Rich-club reorganization of functional brain networks in acute mild traumatic brain injury with cognitive impairment
Introduction
Traumatic brain injury (TBI), including concussion, poses an immense public health burden; in particular, mild traumatic brain injury (mTBI) accounts for close to 90% of all brain injuries (1). Although deemed “mild”, concussed patients remain symptomatic (characterized by physical, emotional, cognitive, and sleep disturbances) for more than 2 weeks in 50% of cases and up to several months in 5–20% of cases after the initial injury (2). Several neuropsychological studies have reported reduced cognitive efficiency in patients with mTBI, especially in processing speed, executive function, connectivity, attention, and memory (3,4). However, to date, the neurobiological mechanism of post-mTBI cognitive impairment remains poorly understood. Meanwhile, clinicians have few objective tools to guide the diagnosis and management of cognitive impairment following mTBI. Therefore, there is increasing demand for the application of neuroimaging to better understand the mechanisms of cognitive impairment on a neurobiological level and to improve patient monitoring.
A key component in the architecture of the brain network is a robust hub structure in which the hub nodes integrate efficient information via utilization of highly connected and functional centers (5). Destruction or abnormal formation in this central system can cause impaired information processing and integration, and ultimately lead to brain dysfunction, through impaired connectivity or network (6). An mTBI, which is typically characterized by temporally limited emotional symptoms and cognitive impairment, is regarded as a brain connectome disorder (7). Functional magnetic resonance imaging (fMRI) studies have provided extensive evidence for impaired brain structure and functional connectivity (FC) in patients with mTBI and TBI (8,9). FC abnormalities have commonly been detected in regions within the attention, default mode, executive, salience, and somatosensory networks in the different timing of data acquisition after mTBI (10-12). Different brain regions and networks have their own tasks and functions. For example, default mode networks (DMN) are known to support several key cognitive functions, such as memory encoding, environmental monitoring, consolidation, self-relevance, and rapid error recognition (13). These higher-order cognitive functions are often disrupted in mTBI patients (10). A study found that decreased network connectivity between the left thalamus and left middle frontal gyrus (MFG) was correlated with measures of cognition (working memory) in patients with mTBI (14). Another study revealed a significant negative relationship between the dorsal attentional network and behavioral symptoms score in mTBI patients (15). In addition, the brain network can be modeled using graphical theory as functional interactions consisting of nodes and edges. Recent graph analytical studies, which examined the brain network topology, have shown increased connection distance and reduced efficiency in mTBI (16-18).
The brain network also has a high level of organization named the “rich-club”, where synergistic hubs work to connect different functional systems in the brain (19,20). The “rich-club phenomenon” may be of particular interest due to its significant impact on cognitive function and critical role in dominating network topology and influencing dynamic integration and communication among network elements (21). The rich-club organization described in the human connectome is a high-capacity central core that comprises a set of regions (22) which are suggested to form an information backbone; it is crucial for brain function and vulnerable to pathogenic agents of disease (23). Consequently, rich-club organization has been studied in several brain disorders, including Alzheimer’s disease (24,25), schizophrenia (26), insomnia (27), and stroke (28). Antonakakis et al. (18) identified with magnetoencephalography (MEG) that patients with mTBI demonstrate hyper-synchronization among rich-club regions compared with healthy controls (HCs) in the δ band and the δ-γ1, θ-γ1, and β-γ2 frequency pairs. Hillary et al.’s (29) findings generally support a hyperconnectivity hypothesis, and this alteration is disproportionately represented in brain regions belonging to the brain’s core subnetworks. However, very few studies have discussed the rich-club organization of brain network disruption in mTBI based on resting-state (rs) fMRI data. Meanwhile, how rich-club organization and network topology are altered in acute mTBI and the relationship with underlying cognitive impairment are also largely unknown, highlighting the need for exploration of this research area.
