Stereotactic co-axial projection imaging for augmented reality neuronavigation: a proof-of-concept study
Introduction
Neuronavigation provides indispensable guidance in modern neurosurgery (1-3). Most of the existing neuronavigation systems are frameless systems equipped with monitors for visualizing intraoperative and preoperative images. Although some of these systems are able to achieve a navigation accuracy of about a couple of millimeters (4,5), their monitor-based interface design suffers multiple drawbacks, such as lack of sufficient intuitiveness and requirement for hand-eye coordination of surgical operators (6).
The emerging technology of augmented reality (AR) provides an intuitive and user-friendly interface for neurosurgical navigation (7-9). The AR-based surgical navigation technique effectively merges virtual images with actual scenes for immersive display of the fused images at the surgical site in real time through a variety of methods, such as semi-transparent mirrors, head-mounted displays, and digital projectors. AR-guided neurosurgery was first demonstrated in 1997, when a semi-transparent mirror was used to superimpose the preoperative magnetic resonance images with the surgeon’s field of view (FOV) for neuronavigation (10). Although the technique has improved with time (11), the presence of the transparent mirror limits the achievable FOV and impedes the surgeon’s intervention. To reduce the device interference in clinical interventions, a variety of wearable AR devices, such as head-mounted binocular glasses (12) and Microsoft HoloLens (13), have been explored recently. These wearable AR devices only provide the immersive display to the person who wears them, and long-term use of these devices may cause nausea, dizziness, or other discomfort, which may eventually affect the clinical intervention (14). Various image-to-patient registration methods in surgical navigation have also been proposed (15-17).
Projection-based surgical navigation has the potential to overcome the existing limitations of AR navigation by direct projection of medical images to the surgical field without any monitor or wearable display (18-22). This technique displays medical information and intraoperative guidance intuitively to the entire surgical team so that clinicians are able to identify target lesions, discuss surgical plans, and carry out interventions without being distracted or switching their sight between the surgical site and the external monitor. Krempien et al. developed a binocular camera and projector system for interstitial brachytherapy (23). Gavaghan et al. developed a portable image overlay system for projecting the planned data during open liver surgery (24). Besharati Tabrizi et al. examined a commercial projector for neurosurgery navigation (25). In these works, before navigation, the projector was registered to the surgical frame of reference by manual adjustment of its position to match those of the fiducial markers.
Despite many encouraging research and development efforts, the field of AR navigation is still in its infant stage with many present drawbacks. For example, the fixed navigation system above the patient makes it difficult to meet all the navigation needs in various surgical scenarios (23). The stability and reliability of handheld projector systems depends on other navigation systems (24). Registration by manually fine-tuning the position of the projector requires substantial time and effort and its accuracy is operator dependent (25). Further, no report is available yet for quantitative assessment of achievable resolution by AR navigation. Therefore, it is still uncertain whether AR navigation can differentiate relevant anatomic structures with sufficient accuracy, typically sub-centimeter, to support appropriate surgical guidance (26). Furthermore, no report is available for performance comparison between an AR navigation device and a monitor-based navigation device. The “parallax error” that will cause navigation error in AR navigation also needs to be solved (27).
To address the above unmet needs in AR navigation, we designed an integrated dexterous stereotactic co-axial projection imaging (sCPI) system and demonstrated its clinical utility in neurosurgery. The sCPI system hardware consists of a camera and a projector co-axially aligned on the same optical path and mounted on a tripod. The system software enabled automated patient tracking and image-to-patient registration. Commonly used surgical navigation systems, such as BrainLab, require multiple “clicks” on the patient’s body surface with a navigation stylet to complete registration. In comparison, image-patient registration for the sCPI system required no navigation stylet but analysis of only a few photographs of markers acquired by the system’s coaxial camera. Our benchtop verification tests showed favorable navigation capabilities with a display resolution of 1.3 mm and a navigation accuracy of 1.5 mm. Our phantom validation tests showed that the sCPI system had an operational accuracy comparable to that of the Kick Navigation Station (BrainLab, Germany), but was more dexterous and required shorter preparation time. Our clinical trial successfully demonstrated the clinical utility of the sCPI system in intraoperative visualization and neurosurgical navigation. To the best of the authors’ knowledge, this is the first clinical study that evaluates the utility of projective real-time AR-based neurosurgical navigation. We present the following article in accordance with the GRRAS reporting checklist (available at https://qims.amegroups.com/article/view/10.21037/qims-21-1144/rc).
