hard film scanned image; DICOM, Digital Imaging and Communications in Medicine; PNG, Portable Network Graphics; PACS, Picture Archiving and Communications System .
The initial readings were based on previous results for spine radiograph OVF epidemiological studies (training data 1, 2, 15, and testing data 3), and chest radiographs were evaluated initially by a trained biomedical engineering graduate (BHX). Reading and labelling were primarily based on lateral radiograph, while frontal radiograph was checked when available and necessary to assist the reading. The final reference readings were established by an experienced radiologist reader (YXJW). The OVF diagnostic criteria were based on previous publications (15,37-43). In addition to vertebral height reductions, attention was paid to alterations in the shape and configuration of a vertebra relative to adjacent vertebrae and expected normal appearances. All the known mimics of OVF were systematically excluded. While a positive ECF sign increases the diagnostic confidence for OVF, an ECF sign was not considered essential for diagnosing an OVF (42). According to the vertebral height loss, OVFs were classified as: minimal and mild grades with <20% or ≥20–25% vertebral height loss respectively, moderate grade with ≥25–40% vertebral height loss, severe grade with ≥40%–2/3 vertebral height loss, and collapsed grade with ≥2/3 vertebral height loss. Since the diagnosis of minimal grade OVF can be sometimes subjective, and these OVFs tend to overall have less immediate clinical relevance, diagnosis of minimal grade OVF was not strictly enforced, i.e., some of the minimal grade OVFs might have been missed during the human reader labelling.
Image pre-processing
Only lateral radiographs were used. Personal information contained in the DICOM format radiographs was removed, and then the images were saved as 1024×1024 PNG format images. Images larger than 1024×1024 were cropped, while images smaller than 1024×1024 were padded with black borders. The contrast and other relevant parameters of the images were adjusted to increase the image clarity.
Based on human labelling, the information of OVF coordinates in the image was obtained.
Measures such as image flipping, rotation at different angles, and random splicing were used to expand the training dataset, for the purpose of improving the robustness and stability of the resulting CVF detection model.
Model training and optimization
Images were normalized to train the CVF detection base model. The detection model structure was composed of three parts, i.e., backbone, neck, and prediction (44), and composed of convolution layers that included convolution kernels of different sizes, pooling layers, up-sampling layers, feature merging layers, and residual modules (45), totaling 213 layers.
The main training parameters were the batch size of 8 (every batch included one negative image), the epoch of 1,000, and the initial learning rate was 0.01. As the number of model iterations increased, the cosine annealing function was used to gradually reduce the learning rate. The dropblock function was used to alleviate the problem of model overfitting. The loss function was (Distance intersection over union) DIOU_Loss, and the (Stochastic Gradient Descent) SGD gradient descent algorithm was used for parameter optimization. Model training was performed using Ubuntu 18.04.4 LTS, python (v3.6.12), pytorch (v1.7.1) machine learning libraries, Nvidia A100(40GB memory) Tensor Core Graphics Processing Unit, and Xenon E5-2698 v4 2.2GHz, 20 Cores Central Processing Unit.
When a CVF is detected, a probability is returned. The model labels a vertebra as with CVF when the probability is ≥0.6. This parameter can be adjusted depending on whether specificity or sensitivity is emphasized.
When the model loss function could not be further decreased, the optimal training model, which we called ‘base-model 1.0’, was obtained.
Base-model 1.0 testing for CVF detection
For testing, in total radiographs of 704 cases were retrieved. These testing radiographs included a mixture of standard DICOM images, film scanned PNG images, and resolution reduced DICOM images downloaded from a PACS. Thus, the testing radiographs were not all of idealized image quality. Lateral radiographs were read by the base-model 1.0, and the reference reading was established by the radiologist reader (YXJW).
After the base-model 1.0 reading, the cases with false positivity and false negativity were further inspected for potential causes.
User interface testing
A user-friendly interface was developed (Figures S1-S4), and the synthesized software was termed Ofeye 1.0. This software allows ‘batch processing’, for example, 100 radiographs can be processed in a single operation. This software can be integrated into hospital PACS, or installed in a standalone personal computer. The clinical usage of a prototype version of the software has been tested externally since Nov 18, 2021, in the Department of Radiology, the First Affiliated Hospital of Zhejiang Chinese Medical University, Hangzhou, China. Users there provided feedback for improving the user-friendliness of this software.
Results
The primary Ofeye 1.0 reading output is demonstrated in Figure 1, the left image window shows the original radiograph; while the right image window shows the radiograph with CVF labelling (if there is/are). If there is no CVF detected, then the left image window and right image window will show the same image. For each suspected CVF detected, a probability is provided. The DICOM view function of this software allows other basic functions including zoom-in, zoom-out, adjusting contrast and window levels, and distance and angle degree measurements.