On the basis of theoretical and empirical work regarding the influence of mTBI on network function, we predicted that mTBI may induce cognitive impairment-related network disturbances, and cognitive impairment would be linked to disturbed rich-club organization or network topological properties. Here, a graph theoretical approach was used to assess the underlying rich-club organization and network topological properties in acute mTBI. Subsequently, we examined whether the functional rich-club organization and network properties were related to cognitive impairment after mTBI to improve mechanistic understandings of cognitive impairment manifestations in mTBI. We present the following article in accordance with the TREND reporting checklist (available at https://qims.amegroups.com/article/view/10.21037/qims-21-915/rc).
Methods
Participants
We recruited 88 patients with acute mTBI (aged 40.16±11.25 years) and 85 matched HCs (aged 39.22±12.07 years) to this study. Among them, 88 mTBI patients were included from the Department of Emergency, Nanjing First Hospital, Nanjing Medical University, and 85 HCs were recruited from the local community. The patients with mTBI were required to meet the following criteria: (I) age (18–60 years); (II) a closed head injury occurring within 7 days of recruitment with either posttraumatic amnesia of less than 24 hours or loss of consciousness of no more than 30 minutes, or with any alteration in mental state (i.e., dazed, disoriented, and confused); and (III) a Glasgow coma scale (GCS) score of between 13 and 15 on presentation to the emergency department. Furthermore, the exclusion criteria for all participants included the following: (I) existence of prior brain injury or other neurological disease that would affect the study results; (II) history of drug or alcohol abuse; (III) MRI contraindications; (IV) abnormal visual acuity or corrected visual acuity; and (V) poor quality of the imaging data. Given the emergency care setting, a complete neuropsychological assessment was not feasible. All participants underwent standardized clinical neuropsychological evaluations via the Beijing version of Montreal Cognitive Assessment (MoCA), which assessed visuospatial, language, naming, attention, memory, localization, and abstraction (30). The major mechanism of trauma among patients was traffic accidents [40 of 88 patients (45.4%)], followed by fall from variable heights [27 of 88 male patients (30.6%)], and assault [21 of 88 patients (23.8%)]. In addition, no patients had loss of consciousness and retrograde or anterograde amnesia.
MRI acquisition
All MRI scans were performed on a 3.0 T MRI scanner (Ingenia, Philips Medical Systems, Best, Netherlands) using a standard 8-channel digital head coil at Nanjing First Hospital within 7 days (3.16±1.83 days) of injury. The rs-fMRI was acquired axially using a gradient echo-planar imaging (EPI) sequence and scanning parameters were as follows: repetition time (TR) =2,000 ms; echo time (TE) =30 ms; slices =36; thickness =4 mm; gap =0 mm; field of view (FOV) =240 mm × 240 mm; matrix =64×64; and flip angle (FA) =90°. The anatomical T1-weighted MR images were obtained by a 3D turbo fast echo (3D-TFE) T1-weighted sequence with the following parameters: TR =8.1 ms; TE =3.7 ms; thickness =1 mm; slices =170; FA =8°; FOV =256 mm × 256 mm; gap =0 mm; and matrix =256×256. The susceptibility-weighted imaging (SWI) used a 3D gradient echo (GRE) sequence, and the specifications were as follows: TR/TE =22/34 ms; slice thickness =1 mm; FOV =220 mm × 220 mm; and matrix =276×319. The sequences of fluid-attenuated inversion recovery (FLAIR) were as follows: TR =7,000 ms; TE =120 ms; slices =18; gap =1.3 mm; slice thickness =6 mm; FA =110°; and voxel size =0.65 mm × 0.95 mm × 6 mm. Both SWI and FLAIR were used to investigate the presence of traumatic lesions.
Image preprocessing
The preprocessing steps were conducted with Statistical Parametric Mapping 12 (SPM12; The Wellcome Center for Human Neuroimaging, UCL Queen Square Institute of Neurology, London, UK) and resting-state data processing and analysis for brain imaging (DPABI) (31). First, the initial 10 scans were removed before preprocessing. Second, functional images were corrected for slice-timing by using slice interpolation to interpolate the voxel time, and head motion correction was performed. According to the criteria of spatial movement, participants with head motion >2.0° of rotation or 2.0 mm of translation in any direction were removed (32). Third, the fMRI images were spatially co-registered with their anatomical T1 image and then resampled to 3×3×3 mm3 voxels (33). To avoid inflation of local connectivity and clustering, no spatial smoothing was applied in this study (34). Linear detrending and bandpass filtering (0.01–0.1 Hz) were carried out to diminish the effects of physiological noise of high-frequency components and low-frequency drift. All regions of interest (ROIs)/participants were checked for signal dropouts, and there was no loss of signal detected.