Methods
The composition and principle of the sCPI neuronavigation system were introduced, and the sCPI system was quantitatively characterized by benchtop and phantom validation. Moreover, a clinical trial was conducted on a meningioma surgery to verify the clinical feasibility of the sCPI system. The study was conducted in accordance with the Declaration of Helsinki (as revised in 2013). The study was approved by the Ethics Committee of the First Affiliated Hospital of University of Science and Technology of China (approval ID: 2020KY218) and informed consent was taken from all individual participants.
sCPI system
The sCPI system, as shown in Figure 1, was a purpose-designed optical system for surgical navigation based on orthotopic projection, where the camera and projector were aligned at conjugate positions.
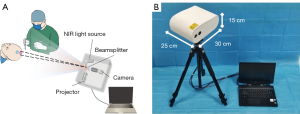
The system consisted of a near infrared (NIR) camera, a projector, a beam splitter, and an 850 nm NIR light source for marker illumination that is invisible to the naked eye, as shown in Figure 1A. The brightness of the projector was 50 lumens. The FOV of the projector was 97 cm × 54 cm at the working distance of 2 m. The pixel density conversion was also required between the camera and the projector. The entire sCPI system had a volume of 30 cm × 25 cm × 15 cm, and could be installed on a tripod-like bracket as shown in Figure 1B.
A laptop was connected with the sCPI system for data processing, and a reference array with fiducial markers installed on a cardan holder was utilized for the sCPI system localization.
sCPI-assisted neuronavigation
In neuronavigation, the steps of using the sCPI system included three-dimensional reconstruction, image-to-patient registration, and intraoperative projection navigation. After the patient underwent a medical imaging scan, 3D models containing brain tumor and incision plans were generated with 3D Slicer (28) and then imported into the sCPI system. After the image-to-patient registration, the sCPI system constantly localized itself relative to the patient by optical tracking, and 2D projections of the 3D model in specific views were generated and projected in situ by the sCPI system. Custom code and OpenCV (29) were also used in this process.
Image-to-patient registration
To project the navigation information to the appropriate location on the patient’s surface, image-to-patient registration was required. Image-patient registration was the process of calculating the transformation matrix P of the patient with respect to the reference frame in the real space. Figure 2 illustrated the coordinate systems and the transformation matrices during the registration and navigation.
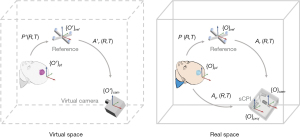
Coordinate systems with and without superscript’ referred to the coordinate system of the same subject in virtual space and in real space, respectively. The process of registration first calculated the transformation matrix of the patient Ap, and the transformation matrix the reference frame Ar with respect to the sCPI.
represents the homogeneous coordinates in the camera’s image coordinate system {O}cam, where u and v are horizontal and vertical coordinate, respectively. are the homogeneous coordinates in the reference or patient coordinate systems ({O}ref and {O}pt). Qpt is known from the reconstructed 3D model, and Qref is known from the design of the reference frame. M is the camera intrinsic parameter matrix, which is calculated by camera calibration and only depended on the camera hardware. A=[R,T] is the coordinate transformation matrix, where R represents coordinate rotation and T represents coordinate translation. M, Q were known prior to registration. After q was extracted from images captured by the sCPI, A was solved iteratively with the Levenberg-Marquardt method (30). Finally, we got the patient-to-reference transformation matrix P:
After calculating P, the image-to-patient registration was completed by setting the position of the patient in the virtual space, i.e., P'=P.
sCPI navigation
After the image-to-patient registration, the sCPI would be moved to the appropriate position in order to eliminate the effect of parallax error. When moving the sCPI system, the reference frame of known size was localized in real time by the calibrated camera, i.e., Aref in Eq. [1] was solved in real time. In the virtual space, Aref' and Aref were kept equal, which meant that the positions of the virtual camera and the sCPI were always the same. Virtual images of the surgical targets at the current view angle and distance, which was conjugate to the patient surface, could be generated on the virtual camera sensor plane. Real-time projected surgical navigation was achieved by projecting the targets’ images taken by the virtual camera in the virtual space onto the patient’s surface in the real space by the sCPI’s projector. Thanks to the coaxial optics design of the sCPI system, {O}proj was equivalent to {O}cam, which meant that additional camera-projector registration was not required. Immediately after the registration, patterns of targets were projected to the patient’s surface, where surgical targets and planned incision could be viewed intuitively.
