Quantitative analysis of high-resolution computed tomography features of idiopathic pulmonary fibrosis: a structure-function correlation study
Introduction
Idiopathic pulmonary fibrosis (IPF) is a progressive fibrotic lung disease of increasing incidence with median survival of 3–5 years (1,2). At present, pulmonary function tests (PFTs) and high-resolution computed tomography (HRCT) are 2 key methods for assessing the severity and progression of IPF (3-5). The typical findings of IPF on HRCT include a subpleural and basal-predominant reticular pattern (RE) and honeycombing (HC) with or without traction bronchiectasis (TRBR) (6,7). Importantly, HC on HRCT independently predicts mortality in patients with IPF (8). However, a CT-based, visual, semi-quantitative evaluation method for assessing disease severity is vulnerable to interobserver variability; PFTs reflect whole lung function and so may not be performed in patients with severe IPF. In recent years, computer-assisted quantitative CT (QCT) has gained attention for proving to be more accurate than visual scoring (9,10). Nowadays, the method of QCT is mainly focused on the pulmonary parenchyma and pulmonary vasculature (11-13). Nakagawa et al. (12,14) developed a computer-aided method to measure the HC area on 3 axial HRCT slices and discovered that the HC area significantly correlated with the forced vital capacity in percent predicted values [FVC (%pred)] and the percentage of predicted diffusing capacity for carbon monoxide [DLco (%pred)]. In our previous research, the deep learning-based segmentation algorithm was used to conduct a quantitative analysis of the volume of clot burden of acute pulmonary embolism and the volume of pulmonary vessels (15,16). There has been evidence of an association between pulmonary vascular-related parameters and the prognosis of IPF (9,17,18). Paradoxically, many of these methods make it difficult to distinguish HC from TRBR and pulmonary vascular.
Thus, there were 3 purposes in our research: (I) to segment and calculate the volumes of imaging features of IPF on HRCT, (II) to ascertain the association of imaging features with pulmonary function tests (PFTs), and (III) to quantify pulmonary vascular-related parameters and analyze the correlation with diverse lesions. We present the following article in accordance with the Strengthening the Reporting of Observational Studies in Epidemiology (STROBE) reporting checklist (available at https://qims.amegroups.com/article/view/10.21037/qims-21-1232/rc).
Methods
Study cohort and design
This single-center, retrospective, cohort study was conducted in accordance with the Declaration of Helsinki (as revised in 2013). The study was approved by the Ethics Board of the China-Japan Friendship Hospital Committee (No. 2017-25), and individual consent for this retrospective analysis was waived. Patients diagnosed with IPF at the China-Japan Friendship Hospital from January 2015 to June 2021 were included. The diagnosis of IPF was made by multidisciplinary teams according to the 2011 American Thoracic Society (ATS), European Respiratory Society (ERS), Japanese Respiratory Society (JRS), and Latin American Thoracic Association (ALAT) criteria (6). All participants underwent standard protocols. The information we collected included age, gender, smoking history, body mass index (BMI), PFTs, and chest HRCT-related parameters. The inclusion criteria were as follows: (I) patients with IPF who had received at least 1 supine HRCT scan and (II) PFTs completed within 1 week of HRCT. The exclusion criteria were as follows: (I) other comorbid lung disease such as combined pulmonary fibrosis and emphysema (CPFE) or pleural effusion or malignancy, (II) patients with heart failure, and (III) significant respiratory motion artifacts on HRCT. The detailed flow chart of patient screening is shown in Figure 1.
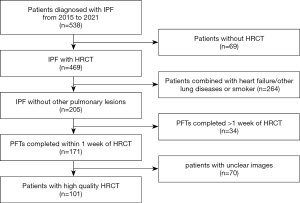
CT protocol
All participants underwent HRCT in a supine position at the end of inspiration from the lung apex to the lung base on a multi-layer spiral CT device (Lightspeed VCT/64, GE Healthcare, Milwaukee, WI, USA; Toshiba Aquilion One TSX-301C/320, Toshiba, Tokyo, Japan; Philips iCT/256, Philips Healthcare, Best, The Netherlands; or Siemens FLASH Dual Source CT, Siemens Healthcare, Forchheim, Germany). The HRCT scanning protocol was spiral mode with the following acquisition and reconstruction parameters: tube voltage of 100–120 kV, tube current of 100–300 mAs, section thickness of 0.625–1 mm, table speed of 39.37 mm/s, gantry rotation time of 0.8 s, and reconstruction increment of 1–1.25 mm.
