Amplitude of low-frequency fluctuations in multiple-frequency bands in patients with intracranial tuberculosis: a prospective cross-sectional study
Introduction
Intracranial tuberculosis, caused by Mycobacterium tuberculosis (MTB) infection of the meninges and brain tissue, is the most severe type of tuberculosis, with high incidence and mortality (1). Studies have shown that even after appropriate treatment, more than half of intracranial tuberculosis patients experience persistent neurological, cognitive, and behavioral symptoms, including motor disorders, sensory disorders, and memory disorders, which represent a lasting social-economic burden to patients and their families (2-6). This limited therapeutic effect may be related to the unclear pathogenesis of intracranial tuberculosis. However, the pathogenesis of neurological dysfunction in patients with intracranial tuberculosis, especially among those with cognitive dysfunction, remains unclear, and relevant studies are very limited. Some studies have shown that deficits in brain structures of patients with intracranial tuberculosis are associated with cognitive impairment. Specifically, Lin et al. (3) reported that white matter (WM) damage directly correlated with worse cognitive performance. Chen et al. (2) found that the gray matter volume (GMV) in multiple brain regions, including the precuneus and the superior temporal gyrus, of intracranial tuberculosis patients was reduced, which was related to the decrease of cognitive function. In addition to the study of structural damage, the study of brain function in intracranial tuberculosis is helpful for investigating the relationship between altered brain function and cognitive impairment. Previous clinical studies on other diseases, such as depression, Alzheimer’s disease, and schizophrenia, have shown that brain function research is of great significance to understanding the pathological mechanisms of neurological deficits to support early clinical diagnosis and adjuvant treatment (7-9). However, the brain function abnormalities leading to cognitive and behavioral symptoms in intracranial tuberculosis have not been reported.
Resting-state functional magnetic resonance imaging (rs-fMRI) is a promising neuroimaging technique for exploring brain function, revealing the intrinsic spontaneous activity of the brain through the changes of magnetic resonance signaling generated by alterations in the blood oxygen level (10). It has been increasingly used to understand the neural mechanisms of various central nervous system diseases, such as depression, Parkinson’s disease, and vascular cognitive impairment (7,11,12). Researchers have established various metrics to study resting-state functional data and to reflect the local and global characteristics of neural activity, which help us to understand spontaneous neural activity changes in the brain from different aspects (13-16). Among these indicators, the amplitude of low-frequency fluctuations (ALFF) and fractional ALFF (fALFF) are the two most used methods to detect local abnormalities in specific brain regions (7,8,14,17). The ALFF method measures the average amplitude of fluctuations in the low-frequency range, which is considered to be a valid method for studying the signal characteristics of single voxels or local brain regions reflecting the spontaneous neural activity of the cerebral cortex (13), and has been generally applied to study spontaneous activity of the human brain in various situations (7,14,18). Based on ALFF, Zou et al. (14) proposed the fALFF, which is obtained by calculating the ratio of the low frequency amplitude to the sum of the amplitudes of the whole frequency band. It is an improved indicator of ALFF and can reduce physiological noise and further improve the specificity and sensitivity of detecting spontaneous activity of brain neurons (17). These two indicators explain the amplitude of low frequency oscillations (LFOs) in different ways: ALFF reflects the strength of LFOs, fALFF indicates the relative contribution of LFOs within a specific frequency band to the whole detectable frequency range (17). Therefore, in this study, ALFF and fALFF were used in combination to better understand functional changes in patients with intracranial tuberculosis.
Traditional rs-fMRI studies have suggested that the brain blood oxygenation level-dependent (BOLD) signals in the frequency range of 0.01 to 0.08 Hz are physiologically significant, because the oscillating activity in this band was considered to be related to neuronal fluctuations in the gray matter (10,19). To date, most rs-fMRI studies have detected spontaneous LFO activities at the specific frequency band of 0.01–0.08 Hz (20,21). However, the human brain is the most complex organ and can produce a large number of oscillating waves (17,22). The neural signals in different frequency bands may come from different neural sources with different physiological characteristics and contribute differently to LFOs (17,22). Zuo et al. (17) focused on the sub-frequency bands of the conventional frequency band and proposed the oscillations in the slow-4 (0.027–0.073 Hz) and slow-5 (0.01–0.027 Hz) bands, which mainly reflected gray matter signals and helped us to clarify the relationship between functional processing and disease. It is worth noting that many studies have indicated that the slow-4 and slow-5 frequency bands contribute differently to the conventional frequency oscillation (11,23), and frequency-dependent abnormalities exist in many disorders (7,11). For example, Yang et al. (8) reported that abnormal amplitudes of Alzheimer’s disease were frequency-dependent, which were mainly related to the slow-5 band as opposed to than the slow-4 band. In addition, Zhan et al. (24) found that in mild traumatic brain injury, abnormal spontaneous neuronal activity in different brain regions could be detected in the slow-4 band. These studies revealed that neural oscillations at different frequencies in the human brain may be sensitive to the activity of different brain regions and can be used to explain different physiological functions of brain activity. Therefore, the present study focuses on the slow-4 band and slow-5 band when detecting spontaneous neuronal activity in patients with intracranial tuberculosis.
To our knowledge, this is the first time that ALFF and fALFF methods have been used to study regional spontaneous brain activity at different frequency bands (conventional frequency band, slow-4, and slow-5) in patients with intracranial tuberculosis. We sought to determine the following: (I) whether there are differences in brain spontaneous neural activity in patients compared to healthy controls (HCs); (II) whether the differences are associated with specific frequency bands; and (III) whether abnormal ALFF and fALFF brain areas correlate with neurocognitive scores of patients. We present the following article in accordance with the STROBE reporting checklist (available at https://qims.amegroups.com/article/view/10.21037/qims-22-17/rc).
Methods
Subjects
This was a prospective, cross-sectional study. Patients with intracranial tuberculosis were recruited from the hospital inpatient population and HCs were recruited from the community between September 2020 and July 2021. The diagnostic criteria for intracranial tuberculosis were applied according to previously published data (2,3,25,26). Intracranial tuberculosis was defined as follows: (I) a positive result for acid-fast bacilli in cerebrospinal fluid (CSF) and/or a positive MTB culture in CSF and/or a positive CSF commercial nucleic acid amplification cation test; or (II) isolation of MTB from outside the central nervous system, with clinical presentations of international tuberculous and typical CSF features, including pleocytosis (>20 cells/uL), a predominance of lymphocytes (>60%), protein >1 g/L, a blood glucose ratio of less than 0.6, and negative India ink studies and cytology for malignant cells.
The patients with intracranial tuberculosis and the HCs all met the following inclusion criteria: (I) age 18–60 years; (II) at least 6 years of education; and (III) right-handedness.