Parallax error
The projection AR neurosurgery navigation system had the problem of parallax error. If the tumor was deep in the brain, the projected pattern of the tumor on the body surface would move with movement of the projector, even though the projected pattern always pointed to the tumor. So the true position of the tumor could not be judged by the projected pattern on the body surface, which could cause ambiguity for surgical guidance. To eliminate parallax error, a procedure was adopted whereby the sCPI host was moved until the projected target and the projected incision coincided, as shown in the Figure 3. The center of the incision and the tumor model could be projected to assist in this coincidence process.
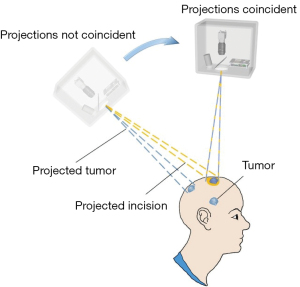
After the parallax correction, the position of the sCPI was fixed during surgical navigation and always provides projected optical navigation. The obstruction of markers would not affect the accuracy of surgical navigation. The surgeon could excavate according to the position prompted by the projection until the tumor was exposed, since the projection light path and the surgical path were coincident.
Performance characterization
AR display resolution, the coaxial degree of the sCPI’s camera and projector and stereotactic guidance accuracy of the sCPI system were tested to verify its capability in neurosurgery navigation.
The sCPI’s minimum projected line width, which was the width of the finest structure that a projector could project, was measured at the working distance of 2 m. The USAF1951 resolution test image was projected on a white board ensuring that the width of largest square was 10 mm, as shown in the Figure 4A. The projected pattern was captured by a camera (resolution of 6,000×4,000) adjacent to the sCPI host, and analyzed using ImageJ (31) by plotting the variation of intensity value of the finest unambiguous element, as shown in the Figure 4B.
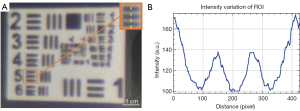
To measure the coaxial degree of the sCPI, we mounted another high-resolution digital camera next to the system. We used the sCPI’s camera to capture a chessboard, and then used the sCPI’s projector to project the captured image onto a whiteboard at the original location. The other camera was used to capture the placed chessboard and the projected checkerboard grid separately. The coaxial degree of the sCPI system was then obtained by comparing the captured images after the perspective correction and converting the pixel units to millimeter units. The experiment was repeated three times at working distances of 1.5, 2, and 2.5 m, respectively.
In order to achieve stereo positioning and projection, it was necessary to calibrate the camera and the projector of the sCPI system. The camera was calibrated by Zhang’s method (32) with MATLAB (MathWorks. Inc., US). The complex projector calibration was simplified by directly using the camera’s intrinsic parameters thanks to the co-axial settings of the camera and projector.
To verify the stereotactic navigation accuracy of the sCPI system, a circle array pattern as the target was projected to the back of a calibration panel, as shown in the Figure 5A.
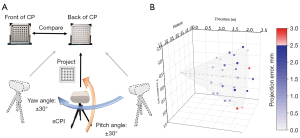
The printed circular array pattern on the front of the calibration panel was the ground truth, and the panel could be turned over for unambiguous recording of the projected circular array pattern as the test group. After registration on the z axis, the sCPI system was moved within a distance range of 1.5 to 2.5 m, and a pitch and yaw angle of 30 degrees relative to the calibration panel. A fixed-position camera was used to record the images of the two groups. The test process was repeated three times, and then centers of circles in the images recorded were extracted by MATLAB after image rectification. By calculating the circles’ mean deviation between the two groups, we obtained the navigation error of the sCPI system under various working distance and orientation.
Benchtop validation
The sCPI system was compared with the BrainLab Kick Navigation Station on a 3D printed skull phantom. To simulate different surgical targets at different possible positions during the surgery, 8 targets distributed in various parts of the cranium were designed. The experiment was completed in a neurosurgery operating room, after the phantom was immobilized via a DORO head holder and immobilized during the entire experiment.