Lesion segmentation and quantitative assessment
Lung segmentation was performed using the deep learning-based segmentation method (InferReadTM CT Lung, version R3.12.3; Infervision Medical Technology Co., Ltd., Beijing, China) and corrected by 2 radiologists. The radiologists, with 9 and 15 years of clinical experience, respectively, manually segmented 4 radiological features of IPF on HRCT: HC, RE, ground glass opacity (GGO), and TRBR. The HC is a subpleural collection of cystic air, the cysts are adjacent and in contact with the pleural surface, and the cyst size is usually 3–5 mm. The RE is composed of a fine network or mesh of overlapping linear lines within the secondary pulmonary lobules. A GGO comprises a homogenous area of increased pulmonary opacity where the increased opacity does not obscure the underlying bronchial and vascular structures and hence is less dense than consolidation. The TRBR involves irreversible dilatation of the airway associated with adjacent pulmonary fibrosis in which the dilated airways are generally irregular and tortuous (7). In this study, 3 equal segmentations of the lung in the vertical direction were performed (through the right upper lung, right middle lung, right lower lung, left upper lung, left middle lung, and left lower lung) (Figure 2) and the computer automatically measured the volume of the lesion at each site and calculated the lesion percentage from the lung volume.
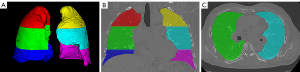
Vessel segmentation and quantitative assessment
Based on our previous method (19), the lung vessels on HRCT were automatically segmented using an automatic integration segmentation method. The main vascular parameters quantified included total pulmonary vascular volume (TPVV), pulmonary vein volume (PVV), pulmonary artery volume (PAV), and the ratio of each to total lung volume along with the corresponding number of vessels. The major steps were as follows: (I) identification of extrapulmonary arteries and veins with the U-Net architecture, (II) identification of the intrapulmonary vessels with a computational differential geometry solution, (III) skeletonization of the intrapulmonary vessels to guide the tracing of adjacent vascular branches, (IV) tracing the skeletons of the intrapulmonary vessels to distinguish arteries and veins starting from the extrapulmonary arteries and veins, and (V) the automatic segmentation of the pulmonary vascular tree, which was examined by the radiologist and manually corrected.
PFTs
The PFTs were performed according to ERS/ATS guidelines, and within 1 week of HRCT. Among them, we focused on analysis of the percentage of predicted vital capacity (VC%), percentage of predicted forced vital capacity (FVC%), forced expiratory volume in 1 second (FEV1%), percentage of predicted total lung capacity (TLC%), and DLco%.
Statistical analysis
The software SPSS 24.0 (IBM Corp., Armonk, NY, USA) was used for statistical analysis. The Kolmogorov-Smirnov test was performed to assess the normality of the data. Continuous variables conforming to a normal distribution were expressed as mean ± standard deviation (SD), and non-normally distributed continuous variables were expressed as median and interquartile range (IQR). Correlations between various vascular parameters, lesion extent, and PFTs were analyzed by Person/Spearman Rho correlation coefficients. A P value <0.05 was considered statistically significant.
Results
Clinical characteristics
A total of 101 patients with a definitive diagnosis of IPF by multidisciplinary teams were included in this study. Demographic data, PFTs, and pulmonary vascular-related parameters are shown in Table 1. The median age of the patients was 63 years (IQR, 58 to 68 years) and they were predominantly male (92.1%). The mean interval between CT and PFTs was 4 days. Mean PFT results were 79.20%±21.76% for VC; 81.42%±23.26% for FVC; 83.50%±21.94% for FEV1; 67.86%±14.13% for TLC; and 56.53%±17.56% for DLco.
Table 1
Characteristics | Value |
---|---|
Median age (years)† | 63 (58 to 68) |
Male/female | 93/8 |
BMI (kg/m2)† | 24.8 (23.9 to 26.1) |
Mean pulmonary function test values# | |
VC% predicted | 79.20±21.76 |
FVC% predicted | 81.42±23.26 |
FEV1% predicted | 83.50±21.94 |
TLC% predicted | 67.86±14.13 |
DLco% predicted | 56.53±17.56 |
Total lung volume (mL) # | 3813.95±914.16 |
Pulmonary vascular-related indexes† | |
TPVV (mL) | 88.80 (63.77 to 121.77) |
TPVV (%) | 0.02 (0.02 to 0.03) |
The number of pulmonary vessel branches | 426 (334 to 556) |
PAV (mL) | 46.94 (34.96 to 62.85) |
PAV (%) | 0.01 (0.01 to 0.02) |
The number of pulmonary artery branches | 237 (191 to 283) |
PVV (mL) | 40.15 (28.58 to 59.84) |
PVV (%) | 0.01 (0 to 0.02) |
The number of pulmonary vein branches | 186 (134 to 279) |
†, numbers in parentheses are the interquartile range; #, data represent mean values with SD. BMI, body mass index; VC%, percentage of predicted vital capacity; FVC%, percentage of predicted forced vital capacity; FEV1%, forced expiratory volume in 1 second; TLC%, percentage of predicted total lung capacity; DLco%, percentage of predicted diffusing capacity for carbon monoxide; TPVV, total pulmonary vascular volume; PAV, pulmonary artery volume; PVV, pulmonary vein volume.
