A simplified scoring protocol to improve diagnostic accuracy with the breast imaging reporting and data system in breast magnetic resonance imaging
Introduction
Breast magnetic resonance imaging (MRI) is an indispensable modality in the detection of breast tumors (1). Current applications of breast MRI include the staging of known cancers (2-5), contralateral breast examination (6-8), breast cancer screening for women at high risk (9,10), treatment evaluation after neoadjuvant chemotherapy (11), and the evaluation of carcinomas of unknown primary origin (12,13). In 2003, the first edition of the American College of Radiology (ACR) breast imaging reporting and data system (BI-RADS) was published, which provided a structured common language for and standardized the reporting of breast MRI (14). The use of a standardized terminology facilitates communication among physicians and radiologists. The morphologic and functional contrast kinetic features of lesions should therefore be described using the BI-RADS lexicon. However, while breast MRI has high sensitivity, ranging from 94% to 99%, it has relatively low specificity for considering every enhancement as suspicious results in unnecessary biopsies (15,16).
When assessing the likelihood of malignancy on breast MRI in practice, radiologists should integrate multiple complex imaging features and combine patient parameters. However, although both morphological and kinetic lesion features should be considered when describing the findings of breast MRI, the relative importance of these features in predicting malignancy remains controversial (17-19). Years of clinical experience and observation show that junior radiologists and breast surgeons often confuse the methods and rules for converting specific imaging features into diagnostic BI-RADS categories (14). As a result, junior radiologists and breast surgeons often have difficulty reaching a consensus on the BI-RADS category with their senior counterparts. The use of multiple diagnostic criteria is associated with low reading consistency among radiologists (20). As a result, the inter-reader consistency of BI-RADS can be low, and its diagnostic accuracy is highly variable (21-23).
Multiparametric breast MRI protocols have been successfully applied in clinical routine. Dynamic evaluation shows the permeability of the vessels and abnormal vasculature of lesions through the shape of the time-intensity curve (TIC). In benign lesions, persistent increase is commonly observed, whereas a decrease in the late phase is common in malignant lesions. Diffusion-weighted imaging (DWI) is influenced by tissue microstructure and cell density, and quantifies the random movement of water molecules by measuring the apparent diffusion coefficient (ADC). Due to high cellular density, compression of extracellular space, and microstructural changes, malignancies tend to show more restricted water molecule diffusivity with a high signal on DWI and low ADC values (15,24,25).
The purpose of this study was to explore the use of a simplified scoring protocol that integrates the morphologic features (MF), TIC, and ADC values with equal weights to help convert specific imaging features into a diagnostic BI-RADS category and to evaluate the efficacy of this protocol by using histopathology results. We present the following article in accordance with following the STARD reporting checklist (available at https://qims.amegroups.com/article/view/10.21037/qims-21-1036/rc).
Methods
Study design
This cross-sectional, retrospective, single-center study was approved by the Ethics Committee of Chinese People’s Liberation Army General Hospital (No. S2019-093-01), and the requirement for individual consent was waived due to the retrospective nature of the analysis. The study was conducted in accordance with the Declaration of Helsinki (as revised in 2013).
Patients
Breast MRI examinations were performed in a routine clinical environment between January 1, 2017, and June 30, 2020. To be included in the study, patients needed to have undergone histopathology either by core biopsy or open surgery as a reference standard after MRI. The exclusion criteria were: (I) patients with a history of biopsy or surgical intervention in the 3 months prior to undergoing MRI examination; and (II) patients whose lesion locations exhibited an evident mismatch between their MRI and surgical or biopsy results. Figure 1 presents the study enrollment flow chart. All patients were also subject to a breast ultrasound and/or digital mammography, either before or after MRI examination. The final surgical treatment, which was either core needle biopsy, vacuum-assisted biopsy, or open surgical biopsy, was decided by the surgeons based on all available information.
Image acquisition
MRI examinations were performed using a 3.0 Tesla MRI scanner with an eight-channel phase array breast coil (Discovery 750, General Electric Healthcare, Milwaukee, WI, USA). The imaging protocol, which lasted for 18 minutes, included four pulse sequences: DWI, T2-weighted imaging (T2WI), T1-weighted imaging (T1WI), and dynamic contrast enhancement (DCE). All sequences were spatially matched in the axial view, and the field of view was 320, 320, and 190 mm in the Z-axis coverage. The b-value of DWI was 0 and 1,000 s/mm2 in three orthogonal diffusion gradients, inversion recovery (IR) was 250 ms for fat suppression, time of repetition (TR) was 5,400 ms, minimum time of echo (TE), and the matrix was 128×128. The T2WI used iterative decomposition of water and fat with echo asymmetry and least-squares estimation (IDEAL) for fat suppression, TR of 5,000 ms, TE of 68 ms, and a matrix of 320×256. For both T1WI and DCE, the same Volume Imaging for Breast Assessment (VIBRANT), spectral-selective inversion recovery (SPECIAL), and three-dimensional spoiled gradient recall sequence were used. The SPECIAL option was disabled for non-fat-suppression T1WI. The T1WI and DCE images had the exact same geometric location, an isotropic spatial resolution of 1.0 mm × 1.0 mm × 1.0 mm, 192 partitions in the axial view, minimum TR/TE, and a flip angle of 120°. The DCE scan repeated six continuous phases without interruption, each of which lasted for 120 s. After the completion of the pre-contrast phase, contrast agent (0.5 M Gd-DTPA) was injected through an antecubital vein at a rate of 2 mL/s, 0.1 mmol/kg body weight, and with 20 mL saline flushing.