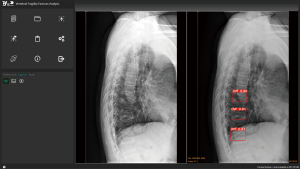
CVF detection performance of the base-model 1.0 is shown in Table 2. Testing data 1 and data 2, which were in original DICOM format, showed slightly better performance than testing data 3 (in film scanned PNG format) and testing data 4 (in PACS downloaded reduced resolution DICOM format).
Table 2
Data | Total | TP case | FP case | TN case | FN case | Sensitivity | Specificity | Accuracy |
---|---|---|---|---|---|---|---|---|
Data 1 | 144 | 43 | 1 | 94 | 6 | 0.878 | 0.989 | 0.951 |
Data 2 | 164 | 34 | 1 | 123 | 6 | 0.85 | 0.992 | 0.957 |
Data 3 | 162 | 46 | 6 | 104 | 6 | 0.885 | 0.945 | 0.926 |
Data 4 | 234 | 62 | 5 | 155 | 12 | 0.838 | 0.969 | 0.927 |
Total | 704 | 185 | 13 | 476 | 30 | 0.860 | 0.973 | 0.938 |
TP, true positive; FP, false positive; TN, true negative; FN, false negative.
In total, 33 OVFs in 30 cases had a false negative reading, which constituted a false negative rate of 14.0% (30/215) for counting all OVF cases (including those with minimal or mild grade OVFs). Among these cases with false negative reading, 15 OVFs in 15 cases were mild or minimal grades, while 18 OVFs in 15 cases had OVFs of ≥ moderate grades missed (Table 3), which constituted a false negative rate of 7.0% (15/215) if only counting cases with ≥ moderate grade OVFs. In other words, for cases with ≥ moderate grades OVF, 93% were detected. False positive reading was recorded in 13 vertebrae in 13 cases (one vertebra in each case, Table 4), which constituted a false positivity rate of 2.7% (13/489). These vertebrae with false positivity labeling had a mean probability of 0.72 (ranging from 0.61 to 0.88). They could mostly be easily evaluated by a radiologist reader as being of no significance or a false positive reading (Figure 2).
Table 3
Case number | OVF number | Grade | Explanation for the false negativity |
---|---|---|---|
4 | 4 | Moderate | OVF close to a border of the image |
1 | 1 | Moderate | OVF overlay with diagram line |
1 | 2 | Moderate | OVF together with osteoarthritis |
1 | 3 | Moderate | OVF together with osteoarthritis and disappearance of disc space |
1 | 1 | Moderate | Oblique filming to the X-ray beam |
3 | 3 | Moderate | n/a |
2 | 2 | Severe | n/a |
1 | 1 | Collapsed | Vertebra totally collapsed |
1 | 1 | Collapsed | Vertebra collapsed + rotation |
OVFs, osteoporotic vertebral fractures; n/a, not available.
Table 4
Case number | OVF number | Explanation for the false positivity |
---|---|---|
3 | 3 | Labeled vertebra above T4 |
3 | 3 | Labeled vertebra had rotation to X-ray beam |
5 | 5 | Labeled vertebra looks like minimal CVF |
1 | 1 | Labeled vertebra looks like minimal CVF + diagram line overlaying |
1 | 1 | n/a |
OVF, osteoporotic vertebral fracture; CVF, compressive vertebral fracture; n/a, not available.
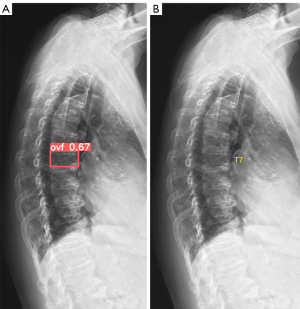
Discussion
In recent a few years, a number of authors reported AI (artificial Intelligence) enabled analysis and detection of VF (vertebral fracture) of spine medical images which included spine radiograph (46-53), DXA (54-56), thoracic and/or abdominal CT (57-62), and spine MR images (63-66). Kim et al. (46) presented a structured hierarchical segmentation method that combines the advantages of two deep-learning methods of pose-driven learning and M-net which allows automated detection and segmentation of lumbar vertebrae from radiograph for CVF evaluation. Kim et al. (47) described an approach of AI enabled automated vertebral segmentation of lateral thoracic and lumbar spine radiograph which is expected to be helpful for the measurement of vertebral compression ratio. Seo et al. (48) described a vertebral body segmentation model and a vertebral compression measurement approach on lateral lumbar spine radiographs. Chou et al. (49) and Li et al. (50) reported AI enabled detection of VFs on thoracic and lumbar radiographs, with good accuracy achieved especially for lumbar Genant Grades 2 and 3 VFs. Murata et al. (51) reported AI enabled detection of VFs on plain spinal radiograph. With MRI as the reference standard, Chen et al. (52) reported identifying fresh CVFs from spine radiograph. Chen et al. (53) reported the application of a deep learning algorithm to detect and visualize VFs on plain frontal abdominal radiographs. Derkatch et al. (54) described a model to identify VFs on DXA images. Mehta and Sebro (55) described an application of a support vector machine learning algorithm using posterior-anterior DXA images to identify lumbar spine (L1-L4) VFs without additional lateral DXA imaging. Monchka et al. (56) described an AI model for automated identification of CVF using dual-energy and or single-energy lateral DXA images. Tomita et al. (57) described an AI enabled method to detect incidental CVFs in chest, abdomen, and pelvis CT examinations. Burns et al. (58) described an AI enabled method which detects, localizes, and classifies CVFs and measures BMD of thoracic and lumbar vertebral bodies on CT images. Kolanu et al. (59) described a computer-aided diagnosis model (Zebra Medical Vision) for Genant grades 2 or 3 OVFs in a single center 2357 abdominal and thoracic CT scans. Roux et al. (60) reported automated detection of VF in 150,000 routine lumbar or abdominal CT scans from 35 hospitals in Paris, France. Rueckel et al. (61) described several pathology-specific AI algorithms enabled detection of relevant initially missed secondary