The exclusion criteria for all participants were as follows: (I) alcoholism or the use of drugs affecting brain function; (II) known history of diabetes, anxiety, depression, or history of psychiatric disorders; (III) history of neurological disorders; (IV) hearing impairment, visual impairment and physical activity impairment affecting the neuropsychological scale test; (V) any contraindications to MRI; and (VI) head motion, more than 2.5 mm of maximal translation and 2.5° of maximal rotation.
A total of 31 inpatients with intracranial tuberculosis and 31 HCs, who were group-matched by age, gender, and educational level, were recruited to the study. A case in the HC group was excluded from further analysis because the slice number was inconsistent with that of other participants. Thus, 31 patients with intracranial tuberculosis and 30 HCs were enrolled in our study (Figure 1). Meanwhile, we finalized a 10-month study time span for the following reasons: the sample size of participants met the study requirements for brain function analysis based on previously published data (24,27-29).
The study was conducted in accordance with the Declaration of Helsinki (as revised in 2013). The study was approved by the Ethics Committee of Beijing Chest Hospital, Capital Medical University (No. YJS-2020-010). All the participants signed written informed consent prior to participating in this study.
Neurocognitive tests
The cognitive status of each participant was assessed using a series of neurocognitive tests in a fixed order, covering major cognitive domains. All participants underwent each neurocognitive test. General cognitive levels were evaluated by the Mini-Mental State Examination (MMSE) and the Montreal Cognitive Assessment (MoCA) tests (30). The Trail Making Test parts A and B (TMT-A and TMT-B) were used to assess attention, executive function, and psychomotor speed (31). The Clock Drawing Test (CDT) was used to evaluate executive function and visuo-spatial abilities (32). The Digit Span Test (DST) was used to assess attention and working memory span (33,34). The Verbal Fluency Test (VFT) was used to measure language or executive function (35). The Rey Auditory Verbal Learning Test, including immediate recall (RAVLT-I), delayed recall (RAVLT-II), and total scores (RAVLT), was used to evaluate verbal memory (36). The Symbol Digit Modalities Test (SDMT) was used to assess information processing speed (36).
MRI data acquisition
We acquired MRI data using a 3-T SIGNA pioneer scanner (GE Healthcare, Chicago, IL, USA) with a 32-channel head coil after undertaking the clinical data collection and neuropsychological testing. Participants were instructed to lie in a supine position with their head secured with a foam sponge pad to reduce head movement and to wear earplugs to reduce noise. During MRI data collection, participants were told to close their eyes and remain awake at all times. The scanning parameters of resting-state functional images were as follows: repetition time (TR) =3,000 ms, echo time (TE) =35 ms, slice =30, slice thickness =3.5 mm, gap =0 mm, voxel size =3.8×3.8×3.5 mm3, flip angle =90°, field of view (FOV) =240×240 mm2, matrix size =64×64, and total scanning time =384 seconds. The scanning parameters of the three-dimensional (3D), high-resolution, T1-weighted images were as follows: TR =7,400 ms, TE =1.0 ms, flip angle =9º, FOV =240×240 mm2, matrix size =256×256, 252 slices, slice thickness =1.2 mm without interslice gap, voxel size =0.9×0.9×1.2 mm3, and total scan time =169 seconds. In addition, T2-weighted fluid-attenuated inversion recovery (T2-FLAIR) images of 31 patients and gadolinium-enhanced T1-weighted (Gd-T1w) images of 29 patients were collected to better characterize the brain lesions. The results are shown in the supplementary materials (see Figures S1-S31 in the supplementary materials for details).
Rs-fMRI preprocessing
We preprocessed the rs-fMRI data using the Resting-State fMRI Data Analysis Toolkit plus (REST plus) v.1.24 toolkit (http://www.restfmri.net) (37), which was implemented using a Matrix Laboratory (MATLAB) 2017b platform (MathWorks, Natick, MA, USA). For each participant, the preprocessing steps were as follows: (I) deleting the first 10 time points to exclude the influence of the participants’ maladaptation at the beginning of the scan and the uneven magnetic field; (II) slice timing correction to correct the differences in image acquisition time between slices; (III) head motion correction to adjust the time series of images so that the brain was in the same position in every image (38); (IV) spatially normalizing the corrected data to the Montreal Neurological Institute (MNI) template (resampling resolution =3 mm3); (V) spatially smoothing the data with a 6 mm3 full width at half maximum (FWHM) Gaussian kernel to ensure a high signal-to-noise ratio; (VI) removing the linear trend to eliminate the influence of long-term physiological shifts, movement related noise remaining after realignment, or instrumental instability that might have contributed to a systematic increase or decrease in the signal with time (39,40); and (VII) nuisance covariates regression (Friston-24 head motion parameters (41), CSF signal, WM signal and global signal) to reduce the effects of head motion and non-neuronal BOLD fluctuations (42,43). No participants were excluded due to excessive head motion (maximum translation in any direction >2.5 mm and maximum rotation >2.5°). Keeping other preprocessing steps unchanged, the spatial patterns of the results of removing the first 5 time points and removing the first 10 time points were compared (see Figures S32-S37 in the supplementary materials for details).
ALFF and fALFF calculation
We analyzed ALFF and fALFF using the REST plus v.1.24 toolkit. In ALFF analysis, the time series of each individual voxel were converted to the frequency domain by the fast Fourier transform (FFT) to obtain the power spectrum. The square root of power spectrum of each frequency was calculated. The average square root of power spectrum for all frequencies was ALFF (13). In addition to the conventional frequency band, we also calculated ALFF in the slow-4 band and the slow-5 band. For standardization purpose, the voxel-wise ALFF data for each participant was converted into a z-score by subtracting the global mean value and then dividing by the standard deviation (SD) of the whole-brain ALFF (14).
The fALFF was calculated as the ratio of the power spectrum at the low-frequency range (0.01 to 0.08 Hz) to that of the whole frequency range (0 to 0.25 Hz) (14). In addition to the conventional low-frequency band of 0.01–0.08 Hz, we also calculated fALFF in the slow-4 band and the slow-5 band. The standardization procedure was the same as that described above.
Statistical analysis
Demographic data and neuropsychological test scores were compared using the statistical software SPSS 20.0 (IBM Corp., Armonk, NY, USA). The independent samples t-test and the Mann-Whitney U test were performed to normally distributed continuous data and non-normally distributed data, respectively. The data distribution was analyzed using the Shapiro-Wilk test. We used the χ2 test for gender proportions. Statistical significance was considered when P<0.05.
The two-sample t-tests were used to analyze the differences of the ALFF and fALFF values in the three different frequency bands mentioned above between the two participant groups and to identify the regions with significant differences. The resultant T-maps were corrected using the Gaussian random field (GRF) theory (voxel P<0.01, cluster P<0.05). All of the statistical analysis was conducted using REST plus v.1.24. At the same time, we tested the normality of the data, and the results are provided in the supplementary materials (see Figures S38-S47 in the supplementary materials for details).