Imaging evaluation and the scoring system for BI-RADS categorization
Image processing was carried out on a GE Advantage Windows workstation. All images were studied independently by two attending radiologists, who analyzed their T1 and T2 signal, DWI-ADC, DCE, and kinetics features, and assessed the lesions according to the BI-RADS (14). The MF, TIC, and background parenchymal enhancement (BPE) readings complied with the ACR BI-RADS guidelines. Both radiologists needed to reach a consensus on the subjective MF reading. The first enhanced phase was selected for sagittal and coronal reformation (Figure 2). The early enhancement ratio (EER) was defined as the percentage between the first enhanced phase and the pre-contrast phases. To avoid undesirable issues related to partial volume effects, the TIC curves and ADC values were taken on the detected target lesions. For large and inhomogeneous lesions for which there were multiple regions of interest, only the maximum TIC and minimum ADC were kept for measurement and assessment (Figure 3).
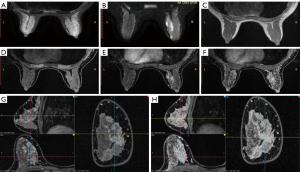
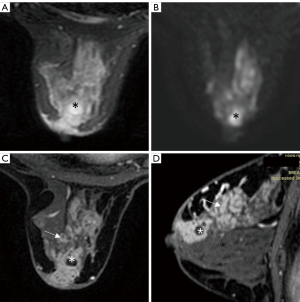
The scoring system comprised three suspicious predictors: (I) MF; (II) TIC profile; and (III) ADC value for mass and non-mass enhancement types. As shown in Table 1, each suspicious predictor was assigned a score of +1. There were also five modifiers introduced for the specific cases. Finally, lesions were categorized based on the sum of the scores as follows, where the category refers to the BI-RADS classification:
Table 1
Score | Mass | NME |
---|---|---|
MF =+1 | Any 1 of the 3 descriptors: | Any 1 of the 2 descriptors: |
(I) Spiculate or irregular margin | (I) Ductal or segmental distribution | |
(II) Irregular shape | (II) Clumped or cluster ring pattern | |
(III) Rim or heterogenous pattern | ||
TIC =+1 | Washout | Washout or plateau |
ADC =+1 | ≤1.05×10−3/cm2/s | ≤1.35×10−3/cm2/s |
Modifier =+1 | High ADC (>2.0×10−3/cm2/s,) of the enhancement lesions | |
Modifier =−1 | (I) EER <120% at first enhanced phase | |
(II) High signal intensity as inflow vessels or cyst on T2WI | ||
(III) Lower ADC at the center of rim enhancement | ||
(IV) Dark septum enhancement (unenhanced septum) |
NME, non-mass-enhancement; MF, morphological feature; TIC, time-intensity curve; ADC, apparent diffusion coefficient; EER, early enhancement ratio; T2WI, T2-weighted imaging.
- Category 5: the sum of scores was +2 or +3.
- Category 4: the sum of scores was +1.
- Category 3: the sum of scores was 0. Focus, scattered foci, or asymmetric maximum BPE included.
- Category 2: specific to benign lesions such as cysts, non-enhanced adenoma, fat necrosis, hamartoma, non-enhanced ductal dilation, and symmetric maximum BPE.
- Category 1: normal breast tissue, including symmetric minimum to moderate BPE.
Histopathology review
A multidisciplinary panel composed of radiologists, surgeons, and pathologists was formed to review the imaging, surgical, and histopathological information. The lesions were classified either as benign, risk, or malignant (Table 2). The risk lesions included atypical ductal hyperplasia, in situ lobular neoplasia [atypical lobular hyperplasia (ALH) and lobular carcinoma in situ (LCIS)], papillary lesions, radial scars/complex sclerosing lesions (RS/CSL), and myoepithelial tumors (phyllodes). These lesions had uncertain malignant potential and were classified as B3 according to the European and British guidelines (26-28). If there was more than one component inside a lesion, only the most suspicious component was recorded.