thoracic findings in emergency whole-body CT scans, including the detection of cardiomegaly, coronary artery plaques, lung lesions, aortic aneurysms, and VFs. Yabu et al. (63) described AI enabled detection of fresh VF on MR images. Del Lama et al. (64) described AI enabled detection of CVFs on spine MRI images. Yeh et al. (65) described an AI enabled model for the diagnosis of VFs on spine MR images. Yoda et al. (66) described an AI enabled approach for automated differentiation between OVF and CVF due to spinal metastasis on MR images. Overall, most of the reports were single center proof-of-concept studies, with unknown generalisability. Roux et al. (60) reported automated detection of VF in 150,000 routine lumbar or abdominal CT scans from 35 hospitals. However, a shorting coming of the study of Roux et al. appears to be that they did not apply different thresholds (or different criteria) for male and female patients. They reported that, of the patients with VFs, 43.7% were male; while this may represent an over-estimation of VFs in male patients (67).
Compared with literature reports, our base-model 1.0 has the following features: (I) the goal of our software is to detect CVF on lateral chest radiographs (instead of spine radiograph or CT/MRI data) which were initially not indicated for spine disorders. It is less likely that important OVFs are missed by a human reader on spine radiographs taken for spine disorders; (II) our software allows the detection of CVF with <20% height loss, which may be clinically relevant in selected cases (68-71); (III) our software used a large sample size from multiple sources (n=16) for training, the performance of our software was tested with data from multiple external centers (n=4); (IV) despite the testing image data were not all in idealised format, our software offers superior diagnostic performance compared with many literature reports. This base-model 1.0 achieved our goal that (I) it has an overall low false positivity rate (<3% according to our own testing); and (II) it has a low false negativity rate of 7.0% for moderate and severe CVFs (i.e., those of ≥25% vertebral height loss).
There are still many limitations of this study and thus many still limitations for our software Ofeye 1.0. Ofeye 1.0 does not grade the severity of the VF, instead it offers a ‘yes/no’ selection and provides a CVF probability estimation. It labels a vertebra as with CVF when the probability is ≥0.6. Overall, a milder vertebral height loss is associated with a smaller value of the probability and vice versa. In the future, we want to further add the function for CVF grading. Ofeye 1.0 was developed with the goal to emphasize CVF detection specificity at the cost of sensitivity, thus Ofeye 1.0 may not be suitable for traumatic VF assessment. We plan to develop another version of Ofeye which will emphasize CVF detection sensitivity, so that it can be applied to spine traumatic patients. Ofeye 1.0 was trained with radiographs of female subjects, how it can be applied to male subjects requires additional adjustment of the parameters (67), and also additional validations. Ofeye 1.0 was developed for lateral chest radiograph, how it can be reliably applied to the lower lumbar spine also requires additional validations. It should also be noted that, the initial OVF human labelling and the subsequent CVF testing were primarily based on the reference reading provided by one radiologist (YXJW) and his perception of radiographic diagnostics of OVF. However, how to best diagnose and classify minimal and mild grade OVFs remains controversial (72), though there is usually no difficulty for human reader diagnosis of moderate and severe grade OVFs. Thus, it is possible that, if Ofeye 1.0 is tested by a third independent party, slightly different CVF detection performance may be obtained as there is no golden standard for labelling minimal grade OVFs. Finally, it should be noted that Ofeye 1.0 suggests the probability of a vertebra having CVF, it does not offer a firm diagnosis of OVF. The diagnosis of OVF should be made by a radiologist or a physician, by further excluding mimics and other causes (such as artefacts due to poor image quality or scoliosis). While we argue for the importance of recognizing OVFs with <20% vertebral height loss, the clinical management of such OVF cases would depend on the clinical data such as BMD or other fragility fracture history. Though minimal grade OVFs may not have immediate further fragility fracture consequences, they are a biomarker of compromised bone quality. In our MsOS (Hong Kong) year-14 follow-up, out of 150 female participants, five women were identified as having baseline minimal OVF and among them three had osteopenia and two had osteoporosis. There was a trend that these minimal OVF subjects had incident OVF risk similar to that of the subjects with baseline apparent OVF (i.e., ≥20% height loss), higher than female subjects without baseline OVF (6). The real-world importance of minimal/mild OVFs may depend on patients individually, and a wait-and-see strategy with follow-up imaging may be sufficient for many non-traumatic cases. Depending on practical scenarios, for CVFs with minimal/mild extent of vertebral height loss and a small value of probability, the diagnostician may also choose to ignore them for practical reasons even when the CVFs look like being osteoporotic.