We further correlated the mean ALFF and mean fALFF of each region with significant differences in the neuropsychological tests by using a Pearson’s correlation analysis for normally distributed data and a Spearman’s correlation analysis for non-normally distributed data, which aimed to investigate the relationship between the regional spontaneous brain activity and the clinical symptoms of intracranial tuberculosis. Bonferroni correction was carried out in correlation analyses. In addition, partial correlations were also conducted between the clinical scores and metric values with gender, age, and educational level used as the covariates for both the HC group and the patient group. The results are presented in the supplementary materials (see Tables S1,S2 in the supplementary materials for details). All of the statistical analysis was conducted with SPSS 20.0 software.
Finally, signals of brain regions showing significant differences were extracted and plotted as a box plot at the conventional frequency band, slow-4 band, and slow-5 band (see Figures S48-S57 in the supplementary materials for details).
Results
Demographic and clinical features of the participants
A total of 31 patients with intracranial tuberculosis and 30 HCs were enrolled in this study. The demographic and clinical characteristics of the participants are summarized in Table 1. There was no significant difference in demographic variables (age, gender, and education) between the groups. The patients with intracranial tuberculosis performed worse on the tests of MMSE, MoCA, TMT-A, VFT, RAVLT-I, RAVLT-II, RAVLT, and SDMT (all P<0.05). No significant between-group differences were observed in the other neuropsychological tests. Based on the findings of T2-FLAIR and Gd-T1w images, there were 6 (6/31, 19.35%) cases with extra-axial structural lesions, 9 (9/31, 29.03%) cases with intra-axial structural lesions, 10 (10/31, 32.26%) cases with both extra-axial and intra-axial structural lesions, and 6 (6/31, 19.35%) cases without structural abnormalities in 31 patients with intracranial tuberculosis.
Table 1
Demographics | Patients (n=31) | HCs (n=30) | P value |
---|---|---|---|
Age (years) | 33 [26, 46] | 31.5 [23.75, 47] | 1.00a |
Gender (male/female) | 20/11 | 19/11 | 0.92b |
Education (years) | 11.16±3.15 | 11.17±3.21 | 1.00 |
MMSE | 28 [28, 30] | 30 [29, 30] | 0.01*a |
MoCA | 24 [19, 26] | 27 [23.75, 28] | 0.00*a |
TMT-A (s) | 52.94±28.77 | 37.77±17.49 | 0.02* |
TMT-B (s) | 132.58±67.96 | 103.53±66.50 | 0.10 |
CDT | 5 [4, 5] | 5 [4, 5] | 0.07a |
DST forwards | 10.03±3.15 | 10.87±1.98 | 0.22 |
DST backwards | 6.87±2.80 | 8.33±2.77 | 0.05 |
VFT | 39.39±9.82 | 45.7±10.50 | 0.02* |
RAVLT-I | 36.74±13.06 | 46.37±11.38 | 0.00* |
RAVLT-II | 7.58±4.02 | 9.93±3.59 | 0.02* |
RAVLT | 44.32±16.72 | 56.30±14.43 | 0.00* |
SDMT | 42.35±13.48 | 50.10±13.53 | 0.03* |
All participants underwent each neurocognitive test. *P<0.05. a, Mann-Whitney U-test for non-normally distributed data {median [lower quartile, upper-quartile]}. b, χ2 test for gender (n). Independent t-test for the other normally distributed continuous data (means ± SD). HCs, healthy controls; MMSE, Mini-Mental State Examination; MoCA, Montreal Cognitive Assessment; TMT, Trail Making Test; CDT, Clock Drawing Test; DST, Digital Span Test; VFT, Verbal Fluency Test; RAVLT-I, Rey Auditory Verbal Learning Test (total immediate recall); RAVLT-II, Rey Auditory Verbal Learning Test (delayed recall); RAVLT, Rey Auditory Verbal Learning Test (total score); SDMT, Symbol-Digit Modalities Test.
Group differences in ALFF in different frequency bands
Compared with HCs, patients with intracranial tuberculosis showed decreased ALFF in the right paracentral lobule [Brodmann Area (BA) 6; T=−4.69; GRF correction with voxel P<0.01; cluster P<0.05] in the conventional frequency band (Table 2, Figure 2A), the right supplementary motor area (BA6; T=−4.85; GRF correction with voxel P<0.01; cluster P<0.05) in the slow-4 band (Table 2, Figure 2B), and the left supplementary motor area (BA6; T=−3.76; GRF correction with voxel P<0.01; cluster P<0.05) in the slow-5 band (Table 2, Figure 2C).
Table 2
Brain regions | BA | Peak MNI coordinates | Cluster (voxels) | Peak T-value | ||
---|---|---|---|---|---|---|
X | Y | Z | ||||
Conventional frequency band (0.01–0.08 Hz) | ||||||
Paracentral_Lobule_R | 6 | 3 | −24 | 78 | 223 | −4.69 |
Slow-4 (0.027–0.073 Hz) | ||||||
Supp_Motor_Area_R | 6 | 0 | −6 | 78 | 201 | −4.85 |
Slow-5 (0.01–0.027 Hz) | ||||||
Supp_Motor_Area_L | 6 | 0 | 6 | 72 | 75 | −3.76 |
BA, Brodmann Area; MNI, Montreal Neurological Institute; Paracentral_Lobule_R, right paracentral lobule; Supp_Motor_Area_R, right supplementary motor area; Supp_Motor_Area_L, left supplementary motor area; ALFF, amplitude of low-frequency fluctuation.
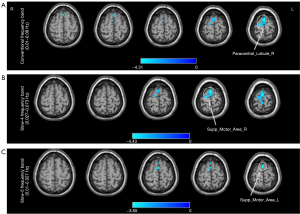
Group differences in fALFF in different frequency bands
Compared with HCs, patients with intracranial tuberculosis showed decreased fALFF in the opercular parts of the right inferior frontal gyrus (BA9; T=−4.50; GRF correction with voxel P<0.01; cluster P<0.05) and left inferior parietal lobe (BA40; T=−4.86; GRF correction with voxel P<0.01; cluster P<0.05), and increased fALFF in the left inferior cerebellum (T=5.84; GRF correction with voxel P<0.01; cluster P<0.05) in the conventional frequency band (Table 3, Figure 3A). In the slow-4 band, fALFF decreased in the opercular parts of the right inferior frontal gyrus (BA46; T=−5.29; GRF correction with voxel P<0.01; cluster P<0.05) and right precuneus (BA7; T=−4.34, GRF correction with voxel P<0.01, cluster P<0.05) (Table 3, Figure 3B). In the slow-5 band, fALFF decreased in the left middle occipital gyrus (BA39; T=−4.65, GRF correction with voxel P<0.01; cluster P<0.05) and right middle frontal gyrus (BA6; T=−5.05; GRF correction with voxel P<0.01; cluster P<0.05) (Table 3, Figure 3C). In addition, we applied voxel-based morphometry (VBM) analysis to investigate whether there were structural differences between the two groups. The specific calculation procedure and the results are shown in the supplementary material (see Figure S58 and Table S3 in the supplementary materials for details).