Table 2
Pathology | BI-RADS category | Total | ||||
---|---|---|---|---|---|---|
1 | 2 | 3 | 4 | 5 | ||
Benign | ||||||
Cyst | 0 | 3 | 0 | 0 | 0 | 3 |
Adenosis | 6 | 34 | 52 | 12 | 13 | 117 |
Fibroadenoma | 0 | 25 | 108 | 19 | 6 | 158 |
Mastitis abscess | 0 | 0 | 4 | 10 | 15 | 29 |
UDH | 2 | 6 | 5 | 1 | 1 | 15 |
Risk | ||||||
ADH | 0 | 1 | 2 | 2 | 8 | 13 |
Papillary lesions | 0 | 11 | 18 | 16 | 42 | 87 |
Phyllodes tumors | 0 | 0 | 4 | 0 | 0 | 4 |
Malignant | ||||||
IDC | 2 | 0 | 2 | 3 | 367 | 374 |
ILC | 0 | 0 | 0 | 0 | 2 | 2 |
MC | 0 | 0 | 0 | 0 | 19 | 19 |
DCIS | 1 | 0 | 0 | 3 | 73 | 77 |
Total | 11 | 80 | 195 | 66 | 546 | 898 |
MRI, magnetic resonance imaging; BI-RADS, breast imaging reporting and data systems; UDH, usual ductal hyperplasia; ADH, atypical ductal hyperplasia; IDC, invasive ductal carcinoma; ILC, invasive lobular carcinoma; MC, mucinous carcinoma; DCIS, ductal carcinoma in situ.
Statistical analysis
Statistical processing was employed at the lesion-based level. To calibrate lesions with a traditional malignant-to-benign differentiation system, the risk lesions were grouped with other lesions that were either benign or malignant in pathology, while the category 4 lesions were grouped with either category 5 or category ≤3 lesions in the final assessment. To assess the proposed scoring system, the receiver operating characteristic (ROC) curve was plotted, and the area under curve (AUC), sensitivity, and specificity for malignant-benign differentiation were calculated using IBM SPSS Statistics 19.
Results
Lesion characteristics
This retrospective study included 879 female patients (46.02±10.60 years; age range, 13 to 84) with 898 MRI and pathologically confirmed lesions. Table 2 lists the BI-RADS categories of the 898 lesions included in this study. Pathologically, 472 of the 898 lesions were malignant, including not otherwise specified invasive and non-invasive breast carcinoma, 104 were risk, and 322 were benign. There were 66 category 4 lesions, of which 27.3% (18/66) were classified as pathological risk, 9.1% (6/66) were malignant, and 63.6% (42/66) were benign. Among the 104 pathologically borderline risk lesions, 48.1% (50/104), 17.3% (18/104), and 23.1% (24/104) were classified as category 5, 4, and 3, respectively, and the remaining 11.5% (12/104) were in category 1 or 2.
The modifiers, which are introduced in Table 1, can increase the diagnostic accuracy for specific diseases. In our study, without any modifiers, 22 non-puerperal mastitis cases and 68 fibroadenomas were overestimated as category 4 and category 4 or 5, respectively, while 4 mucinous carcinomas were underestimated as category 3. When the modifiers were introduced, 14 out of 29 non-puerperal mastitis cases, 68 out of 158 fibroadenomas, and 4 out of 19 mucinous carcinomas were predicted accurately in the final categorization. Non-puerperal mastitis could be easily misclassified as category 4 or 5 but could be corrected if abscess formation was observed (Figure 3), while other histopathological predictions, regardless of accuracy, had no influence on the final assessment.
ROC curve analyses
The scoring of mastitis was similar to that of malignant lesions in our scoring protocol, but a patient’s clinical history was important for diagnosing mastitis. Because our protocol had low accuracy for diagnosing mastitis and there were few cases of mastitis in the analysis (29/898), mastitis was excluded from the ROC curve analyses. The ROC curves for BI-RADS category, TIC alone, and ADC alone are shown in Figure 4. The pooled AUC was 0.896 (95% CI: 0.865–0.926), 0.827 (95% CI: 0.791–0.862), and 0.419 (95% CI: 0.372–0.466) for BI-RADS category, TIC alone, and ADC alone, respectively. This simplified protocol for BI-RADS categorization, integrating MF, TIC, and ADC, had high diagnostic accuracy.