Functional network construction
Within this study, we established the brain functional network based on the Dos-160 template (including 160 ROIs) (35). A total of 160 ROIs of the Dos-160 template were classified into 6 subnetworks (36,37), which included the DMN, cingulo-opercular network (CON), fronto-parietal network (FPN), occipital network (ON), sensorimotor network (SMN), and cerebellum network (CN). These 6 modules are shown in Figure S1. First, we calculated the mean blood-oxygen-level-dependent (BOLD) signal time series for each ROI from the Dos-160 template (spherical radius of 5 mm) by averaging the time courses of all the voxels within the ROIs. Second, we calculated Pearson’s linear correlation (only looking at positive correlations) between average time series for each ROI. A 160×160 symmetric correlation matrix and corresponding P value matrix for each participant were computed, and Fisher-z transformation was used for the symmetric correlation matrix.
Rich-club organization
The GRaph thEoreTical Network Analysis (GRETNA) toolbox (https://www.nitrc.org/projects/gretna) was used for network analyses (37). The rich-club coefficient was calculated using Brain Connectivity Toolbox (http://www.brain-connectivity-toolbox.net). To exclude weak or spurious connections, thresholds were applied to the connectivity matrices by network sparsity following the previous studies of the functional brain network (33,38). We calculated the different network sparsity values as 10%, 15%, 20%, and 25%. The cost of 15% is shown in the main text, while the other costs (10%, 20%, and 25%) are shown in the Supplementary Materials (Appendix 1). In summary, the rich-club organization of a network is characterized by a high degree of above-average connectivity between the nodes of the network. If a rich-club is present, high-degree (“rich”) nodes of the network will be closely connected, forming a closely connected core or “club” of nodes—the rich-club. In this study, rich-club organization based on the group-averaged network was analyzed in the binary functional network. We calculated the “rich-club coefficient”, which refers to the density of connections between rich-club nodes, and is expressed as (19):
where k is the degree that represents the number of connections from a given node to other nodes in the network; N>k means the number of remaining nodes; and E>k is the number of remaining connections after removal of the nodes with connections less than k (23). The rich-club coefficients Φ (k) were computed for each participant based on the group mean networks of each group, and normalized relative to a set of 1,000 comparable random networks, resulting in a normalized rich-club coefficient Φnorm(k) (only looking at positive values) (39,40). A Φnorm(k) >1 over a range of k is indicative of a rich-club organization within a brain network (41).
Definition of nodes and connections
In this study, the top 19 (12%) brain regions with the highest degree (unweighted) averaged across HCs based on the group-average cortical network were chosen as rich-club regions (42,43). The nodes were classified into “rich-club” nodes and “peripheral” (i.e., non-rich-club) nodes. Meanwhile, the classification of rich-club nodes allowed for the categorization of the edges into 3 connection classes: “rich-club connections”, which linked 2 rich-club nodes; “feeder connections”, which linked 1 rich-club node to 1 peripheral node; and “local connections”, which linked 2 peripheral nodes (34). The connectivity strength of “rich-club”, “local”, and “feeder” was computed as the sum of the edge weights for each connection.