After the registration of sCPI, we measured the distance between each target projected by the sCPI system and the corresponding intracranial target center using a millimeter ruler as the navigation error of the sCPI system. By placing the tip of the navigation stylet at the center of the intracranial target after the Kick Navigation Station’s registration, the navigation error (in pixel units) of the Kick Navigation Station could be derived by analyzing the distance between the tip of the stylet and the center of the intracranial target on the screen. The pixel-to-distance conversion was done by using a standard cube with 5 cm dimension within the phantom.
The preparation time for the sCPI system was compared with that of the Kick Navigation Station. For both systems, the time recording started when the power-on button was pressed and ended when the registration was done and the navigation information was displayed. The registration of Kick Navigation Station required multiple clicks on the patient’s body surface with a navigation stylet. The sCPI system’s registration required no navigation stylet but analysis of only a few photographs of markers. The time of parallax correction for the sCPI system was also included.
The above tests were repeated three times on both the sCPI system and the Kick Navigation Station and the operations were performed by the same neurosurgeon in order to eliminate operator-induced variations.
Clinical trial
To verify the clinical feasibility of the sCPI system for neurosurgery navigation, we conducted a pilot clinical trial with a single subject. The study was approved by The Ethics Committee of the First Affiliated Hospital of University of Science and Technology of China (approval ID: 2020KY218) and informed consent was taken from all individual participants. The inclusion criteria for the study were patients with a brain tumor requiring surgical resection, whose physical condition met the experimental requirements, and who had not previously undergone brain surgery. Among suitable candidates, we randomly selected a 56-year-old man with meningioma, who underwent a contrast-enhanced, T1-weighted MRI scan (GE Medical System, Discovery MR750w), and whose images were segmented and reconstructed. The patient underwent tumor resection operation under the guidance of the sCPI system. Before the surgery, the sCPI system and the reference array were sterilized to satisfy operation requirements.
Results
Performance characteristics
The finest clear element of projected USAF1951 pattern was element 5 of group 2, as shown in the Figure 4, which meant the minimum projected line width of the sCPI system was 1.3 mm. This implied that the sCPI system was sufficient to characterize sub-centimeter structure in clinical images. For camera calibration, the mean re-projection error was 0.1331 pixels considering tangential distortion, skew, and the second order of radial distortion coefficients. The average coaxial errors were 0.4±0.1, 0.5±0.2, 0.7±0.3 mm at working distances of 1.5, 2, and 2.5 m, respectively. In order to illustrate the error distribution of different navigation positions, a 3D scatter plot was drawn as shown in Figure 5B.
The maximum and minimum error of the sCPI system were 2.9 and 0.3 mm when the sCPI system was located at distances of 2.5 and 1.5 m, respectively, and the mean error was 1.5 mm. It can be seen from the scatter plot that when the angle between the optical axis of the sCPI system and the Z axis was 0, the average error was smaller than at larger angles (1.1±0.7 mm at 0° and 1.6±1.3 mm at angle of 30°). This indicated that the navigation error of the sCPI system would increase with the pitch and yaw angle. After our analysis, there was no obvious difference in navigation errors caused by different yaw directions, which meant that the navigation error is unbiased across all directions.
Benchtop validation
The sCPI system and the Kick Navigation Station were conducted for phantom navigation experiments at the same time, as shown in Figure 6.
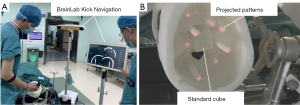
The average preparation time of the sCPI system and the Kick Navigation Station were 3 min 24 sec and 6 min 8 sec respectively. Box plot (Figure 7) of navigation errors showed that the errors of both navigation systems were within 3 mm.
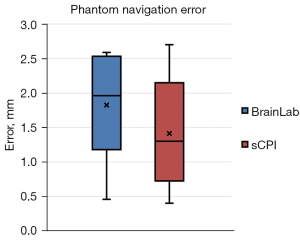
The average errors of the sCPI system and the Kick Navigation Station were 1.4±0.8 and 1.8±0.7 mm, and the medians were 1.3 and 1.9 mm, respectively. It could be seen that the average and median errors of the sCPI system were smaller than those of the Kick Navigation Station system, however these differences were not statistically significant (P>0.05). Taken together, compared with the Kick Navigation Station, the sCPI system had the similar navigation accuracy with shorter preparation time.