Quantitative HRCT analysis
Mean total lung volume was 3,813.95±914.16 mL. The RE was the most widespread class among the 4 major lesions, with a median volume of 286.78 mL (IQR, 126.36 to 595.97 mL) and a median ratio of 8.30% (IQR, 3.37% to 15.26%), followed by HC [0 mL (IQR, 0 to 103.80 mL) and 0% (IQR, 0 to 3.52%), respectively], TRBR [1.55 mL (IQR, 0.45 to 9.96 mL) and 0.04% (IQR, 0.01% to 0.25%), respectively], and GGO [0% (IQR, 0 to 0%) and 0% (IQR, 0 to 0%), respectively] (Figure 3A,3B). Meanwhile, the lesion of the right lower lung was significantly higher than that of the middle lung and upper lung [27.50% (IQR, 14.44% to 51.0%) vs. 7.05% (IQR, 3.33% to 17.03%) vs. 4.65% (IQR, 0.77% to 15.47%), P<0.05], and the same phenomenon was also observed in the left lung (Table 2). Additionally, the median TPVV, TPVV ratios, and the number of vascular branches were 88.80 mL (IQR, 63.77 to 121.77 mL), 0.02% (IQR, 0.02% to 0.03%), and 426 (IQR, 334 to 556), respectively (Figure 3C).
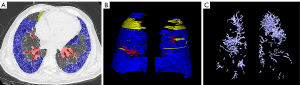
Table 2
Characteristics | Value |
---|---|
Total lesion volume (mL) | 522.42 (251.32 to 836.55) |
Total lesion ratio (%) | 12.85 (6.24 to 22.53) |
HC (mL) | 0 (0 to 103.80) |
HC (%) | 0 (0 to 3.52) |
RE (mL) | 286.78 (126.36 to 595.97) |
RE (%) | 8.30 (3.37 to 15.26) |
TRBR (mL) | 1.55 (0.45 to 9.96) |
TRBR (%) | 0.04 (0.01 to 0.25) |
GGO (mL) | 0 (0 to 0) |
GGO (%) | 0 (0 to 0) |
Right upper lung lesion (%) | 4.65 (0.77 to 15.47) |
Right middle lung lesion (%) | 7.05 (3.33 to 17.03) |
Right lower lung lesion (%) | 27.50 (14.44 to 51.00) |
Left upper lung lesion (%) | 2.82 (0.50 to 8.21) |
Left middle lunge lesion (%) | 10.31 (2.96 to 18.48) |
Left lower lung lesion (%) | 28.61 (9.65 to 53.93) |
All values are presented as median and interquartile range (IQR). IPF, idiopathic pulmonary fibrosis; HRCT, high-resolution computed tomography; TRBR, traction bronchiectasis; RE, reticular pattern; HC, honeycombing; GGO, ground glass opacity.
Correlation between HRCT features and PFTs
There was a positive correlation between total lung volume and PFTs. Whereas lesion extent was negatively associated with lung function, with the strongest correlation coefficient (r) between the total lesion volume ratio and PFTs (VC, r=−0.62, P<0.001; FVC, r=−0.60, P<0.001; FEV1, r=−0.57, P<0.001; TLC, r=−0.61, P<0.001; DLco, r=−0.67, P<0.001) (Figure S1A,S1B). Among the sub-lesion categories, HC showed the strongest correlation with DLco (r=−0.45, P<0.001) followed by RE (r=−0.41, P<0.001), and TRBR correlated weakly with DLco (r=−0.25, P<0.001). The RE had the strongest relationship with VC (r=−0.48, P<0.001), FVC (r=−0.47, P<0.001), FEV1 (r=−0.47, P<0.001), and TLC (r=−0.47, P<0.001). There was no statistical correlation between GGO and PFTs (P>0.05). Analysis of the extent of upper, middle, and lower lung lesions separately all revealed relationship with PFTs (P<0.001) (Figure S1C-S1H). Table 3 indicates that pulmonary vascular-related indices, such as pulmonary vascular volume and the number of vascular branches, were positively correlated with PFTs, and the highest correlation was found with DLco (r=0.28 to 0.41).