Network topological metrics
Network metrics and the results were calculated using the GRETNA toolbox (37) and BrainNet Viewer toolbox (http://www.nitrc.org/projects/bnv) (44). To exclude weak or spurious connections, the threshold range of network sparsity was identified as 0.05–0.50 with an increment of 0.01 based on the previous graph-based study of the functional brain network (45). Only the positive relationships were considered. To avoid the selection of a specific threshold, we adopted the area under the curve (AUC) method, which is widely used in graph theory-based network research (46). For each network topological metric, the AUC was calculated within a defined threshold range based on rs-fMRI data. Selection of specific global and local measures was based on previous literature and review (47,48). Global metrics included the following: global efficiency (Eg), reflecting the efficiency for network-wide communication; mean local efficiency (Eloc), reflecting the mean efficiency of information propagation through a node’s direct neighbors; mean clustering coefficient (Cp), reflecting the average likelihood that the neighbors of a node are interconnected; mean shortest path length (Lp), reflecting the mean minimal travel distance between nodes; and small-world properties. In addition, nodal network graph metrics included nodal clustering coefficient (Ci) and nodal local efficiency (Eloci), reflecting the inverse of the characteristic Lp between the pair of nodes.
Statistical analysis
The software SPSS 20.0 (IBM Corp., Armonk, NY, USA) and the GRETNA toolbox were used for statistical analyses. Age and education were evaluated using an independent t-test, and the gender was evaluated using the chi-square test. A 2-sample t-test was used to test for group differences in neuropsychological scores. A 1-sample t-test (Bonferroni correction) was applied at each level of k to test whether the normalized rich-club coefficients exceed 1 and to probe the existence of the rich-club organization. Bonferroni correction was used to calibrate multiple tests across all test levels of k (49). We used the 2-sample t-test (age, gender, and education as covariates) to test group differences in rich-club coefficients, nodal efficiency, and nodal cluster coefficients with Bonferroni corrections for multiple comparisons. The between-group differences in 3 classes of connectivity density and other network topology metrics were assessed by the 2-sample t-test (age, gender, and education as covariates). Spearman’s rank correlation (controlled for age, gender, and education) was used to assess the relationship among rich-club connection, graph metrics, and cognitive functional performance in the mTBI group (Bonferroni correction). Statistical significance was considered when P<0.05.
Ethical statement
The study was conducted in accordance with the Declaration of Helsinki (as revised in 2013). The current study was approved by the Institutional Review Board of Nanjing Medical University. Written informed consent was provided by all participants before their participation in the study protocol.
Results
Demographic and neuropsychological characteristics
The demographic characteristics and neuropsychological performance of the 2 groups are presented in Table 1. No significant group differences were found in age, gender, and education (P>0.05). Compared with HC, the score of the MoCA scale (P<0.001) was lower in the patients with acute mTBI. For cognitive variables, the patients with mTBI performed significantly worse than HCs on visuospatial/executive (P<0.001), attention (P=0.002), and memory (P=0.005). No significant differences in other cognitive performances were observed between the mTBI group and HCs group in this study. In addition, all patients showed no MRI/computed tomography (CT) abnormalities on the FLAIR or SWI scan.
Table 1
Characteristics | mTBI (n=88) | Controls (n=85) | P value |
---|---|---|---|
Age (years) | 40.16±11.25 | 39.22±12.07 | 0.599 |
Education (years) | 12.76±3.05 | 13.49±3.57 | 0.149 |
Gender (female/male) | 45/43 | 47/38 | 0.648 |
MoCA scores | 24.26±2.47 | 26.32±1.91 | <0.001* |
Visuospatial/executive | 3.48±1.10 | 4.26±0.84 | <0.001* |
Language | 2.39±0.61 | 2.53±0.60 | 0.126 |
Attention | 5.42±0.88 | 5.76±0.53 | 0.002* |
Naming | 2.85±0.38 | 2.85±0.36 | 0.927 |
Memory | 2.53±1.23 | 3.11±1.39 | 0.005* |
Abstraction | 1.76±0.52 | 1.86±0.38 | 0.166 |
Orientation | 5.69±0.49 | 5.75±0.65 | 0.495 |
Data are represented as mean ± SD. *, P<0.05. mTBI, mild traumatic brain injury; MoCA, Montreal Cognitive Assessment.