Clinical trial
The MRI image (Figure 8A) showed a tumor measuring 1.7 cm × 1.0 cm on the top left side of the brain. The Figure 8B showed that the reconstructed target was projected on the dura mater for surgery guidance.
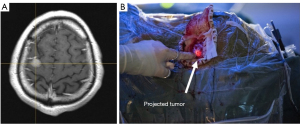
The operation lasted for 3 h, and the time taken to prepare the sCPI system was 4 min. The interference of the sCPI system to the surgery was quite limited since no manual operation was required for registration, and there was no need to replace the scalpel with a navigation stylet for intraoperative navigation. The operation went smoothly, and a total of about 2.5 cm × 2.5 cm of tissue was removed, with about 100 mL bleeding during the operation and no blood transfusion. Pathological results showed that the tumor was a transitional meningioma of WHO grade I.
Discussion
AR neuronavigation system
The sCPI system has a portable and stable body consisting of a camera and projector, providing practical, flexible, and affordable projection-based AR surgery navigation equipment. Since the camera and projector are co-axial, neither extra projector calibration nor camera-projector coordinate transformation are required. The sCPI system is also low-cost, as the key components of the sCPI system include a NIR camera, a projector, a NIR light source and a laptop, with the total cost equaling about 4,000 US dollars. The color projected by the projector can be adjusted in order to achieve the best visualization contrast in different clinical scenarios. We can further optimize the sCPI hardware by introducing a brighter laser projector and selecting a better matched camera and projector to reduce performance loss in pixel density conversion. In the clinical trial, the sCPI system has successfully assisted clinicians in localizing tumors during surgery, indicating its potential clinical value that can be further validated by larger scale clinical trials.
As mentioned above, projected AR navigation has a problem of parallax error. The position of the projected pattern is determined by the intersection of the body surface and the connecting line between the projector and the target. Therefore, when the targets are deep in the brain, the projected pattern is more likely to be affected by the movement of the projector, while the targets closer to the body surface will be less affected. Here we minimize the impact of the parallax error by adding the step of moving the sCPI system until the projected tumor coincides with the projected incision. After the sCPI system is placed in the correct position, unambiguous guidance is provided for the surgery. Currently, the parallax correction process is carried out manually by the operator. In the future, this process can be operated automatically by mounting the sCPI system on a robotic arm.
Navigation accuracy
In terms of the navigation error, our benchtop experiment showed no significant difference between the sCPI system and the Kick Navigation Station. However, the sCPI system required less time for preparation, was relatively smaller, and could be easily carried or even integrated into a robotic arm for automatic navigation. Since the navigation information was projected on the patient without need for screen display, a battery-powered sCPI system can be potentially deployed in low resource settings where electrical power is unavailable.
The classification of neurosurgery navigation error was discussed in detail by Wang (33). Errors were divided into two categories, the first was caused by the anatomic structures’ differences between the image space and the patient space, and the second was caused by transformation errors between patient space and image space during the surgery. The second type of error was largely influenced by the navigation system. For the sCPI system, the camera resolution and camera calibration accuracy were key factors that affect the navigation error.
The resolution of the current camera is 1,280×1,024, and it can be expected that a higher-resolution camera has a better ability to distinguish markers and can obtain a higher navigation accuracy. The accuracy of camera calibration is affected by the distortion of the camera lens, and distortion largely depends on the focal length of the lens. A telephoto lens means smaller distortion (higher calibration accuracy) but a smaller FOV, and vice versa for a wide-angle lens. In order to balance the competing requirements between lens calibration and FOV, the sCPI system uses a 12-mm focal length lens. To meet different surgical navigation scenarios, a lens with higher calibration accuracy or a larger FOV can be selected.