In conclusion, we developed a user-friendly software program, Ofeye 1.0, for CVF detection on elderly women’s lateral chest radiographs. This software has an overall low false positivity rate (2.7%), and also for moderate and severe CVFs a low false negativity rate (7.0%). Ofeye 1.0 has batch processing function and can be integrated into hospital PACS. We expect the integration of such a software program into radiological practice will improve osteoporosis management for elderly patients.
Acknowledgments
Funding: This study was supported by Hong Kong ITSP project (No. ITS/334/18).
Footnote
Conflicts of Interest: All authors have completed the ICMJE uniform disclosure form (available at https://qims.amegroups.com/article/view/10.21037/qims-22-433/coif). YXJW serves as the Editor-in-Chief of Quantitative Imaging in Medicine and Surgery. YXJW is the founder of Yingran Medicals Ltd, which develops medical image-based diagnostics software, including Ofeye. BHX and MSYZ contributed to the development of Ofeye 1.0. The other authors have no conflicts of interest to declare.
Ethical Statement: The authors are accountable for all aspects of the work in ensuring that questions related to the accuracy or integrity of any part of the work are appropriately investigated and resolved. The study was conducted in accordance with the Declaration of Helsinki (as revised in 2013). This retrospective study was approved by our institutional ethics committees, study subject consent was obtained for training data 1, 2, 15, and testing data 3, while patient consent was waived for the other data.
Open Access Statement: This is an Open Access article distributed in accordance with the Creative Commons Attribution-NonCommercial-NoDerivs 4.0 International License (CC BY-NC-ND 4.0), which permits the non-commercial replication and distribution of the article with the strict proviso that no changes or edits are made and the original work is properly cited (including links to both the formal publication through the relevant DOI and the license). See: https://creativecommons.org/licenses/by-nc-nd/4.0/.
References
- Siris ES, Genant HK, Laster AJ, Chen P, Misurski DA, Krege JH. Enhanced prediction of fracture risk combining vertebral fracture status and BMD. Osteoporos Int 2007;18:761-70. [Crossref] [PubMed]
- Johansson L, Sundh D, Magnusson P, Rukmangatharajan K, Mellström D, Nilsson AG, Lorentzon M. Grade 1 Vertebral Fractures Identified by Densitometric Lateral Spine Imaging Predict Incident Major Osteoporotic Fracture Independently of Clinical Risk Factors and Bone Mineral Density in Older Women. J Bone Miner Res 2020;35:1942-51. [Crossref] [PubMed]
- Lentle BC, Berger C, Probyn L, Brown JP, Langsetmo L, Fine B, Lian K, Shergill AK, Trollip J, Jackson S, Leslie WD, Prior JC, Kaiser SM, Hanley DA, Adachi JD, Towheed T, Davison KS, Cheung AM, Goltzman D. CaMos Research Group. Comparative Analysis of the Radiology of Osteoporotic Vertebral Fractures in Women and Men: Cross-Sectional and Longitudinal Observations from the Canadian Multicentre Osteoporosis Study (CaMos). J Bone Miner Res 2018;33:569-79. [Crossref] [PubMed]
- Kadowaki E, Tamaki J, Iki M, Sato Y, Chiba Y, Kajita E, Kagamimori S, Kagawa Y, Yoneshima H. Prevalent vertebral deformity independently increases incident vertebral fracture risk in middle-aged and elderly Japanese women: the Japanese Population-based Osteoporosis (JPOS) Cohort Study. Osteoporos Int 2010;21:1513-22. [Crossref] [PubMed]
- Black DM, Arden NK, Palermo L, Pearson J, Cummings SR. Prevalent vertebral deformities predict hip fractures and new vertebral deformities but not wrist fractures. Study of Osteoporotic Fractures Research Group. J Bone Miner Res 1999;14:821-8. [Crossref] [PubMed]
- Wáng YXJ, Che-Nordin N, Leung JCS, Man Yu BW, Griffith JF, Kwok TCY. Elderly men have much lower vertebral fracture risk than elderly women even at advanced age: the MrOS and MsOS (Hong Kong) year 14 follow-up radiology results. Arch Osteoporos 2020;15:176. [Crossref] [PubMed]
- Kanis JA. Assessment of fracture risk and its application to screening for postmenopausal osteoporosis: synopsis of a WHO report. WHO Study Group. Osteoporos Int 1994;4:368-81. [Crossref] [PubMed]
- Wang YX. Medical imaging in pharmaceutical clinical trials: what radiologists should know. Clin Radiol 2005;60:1051-7. [Crossref] [PubMed]