Rich-club organization and reorganization
The increasing rich-club coefficient (Φnorm) >1 reflects the existence of the rich-club organization in the brain network over a certain range of degrees (k). In our study, for the FC networks, the rich-club organization was evident in both acute mTBI and HCs with normalized rich-club coefficient (Φnorm) increasing higher than 1 as a function of node degree (k) (1<k<45). Figure 1A,1B shows Φnorm and Φ as a function of node degree (k) for HCs (blue) and mTBI groups (red), respectively. Inter-connected brain regions dropped off more readily in acute mTBI than HCs. In our results, Φ was significantly higher in mTBI groups relative to HCs at the range of k=20 to k=45 (P<0.05) but especially at high-degree k-levels where k=25–39 and 40–45 (P<0.01). In the whole-brain network, compared with the HCs group, Φnorm was found to be significantly higher in the mTBI group at the range of k=23 to k=44 (P<0.01; 1,000 permutations). Notably, these findings were supported by rich-club organization analyses under different network connection density threshold levels, and the results were consistent with our main findings [Figures S2-S4; see Supplementary Materials for rich-club organization results (Appendix 1)].
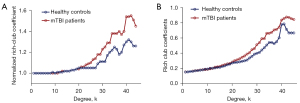
The functional rich-club regions were set at the top 19 (12%) highest-degree nodes [Figure 2A (red nodes) and Table 2] (aDC, means the sum of AUC of average node degree for different sparsity threshold levels within the defined threshold range), comprising the bilateral precuneus, bilateral occipital gyrus, bilateral post occipital gyrus, left anterior cingulate cortex (L-ACC), medial frontal cortex (mFC), left temporoparietal junction (L-TPJ), supplementary motor area (SMA), L-temporal lobe, L-inferior cerebellum, L-lateral cerebellum, and L-parietal lobe. The remaining regions were recognized as peripheral regions (Figure 2A, gray nodes). The distribution of rich-club regions in the brain’s intrinsic functional networks is shown in Figure 2B. The rich-club nodes were distributed in the DMN (10.53%), FPN (5.26%), CON (15.79%), SMN (10.53%), ON (42.11%), and CN (15.79%).
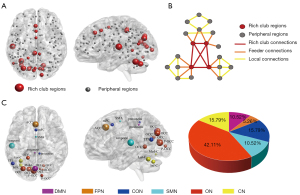
Table 2
Labels | Regions | Hemisphere | Subnetwork | Peak MNI coordinates x, y, z (mm) | aDC |
---|---|---|---|---|---|
111 | Temporal | L | Sensorimotor | −54, −22, 9 | 36.380 |
133 | Occipital | R | Occipital | 15, −77, 32 | 34.915 |
87 | TPJ | L | Cingulo-opercular | −52, −63,15 | 33.835 |
153 | Inf-cerebellum | L | Cerebellum | −34, −67, −29 | 33.590 |
146 | Lat-cerebellum | L | Cerebellum | −34, −57, −24 | 32.950 |
135 | Post-occipital | L | Occipital | −5, −80, 9 | 32.815 |
140 | Post-occipital | R | Occipital | 13, −91, 2 | 31.675 |
130 | Occipital | L | Occipital | −29, −75, 28 | 31.025 |
154 | Med-cerebellum | L | Cerebellum | −11, −72, −14 | 30.720 |
131 | Occipital | L | Occipital | −16, −76, 14 | 30.705 |
33 | Occipital | L | Default | −2, −75, 32 | 30.320 |
127 | Occipital | R | Occipital | 17, −68, 20 | 29.490 |
64 | mFC | – | Cingulo-opercular | 0, 15, 45 | 29.400 |
93 | SMA | – | Sensorimotor | 0, −1, 52 | 29.105 |
16 | Precuneus | L | Default | −3, −38, 45 | 28.860 |
132 | Occipital | R | Occipital | 9, −76, 14 | 28.855 |
80 | Precuneus | R | Cingulo-opercular | 8, −40, 50 | 28.660 |
41 | ACC | L | Fronto-parietal | −1, 28, 40 | 28.655 |
121 | Occipital | L | Occipital | −18, −50, 1 | 28.585 |
aDC, the sum of area under the cure of average node degree for different sparsity threshold levels within the defined threshold range. MNI, Montreal Neurological Institute; TPJ, temporoparietal junction; mFC, medial frontal cortex; SMA, supplementary motor area; ACC, anterior cingulate cortex; L, left; R, right.