Table 3
Characteristics | VC% | FVC% | FEV1% | TLC% | DLco% |
---|---|---|---|---|---|
Total lung volume (mL) | 0.45** | 0.47** | 0.50** | 0.49** | 0.24* |
All lesion volume (mL) | −0.51** | −0.49** | −0.45** | −0.50** | −0.62** |
All lesion ratio (%) | −0.62** | −0.60** | −0.57** | −0.61** | −0.67** |
HC (%) | −0.12 | −0.12 | −0.09 | −0.19 | −0.45** |
RE (%) | −0.48** | −0.47** | −0.47** | −0.47** | −0.41** |
TRBR (%) | −0.29** | −0.27** | −0.17 | −0.31** | −0.25* |
GGO (%) | −0.07 | −0.10 | −0.08 | −0.04 | 0.05 |
Right upper lung lesion (%) | −0.54** | −0.54** | −0.51** | −0.58** | −0.57** |
Right middle lung lesion (%) | −0.52** | −0.51** | −0.48** | −0.53** | −0.52** |
Right lower lung lesion (%) | −0.49** | −0.49** | −0.48** | −0.46** | −0.52** |
Left upper lung lesion (%) | −0.43** | −0.42** | −0.38** | −0.40** | −0.48** |
Left middle lunge lesion (%) | −0.61** | −0.58** | −0.55** | −0.55** | −0.56** |
Left lower lung lesion (%) | −0.40** | −0.39** | −0.39** | −0.38** | −0.51** |
Pulmonary vascular-related indexes | |||||
TPVV (mL) | 0.31** | 0.33** | 0.34** | 0.34** | 0.36** |
TPVV (%) | 0.13 | 0.13 | 0.13 | 0.13 | 0.33** |
The number of pulmonary vessel branches | 0.29** | 0.30** | 0.31** | 0.32** | 0.36** |
PAV (mL) | 0.32** | 0.34** | 0.35** | 0.36** | 0.41** |
PAV (%) | 0.11 | 0.10 | 0.10 | 0.13 | 0.39** |
The number of pulmonary artery branches | 0.31** | 0.33** | 0.34** | 0.37** | 0.40** |
PVV (mL) | 0.29** | 0.30** | 0.31** | 0.31** | 0.31** |
PVV (%) | 0.15 | 0.15 | 0.15 | 0.15 | 0.28** |
The number of pulmonary vein branches | 0.27** | 0.28** | 0.29** | 0.29** | 0.33** |
*, P<0.05; **, P<0.001. VC%, percentage of predicted vital capacity; FVC%, percentage of predicted forced vital capacity; FEV1%, percentage of forced expiratory volume in 1 second; TLC%, percentage of predicted total lung capacity; DLco%, percentage of predicted diffusing capacity for carbon monoxide. TPVV, total pulmonary vascular volume; PAV, pulmonary artery volume; PVV, pulmonary vein volume; TRBR, traction bronchiectasis; RE, reticular pattern; HC, honeycombing; GGO, ground glass opacity.
Correlation of lesions with vascular-related parameters
Total lesion volume was negatively correlated with both pulmonary vascular volume and the number of vascular branches (r=−0.43 to −0.34, P<0.001) (Figure S2). Particularly, HC (r=−0.48, P<0.001) was in significantly correlated with vascular volume, while TRBR and GGO were not associated with vascular-related indicators (P>0.05). Upper, middle, and lower lung lesions were all negatively related with vessel volume and the number of branches (P<0.05) (Table 4).
Table 4
Characteristics | TPVV (mL) | TPVV (%) | The total number of pulmonary vascular branches | PAV (mL) | PAV (%) | The number of pulmonary artery branches | PVV (mL) | PVV (%) | The number of pulmonary vein branches |
---|---|---|---|---|---|---|---|---|---|
Total lung volume (mL) | 0.53** | 0.01 | 0.47** | 0.59** | −0.01 | 0.549** | 0.45** | 0.02 | 0.38** |
All lesion volume (mL) | −0.26** | −0.35** | −0.26** | −0.26* | −0.36** | −0.29** | −0.26** | −0.33** | −0.26** |
All lesion volume (%) | −0.39** | −0.36** | −0.38** | −0.40** | −0.36** | −0.43** | −0.37** | −0.34** | −0.36** |
HC (%) | −0.39** | −0.48** | −0.41** | −0.32** | −0.43** | −0.31** | −0.42** | −0.47** | −0.44** |
RE (%) | −0.18 | −0.12 | −0.19 | −0.12 | −0.20 | −0.27** | −0.12 | −0.16 | −0.15 |
TRBR (%) | 0.04 | 0.04 | 0.03 | 0 | 0.02 | −0.04 | 0.05 | 0.05 | 0.05 |
GGO (%) | 0.09 | 0.12 | 0.06 | 0.15 | 0.11 | 0.04 | 0.09 | 0.07 | 0.05 |
Right upper lung lesion (%) | −0.30** | −0.26** | −0.33** | −0.27** | −0.22* | −0.35** | −0.31** | −0.27** | −0.33** |
Right middle lung lesion (%) | −0.25* | −0.23* | −0.28** | −0.24* | −0.20* | −0.31** | −0.25* | −0.22* | −0.26** |
Right lower lung lesion (%) | −0.24* | −0.22* | −0.23* | −0.25* | −0.24* | −0.28** | −0.21* | −0.19 | −0.21* |
Left upper lung lesion (%) | −0.30** | −0.19 | −0.28** | −0.29** | −0.17 | −0.32** | −0.29** | −0.21* | −0.26** |
Left middle lunge lesion (%) | −0.36** | −0.25* | −0.34** | −0.40** | −0.26* | −0.42** | −0.33** | −0.23* | −0.29** |
Left lower lung lesion (%) | −0.36** | −0.36** | −0.34** | −0.38** | −0.38* | −0.39** | −0.33** | −0.33** | −0.32** |
*, P<0.05; **, P<0.001. TPVV, total pulmonary vascular volume; PAV, pulmonary artery volume; PVV, pulmonary vein volume; TRBR, traction bronchiectasis; RE, reticular pattern; HC, honeycombing; GGO, ground glass opacity.