Table 3
Brain regions | BA | Peak MNI coordinates | Cluster (voxels) | Peak T-value | ||
---|---|---|---|---|---|---|
X | Y | Z | ||||
Conventional frequency band (0.01–0.08 Hz) | ||||||
Cerebelum_9_L | −12 | −57 | −57 | 158 | 5.84 | |
Frontal_Inf_Oper_R | 9 | 42 | 9 | 24 | 230 | −4.50 |
Parietal_Inf_L | 40 | −33 | −39 | 36 | 83 | −4.86 |
Slow-4 (0.027–0.073 Hz) | ||||||
Frontal_Inf_Oper_R | 46 | 42 | 9 | 30 | 110 | −5.29 |
Precuneus_R | 7 | 3 | −69 | 33 | 71 | −4.34 |
Slow-5 (0.01–0.027 Hz) | ||||||
Occipital_Mid_L | 39 | −33 | −75 | 36 | 147 | −4.65 |
Frontal_Mid_R | 6 | 33 | 3 | 54 | 122 | −5.05 |
fALFF, fractional amplitude of low-frequency fluctuation; BA, Brodmann Area; MNI, Montreal Neurological Institute; Cerebelum_9_L, left cerebellum_Inferior; Frontal_Inf_Oper_R, opercular parts of the right inferior frontal gyrus; Parietal_Inf_L, left inferior parietal; Precuneus_R, right precuneus; Occipital_Mid_L, left middle occipital gyrus; Frontal_Mid_R, right middle frontal gyrus.
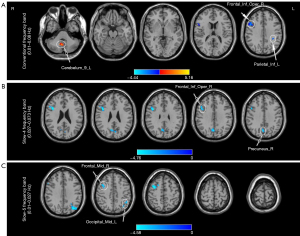
Correlation analysis
Correlation analysis with Bonferroni correction showed no significant correlation between altered ALFF/fALFF values of patients with intracranial tuberculosis in brain regions and all of the neuropsychological test scores in the conventional band, the slow-4 band, and the slow-5 band.
Discussion
To our knowledge, this was the first study to explore the changes of brain activity in patients with intracranial tuberculosis. We analyzed the ALFF and fALFF of intracranial tuberculosis patients in the conventional frequency band and two sub-frequency bands (slow-4 and slow-5), respectively. Our study demonstrated that patients with intracranial tuberculosis showed decreased ALFF in the right paracentral lobule (BA6) in the conventional frequency band, the right supplementary motor area (BA6) in the slow-4 band, and the left supplementary motor area (BA6) in the slow-5 band. Notably, compared to the slow-5 band, the voxels with decreased ALFF were spatially more extensive in the slow-4 band. Compared with HCs, decreased fALFF in the opercular parts of the right inferior frontal gyrus in the conventional frequency band and the slow-4 band was found in patients with intracranial tuberculosis. In the conventional frequency band, fALFF was decreased in the left inferior parietal lobe (BA40) and increased in the left inferior cerebellum. In the slow-4 band, fALFF decreased in the right precuneus (BA7). In the slow-5 band, fALFF decreased in the left middle occipital gyrus (BA39) and the right middle frontal gyrus (BA6). Thus, our results showed that sub-frequency band analyses can provide additional information that may be more useful in detecting disease abnormalities.
In this present study, we found decreased ALFF values in the right paracentral lobule (BA6) and the bilateral supplementary motor areas (BA6), which directly implied reduced neuronal activity and associated functional deficits in these areas (44). Researchers (45-47) have asserted that the state of neurons may be related to this phenomenon. Neurons are the carriers of redox reactions. When the central nervous system is injured, excessive oxidative stress can cause functional abnormalities in nerve cells, and such changes are detected by fMRI. The paracentral lobule is involved in somatic movement and sensation as the first somatosensory area, and also participates in motor control and limbic sensory function as an important structure connecting the precentral gyrus and the postcentral gyrus (48,49). It also has been found that the supplementary motor area, as an important node of the motor network, is closely involved in both simple spontaneous somatic movements and advanced complex movement control and coordination (50,51). A clinical study (4) on patients with intracranial tuberculosis reported that the prevalence of mild alteration of sensorium, focal motor deficit, and hemiparesis in 65 patients were 15.38%, 40%, and 24.62% respectively. Wen et al. (5) also found that patients with intracranial tuberculosis experienced focal motor deficits and sensory changes. Based on these results, we speculated that altered local neuronal activity in the cortex related to motion and sensation might be an important cause of impaired motor and sensory functions in patients with intracranial tuberculosis.