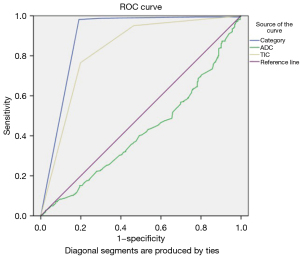
Sensitivity, specificity, accuracy, and ROC of the protocol for BI-RADS categorization
When category 4 and 5 lesions were classified as malignant, and risk and malignant lesions were grouped together, the sensitivity, specificity, accuracy, and AUC were 92.9% (535/576), 82.3% (241/293), 89.3% (776/869), and 0.876 (95% CI: 0.847–0.904), respectively. When category 5 lesions were classified as malignant, and risk and malignant lesions were grouped together, the sensitivity, specificity, accuracy, and AUC were 88.7% (511/576), 93.2% (273/293), 90.2% (784/869), and 0.909 (95% CI: 0.887–0.932), respectively. When category 5 lesions were classified as malignant, and risk and benign lesions were grouped together, the sensitivity, specificity, accuracy, and AUC were 97.7% (461/472), 82.4% (327/397), 90.7% (788/869), and 0.900 (95% CI: 0.876–0.924), respectively. When category 4 and 5 lesions were classified as malignant, and risk and benign lesions were grouped together, the sensitivity, specificity, accuracy, and AUC were 98.9% (467/472), 69.8% (277/397), 85.6% (744/869), and 0.844 (95% CI: 0.815–0.872), respectively. Therefore, to improve the classification accuracy of borderline category 4 lesions, category 4 lesions were classified as benign lesions and only category 5 lesions were classified as malignant lesions. When risk and malignant lesions were grouped together, both the specificity (93.2% vs. 82.4%) and the AUC value (0.909 vs. 0.900) were improved compared to when the risk and benign lesions were grouped together, and the sensitivity was satisfactory. Therefore, to optimize the diagnostic efficacy, the risk lesions were grouped with the malignant lesions for traditional two-level malignant-benign differentiation. Statistical optimization was achieved when the pathological “risk + malignant” lesions—corresponding to category 5—were grouped together (Table 3; Figure 5).
Table 3
Imaging prediction of malignancy | Pathological malignancy | Sensitivity | Specificity | Accuracy | AUC |
---|---|---|---|---|---|
Category 4 and 5 | Risk and malignant lesions | 92.9% | 82.3% | 89.3% | 0.876 (0.847–0.904) |
Category 5 | Risk and malignant lesions | 88.7% | 93.2% | 90.2% | 0.909 (0.887–0.932) |
Category 5 | Malignant lesions | 97.7% | 82.4% | 90.7% | 0.900 (0.876–0.924) |
Category 4 and 5 | Malignant lesions | 98.9% | 69.8% | 85.6% | 0.844 (0.815–0.872) |
AUC, area under the receiver operating characteristic curve.
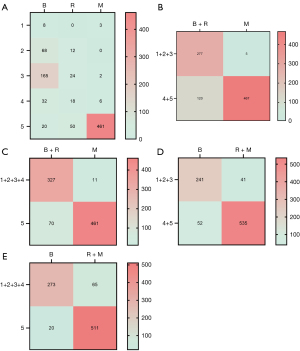
Discussion
In this study, a simplified scoring protocol that used spatially matched axial T2WI, T1WI, DWI, and DCE images was proposed to improve the consistency of BI-RADS categorization. The protocol integrated MF, TIC, and ADC features with equal weights and can be used in a straightforward manner (e.g., lesions were classified as category 4 if one of those three features was suspicious, and as category 5 if any two of them were suspicious). Multiparametric MRI improved the diagnostic accuracy, which is a finding that has also been reported by some existing studies (29-31). Although protocols with more complicated logistic regression have been used in previous research (32-34), our proposed simplified and straightforward scoring system had sensitivity and specificity comparable with those reported in these earlier studies. This protocol emphasized a simplified approach, which is more convenient and practical in breast MRI reading, whereas protocols with more complicated logistic regression are more suitable for computer pattern recognition. Moreover, each image was reported via the process of composition-supervisor audit to reach a consensus on the BI-RADS category. Our simplified scoring protocol aids in converting specific imaging features into a diagnostic BI-RADS category; thus, it may help to achieve a consensus on subjective reading and BI-RADS categorization between junior doctors and senior doctors with less clinical experience. This simplified scoring protocol therefore holds promise as a helpful and practical tool for use by even the least-experienced radiologist to reduce inter-observer variability related to reader experience.
In 2013, a classification tree flowchart with 17 different variables based on a large database, later termed the Kaiser score, was introduced to help with the interpretation of enhanced breast MRI lesions (35). This classification tree includes five major diagnostic criteria: root sign, dynamic enhancement curve type, margins, internal enhancement pattern, and edema. Since its introduction in 2013, the Kaiser score has been reported to have high diagnostic accuracy for the differential diagnosis of benign and malignant lesions in a variety of selected patient populations (36-40). However, the Kaiser score does not include quantitative MRI techniques, such as DWI or quantitative DCE, and it is not associated with the BI-RADS lexicon. Moreover, the Kaiser score, which includes 17 variables, still requires further simplification for easy clinical application. In this study, a simplified scoring protocol was proposed for BI-RADS categorization based on dynamic protocols and quantitative techniques to improve diagnostic accuracy.