Further study
In the current work, we used a single clinical case to demonstrate the clinical utility of an integrated dexterous sCPI system for AR neurosurgical navigation. In this proof-of-concept study, the clinical performances of the sCPI system and the Kick Navigation Station were not compared objectively due to many sources of variation such as brain shift (34) during the surgery. In the future, further benchtop validations and larger scale clinical trials are needed in order to collect sufficient data for quantitative validation of the sCPI system’s clinical utility. In this work, instead of meticulously quantifying registration and projection errors separately, we characterized the overall navigation error, which could better demonstrate the performance of the system in real scenarios. In future work, individual assessment and analysis of each error will be carried out. The information displayed by the sCPI is limited to the preoperative MRI or CT data, but the future of neuronavigation lies in multi-modal image information fusion (35,36), since preoperative data can’t compensate for brain shift during the operation, which will cause non-negligible navigation error. The co-axial designed system has great potential to be combined with intraoperative fluorescence imaging (37), and this multimodal AR neuronavigation is a direction for our future work.
In this study we presented an integrated dexterous sCPI system for AR neurosurgical navigation. The system featured automatic registration and multi-angle real-time orthotopic image projection, and reduced the problem of parallax error during the AR image-guided surgery. Our benchtop experiment showed that the average navigation errors of the sCPI system and Kick Navigation Station were 1.4±0.8 and 1.8±0.7 mm, and the average preparation times were 3 min 24 s and 6 min 8 s, respectively. Our Clinical study demonstrated the technical feasibility of the sCPI system in surgical navigation and points to its clinical potential for intraoperative visualization. The sCPI technique can be potentially used in many surgical applications for intuitive visualization of medical information and intraoperative guidance of surgical trajectories.
Acknowledgments
We gratefully thank Prof. Zachary J. Smith (Department of Precision Machinery and Precision Instrumentation, University of Science and Technology of China) for helping with the English language editing. We gratefully thank the reviewers for their constructive comments.
Funding: This work was supported by the Fundamental Research Funds for the Central Universities, China (Grant No. WK20900000254), and the Strategic Priority Research Program of the Chinese Academy of Sciences (Grant No. XDA16021303).
Footnote
Reporting Checklist: The authors have completed the GRRAS reporting checklist. Available at https://qims.amegroups.com/article/view/10.21037/qims-21-1144/rc
Conflicts of Interest: All authors have completed the ICMJE uniform disclosure form (available at https://qims.amegroups.com/article/view/10.21037/qims-21-1144/coif). The authors have no conflicts of interest to declare.
Ethical Statement: The authors are accountable for all aspects of the work in ensuring that questions related to the accuracy or integrity of any part of the work are appropriately investigated and resolved. The study was conducted in accordance with the Declaration of Helsinki (as revised in 2013). The study was approved by the Ethics Committee of the First Affiliated Hospital of University of Science and Technology of China (approval ID: 2020KY218) and informed consent was taken from all individual participants.
Open Access Statement: This is an Open Access article distributed in accordance with the Creative Commons Attribution-NonCommercial-NoDerivs 4.0 International License (CC BY-NC-ND 4.0), which permits the non-commercial replication and distribution of the article with the strict proviso that no changes or edits are made and the original work is properly cited (including links to both the formal publication through the relevant DOI and the license). See: https://creativecommons.org/licenses/by-nc-nd/4.0/.