In the present study, decreased fALFF in the opercular parts of the right inferior frontal gyrus (BA9, BA46) and right middle frontal gyrus (BA6) indicated reduced spontaneous neuronal activity and metabolism in these brain regions in patients with intracranial tuberculosis, suggesting that patients may have associated functional deficits (14,17). Misra et al. (52) used single photon emission computed tomography (SPECT) to measure changes in perfusion in tuberculous meningitis patients and showed hypoperfusion in the frontal cortex. The inferior frontal gyrus, the opercular part of the inferior frontal gyrus (BA9, BA46), and the middle frontal gyrus (BA6) are important parts of the dorsolateral prefrontal cortex, and they play key roles in many cognitive processes, including attention, working memory, decision making, and executive function, among other functions (53,54). In this study, patients with intracranial tuberculosis performed more poorly than HCs on the TMT-A, VFT, and SDMT tests, indicating that cognitive dysfunction in these patients is more likely to involve the domains of executive function, attention, working memory, and information processing speed, respectively. These results are consistent with the research of Chen et al. (2). Thus, we speculated that the decrease of spontaneous neuronal activity in the functional areas of the inferior frontal gyrus, opercular part of the inferior frontal gyrus (BA9, BA46), and the middle frontal gyrus (BA6) might be the neural basis of cognitive impairment in patients. In addition to the frontal lobe, fALFF was decreased in the left inferior parietal lobe (BA40) and right precuneus (BA7) of the patients, indicating that infection with intracranial tuberculosis reduced spontaneous neural activity in these brain regions. The left inferior parietal lobe (BA40) and precuneus (BA7) are important brain regions that make up the default mode network (DMN) associated with attention and memory (55,56). In this study, patients with intracranial tuberculosis performed more poorly than HCs on the TMT-A, indicating that cognitive dysfunction in these patients is more likely to involve the domains of attention and working memory. These results were consistent with the findings of Chen et al. (2). In addition, as one of the brain regions with the highest metabolic rate, the precuneus plays a key role in a variety of advanced cognitive processes, such as cognitive function, visuospatial imagery, and memory (57,58). Luo et al. (59) and Cui et al. (60) reported that brain activity was decreased in the precuneus in patients with mild cognitive impairment. Meanwhile, Chen et al. (2) observed that patients with cerebral tuberculosis had lower regional gray matter volumes in the right precuneus, which was associated with lower scores for memory. We found that patients had poorer performance and significantly reduced cognitive function on the MMSE and MoCA tests, relative to HCs. Therefore, changes in the structural and neuronal activity of the precuneus may imply an important role for the incidence of cognitive impairment in patients with intracranial tuberculosis. Notably, we also identified reduced fALFF in the left middle occipital gyrus in patients with intracranial tuberculosis, suggesting decreased spontaneous neural activity. The function of the middle occipital gyrus located in the visual processing center is to synthesize visual information (61). Chen et al. (62) reported decreased fALFF values in the left middle occipital gyrus in patients with thyroid-associated ophthalmopathy. Intracranial tuberculosis patients may have symptoms of diplopia and visual disturbance (63). Therefore, we hypothesized that changes in structural and neuronal activity of the left middle occipital gyrus may imply an important role in the incidence of visual impairment in intracranial tuberculosis patients. In addition, abnormalities of the structure in the brains of patients with intracranial tuberculosis were quantified using VBM. We found the GMV of the left middle occipital gyrus was reduced significantly in patients with intracranial tuberculosis compared with HCs. Previous studies have shown that anatomical and functional deficits are linked to each other, and abnormal baseline brain activity may be due to brain injury (64,65). Therefore, we also suggested that fALFF abnormalities in the middle occipital gyrus may be associated with structural damage. Besides, studies have found that the cerebellum contributes to complex cognitive operations (66,67). Thomann et al. (68) also reported that posterior cerebellar atrophy was related to poor cognitive ability in Alzheimer’s disease. However, in our study, the fALFF value of the left inferior cerebellum in patients with intracranial tuberculosis was increased compared with HCs, suggesting that spontaneous brain neural activity was enhanced in this area. This may be a compensation mechanism for maintaining normal performance (69) and needs to be further investigated in the future.
It is worth noting that the abnormal spontaneous neural activity measured by rs-fMRI in patients with intracranial tuberculosis was frequency-specific. Specifically, compared to the slow-5 band, we found that the voxels with decreased ALFF were spatially more extensive in the slow-4 band. Previous studies have also shown that the slow-4 band and slow-5 band have different degrees of sensitivity to different diseases. For example, Zhan et al. (24) found that the slow-4 band was more sensitive in detecting changes of spontaneous brain activity in the frontal regions of mild traumatic brain injury patients, using the ALFF method. Hoptman et al. (70) observed widespread abnormal ALFF values in slow-4 bands in patients with schizophrenia. Our results therefore suggest that ALFF analysis of the slow-4 band in patients with intracranial tuberculosis may provide additional useful information, compared with ALFF analysis of the slow-5 band. Meantime, we used the fALFF method to detect local spontaneous brain activity of intracranial tuberculosis and found that fALFF alterations in two sub-frequency bands (slow-4 and slow-5) showed no significant specificity. Two sub-frequency bands both contributed to the results detected in the conventional frequency band and both identified new brain regions showing altered LFOs which were different from the brain regions detected in the conventional frequency band. This may reveal that fALFF is not sensitive to exploring frequency band specificity, which has been shown in some previous studies. Yang et al. (44) reported no frequency band specificity in two sub-frequency bands (slow-4 and slow-5) in patients with Alzheimer’s disease using the fALFF method. Liu et al. (71) also produced similar results from patients with amnestic mild cognitive impairment using the fALFF method. In conclusion, our results suggest that brain regions with altered spontaneous neural activity in patients with intracranial tuberculosis differed in different frequency bands. The mechanisms of this phenomenon need to be further investigated in the future. Notably, we also found that compared with the slow-5 band, the slow-4 band was more sensitive in detecting ALFF abnormalities in patients with intracranial tuberculosis, suggesting that ALFF analysis of the slow-4 band may provide additional useful information compared with that of the slow-5 band.
Although we found different regional characteristics with mostly widespread decreases in brain functional changes in intracranial tuberculosis patients with ALFF and fALFF, some limitations should be considered. First, due to the cross-sectional group data, we were unable to observe the dynamic changes in ALFF and fALFF during different progressions in patients with intracranial tuberculosis. In future studies, further attention needs to be given to longitudinal changes in neuronal activity. Second, the fMRI data of patients with intracranial tuberculosis were difficult to obtain, resulting in a relatively small sample size in this study, which also made it difficult for us to further distinguish the types of patients with intracranial tuberculosis. In addition, the sample distribution in this study was not normal. Therefore, the results of this study should be extrapolated to the whole population of patients with intracranial tuberculosis with caution. In future studies, more multi-center studies with larger sample sizes are needed to overcome these difficulties. Third, in order to recruit more patients with intracranial tuberculosis, patients with structural lesions demonstrated by T2-FLAIR and Gd-T1w imaging scans were not excluded. In future studies, patients should be further strictly screened to exclude the impact of structural lesions on the results. Fourth, we mainly studied the local brain neural activity in patients with intracranial tuberculosis and could not obtain the characteristics of changes in the correlation of neural activity between different brain regions of the whole brain. Future studies should focus on the characteristics of the brain network level in patients with intracranial tuberculosis.
Conclusions
In conclusion, we found that aberrant ALFF and fALFF were mainly distributed in the frontal lobe, parietal lobe, middle occipital gyrus, and cerebellum, which are related to cognitive impairment. Furthermore, altered spontaneous neural activity was frequency specific. Specifically, the slow-4 band may be better for detecting ALFF abnormalities in comparison to the slow-5 band. In future studies, we should consider frequency specificity when measuring changes in intrinsic brain activity in patients with intracranial tuberculosis. To our knowledge, this was the first study of changes in the spontaneous brain activity of intracranial tuberculosis in different frequency bands. Thus, our findings may help elucidate the neuropathological basis of intracranial tuberculosis, which would be of great significance for supporting an early clinical diagnosis. Concurrently, these observations also make the future treatment of intracranial tuberculosis more directional and targeted, and promote the development of intracranial tuberculosis treatment.