- Wáng YXJ, Xiao BH. Estimations of bone mineral density defined osteoporosis prevalence and cutpoint T-scores for defining osteoporosis among Chinese population: A framework based on relative fragility fracture risks. Quant Imaging Med Surg 2022; [Crossref]
- Siris ES, Chen YT, Abbott TA, Barrett-Connor E, Miller PD, Wehren LE, Berger ML. Bone mineral density thresholds for pharmacological intervention to prevent fractures. Arch Intern Med 2004;164:1108-12. [Crossref] [PubMed]
- Cheng X, Griffith JF, Chan WP. Top-Ten Pitfalls When Imaging Osteoporosis. Semin Musculoskelet Radiol 2019;23:453-64. [Crossref] [PubMed]
- Nuti R, Brandi ML, Isaia G, Tarantino U, Silvestri S, Adami S. New perspectives on the definition and the management of severe osteoporosis: the patient with two or more fragility fractures. J Endocrinol Invest 2009;32:783-8. [Crossref] [PubMed]
- Schuit SC, van der Klift M, Weel AE, de Laet CE, Burger H, Seeman E, Hofman A, Uitterlinden AG, van Leeuwen JP, Pols HA. Fracture incidence and association with bone mineral density in elderly men and women: the Rotterdam Study. Bone 2004;34:195-202. [Crossref] [PubMed]
- Wainwright SA, Marshall LM, Ensrud KE, Cauley JA, Black DM, Hillier TA, Hochberg MC, Vogt MT, Orwoll ESStudy of Osteoporotic Fractures Research Group. Hip fracture in women without osteoporosis. J Clin Endocrinol Metab 2005;90:2787-93. [Crossref] [PubMed]
- Wang YX. An update of our understanding of radiographic diagnostics for prevalent osteoporotic vertebral fracture in elderly women. Quant Imaging Med Surg 2022; [Crossref]
- Cheng X, Yuan H, Cheng J, Weng X, Xu H, Gao J, Huang M, Wáng YXJ, Wu Y, Xu W, Liu L, Liu H, Huang C, Jin Z, Tian W. Bone and Joint Group of Chinese Society of Radiology, Chinese Medical Association (CMA), Musculoskeletal Radiology Society of Chinese Medical Doctors Association, Osteoporosis Group of Chinese Orthopedic Association, Bone Density Group of Chinese Society of Imaging Technology, CMA*. Chinese expert consensus on the diagnosis of osteoporosis by imaging and bone mineral density. Quant Imaging Med Surg 2020;10:2066-77. [Crossref] [PubMed]
- International Society for Clinical Densitometry. 2019 ISCD Official Positions–Adult. International Society for Clinical Densitometry (ISCD). Available online: https://iscd.org/wp-content/uploads/2021/09/2019-Official-Positions-Adult-1.pdf. Accessed on 17 May April 2022
- Sanchez-Rodriguez D, Bergmann P, Body JJ, Cavalier E, Gielen E, Goemaere S, Lapauw B, Laurent MR, Rozenberg S, Honvo G, Beaudart C, Bruyère O. The Belgian Bone Club 2020 guidelines for the management of osteoporosis in postmenopausal women. Maturitas 2020;139:69-89. [Crossref] [PubMed]
- Nuti R, Brandi ML, Checchia G, Di Munno O, Dominguez L, Falaschi P, Fiore CE, Iolascon G, Maggi S, Michieli R, Migliaccio S, Minisola S, Rossini M, Sessa G, Tarantino U, Toselli A, Isaia GC. Guidelines for the management of osteoporosis and fragility fractures. Intern Emerg Med 2019;14:85-102. [Crossref] [PubMed]
- Kwok TCY, Law SW, Leung EMF, Choy DTK, Lam PMS, Leung JCS, Wong SH, Ip TP, Cheung CL. Hip fractures are preventable: a proposal for osteoporosis screening and fall prevention in older people. Hong Kong Med J 2020;26:227-35. [Crossref] [PubMed]
- Curry SJ, Krist AH, Owens DK, Barry MJ, Caughey AB, Davidson KW, Doubeni CA, Epling JW Jr, Kemper AR, Kubik M, Landefeld CS, Mangione CM, Phipps MG, Pignone M, Silverstein M, Simon MA, Tseng CW, Wong JB. Screening for Osteoporosis to Prevent Fractures: US Preventive Services Task Force Recommendation Statement. JAMA 2018;319:2521-31. [Crossref] [PubMed]
- Brincat M, Calleja-Agius J, Erel CT, Gambacciani M, Lambrinoudaki I, Moen MH, Schenck-Gustafsson K, Tremollieres F, Vujovic S, Rees M, Rozenberg S. EMAS. EMAS position statement: Bone densitometry screening for osteoporosis. Maturitas 2011;68:98-101. [Crossref] [PubMed]
- Fink HA, MacDonald R, Forte ML, Rosebush CE, Ensrud KE, Schousboe JT, Nelson VA, Ullman K, Butler M, Olson CM, Taylor BC, Brasure M, Wilt TJ. Long-Term Drug Therapy and Drug Discontinuations and Holidays for Osteoporosis Fracture Prevention: A Systematic Review. Ann Intern Med 2019;171:37-50. [Crossref] [PubMed]
- Eastell R, Rosen CJ, Black DM, Cheung AM, Murad MH, Shoback D. Pharmacological Management of Osteoporosis in Postmenopausal Women: An Endocrine Society* Clinical Practice Guideline. J Clin Endocrinol Metab 2019;104:1595-622. [Crossref] [PubMed]
- Crandall CJ, Newberry SJ, Diamant A, Lim YW, Gellad WF, Booth MJ, Motala A, Shekelle PG. Comparative effectiveness of pharmacologic treatments to prevent fractures: an updated systematic review. Ann Intern Med 2014;161:711-23. [Crossref] [PubMed]