References
- Mezger U, Jendrewski C, Bartels M. Navigation in surgery. Langenbecks Arch Surg 2013;398:501-14. [Crossref] [PubMed]
- Tasserie J, Lozano AM. Editorial. 7T MRI for neuronavigation: toward better visualization during functional surgery. J Neurosurg 2022; Epub ahead of print. [Crossref] [PubMed]
- Gutmann S, Tästensen C, Böttcher IC, Dietzel J, Loderstedt S, Kohl S, Matiasek K, Flegel T. Clinical use of a new frameless optical neuronavigation system for brain biopsies: 10 cases (2013-2020). J Small Anim Pract 2022; Epub ahead of print. [Crossref] [PubMed]
- Paraskevopoulos D, Unterberg A, Metzner R, Dreyhaupt J, Eggers G, Wirtz CR. Comparative study of application accuracy of two frameless neuronavigation systems: experimental error assessment quantifying registration methods and clinically influencing factors. Neurosurg Rev 2010;34:217-28. [Crossref] [PubMed]
- Rusheen AE, Goyal A, Owen RL, Berning EM, Bothun DT, Giblon RE, Blaha CD, Welker KM, Huston J, Bennet KE, Oh Y, Fagan AJ, Lee KH. The development of ultra-high field MRI guidance technology for neuronavigation. J Neurosurg 2022; Epub ahead of print. [Crossref] [PubMed]
- Kockro RA, Tsai YT, Ng I, Hwang P, Zhu C, Agusanto K, Hong LX, Serra L. Dex-ray: augmented reality neurosurgical navigation with a handheld video probe. Neurosurgery 2009;65:795-807; discussion 807-8. [Crossref] [PubMed]
- Meola A, Cutolo F, Carbone M, Cagnazzo F, Ferrari M, Ferrari V. Augmented reality in neurosurgery: a systematic review. Neurosurg Rev 2017;40:537-48. [Crossref] [PubMed]
- Liu P, Li C, Xiao C, Zhang Z, Ma J, Gao J, Shao P, Valerio I, Pawlik TM, Ding C, Yilmaz A, Xu R. A Wearable Augmented Reality Navigation System for Surgical Telementoring Based on Microsoft HoloLens. Ann Biomed Eng 2021;49:287-98. [Crossref] [PubMed]
- Cote DJ, Ruzevick J, Strickland BA, Zada G. Commentary: Development of a New Image-Guided Neuronavigation System: Mixed-Reality Projection Mapping Is Accurate and Feasible. Oper Neurosurg (Hagerstown) 2022;22:e100. [Crossref] [PubMed]
- Iseki H, Masutani Y, Iwahara M, Tanikawa T, Muragaki Y, Taira T, Dohi T, Takakura K. Volumegraph (overlaid three-dimensional image-guided navigation) - clinical application of augmented reality in neurosurgery. Stereotact Funct Neurosurg 1997;68:18-24. [Crossref] [PubMed]
- Liao H, Inomata T, Sakuma I, Dohi T. 3-D augmented reality for MRI-guided surgery using integral videography autostereoscopic image overlay. IEEE Trans Biomed Eng 2010;57:1476-86. [Crossref] [PubMed]
- Birkfellner W, Figl M, Huber K, Watzinger F, Wanschitz F, Hummel J, Hanel R, Greimel W, Homolka P, Ewers R, Bergmann H. A head-mounted operating binocular for augmented reality visualization in medicine - design and initial evaluation. IEEE Trans Med Imaging 2002;21:991-7. [Crossref] [PubMed]
- Incekara F, Smits M, Dirven C, Vincent A. Clinical Feasibility of a Wearable Mixed-Reality Device in Neurosurgery. World Neurosurg 2018;118:e422-7. [Crossref] [PubMed]
- Kolodzey L, Grantcharov PD, Rivas H, Schijven MP, Grantcharov TP. Wearable technology in the operating room: a systematic review. BMJ Innov 2017;3:55-63. [Crossref]
- Ma L, Zhao Z, Chen F, Zhang B, Fu L, Liao H. Augmented reality surgical navigation with ultrasound-assisted registration for pedicle screw placement: a pilot study. Int J Comput Assist Radiol Surg 2017;12:2205-15. [Crossref] [PubMed]
- Chen ECS, Ma B, Peters TM. Contact-less stylus for surgical navigation: registration without digitization. Int J Comput Assist Radiol Surg 2017;12:1231-41. [Crossref] [PubMed]
- Suenaga H, Tran HH, Liao H, Masamune K, Dohi T, Hoshi K, Takato T. Vision-based markerless registration using stereo vision and an augmented reality surgical navigation system: a pilot study. BMC Med Imaging 2015;15:51. [Crossref] [PubMed]
- Zhang F, Zhu X, Gao J, Wu B, Liu P, Shao P, Xu M, Pawlik TM, Martin EW, Xu RX. Coaxial projective imaging system for surgical navigation and telementoring. J Biomed Opt 2019;24:1-9. [Crossref] [PubMed]
- Chan HHL, Haerle SK, Daly MJ, Zheng J, Philp L, Ferrari M, Douglas CM, Irish JC. An integrated augmented reality surgical navigation platform using multi-modality imaging for guidance. PLoS One 2021;16:e0250558. [Crossref] [PubMed]
- Edgcumbe P, Singla R, Pratt P, Schneider C, Nguan C, Rohling R. Follow the light: projector-based augmented reality intracorporeal system for laparoscopic surgery. J Med Imaging (Bellingham) 2018;5:021216. [Crossref] [PubMed]
- Wen R, Nguyen BP, Chng CB, Chui CK. In situ spatial AR surgical planning using projector-Kinect system. In: Proceedings of the Fourth Symposium on Information and Communication Technology 2013:164-71.