Selection of the rich-club and non-rich-club regions allowed for classification of the connections into different types: rich-club connections between rich-club nodes; feeder connections between rich-club nodes and peripheral nodes; and local connections between peripheral nodes. Figure 2C shows a schematic representation of 3 types of connections and 2 classes of nodes. Compared with the HCs groups, the patients with acute mTBI showed a significant increase in the FC strength of rich-club connections (P=0.01), local connections (P=0.006), and feeder connections (P=0.01) (Figure 3A). In patients with mTBI, the visuospatial/executive performance was significantly negatively correlated with local connectivity (rho=−0.289; P=0.007). A similar association was found between MoCA scores and rich-club connectivity (rho=−0.349; P=0.001) (Figure 3B).
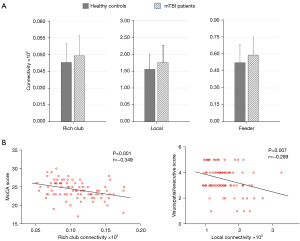
Network topological metrics
The destruction of the brain network’s topological organization may lead to changes in brain information transmission efficiency (25). Globally, group comparison showed that the mean Eloc (P=0.015), whole-brain mean Cp (P=0.003), and mean Lp (P=0.005) significantly increased in patients with acute mTBI relative to the controls. In addition, a significant reduction in Eg (P=0.007) was observed in the acute mTBI group compared with the HCs group (age, gender, and education as covariates; Figure 4A). Positive linear relationships between Eg and attention performance (rho=0.319; P=0.003), as well as the visuospatial/executive score (rho=0.272; P=0.011) of functional connectomes, were observed (Figure 4B). Specifically, in our study, the brain functional networks in the mTBI and HCs groups showed small-world properties, but no group difference was found for the value of global small-world properties (P>0.05).
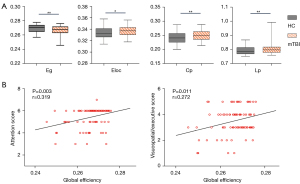
For the local topological properties of the FC networks in the patients with mTBI, the nodal efficiency significantly increased in the patients with mTBI compared with that in the HCs group (P<0.05, Bonferroni corrected; Figure 5A). No significant difference was found in the Ci between the mTBI and HCs groups (P>0.05). In addition, in the mTBI group, 13 abnormal regions in nodal efficiency were observed compared with the HCs group, including 3 rich-club regions, namely, L_occipital (ON), L_inferior cerebellum (CN), and R_precuneus (DMN), and 10 non-rich-club regions, which were located in the frontal lobe (4 nodes), temporal lobe (2 nodes), insular lobe (1 node), limbic lobe (1 node), and cerebellum lobe (2 nodes) (Figure 5B; Table 3).
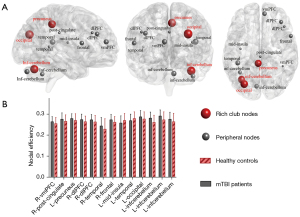
Table 3
Labels | Regions | Hemisphere | Subnetwork | Peak MNI coordinates x, y, z (mm) | P value |
---|---|---|---|---|---|
4 | vmPFC | R | Default | 9, 51, 16 | 0.018 |
14 | Post cingulate | R | Default | 1, −26, 31 | 0.036 |
16 | Precuneus | L | Default | −3, −38, 45 | 0.038# |
40 | dlPFC | R | Fronto-parietal | 40, 36, 29 | 0.012 |
42 | dlPFC | R | Fronto-parietal | 46, 28, 31 | 0.022 |
82 | Temporal | R | Cingulo-opercular | 43, −43, 8 | 0.028 |
88 | Frontal | R | Sensorimotor | 58, 11, 14 | 0.005 |
104 | Mid-insula | L | Sensorimotor | −36, −12, 15 | 0.023 |
118 | Temporal | L | Sensorimotor | −41, −37, 16 | 0.031 |
130 | Occipital | L | Occipital | −29, −75, 28 | 0.040# |
145 | Inf cerebellum | L | Cerebellum | −37, −54, −37 | <0.001 |
153 | Inf cerebellum | L | Cerebellum | −34, −67, −29 | <0.001# |
159 | Inf cerebellum | L | Cerebellum | −6, −79, −33 | 0.038 |
#, rich club nodes. mTBI, mild traumatic brain injury; MNI, Montreal Neurological Institute; vmPFC, ventromedial prefrontal cortex; dlPFC, dorsolateral prefrontal cortex; Inf, inferior; L, left; R, right.