Acknowledgments
Funding: This study was supported by the Beijing Hospitals Authority Clinical Medicine Development of Special Funding (No. XMLX202146) and the Talent Introduction Project of Beijing Chest Hospital, Capital Medical University (No. 2019-3).
Footnote
Reporting Checklist: The authors have completed the STROBE reporting checklist. Available at https://qims.amegroups.com/article/view/10.21037/qims-22-17/rc
Conflicts of Interest: All authors have completed the ICMJE uniform disclosure form (available at https://qims.amegroups.com/article/view/10.21037/qims-22-17/coif). The authors report that this study was supported by the Beijing Hospitals Authority Clinical Medicine Development of Special Funding (No. XMLX202146) and Talent Introduction Project of Beijing Chest Hospital, Capital Medical University (No. 2019-3). The authors have no other conflicts of interest to declare.
Ethical Statement: The authors are accountable for all aspects of the work in ensuring that questions related to the accuracy or integrity of any part of the work are appropriately investigated and resolved. The study was conducted in accordance with the Declaration of Helsinki (as revised in 2013). The study was approved by the Ethics Committee of Beijing Chest Hospital, Capital Medical University (No. YJS-2020-010). All the participants signed written informed consent prior to participating in this study.
Open Access Statement: This is an Open Access article distributed in accordance with the Creative Commons Attribution-NonCommercial-NoDerivs 4.0 International License (CC BY-NC-ND 4.0), which permits the non-commercial replication and distribution of the article with the strict proviso that no changes or edits are made and the original work is properly cited (including links to both the formal publication through the relevant DOI and the license). See: https://creativecommons.org/licenses/by-nc-nd/4.0/.
References
- Dian S, Ganiem AR, van Laarhoven A. Central nervous system tuberculosis. Curr Opin Neurol 2021;34:396-402. [Crossref] [PubMed]
- Chen HL, Lu CH, Chang CD, Chen PC, Chen MH, Hsu NW, Chou KH, Lin WM, Lin CP, Lin WC. Structural deficits and cognitive impairment in tuberculous meningitis. BMC Infect Dis 2015;15:279. [Crossref] [PubMed]
- Lin WC, Chen PC, Wang HC, Tsai NW, Chou KH, Chen HL, Su YJ, Lin CP, Li SH, Chang WN, Lu CH. Diffusion tensor imaging study of white matter damage in chronic meningitis. PLoS One 2014;9:e98210. [Crossref] [PubMed]
- Kalita J, Misra UK, Ranjan P. Predictors of long-term neurological sequelae of tuberculous meningitis: a multivariate analysis. Eur J Neurol 2007;14:33-7. [Crossref] [PubMed]
- Wen L, Li M, Xu T, Yu X, Wang L, Li K. Clinical features, outcomes and prognostic factors of tuberculous meningitis in adults worldwide: systematic review and meta-analysis. J Neurol 2019;266:3009-21. [Crossref] [PubMed]
- Rock RB, Olin M, Baker CA, Molitor TW, Peterson PK. Central nervous system tuberculosis: pathogenesis and clinical aspects. Clin Microbiol Rev 2008;21:243-61. table of contents. [Crossref] [PubMed]
- Zhou J, Ma X, Li C, Liao A, Yang Z, Ren H, Tang J, Li J, Li Z, He Y, Chen X. Frequency-Specific Changes in the Fractional Amplitude of the Low-Frequency Fluctuations in the Default Mode Network in Medication-Free Patients With Bipolar II Depression: A Longitudinal Functional MRI Study. Front Psychiatry 2021;11:574819. [Crossref] [PubMed]
- Yang L, Yan Y, Li Y, Hu X, Lu J, Chan P, Yan T, Han Y. Frequency-dependent changes in fractional amplitude of low-frequency oscillations in Alzheimer's disease: a resting-state fMRI study. Brain Imaging Behav 2020;14:2187-201. [Crossref] [PubMed]
- Zhang Y, Yang R, Cai X. Frequency-specific alternations in the moment-to-moment BOLD signals variability in schizophrenia. Brain Imaging Behav 2021;15:68-75. [Crossref] [PubMed]
- Fox MD, Raichle ME. Spontaneous fluctuations in brain activity observed with functional magnetic resonance imaging. Nat Rev Neurosci 2007;8:700-11. [Crossref] [PubMed]
- Rong S, Zhang P, He C, Li Y, Li X, Li R, Nie K, Huang S, Wang L, Wang L, Zhang Y. Abnormal Neural Activity in Different Frequency Bands in Parkinson's Disease With Mild Cognitive Impairment. Front Aging Neurosci 2021;13:709998. [Crossref] [PubMed]
- Wang R, Liu N, Tao YY, Gong XQ, Zheng J, Yang C, Yang L, Zhang XM. The Application of rs-fMRI in Vascular Cognitive Impairment. Front Neurol 2020;11:951. [Crossref] [PubMed]
- Zang YF, He Y, Zhu CZ, Cao QJ, Sui MQ, Liang M, Tian LX, Jiang TZ, Wang YF. Altered baseline brain activity in children with ADHD revealed by resting-state functional MRI. Brain Dev 2007;29:83-91. [Crossref] [PubMed]
- Zou QH, Zhu CZ, Yang Y, Zuo XN, Long XY, Cao QJ, Wang YF, Zang YF. An improved approach to detection of amplitude of low-frequency fluctuation (ALFF) for resting-state fMRI: fractional ALFF. J Neurosci Methods 2008;172:137-41. [Crossref] [PubMed]