Our study also investigated the borderline effect of pathological risk and category 4 lesions. The sensitivity and specificity, reported from traditional two-scale differentiation between malignant and benign lesions, varied considerably. Possible reasons for this variation included whether the risk lesions were classified together with malignant or benign lesions and the classification of category 4 risk lesions (as malignant or benign) (41). Our results showed that when the risk lesions were classified together with malignant lesions, the AUC value improved. This result shows that the MF, TIC, and ADC of risk lesions showed greater similarity with features of malignant lesions than benign lesions. This finding also supports the current recommendation that patients exhibiting risk lesions should undergo surgical excision or, at the least, be closely followed up (42,43), due to their potential for malignancy. Grouping category 4 lesions together with malignant or benign lesions has a direct impact on the BI-RADS categorization; therefore, category 4 lesions should be treated with extreme caution. Our results showed that only 17.3% (18/104) of lesions were classified as category 4. When category 4 and 5 lesions were classified as malignant, the sensitivity, specificity, accuracy, and ROC all decreased. Therefore, to improve the diagnostic accuracy of the protocol for BI-RADS categorization, only category 5 lesions should be treated as malignant lesions. Ideally, three-level differentiation is recommended, with malignant, risk, and benign lesions corresponding to category 5, category 4, and category 3 or lower, respectively. The management of category 4 lesions depends on localization—if the lesions are local, then a biopsy is recommended; otherwise, a short-term follow-up should be suggested (44).
Another advantage of our proposed simplified protocol is that we acquired isotropic, high spatial resolution images, with a DCE sequence at 1 mm × 1 mm × 1 mm. Such high spatial resolution was sufficient for providing finer structural details, such as a spiculated margin and cluster-ring patterns, and at the same time preserved the TIC information without compromising the kinetic curve profile. Our study also showed that the MF should be evaluated on the first passage of the DCE scan in the three orthogonal views (axial, sagittal, and coronal) (45,46). The complementary but delayed sagittal or coronal scan was not chosen, as such a delay might enhance BPE (47), which may obscure the lesions and in turn lead to imaging misinterpretation and, ultimately, underestimation or overestimation of lesions, or even missing them altogether (48-50). The isotropic acquisition was set to a resolution that allows three orthogonal views to be acquired in the same phase by multiplanar reconstruction or maximum intensity projection processing in a fast manner. Therefore, the examination time was shortened and better patient cooperation could be achieved.
Our study has potential limitations. First, only histopathologically verified lesions were considered, thus potentially biasing our lesion database toward more complex cases for which invasive diagnosis was requested in the first place. However, we included only these lesions so as to provide a more accurate reference standard. Second, according to the Centre for Evidence-Based Medicine reasoning, our simplified scoring protocol was exploratory. To achieve a high level of diagnostic accuracy, a validation study in a single clinical center is required. We expect other research centers to validate our protocol. Furthermore, our study was retrospective and included ahigh ratio of malignant to benign lesions. Therefore, prospective studies are needed to confirm the validity of breast MRI and our score in this setting. Third, our study was performed considering only MRI features and did not integrate patient characteristics.
Conclusions
Our simplified scoring protocol integrates MF, TIC, and ADC values with equal weights to improve the consistency of BI-RADS categorization, diagnostic performance in the clinical practice, and the understanding of benign, risk, and malignant breast diseases.
Acknowledgments
Funding: None.
Footnote
Reporting Checklist: The authors have completed the STARD reporting checklist. Available at https://qims.amegroups.com/article/view/10.21037/qims-21-1036/rc
Conflicts of Interest: All authors have completed the ICMJE uniform disclosure form (available at https://qims.amegroups.com/article/view/10.21037/qims-21-1036/coif). The authors have no conflicts of interest to declare.
Ethical Statement: The authors are accountable for all aspects of the work in ensuring that questions related to the accuracy or integrity of any part of the work are appropriately investigated and resolved. The study was conducted in accordance with the Declaration of Helsinki (as revised in 2013). The study was approved by the Ethics Committee of Chinese People’s Liberation Army General Hospital (No. S2019-093-01), and individual consent for this retrospective analysis was waived.
Open Access Statement: This is an Open Access article distributed in accordance with the Creative Commons Attribution-NonCommercial-NoDerivs 4.0 International License (CC BY-NC-ND 4.0), which permits the non-commercial replication and distribution of the article with the strict proviso that no changes or edits are made and the original work is properly cited (including links to both the formal publication through the relevant DOI and the license). See: https://creativecommons.org/licenses/by-nc-nd/4.0/.