Discussion
In this study, we quantitatively measured the volume of CT features, including HC, RE, GGO, TRBR, and pulmonary vessels, and analyzed the correlation of these features with PFTs in patients with IPF. There were several findings in this cohort: (I) the total lesion extent and lesions at different sites all demonstrated significant negative correlations between PTFs and vascular-related parameters; (II) in the sub-lesion analysis, several lesion patterns, including HC, RE, and TRBR, showed negative correlations with PTFs, although GGO did not; and (III) HC and RE were negatively correlated with vascular-related parameters.
As a parameter that best reflects baseline disease severity in IPF, DLco is limited by measurement noise in the range of 5% to 15% (20,21). Accordingly, there is a growing trend to explore other potential markers of IPF with regards to disease severity or progression, such as hematological (22-24) and imaging markers (13,25). The extent of HC determined by quantitative CT analysis is correlated with PFTs and might be an important and independent predictor of mortality in IPF patients with a definite usual interstitial pneumonia (UIP) pattern (12). Quantitative analysis of HRCT by Torrisi et al. (26) indicated that mean lung density, high attenuation areas (HAA%), and fibrotic areas (FA%) were also good predictors of the mortality of patients with IPF. Moreover, total lung volume and lung density qualified on HRCT correlated significantly with FVC as well as DLco and could predict the mortality of patients with CPFE (27,28). We qualified the lung volume of the and the volume ratios of 4 HRCT features with total lung in this study. These measurements considered the characteristics and ranges of different radiological features in the whole lung.
In our work, the median total lesion volume and ratio were 522.42 mL and 12.85%, respectively, whereas detailed analysis of the median volumes of the 4 lesion ratios demonstrated significant differences. The median volume of the HC lesion was 0%, as some patients possibly had the pattern of UIP without accompanying HC changes. It is probable that HC may show a stronger correlation with PFT when the number of patients with the UIP pattern increases. It is not difficult to explain that UIP characterized with predominantly subpleural and basal HC and RE changes is a typical imaging feature of IPF, and the increases in these lesions were shown to be associated with a poorer prognosis of IPF (12,29,30). The total lesion volume ratio negatively correlated with the DLco. The predominance of HC and RE in multiple lesion types showed the strongest correlation with the DLco (HC%, r=−0.45, P<0.001; RE%, r=−0.41, P<0.001), which was consistent with previous research (12,14). The TRBR is often excluded from a majority of quantitative studies as it represents a rather small percentage of lesions. Nevertheless, we identified relatively weak correlations between the TRBR ratio and lung function, as had been previously reported (9), which indicated that TRBR is associated with disease severity and cannot be ignored in future investigations. Analogously, we also triangulated the left and right lungs in the vertical direction to obtain 6 different regions. The lesions in different areas all correlated negatively with lung function but the correlation was weaker than that of total lesion extent.
The role of TPVV in the prognosis of IPF has been reported (9,17). In most studies, increased PVV tends to predict poorer prognosis, partly explained by decreased local pulmonary blood perfusion as a consequence of vascular disruption and remodeling within the fibrotic zone, which may lead to increased pulmonary artery pressure and vessel volume of uninvolved vessels in normal parenchymal areas adjacent to the fibrotic zone (31,32). Inversely, different results appeared to be obtained in our research. A positive correlation was observed between pulmonary vascular and pulmonary function, which was especially more pronounced with the DLco (P<0.001). The pulmonary artery parameters mildly enhanced the functional correlation compared to the total vascular parameters. Homoplastically, pulmonary vascular-related parameters such as pulmonary vessel volume and the number of vascular branches were negatively correlated with lesion extent, of which consistent results were also observed for the extent of the lesion at different sites. The HC still demonstrated the strongest correspondence with pulmonary vascular parameters. This phenomenon may be attributed to the severe destruction of capillary beds due to fibrosis, where compensatory vascular increase is not sufficient to compensate for the deficit of vessels. However, this result also prompted a potential question. The HC and pulmonary vessels are sometimes difficult to clearly delineate on CT because of similar imaging characteristics, and several studies have demonstrated possible errors in the segmentation of HC/RE and pulmonary vessels (21). The same problem also existed in our research, in which HC showed a significant correlation with vessel volume, which was potentially the result of confounding.