- Su T, Yuan Q, Liao XL, Shi WQ, Zhou XZ, Lin Q, Min YL, Li B, Jiang N, Shao Y. Altered intrinsic functional connectivity of the primary visual cortex in patients with retinal vein occlusion: a resting-state fMRI study. Quant Imaging Med Surg 2020;10:958-69. [Crossref] [PubMed]
- Feng N, Gao M, Wu J, Yang G, Piao R, Liu P. Higher inter-hemispheric homotopic connectivity in lifelong premature ejaculation patients: a pilot resting-state fMRI study. Quant Imaging Med Surg 2021;11:3234-43. [Crossref] [PubMed]
- Zuo XN, Di Martino A, Kelly C, Shehzad ZE, Gee DG, Klein DF, Castellanos FX, Biswal BB, Milham MP. The oscillating brain: complex and reliable. Neuroimage 2010;49:1432-45. [Crossref] [PubMed]
- Zhang Z, Bo Q, Li F, Zhao L, Wang Y, Liu R, Chen X, Wang C, Zhou Y. Increased ALFF and functional connectivity of the right striatum in bipolar disorder patients. Prog Neuropsychopharmacol Biol Psychiatry 2021;111:110140. [Crossref] [PubMed]
- Biswal B, Yetkin FZ, Haughton VM, Hyde JS. Functional connectivity in the motor cortex of resting human brain using echo-planar MRI. Magn Reson Med 1995;34:537-41. [Crossref] [PubMed]
- Feng M, Wen H, Xin H, Zhang N, Liang C, Guo L. Altered Spontaneous Brain Activity Related to Neurologic Dysfunction in Patients With Cerebral Small Vessel Disease. Front Aging Neurosci 2021;13:731585. [Crossref] [PubMed]
- Huang L, Huang G, Ding Q, Liang P, Hu C, Zhang H, Zhan L, Wang Q, Cao Y, Zhang J, Shen W, Jia X, Xing W. Amplitude of low-frequency fluctuation (ALFF) alterations in adults with subthreshold depression after physical exercise: A resting-state fMRI study. J Affect Disord 2021;295:1057-65. [Crossref] [PubMed]
- Buzsáki G, Draguhn A. Neuronal oscillations in cortical networks. Science 2004;304:1926-9. [Crossref] [PubMed]
- Luo FF, Wang JB, Yuan LX, Zhou ZW, Xu H, Ma SH, Zang YF, Zhang M. Higher Sensitivity and Reproducibility of Wavelet-Based Amplitude of Resting-State fMRI. Front Neurosci 2020;14:224. [Crossref] [PubMed]
- Zhan J, Gao L, Zhou F, Bai L, Kuang H, He L, Zeng X, Gong H. Amplitude of Low-Frequency Fluctuations in Multiple-Frequency Bands in Acute Mild Traumatic Brain Injury. Front Hum Neurosci 2016;10:27. [Crossref] [PubMed]
- Marais S, Thwaites G, Schoeman JF, Török ME, Misra UK, Prasad K, Donald PR, Wilkinson RJ, Marais BJ. Tuberculous meningitis: a uniform case definition for use in clinical research. Lancet Infect Dis 2010;10:803-12. [Crossref] [PubMed]
- Thwaites G, Fisher M, Hemingway C, Scott G, Solomon T, Innes JBritish Infection Society. British Infection Society guidelines for the diagnosis and treatment of tuberculosis of the central nervous system in adults and children. J Infect 2009;59:167-87. [Crossref] [PubMed]
- Xue S, Wang X, Wang W, Liu J, Qiu J. Frequency-dependent alterations in regional homogeneity in major depression. Behav Brain Res 2016;306:13-9. [Crossref] [PubMed]
- Zhang S, Li H, Xu Q, Wang C, Li X, Sun J, Wang Y, Sun T, Wang Q, Zhang C, Wang J, Jia X, Sun X. Regional homogeneity alterations in multi-frequency bands in tension-type headache: a resting-state fMRI study. J Headache Pain 2021;22:129. [Crossref] [PubMed]
- Desmond JE, Glover GH. Estimating sample size in functional MRI (fMRI) neuroimaging studies: statistical power analyses. J Neurosci Methods 2002;118:115-28. [Crossref] [PubMed]
- Pinto TCC, Machado L, Bulgacov TM, Rodrigues-Júnior AL, Costa MLG, Ximenes RCC, Sougey EB. Is the Montreal Cognitive Assessment (MoCA) screening superior to the Mini-Mental State Examination (MMSE) in the detection of mild cognitive impairment (MCI) and Alzheimer's Disease (AD) in the elderly?. Int Psychogeriatr 2019;31:491-504. [Crossref] [PubMed]
- Bowie CR, Harvey PD. Administration and interpretation of the Trail Making Test. Nat Protoc 2006;1:2277-81. [Crossref] [PubMed]
- Shulman KI. Clock-drawing: is it the ideal cognitive screening test? Int J Geriatr Psychiatry 2000;15:548-61. [Crossref] [PubMed]
- Amiel Castro R, Kunovac Kallak T, Sundström Poromaa I, Willebrand M, Lager S, Ehlert U, Skalkidou A. Pregnancy-related hormones and COMT genotype: Associations with maternal working memory. Psychoneuroendocrinology 2021;132:105361. [Crossref] [PubMed]
- Zhou H, Lu S, Chen J, Wei N, Wang D, Lyu H, Shi C, Hu S. The landscape of cognitive function in recovered COVID-19 patients. J Psychiatr Res 2020;129:98-102. [Crossref] [PubMed]
- Whiteside DM, Kealey T, Semla M, Luu H, Rice L, Basso MR, Roper B. Verbal Fluency: Language or Executive Function Measure? Appl Neuropsychol Adult 2016;23:29-34. [Crossref] [PubMed]
- Lezak MD, Howison DB, Loring DW. Neuropsychological assessment. 4th ed. New York: Oxford University Press; 2004. p. 478-514.
- Jia XZ, Wang J, Sun HY, Zhang H, Liao W, Wang Z, Yan CG, Song XW, Zang YF. RESTplus: an improved toolkit for resting-state functional magnetic resonance imaging data processing. Science Bulletin 2019;64. [Crossref]
- Huettel SA, Song AW, McCarthy G. Functional magnetic resonance imaging. 2nd ed. Sinauer, Sunderland; 2008.