- Viswanathan M, Reddy S, Berkman N, Cullen K, Middleton JC, Nicholson WK, Kahwati LC. Screening to Prevent Osteoporotic Fractures: Updated Evidence Report and Systematic Review for the US Preventive Services Task Force. JAMA 2018;319:2532-51. [Crossref] [PubMed]
- Agency for Healthcare Research and Quality. Pathways to Prevention (P2P). Workshop draft systematic evidence review: long-term drug therapy and drug holidays for osteoporosis fracture prevention. Available online: https://effectivehealthcare.ahrq.gov/products/osteoporosis-fracture-prevention. Accessed on 30 April 2022
- Committee on Practice Bulletins-Gynecology, The American College of Obstetricians and Gynecologists. ACOG Practice Bulletin N. 129. Osteoporosis. Obstet Gynecol 2012;120:718-34. [Crossref] [PubMed]
- Howe TE, Shea B, Dawson LJ, Downie F, Murray A, Ross C, Harbour RT, Caldwell LM, Creed G. Exercise for preventing and treating osteoporosis in postmenopausal women. Cochrane Database Syst Rev 2011;CD000333. [Crossref] [PubMed]
- Levis S, Theodore G. Summary of AHRQ's comparative effectiveness review of treatment to prevent fractures in men and women with low bone density or osteoporosis: update of the 2007 report. J Manag Care Pharm 2012;18:S1-15; discussion S13. [Crossref] [PubMed]
- Conley RB, Adib G, Adler RA, Åkesson KE, Alexander IM, Amenta KC, et al. Secondary Fracture Prevention: Consensus Clinical Recommendations from a Multistakeholder Coalition. J Bone Miner Res 2020;35:36-52. [Crossref] [PubMed]
- Bartalena T, Rinaldi MF, Modolon C, Braccaioli L, Sverzellati N, Rossi G, Rimondi E, Busacca M, Albisinni U, Resnick D. Incidental vertebral compression fractures in imaging studies: Lessons not learned by radiologists. World J Radiol 2010;2:399-404. [Crossref] [PubMed]
- Du MM, Che-Nordin N, Ye PP, Qiu SW, Yan ZH, Wang YXJ. Underreporting characteristics of osteoporotic vertebral fracture in back pain clinic patients of a tertiary hospital in China. J Orthop Translat 2019;23:152-8. [Crossref] [PubMed]
- Weaver J, Sajjan S, Lewiecki EM, Harris ST, Marvos P. Prevalence and Cost of Subsequent Fractures Among U.S. Patients with an Incident Fracture. J Manag Care Spec Pharm 2017;23:461-71. [Crossref] [PubMed]
- Delmas PD, Genant HK, Crans GG, Stock JL, Wong M, Siris E, Adachi JD. Severity of prevalent vertebral fractures and the risk of subsequent vertebral and nonvertebral fractures: results from the MORE trial. Bone 2003;33:522-32. [Crossref] [PubMed]
- Wáng YXJ, Che-Nordin N, Deng M, Leung JCS, Kwok AWL, He LC, Griffith JF, Kwok TCY, Leung PC. Osteoporotic vertebral deformity with endplate/cortex fracture is associated with higher further vertebral fracture risk: the Ms. OS (Hong Kong) study results. Osteoporos Int 2019;30:897-905. [Crossref] [PubMed]
- Genant HK, Wu CY, van Kuijk C, Nevitt MC. Vertebral fracture assessment using a semiquantitative technique. J Bone Miner Res 1993;8:1137-48. [Crossref] [PubMed]
- Genant HK, Jergas M. Assessment of prevalent and incident vertebral fractures in osteoporosis research. Osteoporos Int 2003;14:S43-55. [Crossref] [PubMed]
- Jiang G, Eastell R, Barrington NA, Ferrar L. Comparison of methods for the visual identification of prevalent vertebral fracture in osteoporosis. Osteoporos Int 2004;15:887-96. [Crossref] [PubMed]
- Wáng YXJ, Diacinti D, Yu W, Cheng XG, Nogueira-Barbosa MH, Che-Nordin N, Guglielmi G, Ruiz Santiago F. Semi-quantitative grading and extended semi-quantitative grading for osteoporotic vertebral deformity: a radiographic image database for education and calibration. Ann Transl Med 2020;8:398. [Crossref] [PubMed]
- Wáng YXJ, Santiago FR, Deng M, Nogueira-Barbosa MH. Identifying osteoporotic vertebral endplate and cortex fractures. Quant Imaging Med Surg 2017;7:555-91. [Crossref] [PubMed]
- Du EZ, Wáng YXJ. CT detects more osteoporotic endplate depressions than radiograph: a descriptive comparison of 76 vertebrae. Osteoporos Int 2022; Epub ahead of print. [Crossref] [PubMed]
- Wáng YXJ, Deng M, He LC, Che-Nordin N, Santiago FR. Osteoporotic vertebral endplate and cortex fractures: A pictorial review. J Orthop Translat 2018;15:35-49. [Crossref] [PubMed]
- Redmon J, Divvala S, Girshick R, Farhadi A. You only look once: Unified, real-time object detection. Proceedings of the IEEE conference on computer vision and pattern recognition. 2016, pp. 779-788.