There were several limitations to this study. First, the number of patients was limited due to the strict inclusion criteria. However, patients with well-characterized IPF remain scarce even in tertiary care centers, and multicenter collaboration may ameliorate this problem to some extent. Second, in cases of severe fibrosis, TPVV quantification may be confounded by RE, which may reduce the relationship between TPVV and pulmonary function. It is believed that a stronger correlation will be elucidated by algorithm optimization. Finally, our cohort excluded patients who were unable to perform PFTs due to severe disease, and patients, overall, had relatively mild disease; hence, our findings cannot be generalized to those with more severe disease.
Conclusions
Quantitative analysis of HRCT features demonstrated that increased HC and RE exhibit the strongest association decreased DLco in IPF. The pulmonary vascular volumes, especially pulmonary artery volumes, have a strong positive correlation with pulmonary function indicators and are negatively correlated with lesion extent. The covariance between vascular-related parameters and lesion extent as well as pulmonary function indicate that TPVV may be representative of a variable that simultaneously responds to interstitial disease and function.
Acknowledgments
Funding: This work was supported by National Key R&D Program of China (No. 2021YFC2500700 and No. 2016YFC0901101 to HD) and the National Natural Science Foundation of China (No. 81870056 to HD).
Footnote
Reporting Checklist: The authors have completed the STROBE reporting checklist. Available at https://qims.amegroups.com/article/view/10.21037/qims-21-1232/rc
Conflicts of Interest: All authors have completed the ICMJE uniform disclosure form (available at https://qims.amegroups.com/article/view/10.21037/qims-21-1232/coif). HK and RZ are staff of Advanced Research, Infervision Medical Technology. The school/hospital and the company are in a collaborative relationship. The other authors have no conflicts of interest to declare.
Ethical Statement: The authors are accountable for all aspects of the work in ensuring that questions related to the accuracy or integrity of any part of the work are appropriately investigated and resolved. The study was conducted in accordance with the Declaration of Helsinki (as revised in 2013). The study was approved by Ethics Board of China-Japan Friendship Hospital Committee (No. 2017-25) and individual consent for this retrospective analysis was waived.
Open Access Statement: This is an Open Access article distributed in accordance with the Creative Commons Attribution-NonCommercial-NoDerivs 4.0 International License (CC BY-NC-ND 4.0), which permits the non-commercial replication and distribution of the article with the strict proviso that no changes or edits are made and the original work is properly cited (including links to both the formal publication through the relevant DOI and the license). See: https://creativecommons.org/licenses/by-nc-nd/4.0/.
References
- Hutchinson J, Fogarty A, Hubbard R, McKeever T. Global incidence and mortality of idiopathic pulmonary fibrosis: a systematic review. Eur Respir J 2015;46:795-806. [Crossref] [PubMed]
- Harari S, Madotto F, Caminati A, Conti S, Cesana G. Epidemiology of Idiopathic Pulmonary Fibrosis in Northern Italy. PLoS One 2016;11:e0147072. [Crossref] [PubMed]
- Schwartz DA, Helmers RA, Galvin JR, Van Fossen DS, Frees KL, Dayton CS, Burmeister LF, Hunninghake GW. Determinants of survival in idiopathic pulmonary fibrosis. Am J Respir Crit Care Med 1994;149:450-4. [Crossref] [PubMed]
- Nathan SD, Shlobin OA, Weir N, Ahmad S, Kaldjob JM, Battle E, Sheridan MJ, du Bois RM. Long-term course and prognosis of idiopathic pulmonary fibrosis in the new millennium. Chest 2011;140:221-9. [Crossref] [PubMed]
- Jacob J, Bartholmai BJ, Rajagopalan S, Kokosi M, Egashira R, Brun AL, Nair A, Walsh SLF, Karwoski R, Wells AU. Serial automated quantitative CT analysis in idiopathic pulmonary fibrosis: functional correlations and comparison with changes in visual CT scores. Eur Radiol 2018;28:1318-27. [Crossref] [PubMed]