References
- Hovis KK, Lee JM, Hippe DS, Linden H, Flanagan MR, Kilgore MR, Yee J, Partridge SC, Rahbar H. Accuracy of Preoperative Breast MRI Versus Conventional Imaging in Measuring Pathologic Extent of Invasive Lobular Carcinoma. J Breast Imaging 2021;3:288-98. [Crossref] [PubMed]
- Orel SG, Schnall MD, Powell CM, Hochman MG, Solin LJ, Fowble BL, Torosian MH, Rosato EF. Staging of suspected breast cancer: effect of MR imaging and MR-guided biopsy. Radiology 1995;196:115-22. [Crossref] [PubMed]
- Plana MN, Carreira C, Muriel A, Chiva M, Abraira V, Emparanza JI, Bonfill X, Zamora J. Magnetic resonance imaging in the preoperative assessment of patients with primary breast cancer: systematic review of diagnostic accuracy and meta-analysis. Eur Radiol 2012;22:26-38. [Crossref] [PubMed]
- Houssami N, Ciatto S, Macaskill P, Lord SJ, Warren RM, Dixon JM, Irwig L. Accuracy and surgical impact of magnetic resonance imaging in breast cancer staging: systematic review and meta-analysis in detection of multifocal and multicentric cancer. J Clin Oncol 2008;26:3248-58. [Crossref] [PubMed]
- Sindi R, Wong YH, Yeong CH, Sun Z. Development of patient-specific 3D-printed breast phantom using silicone and peanut oils for magnetic resonance imaging. Quant Imaging Med Surg 2020;10:1237-48. [Crossref] [PubMed]
- Brennan ME, Houssami N, Lord S, Macaskill P, Irwig L, Dixon JM, Warren RM, Ciatto S. Magnetic resonance imaging screening of the contralateral breast in women with newly diagnosed breast cancer: systematic review and meta-analysis of incremental cancer detection and impact on surgical management. J Clin Oncol 2009;27:5640-9. [Crossref] [PubMed]
- Wang SY, Long JB, Killelea BK, Evans SB, Roberts KB, Silber A, Gross CP. Preoperative Breast Magnetic Resonance Imaging and Contralateral Breast Cancer Occurrence Among Older Women With Breast Cancer. J Clin Oncol 2016;34:321-8. [Crossref] [PubMed]
- Susnik B, Schneider L, Swenson KK, Krueger J, Braatz C, Lillemoe T, Tsai M, DeFor TE, Knaack M, Rueth N. Predictive value of breast magnetic resonance imaging in detecting mammographically occult contralateral breast cancer: Can we target women more likely to have contralateral breast cancer? J Surg Oncol 2018;118:221-7. [Crossref] [PubMed]
- Riedl CC, Luft N, Bernhart C, Weber M, Bernathova M, Tea MK, Rudas M, Singer CF, Helbich TH. Triple-modality screening trial for familial breast cancer underlines the importance of magnetic resonance imaging and questions the role of mammography and ultrasound regardless of patient mutation status, age, and breast density. J Clin Oncol 2015;33:1128-35. [Crossref] [PubMed]
- Warner E, Messersmith H, Causer P, Eisen A, Shumak R, Plewes D. Systematic review: using magnetic resonance imaging to screen women at high risk for breast cancer. Ann Intern Med 2008;148:671-9. [Crossref] [PubMed]
- Zhang X, Wang D, Liu Z, Wang Z, Li Q, Xu H, Zhang B, Liu T, Jin F. The diagnostic accuracy of magnetic resonance imaging in predicting pathologic complete response after neoadjuvant chemotherapy in patients with different molecular subtypes of breast cancer. Quant Imaging Med Surg 2020;10:197-210. [Crossref] [PubMed]
- Orel SG, Weinstein SP, Schnall MD, Reynolds CA, Schuchter LM, Fraker DL, Solin LJ. Breast MR imaging in patients with axillary node metastases and unknown primary malignancy. Radiology 1999;212:543-9. [Crossref] [PubMed]
- de Bresser J, de Vos B, van der Ent F, Hulsewé K. Breast MRI in clinically and mammographically occult breast cancer presenting with an axillary metastasis: a systematic review. Eur J Surg Oncol 2010;36:114-9. [Crossref] [PubMed]
- D'Orsi C, Morris E, Mendelson E. ACR BI-RADS® Atlas, Breast Imaging Reporting and Data System, 2013.
- Pinker K, Helbich TH, Morris EA. The potential of multiparametric MRI of the breast. Br J Radiol 2017;90:20160715. [Crossref] [PubMed]
- Bennani-Baiti B, Baltzer PA. MR Imaging for Diagnosis of Malignancy in Mammographic Microcalcifications: A Systematic Review and Meta-Analysis. Radiology 2017;283:692-701. [Crossref] [PubMed]
- Fujiwara K, Yamada T, Kanemaki Y, Okamoto S, Kojima Y, Tsugawa K, Nakajima Y. Grading System to Categorize Breast MRI in BI-RADS 5th Edition: A Multivariate Study of Breast Mass Descriptors in Terms of Probability of Malignancy. AJR Am J Roentgenol 2018;210:W118-27.