- He K, Zhang X, Ren S, Sun J. Deep Residual Learning for Image Recognition. Proceedings of the IEEE Conference on Computer Vision and Pattern Recognition (CVPR) 2016, pp. 770-778.
- Kim KC, Cho HC, Jang TJ, Choi JM, Seo JK. Automatic detection and segmentation of lumbar vertebrae from X-ray images for compression fracture evaluation. Comput Methods Programs Biomed 2021;200:105833. [Crossref] [PubMed]
- Kim DH, Jeong JG, Kim YJ, Kim KG, Jeon JY. Automated Vertebral Segmentation and Measurement of Vertebral Compression Ratio Based on Deep Learning in X-Ray Images. J Digit Imaging 2021;34:853-61. [Crossref] [PubMed]
- Seo JW, Lim SH, Jeong JG, Kim YJ, Kim KG, Jeon JY. A deep learning algorithm for automated measurement of vertebral body compression from X-ray images. Sci Rep 2021;11:13732. [Crossref] [PubMed]
- Chou PH, Jou TH, Wu HH, Yao YC, Lin HH, Chang MC, Wang ST, Lu HH, Chen HH. Ground truth generalizability affects performance of the artificial intelligence model in automated vertebral fracture detection on plain lateral radiographs of the spine. Spine J 2022;22:511-23. [Crossref] [PubMed]
- Li YC, Chen HH, Horng-Shing Lu H, Hondar Wu HT, Chang MC, Chou PH. Can a Deep-learning Model for the Automated Detection of Vertebral Fractures Approach the Performance Level of Human Subspecialists? Clin Orthop Relat Res 2021;479:1598-612. [Crossref] [PubMed]
- Murata K, Endo K, Aihara T, Suzuki H, Sawaji Y, Matsuoka Y, Nishimura H, Takamatsu T, Konishi T, Maekawa A, Yamauchi H, Kanazawa K, Endo H, Tsuji H, Inoue S, Fukushima N, Kikuchi H, Sato H, Yamamoto K. Artificial intelligence for the detection of vertebral fractures on plain spinal radiography. Sci Rep 2020;10:20031. [Crossref] [PubMed]
- Chen W, Liu X, Li K, Luo Y, Bai S, Wu J, Chen W, Dong M, Guo D. A deep-learning model for identifying fresh vertebral compression fractures on digital radiography. Eur Radiol 2022;32:1496-505. [Crossref] [PubMed]
- Chen HY, Hsu BW, Yin YK, Lin FH, Yang TH, Yang RS, Lee CK, Tseng VS. Application of deep learning algorithm to detect and visualize vertebral fractures on plain frontal radiographs. PLoS One 2021;16:e0245992. [Crossref] [PubMed]
- Derkatch S, Kirby C, Kimelman D, Jozani MJ, Davidson JM, Leslie WD. Identification of Vertebral Fractures by Convolutional Neural Networks to Predict Nonvertebral and Hip Fractures: A Registry-based Cohort Study of Dual X-ray Absorptiometry. Radiology 2019;293:405-11. [Crossref] [PubMed]
- Mehta SD, Sebro R. Computer-Aided Detection of Incidental Lumbar Spine Fractures from Routine Dual-Energy X-Ray Absorptiometry (DEXA) Studies Using a Support Vector Machine (SVM) Classifier. J Digit Imaging 2020;33:204-10. [Crossref] [PubMed]
- Monchka BA, Kimelman D, Lix LM, Leslie WD. Feasibility of a generalized convolutional neural network for automated identification of vertebral compression fractures: The Manitoba Bone Mineral Density Registry. Bone 2021;150:116017. [Crossref] [PubMed]
- Tomita N, Cheung YY, Hassanpour S. Deep neural networks for automatic detection of osteoporotic vertebral fractures on CT scans. Comput Biol Med 2018;98:8-15. [Crossref] [PubMed]
- Burns JE, Yao J, Summers RM. Vertebral Body Compression Fractures and Bone Density: Automated Detection and Classification on CT Images. Radiology 2017;284:788-97. [Crossref] [PubMed]
- Kolanu N, Silverstone EJ, Ho BH, Pham H, Hansen A, Pauley E, Quirk AR, Sweeney SC, Center JR, Pocock NA. Clinical Utility of Computer-Aided Diagnosis of Vertebral Fractures From Computed Tomography Images. J Bone Miner Res 2020;35:2307-12. [Crossref] [PubMed]