- Edgcumbe P, Pratt P, Yang GZ, Nguan C, Rohling R. Pico Lantern: a pick-up projector for augmented reality in laparoscopic surgery. Med Image Comput Comput Assist Interv 2014;17:432-9.
- Krempien R, Hoppe H, Kahrs L, Daeuber S, Schorr O, Eggers G, Bischof M, Munter MW, Debus J, Harms W. Projector-based augmented reality for intuitive intraoperative guidance in image-guided 3D interstitial brachytherapy. Int J Radiat Oncol Biol Phys 2008;70:944-52. [Crossref] [PubMed]
- Gavaghan KA, Peterhans M, Oliveira-Santos T, Weber S. A portable image overlay projection device for computer-aided open liver surgery. IEEE Trans Biomed Eng 2011;58:1855-64. [Crossref] [PubMed]
- Besharati Tabrizi L, Mahvash M. Augmented reality-guided neurosurgery: accuracy and intraoperative application of an image projection technique. J Neurosurg 2015;123:206-11. [Crossref] [PubMed]
- Reeth EV, Tham IWK, Tan CH, Poh CL. Super-resolution in magnetic resonance imaging: a review. Concept Magn Reson A 2012;40:306-25. [Crossref]
- Ferrari V, Cutolo F. Letter to the Editor: Augmented reality-guided neurosurgery. J Neurosurg 2016;125:235-7.
- Kikinis R, Pieper SD, Vosburgh KG. 3d slicer: a platform for subject-specific image analysis, visualization, and clinical support. In New York, NY: Springer New York; 2013:277-89.
- Bradski G. The OpenCV Library. Dr Dobb’s Journal of Software Tools 2000;
- Madsen K, Nielsen HB, Tingleff O. Methods for non-linear least squares problems (2nd ed). 2004.
- Schindelin J, Arganda-Carreras I, Frise E, Kaynig V, Longair M, Pietzsch T, Preibisch S, Rueden C, Saalfeld S, Schmid B, Tinevez JY, White DJ, Hartenstein V, Eliceiri K, Tomancak P, Cardona A. Fiji: an open-source platform for biological-image analysis. Nat Methods 2012;9:676-82. [Crossref] [PubMed]
- Zhang Z. A flexible new technique for camera calibration. IEEE Trans Pattern Anal Mach Intell 2000;22:1330-4. [Crossref]
- Wang MN, Song ZJ. Classification and analysis of the errors in neuronavigation. Neurosurgery 2011;68:1131-43; discussion 1143. [Crossref] [PubMed]
- Roberts DW, Hartov A, Kennedy FE, Miga MI, Paulsen KD. Intraoperative brain shift and deformation: a quantitative analysis of cortical displacement in 28 cases. Neurosurgery 1998;43:749-58; discussion 758-60. [Crossref] [PubMed]
- Catapano G, Sgulò F, Laleva L, Columbano L, Dallan I, de Notaris M. Multimodal use of indocyanine green endoscopy in neurosurgery: a single-center experience and review of the literature. Neurosurg Rev 2018;41:985-98. [Crossref] [PubMed]
- Cho SS, Ramayya AG, Teng CW, Brem S, Singhal S, Lee JYK. Intraoperative Near-Infrared Fluorescence Imaging With Second-Window Indocyanine-Green Offers Accurate and Real-Time Correction for Brain Shift and Anatomic Inconsistencies in Framless Neuronavigation. Neurosurgery 2019;66:310-460. [Crossref]
- Liu P, Shao P, Ma J, Xu M, Li C. A co-axial projection surgical navigation system for breast cancer sentinel lymph node mapping: system design and clinical trial. In: Proceedings Volume 10868, Advanced Biomedical and Clinical Diagnostic and Surgical Guidance Systems XVII; 108680N (2019). Available online:
10.1117/12.2509852 .10.1117/12.2509852