- Zhang M, Horvat JV, Bernard-Davila B, Marino MA, Leithner D, Ochoa-Albiztegui RE, Helbich TH, Morris EA, Thakur S, Pinker K. Multiparametric MRI model with dynamic contrast-enhanced and diffusion-weighted imaging enables breast cancer diagnosis with high accuracy. J Magn Reson Imaging 2019;49:864-74. [Crossref] [PubMed]
- Shimauchi A, Ota H, Machida Y, Yoshida T, Satani N, Mori N, Takase K, Tozaki M. Morphology evaluation of nonmass enhancement on breast MRI: Effect of a three-step interpretation model for readers' performances and biopsy recommendations. Eur J Radiol 2016;85:480-8. [Crossref] [PubMed]
- Benndorf M, Baltzer PA, Kaiser WA. Assessing the degree of collinearity among the lesion features of the MRI BI-RADS lexicon. Eur J Radiol 2011;80:e322-4. [Crossref] [PubMed]
- El Khoury M, Lalonde L, David J, Labelle M, Mesurolle B, Trop I. Breast imaging reporting and data system (BI-RADS) lexicon for breast MRI: interobserver variability in the description and assignment of BI-RADS category. Eur J Radiol 2015;84:71-6. [Crossref] [PubMed]
- Lunkiewicz M, Forte S, Freiwald B, Singer G, Leo C, Kubik-Huch RA. Interobserver variability and likelihood of malignancy for fifth edition BI-RADS MRI descriptors in non-mass breast lesions. Eur Radiol 2020;30:77-86.
- Grimm LJ, Anderson AL, Baker JA, Johnson KS, Walsh R, Yoon SC, Ghate SV. Interobserver Variability Between Breast Imagers Using the Fifth Edition of the BI-RADS MRI Lexicon. AJR Am J Roentgenol 2015;204:1120-4.
- Mann RM, Cho N, Moy L. Breast MRI: State of the Art. Radiology 2019;292:520-36. [Crossref] [PubMed]
- Leithner D, Wengert GJ, Helbich TH, Thakur S, Ochoa-Albiztegui RE, Morris EA, Pinker K. Clinical role of breast MRI now and going forward. Clin Radiol 2018;73:700-14. [Crossref] [PubMed]
- Perry N, Broeders M, de Wolf C, Törnberg S, Holland R, von Karsa L. European guidelines for quality assurance in breast cancer screening and diagnosis. Fourth edition--summary document. Ann Oncol 2008;19:614-22.
- Rageth CJ, O'Flynn EAM, Pinker K, Kubik-Huch RA, Mundinger A, Decker T, Tausch C, Dammann F, Baltzer PA, Fallenberg EM, Foschini MP, Dellas S, Knauer M, Malhaire C, Sonnenschein M, Boos A, Morris E, Varga Z. Second International Consensus Conference on lesions of uncertain malignant potential in the breast (B3 lesions). Breast Cancer Res Treat 2019;174:279-96. [Crossref] [PubMed]
- Tan E, Arachchi A, Cheng M, Lockie D. Indeterminate (B3) Breast Lesions and the Ongoing Role of Diagnostic Open Biopsy. Int J Breast Cancer 2021;2021:5555458. [Crossref] [PubMed]
- Ma D, Lu F, Zou X, Zhang H, Li Y, Zhang L, Chen L, Qin D, Wang B. Intravoxel incoherent motion diffusion-weighted imaging as an adjunct to dynamic contrast-enhanced MRI to improve accuracy of the differential diagnosis of benign and malignant breast lesions. Magn Reson Imaging 2017;36:175-9. [Crossref] [PubMed]
- Li K, Machireddy A, Tudorica A, Moloney B, Oh KY, Jafarian N, Partridge SC, Li X, Huang W. Discrimination of Malignant and Benign Breast Lesions Using Quantitative Multiparametric MRI: A Preliminary Study. Tomography 2020;6:148-59. [Crossref] [PubMed]
- Pinker K, Bogner W, Baltzer P, Gruber S, Bickel H, Brueck B, Trattnig S, Weber M, Dubsky P, Bago-Horvath Z, Bartsch R, Helbich TH. Improved diagnostic accuracy with multiparametric magnetic resonance imaging of the breast using dynamic contrast-enhanced magnetic resonance imaging, diffusion-weighted imaging, and 3-dimensional proton magnetic resonance spectroscopic imaging. Invest Radiol 2014;49:421-30. [Crossref] [PubMed]
- Sorace AG, Partridge SC, Li X, Virostko J, Barnes SL, Hippe DS, Huang W, Yankeelov TE. Distinguishing benign and malignant breast tumors: preliminary comparison of kinetic modeling approaches using multi-institutional dynamic contrast-enhanced MRI data from the International Breast MR Consortium 6883 trial. J Med Imaging (Bellingham) 2018;5:011019. [PubMed]
- Szabó BK, Aspelin P, Wiberg MK, Boné B. Dynamic MR imaging of the breast. Analysis of kinetic and morphologic diagnostic criteria. Acta Radiol 2003;44:379-86. [PubMed]
- Demartini WB, Kurland BF, Gutierrez RL, Blackmore CC, Peacock S, Lehman CD. Probability of malignancy for lesions detected on breast MRI: a predictive model incorporating BI-RADS imaging features and patient characteristics. Eur Radiol 2011;21:1609-17. [Crossref] [PubMed]