- Lowe MJ, Russell DP. Treatment of baseline drifts in fMRI time series analysis. J Comput Assist Tomogr 1999;23:463-73. [Crossref] [PubMed]
- Song XW, Dong ZY, Long XY, Li SF, Zuo XN, Zhu CZ, He Y, Yan CG, Zang YF. REST: a toolkit for resting-state functional magnetic resonance imaging data processing. PLoS One 2011;6:e25031. [Crossref] [PubMed]
- Friston KJ, Williams S, Howard R, Frackowiak RS, Turner R. Movement-related effects in fMRI time-series. Magn Reson Med 1996;35:346-55. [Crossref] [PubMed]
- Fox MD, Snyder AZ, Vincent JL, Corbetta M, Van Essen DC, Raichle ME. The human brain is intrinsically organized into dynamic, anticorrelated functional networks. Proc Natl Acad Sci U S A 2005;102:9673-8. [Crossref] [PubMed]
- Kelly AM, Uddin LQ, Biswal BB, Castellanos FX, Milham MP. Competition between functional brain networks mediates behavioral variability. Neuroimage 2008;39:527-37. [Crossref] [PubMed]
- Yang L, Yan Y, Wang Y, Hu X, Lu J, Chan P, Yan T, Han Y. Gradual Disturbances of the Amplitude of Low-Frequency Fluctuations (ALFF) and Fractional ALFF in Alzheimer Spectrum. Front Neurosci 2018;12:975. [Crossref] [PubMed]
- Zheng P, Mei J, Leng J, Jia S, Gu Z, Chen S, Zhang W, Cheng A, Guo D, Lang J. Evaluation of the brain functional activities in rats various location-endometriosis pain model. Ann Transl Med 2019;7:767. [Crossref] [PubMed]
- Pei JP, Fan LH, Nan K, Li J, Dang XQ, Wang KZ. HSYA alleviates secondary neuronal death through attenuating oxidative stress, inflammatory response, and neural apoptosis in SD rat spinal cord compression injury. J Neuroinflammation 2017;14:97. [Crossref] [PubMed]
- Lee JY, Maeng S, Kang SR, Choi HY, Oh TH, Ju BG, Yune TY. Valproic acid protects motor neuron death by inhibiting oxidative stress and endoplasmic reticulum stress-mediated cytochrome C release after spinal cord injury. J Neurotrauma 2014;31:582-94. [Crossref] [PubMed]
- Lim SH, Dinner DS, Pillay PK, Lüders H, Morris HH, Klem G, Wyllie E, Awad IA. Functional anatomy of the human supplementary sensorimotor area: results of extraoperative electrical stimulation. Electroencephalogr Clin Neurophysiol 1994;91:179-93. [Crossref] [PubMed]
- Zhang Y, Liu H, Wang L, Yang J, Yan R, Zhang J, Sang L, Li P, Wang J, Qiu M. Relationship between functional connectivity and motor function assessment in stroke patients with hemiplegia: a resting-state functional MRI study. Neuroradiology 2016;58:503-11. [Crossref] [PubMed]
- Cona G, Marino G, Semenza C. TMS of supplementary motor area (SMA) facilitates mental rotation performance: Evidence for sequence processing in SMA. Neuroimage 2017;146:770-7. [Crossref] [PubMed]
- Bathla G, Gene MN, Peck KK, Jenabi M, Tabar V, Holodny AI. Resting State Functional Connectivity of the Supplementary Motor Area to Motor and Language Networks in Patients with Brain Tumors. J Neuroimaging 2019;29:521-6. [Crossref] [PubMed]
- Misra UK, Kalita J, Das BK. Single photon emission computed tomography in tuberculous meningitis. Postgrad Med J 2000;76:642-5. [Crossref] [PubMed]
- Fried PJ, Rushmore RJ 3rd, Moss MB, Valero-Cabré A, Pascual-Leone A. Causal evidence supporting functional dissociation of verbal and spatial working memory in the human dorsolateral prefrontal cortex. Eur J Neurosci 2014;39:1973-81. [Crossref] [PubMed]
- Ngetich R, Zhou J, Zhang J, Jin Z, Li L. Assessing the Effects of Continuous Theta Burst Stimulation Over the Dorsolateral Prefrontal Cortex on Human Cognition: A Systematic Review. Front Integr Neurosci 2020;14:35. [Crossref] [PubMed]
- Buckner RL, Andrews-Hanna JR, Schacter DL. The brain's default network: anatomy, function, and relevance to disease. Ann N Y Acad Sci 2008;1124:1-38. [Crossref] [PubMed]
- Raichle ME. The brain's default mode network. Annu Rev Neurosci 2015;38:433-47. [Crossref] [PubMed]
- Cavanna AE, Trimble MR. The precuneus: a review of its functional anatomy and behavioural correlates. Brain 2006;129:564-83. [Crossref] [PubMed]
- Wallentin M, Roepstorff A, Glover R, Burgess N. Parallel memory systems for talking about location and age in precuneus, caudate and Broca's region. Neuroimage 2006;32:1850-64. [Crossref] [PubMed]
- Luo X, Jiaerken Y, Huang P, Xu XJ, Qiu T, Jia Y, Shen Z, Guan X, Zhou J, Zhang MAlzheimer’s Disease Neuroimaging Initiative (ADNI). Alteration of regional homogeneity and white matter hyperintensities in amnestic mild cognitive impairment subtypes are related to cognition and CSF biomarkers. Brain Imaging Behav 2018;12:188-200. [Crossref] [PubMed]
- Cui L, Zhang Z, Zac Lo CY, Guo Q. Local Functional MR Change Pattern and Its Association With Cognitive Function in Objectively-Defined Subtle Cognitive Decline. Front Aging Neurosci 2021;13:684918. [Crossref] [PubMed]
- Wandell BA, Dumoulin SO, Brewer AA. Visual field maps in human cortex. Neuron 2007;56:366-83. [Crossref] [PubMed]
- Chen W, Wu Q, Chen L, Zhou J, Chen HH, Xu XQ, Wu FY, Hu H. Aberrant brain voxel-wise resting state fMRI in patients with thyroid-associated ophthalmopathy. J Neuroimaging 2021;31:773-83. [Crossref] [PubMed]
- Leonard JM. Central Nervous System Tuberculosis. Microbiol Spectr 2017; [Crossref] [PubMed]
- Yin P, Liu Y, Xiong H, Han Y, Sah SK, Zeng C, Wang J, Li Y. Structural abnormalities and altered regional brain activity in multiple sclerosis with simple spinal cord involvement. Br J Radiol 2018;91:20150777. [Crossref] [PubMed]
- Zhao ZL, Fan FM, Lu J, Li HJ, Jia LF, Han Y, Li KC. Changes of gray matter volume and amplitude of low-frequency oscillations in amnestic MCI: An integrative multi-modal MRI study. Acta Radiol 2015;56:614-21. [Crossref] [PubMed]
- Klaus J, Schutter DJLG. Functional topography of anger and aggression in the human cerebellum. Neuroimage 2021;226:117582. [Crossref] [PubMed]
- E KH. Chen SH, Ho MH, Desmond JE. A meta-analysis of cerebellar contributions to higher cognition from PET and fMRI studies. Hum Brain Mapp 2014;35:593-615. [Crossref] [PubMed]
- Thomann PA, Schläfer C, Seidl U, Santos VD, Essig M, Schröder J. The cerebellum in mild cognitive impairment and Alzheimer's disease - a structural MRI study. J Psychiatr Res 2008;42:1198-202. [Crossref] [PubMed]
- Sun Y, Dai Z, Li Y, Sheng C, Li H, Wang X, Chen X, He Y, Han Y. Subjective Cognitive Decline: Mapping Functional and Structural Brain Changes-A Combined Resting-State Functional and Structural MR Imaging Study. Radiology 2016;281:185-92. [Crossref] [PubMed]
- Hoptman MJ, Zuo XN, Butler PD, Javitt DC, D'Angelo D, Mauro CJ, Milham MP. Amplitude of low-frequency oscillations in schizophrenia: a resting state fMRI study. Schizophr Res 2010;117:13-20. [Crossref] [PubMed]
- Liu P, Jia XZ, Chen Y, Yu Y, Zhang K, Lin YJ, Wang BH, Peng GP. Gut microbiota interacts with intrinsic brain activity of patients with amnestic mild cognitive impairment. CNS Neurosci Ther 2021;27:163-73. [Crossref] [PubMed]