- Raghu G, Collard HR, Egan JJ, Martinez FJ, Behr J, Brown KK, et al. An official ATS/ERS/JRS/ALAT statement: idiopathic pulmonary fibrosis: evidence-based guidelines for diagnosis and management. Am J Respir Crit Care Med 2011;183:788-824. [Crossref] [PubMed]
- Hobbs S, Chung JH, Leb J, Kaproth-Joslin K, Lynch DA. Practical Imaging Interpretation in Patients Suspected of Having Idiopathic Pulmonary Fibrosis: Official Recommendations from the Radiology Working Group of the Pulmonary Fibrosis Foundation. Radiol Cardiothorac Imaging 2021;3:e200279. [Crossref] [PubMed]
- Lynch DA, Godwin JD, Safrin S, Starko KM, Hormel P, Brown KK, Raghu G, King TE Jr, Bradford WZ, Schwartz DA, Richard Webb WIdiopathic Pulmonary Fibrosis Study Group. High-resolution computed tomography in idiopathic pulmonary fibrosis: diagnosis and prognosis. Am J Respir Crit Care Med 2005;172:488-93. [Crossref] [PubMed]
- Jacob J, Bartholmai BJ, Rajagopalan S, Kokosi M, Nair A, Karwoski R, Walsh SL, Wells AU, Hansell DM. Mortality prediction in idiopathic pulmonary fibrosis: evaluation of computer-based CT analysis with conventional severity measures. Eur Respir J 2017;49:1601011. [Crossref] [PubMed]
- Clukers J, Lanclus M, Mignot B, Van Holsbeke C, Roseman J, Porter S, Gorina E, Kouchakji E, Lipson KE, De Backer W, De Backer J. Quantitative CT analysis using functional imaging is superior in describing disease progression in idiopathic pulmonary fibrosis compared to forced vital capacity. Respir Res 2018;19:213. [Crossref] [PubMed]
- Iwasawa T, Kanauchi T, Hoshi T, Ogura T, Baba T, Gotoh T, Oba MS. Multicenter study of quantitative computed tomography analysis using a computer-aided three-dimensional system in patients with idiopathic pulmonary fibrosis. Jpn J Radiol 2016;34:16-27. [Crossref] [PubMed]
- Nakagawa H, Ogawa E, Fukunaga K, Kinose D, Yamaguchi M, Nagao T, Tanaka-Mizuno S, Nakano Y. Quantitative CT analysis of honeycombing area predicts mortality in idiopathic pulmonary fibrosis with definite usual interstitial pneumonia pattern: A retrospective cohort study. PLoS One 2019;14:e0214278. [Crossref] [PubMed]
- Kim GHJ, Weigt SS, Belperio JA, Brown MS, Shi Y, Lai JH, Goldin JG. Prediction of idiopathic pulmonary fibrosis progression using early quantitative changes on CT imaging for a short term of clinical 18-24-month follow-ups. Eur Radiol 2020;30:726-34. [Crossref] [PubMed]
- Nakagawa H, Nagatani Y, Takahashi M, Ogawa E, Tho NV, Ryujin Y, Nagao T, Nakano Y. Quantitative CT analysis of honeycombing area in idiopathic pulmonary fibrosis: Correlations with pulmonary function tests. Eur J Radiol 2016;85:125-30. [Crossref] [PubMed]
- Shen C, Yu N, Wen L, Zhou S, Dong F, Liu M, Guo Y. Risk stratification of acute pulmonary embolism based on the clot volume and right ventricular dysfunction on CT pulmonary angiography. Clin Respir J 2019;13:674-82. [Crossref] [PubMed]
- Liu W, Liu M, Guo X, Zhang P, Zhang L, Zhang R, Kang H, Zhai Z, Tao X, Wan J, Xie S. Evaluation of acute pulmonary embolism and clot burden on CTPA with deep learning. Eur Radiol 2020;30:3567-75. [Crossref] [PubMed]
- Jacob J, Pienn M, Payer C, Urschler M, Kokosi M, Devaraj A, Wells AU, Olschewski H. Quantitative CT-derived vessel metrics in idiopathic pulmonary fibrosis: A structure-function study. Respirology 2019;24:445-52. [Crossref] [PubMed]
- Jacob J, Bartholmai BJ, Rajagopalan S, van Moorsel CHM, van Es HW, van Beek FT, et al. Predicting Outcomes in Idiopathic Pulmonary Fibrosis Using Automated Computed Tomographic Analysis. Am J Respir Crit Care Med 2018;198:767-76. [Crossref] [PubMed]
- Sun X, Meng X, Zhang P, Wang L, Ren Y, Xu G, Yang T, Liu M. Quantification of pulmonary vessel volumes on low-dose computed tomography in a healthy male Chinese population: the effects of aging and smoking. Quant Imaging Med Surg 2022;12:406-16. [Crossref] [PubMed]