- Baltzer PA, Dietzel M, Kaiser WA. A simple and robust classification tree for differentiation between benign and malignant lesions in MR-mammography. Eur Radiol 2013;23:2051-60. [Crossref] [PubMed]
- Marino MA, Clauser P, Woitek R, Wengert GJ, Kapetas P, Bernathova M, Pinker-Domenig K, Helbich TH, Preidler K, Baltzer PA. A simple scoring system for breast MRI interpretation: does it compensate for reader experience? Eur Radiol 2016;26:2529-37. [Crossref] [PubMed]
- Wengert GJ, Pipan F, Almohanna J, Bickel H, Polanec S, Kapetas P, Clauser P, Pinker K, Helbich TH, Baltzer PAT. Impact of the Kaiser score on clinical decision-making in BI-RADS 4 mammographic calcifications examined with breast MRI. Eur Radiol 2020;30:1451-9. [Crossref] [PubMed]
- Woitek R, Spick C, Schernthaner M, Rudas M, Kapetas P, Bernathova M, Furtner J, Pinker K, Helbich TH, Baltzer PAT. A simple classification system (the Tree flowchart) for breast MRI can reduce the number of unnecessary biopsies in MRI-only lesions. Eur Radiol 2017;27:3799-809. [Crossref] [PubMed]
- Istomin A, Masarwah A, Vanninen R, Okuma H, Sudah M. Diagnostic performance of the Kaiser score for characterizing lesions on breast MRI with comparison to a multiparametric classification system. Eur J Radiol 2021;138:109659. [Crossref] [PubMed]
- Jajodia A, Sindhwani G, Pasricha S, Prosch H, Puri S, Dewan A, Batra U, Doval DC, Mehta A, Chaturvedi AK. Application of the Kaiser score to increase diagnostic accuracy in equivocal lesions on diagnostic mammograms referred for MR mammography. Eur J Radiol 2021;134:109413. [Crossref] [PubMed]
- Petrovecki M, Alduk AM, Jakic-Razumovic J, Stern-Padovan R, Bubic V, Prutki M. Role of breast imaging in predicting outcome of lesions of uncertain malignant potential (B3) diagnosed at core needle biopsy. Tumori 2016;102:203-8. [Crossref] [PubMed]
- Silverstein MJ, Recht A, Lagios MD, Bleiweiss IJ, Blumencranz PW, Gizienski T, Harms SE, Harness J, Jackman RJ, Klimberg VS, Kuske R, Levine GM, Linver MN, Rafferty EA, Rugo H, Schilling K, Tripathy D, Vicini FA, Whitworth PW, Willey SC. Special report: Consensus conference III. Image-detected breast cancer: state-of-the-art diagnosis and treatment. J Am Coll Surg 2009;209:504-20. [Crossref] [PubMed]
- Simmons RM, Osborne MP. The evaluation of high risk and pre-invasive breast lesions and the decision process for follow up and surgical intervention. Surg Oncol 1999;8:55-65. [Crossref] [PubMed]
- Preibsch H, Wanner LK, Staebler A, Hahn M, Siegmann-Luz KC. Malignancy rates of B3-lesions in breast magnetic resonance imaging - do all lesions have to be excised? BMC Med Imaging 2018;18:27. [Crossref] [PubMed]
- Yabuuchi H, Matsuo Y, Kamitani T, Setoguchi T, Okafuji T, Soeda H, Sakai S, Hatakenaka M, Kubo M, Tokunaga E, Yamamoto H, Honda H. Non-mass-like enhancement on contrast-enhanced breast MR imaging: lesion characterization using combination of dynamic contrast-enhanced and diffusion-weighted MR images. Eur J Radiol 2010;75:e126-32. [Crossref] [PubMed]
- Lee SM, Nam KJ, Choo KS, Kim JY, Jeong DW, Kim HY, Kim JY. Patterns of malignant non-mass enhancement on 3-T breast MRI help predict invasiveness: using the BI-RADS lexicon fifth edition. Acta Radiol 2018;59:1292-9.
- Hu N, Zhao J, Li Y, Fu Q, Zhao L, Chen H, Qin W, Yang G. Breast cancer and background parenchymal enhancement at breast magnetic resonance imaging: a meta-analysis. BMC Med Imaging 2021;21:32. [Crossref] [PubMed]
- Clauser P, Dietzel M, Weber M, Kaiser CG, Baltzer PA. Motion artifacts, lesion type, and parenchymal enhancement in breast MRI: what does really influence diagnostic accuracy? Acta Radiol 2019;60:19-27. [Crossref] [PubMed]
- Tomida T, Urikura A, Uematsu T, Shirata K, Nakaya Y. Contrast Enhancement in Breast Cancer and Background Mammary-Gland Tissue During the Super-Early Phase of Dynamic Breast Magnetic Resonance Imaging. Acad Radiol 2017;24:1380-6. [Crossref] [PubMed]
- Baek JE, Kim SH, Lee AW. Background parenchymal enhancement in breast MRIs of breast cancer patients: impact on tumor size estimation. Eur J Radiol 2014;83:1356-62. [Crossref] [PubMed]