In the patients with acute mTBI, the normalized rich-club coefficient (k=23) was significantly negatively correlated with visuospatial/executive performance (rho=−0.475; P=0.03). No significant relationships with other cognitive performance were observed. For the rich-club coefficient in patients with mTBI, this metric did not show any significant correlations with cognitive performance (P>0.05). Moreover, no significant correlations were observed between any cognitive performance and the feeder connectivity or Eloc (P>0.05) (Table 4).
Table 4
Covariates: age, gender, education | Values | MoCA | Visuospatial/executive | Memory | Attention |
---|---|---|---|---|---|
Rich-club connectivity | r | −0.349# | −0.164 | 0.013 | −0.121 |
P | 0.001**# | 0.128 | 0.901 | 0.263 | |
Feeder connectivity | r | 0.083 | −0.181 | 0.038 | −0.130 |
P | 0.083 | 0.093 | 0.727 | 0.232 | |
Local connectivity | r | −0.210 | −0.289# | −0.083 | −0.166 |
P | 0.050 | 0.007**# | 0.444 | 0.124 | |
Global efficiency | r | −0.204 | 0.272# | −0.198 | 0.319# |
P | 0.059 | 0.011*# | 0.066 | 0.003**# | |
Local efficiency | r | −0.069 | −0.096 | 0.156 | −0.097 |
P | 0.526 | 0.378 | 0.150 | 0.374 |
The star-labeled numbers represent significant correlations (*, P<0.05; **, P<0.01). #, indicated significant correlation (P<0.05). MoCA, Montreal Cognitive Assessment.
Discussion
The main finding of this study was that patients with acute mTBI had relatively higher resting-state functional rich-club connectivity than HCs. Peripheral region connectivity and network topological metrics were also significantly influenced in patient groups. Another main observation was that the changes in rich-club connectivity and network topological metrics were associated with cognitive impairment in patients with acute mTBI.
Rich-club organization is a key attribute of the brain network, in which highly concentrated centers form a tightly connected core. To date, no research group has assessed the rich-club coefficient within fMRI networks in acute mTBI. In the present study, we observed that both acute mTBI and HCs networks possessed rich-club organization characters, and mTBI patients had increased Φnorm, mostly in the high-degree regime. Therefore, the high-degree k-value regions may be more affected in patients with mTBI than in HCs. However, the rich-club coefficient varies among different groups. This finding was important because it suggests that the network information in the acute mTBI brain network is quantitative. Moreover, this work also reported a group of 19 strongly interconnected rich-club nodes, which are predominantly located in the ON, CON, DMN, FPN, and CN. They are associated with vision, language, cognitive control, emotion, and social cognition function. These findings showed that the rich-club organization exists across many different static networks (20). Meanwhile, our results also suggested a characteristic pattern of disruption where brain connectivity is targeted globally rather than at the central network core in patients with acute mTBI.