- Roux C, Rozes A, Reizine D, Hajage D, Daniel C, Maire A, Bréant S, Taright N, Gordon R, Fechtenbaum J, Kolta S, Feydy A, Briot K, Tubach F. Fully automated opportunistic screening of vertebral fractures and osteoporosis on more than 150,000 routine computed tomography scans. Rheumatology (Oxford) 2021; [Epub ahead of print]. [Crossref] [PubMed]
- Rueckel J, Sperl JI, Kaestle S, Hoppe BF, Fink N, Rudolph J, Schwarze V, Geyer T, Strobl FF, Ricke J, Ingrisch M, Sabel BO. Reduction of missed thoracic findings in emergency whole-body computed tomography using artificial intelligence assistance. Quant Imaging Med Surg 2021;11:2486-98. [Crossref] [PubMed]
- Aggarwal V, Maslen C, Abel RL, Bhattacharya P, Bromiley PA, Clark EM, Compston JE, Crabtree N, Gregory JS, Kariki EP, Harvey NC, Ward KA, Poole KES. Opportunistic diagnosis of osteoporosis, fragile bone strength and vertebral fractures from routine CT scans; a review of approved technology systems and pathways to implementation. Ther Adv Musculoskelet Dis 2021;13:1759720X211024029.
- Yabu A, Hoshino M, Tabuchi H, Takahashi S, Masumoto H, Akada M, et al. Using artificial intelligence to diagnose fresh osteoporotic vertebral fractures on magnetic resonance images. Spine J 2021;21:1652-8. [Crossref] [PubMed]
- Del Lama RS, Candido RM, Chiari-Correia NS, Nogueira-Barbosa MH, de Azevedo-Marques PM, Tinós R. Computer-Aided Diagnosis of Vertebral Compression Fractures Using Convolutional Neural Networks and Radiomics. J Digit Imaging 2022; [Epub ahead of print]. [Crossref] [PubMed]
- Yeh LR, Zhang Y, Chen JH, Liu YL, Wang AC, Yang JY, Yeh WC, Cheng CS, Chen LK, Su MY. A deep learning-based method for the diagnosis of vertebral fractures on spine MRI: retrospective training and validation of ResNet. Eur Spine J 2022; Epub ahead of print. [Crossref] [PubMed]
- Yoda T, Maki S, Furuya T, Yokota H, Matsumoto K, Takaoka H, Miyamoto T, Okimatsu S, Shiga Y, Inage K, Orita S, Eguchi Y, Yamashita T, Masuda Y, Uno T, Ohtori S. Automated Differentiation Between Osteoporotic Vertebral Fracture and Malignant Vertebral Fracture on MRI Using a Deep Convolutional Neural Network. Spine (Phila Pa 1976) 2022;47:E347-52. [Crossref] [PubMed]
- Wáng YXJ, Lentle BC. Radiographic osteoporotic vertebral fractures in elderly men: a brief review focusing on differences between the sexes. Quant Imaging Med Surg 2020;10:1863-76. [Crossref] [PubMed]
- Lentle BC, Brown JP, Khan A, Leslie WD, Levesque J, Lyons DJ, Siminoski K, Tarulli G, Josse RG, Hodsman ACanadian Association of Radiologists. Recognizing and reporting vertebral fractures: reducing the risk of future osteoporotic fractures. Can Assoc Radiol J 2007;58:27-36. [PubMed]
- Sugita M, Watanabe N, Mikami Y, Hase H, Kubo T. Classification of vertebral compression fractures in the osteoporotic spine. J Spinal Disord Tech 2005;18:376-81. [Crossref] [PubMed]
- Yoshida T, Nanba H, Mimatsu K, Kasai T. Treatment of osteoporotic spinal compression fractures. Conservative therapy and its limitation. Clin Calcium 2000;10:53-8.
- Wáng YXJ, Che-Nordin N. Some radiographically 'occult' osteoporotic vertebral fractures can be evidential if we look carefully. Quant Imaging Med Surg 2019;9:1992-5. [Crossref] [PubMed]
- Lentle B, Koromani F, Brown JP, Oei L, Ward L, Goltzman D, Rivadeneira F, Leslie WD, Probyn L, Prior J, Hammond I, Cheung AM, Oei EH. Vertebral Fracture Research Groups of the CaMos, STOPP, and Rotterdam Studies. The Radiology of Osteoporotic Vertebral Fractures Revisited. J Bone Miner Res 2019;34:409-18. [Crossref] [PubMed]