- Hathaway EH, Tashkin DP, Simmons MS. Intraindividual variability in serial measurements of DLCO and alveolar volume over one year in eight healthy subjects using three independent measuring systems. Am Rev Respir Dis 1989;140:1818-22. [Crossref] [PubMed]
- Jacob J, Bartholmai BJ, Rajagopalan S, Kokosi M, Nair A, Karwoski R, Raghunath SM, Walsh SL, Wells AU, Hansell DM. Automated Quantitative Computed Tomography Versus Visual Computed Tomography Scoring in Idiopathic Pulmonary Fibrosis: Validation Against Pulmonary Function. J Thorac Imaging 2016;31:304-11. [Crossref] [PubMed]
- Kass DJ, Nouraie M, Glassberg MK, Ramreddy N, Fernandez K, Harlow L, Zhang Y, Chen J, Kerr GS, Reimold AM, England BR, Mikuls TR, Gibson KF, Dellaripa PF, Rosas IO, Oddis CV, Ascherman DP. Comparative Profiling of Serum Protein Biomarkers in Rheumatoid Arthritis-Associated Interstitial Lung Disease and Idiopathic Pulmonary Fibrosis. Arthritis Rheumatol 2020;72:409-19. [Crossref] [PubMed]
- Adegunsoye A, Alqalyoobi S, Linderholm A, Bowman WS, Lee CT, Pugashetti JV, Sarma N, Ma SF, Haczku A, Sperling A, Strek ME, Noth I, Oldham JM. Circulating Plasma Biomarkers of Survival in Antifibrotic-Treated Patients With Idiopathic Pulmonary Fibrosis. Chest 2020;158:1526-34. [Crossref] [PubMed]
- Guo L, Yang Y, Liu F, Jiang C, Yang Y, Pu H, Li W, Zhong Z. Clinical Research on Prognostic Evaluation of Subjects With IPF by Peripheral Blood Biomarkers, Quantitative Imaging Characteristics and Pulmonary Function Parameters. Arch Bronconeumol 2020;56:365-72. (Engl Ed). [PubMed]
- Fraioli F, Lyasheva M, Porter JC, Bomanji J, Shortman RI, Endozo R, Wan S, Bertoletti L, Machado M, Ganeshan B, Win T, Groves AM. Synergistic application of pulmonary 18F-FDG PET/HRCT and computer-based CT analysis with conventional severity measures to refine current risk stratification in idiopathic pulmonary fibrosis (IPF). Eur J Nucl Med Mol Imaging 2019;46:2023-31. [Crossref] [PubMed]
- Torrisi SE, Palmucci S, Stefano A, Russo G, Torcitto AG, Falsaperla D, Gioè M, Pavone M, Vancheri A, Sambataro G, Sambataro D, Mauro LA, Grassedonio E, Basile A, Vancheri C. Assessment of survival in patients with idiopathic pulmonary fibrosis using quantitative HRCT indexes. Multidiscip Respir Med 2018;13:43. [Crossref] [PubMed]
- Nemoto M, Nei Y, Bartholmai B, Yoshida K, Matsui H, Nakashita T, Motojima S, Aoshima M, Ryu JH. Automated computed tomography quantification of fibrosis predicts prognosis in combined pulmonary fibrosis and emphysema in a real-world setting: a single-centre, retrospective study. Respir Res 2020;21:275. [Crossref] [PubMed]
- Üçsular F, Karadeniz G, Polat G, Yalnız E, Ayrancı A, Çinkooğlu A, Savaş R, Solmaz H, Güldaval F, Büyükşirin M. Quantitative CT in mortality prediction in pulmonary fibrosis with or without emphysema. Sarcoidosis Vasc Diffuse Lung Dis 2021;38:e2021024. [PubMed]
- Handa T, Tanizawa K, Oguma T, Uozumi R, Watanabe K, Tanabe N, Niwamoto T, Shima H, Mori R, Nobashi TW, Sakamoto R, Kubo T, Kurosaki A, Kishi K, Nakamoto Y, Hirai T. Novel Artificial Intelligence-based Technology for Chest Computed Tomography Analysis of Idiopathic Pulmonary Fibrosis. Ann Am Thorac Soc 2022;19:399-406. [Crossref] [PubMed]
- Romei C, Tavanti LM, Taliani A, De Liperi A, Karwoski R, Celi A, Palla A, Bartholmai BJ, Falaschi F. Automated Computed Tomography analysis in the assessment of Idiopathic Pulmonary Fibrosis severity and progression. Eur J Radiol 2020;124:108852. [Crossref] [PubMed]
- Renzoni EA, Walsh DA, Salmon M, Wells AU, Sestini P, Nicholson AG, Veeraraghavan S, Bishop AE, Romanska HM, Pantelidis P, Black CM, Du Bois RM. Interstitial vascularity in fibrosing alveolitis. Am J Respir Crit Care Med 2003;167:438-43. [Crossref] [PubMed]
- Cosgrove GP, Brown KK, Schiemann WP, Serls AE, Parr JE, Geraci MW, Schwarz MI, Cool CD, Worthen GS. Pigment epithelium-derived factor in idiopathic pulmonary fibrosis: a role in aberrant angiogenesis. Am J Respir Crit Care Med 2004;170:242-51. [Crossref] [PubMed]