The rich-club plays a unique role in maintaining overall functional coordination and is related to higher-order cognitive abilities, which are vulnerable in most brain disorders and may be related to rich-club vulnerability in pathological conditions (6). Disturbances in rich-club organization of the acute mTBI patients were observed in rich-club connections and in feeder and peripheral connections, suggesting that peripheral regions may also lead to cognitive impairment. These findings also suggested that global network functions, rather than only rich-club disruptions, play an important role in patients with mTBI. Nevertheless, how can the increased rich-club connectivity be understood in patients with acute mTBI? An explanation may be that the observed increase of rich-club connectivity reflects a compensatory mechanism of neurological stress. Consistent with our results, the study by Hillary et al. (29) showed a hyperconnectivity hypothesis that during the first year of recovery after TBI, neural networks will show increased connectivity. Our findings exhibited that aberrant brain network organization, especially disrupted rich-club connectivity, is associated with cognitive impairment after acute mTBI. Recent structural network studies utilizing high angular diffusion MRI in long-term TBI showed an altered overall network organization and weak rich-club and local connectivity density (50). Remarkably, the rich-club connections were reorganized during the onset and development of cognitive impairment. Therefore, the mechanism of cognitive impairment after mTBI may be closely related to the disruption and redistribution of brain hubs in the functional networks.
The topological characterization of neural network locates the rich-club in the center of brain communication pathways. However, previous studies have shown that the various indicators of brain network organization are not isolated, but interrelated (51). In many real-world networks, the presence of the rich-club is considered the basis for important organizational attributes, including high clustering (52). Therefore, our findings suggested that the observed differences in topological characterization metrics may partly be due to disrupted rich-club connectivity. Although different disorders may target different central subsets, the parallel loss of rich club impairment and network efficiency is a common feature of brain pathology (53). Local topological properties of the FC networks also showed different changes across groups, such that nodal efficiency values were significantly higher in patients with acute mTBI than in the HCs. Abnormalities in nodal efficiency appear to be overrepresented among rich-club members, and this result was consistent with previous reports. Therefore, the high-efficiency state always involves some brain regions overlapping with the rich-club structure to accurately perform cognitive tasks requiring integration of multiple functional domains (54,55).
In addition, impairment in numerous cognitive domains has been reported in mTBI; these mainly include memory and learning, executive function, processing speed, and attention (4,56). It remains unclear why some of the cognitive domains affected by mTBI while others were spared. Our brain is a very efficient network of different brain regions, each with its own tasks and functions, that are constantly sharing information with each other. Functional communication between brain regions is likely to play a key role in complex cognitive processes. Evidence indicates that a single concussion can disrupt the neurological mechanisms underlying cognition (57). Moreover, previous studies have found that structural brain changes occur in numerous brain regions following mTBI. For example, a study showed that temporal and frontal association white matter pathways are most frequently damaged in mTBI, and the integrity of these paths showed some correlation with cognitive impairment (58). Therefore, we speculate that mTBI can affect these related cognitive functions, since it causes structural changes in various brain regions with different cognitive functions. However, the specific relationship between mTBI and symptoms of cognitive impairment remains unclear and needs further study.
This study had several limitations. First, the sample size of the current study was relatively small; our results should be validated by repeating our analysis with a larger sample of participants. Moreover, a limitation of graph theory analysis is the arbitrary threshold and binarization process, which leads to information loss. However, in this study, we calculated the graph theoretical measures based on binary networks, given the theories that binary networks maintain the most important correlations and may be less sensitive to noise and easier to explain (39). Finally, the selection of the threshold program is still a controversial topic in the study of brain networks (59,60). In this study, we used only 1 type of thresholding procedure, namely the fixed-density threshold procedure. Different threshold methods may lead to different topological organization and result in different findings during comparison between groups. Future research should keep in mind the different conceptual characteristics of different threshold strategies and apply them in network analysis and comparative research.
In conclusion, our study found that brain network abnormalities, including impaired rich-club organization and topology, are related to cognitive impairment after acute mTBI. These findings may contribute to a comprehensive understanding of rich club connectivity as an early neurobiomarker of cognitive impairment after acute mTBI. Findings may also, to some extent, help clinicians identify interventions or treatments that are more appropriate to ameliorate these impairments, for example, neurotrophic drug therapy and targeted therapies (physical therapy, cognitive behavioral therapy, etc.) that can improve the corresponding cognitive impairment.
Acknowledgments
Funding: This work was funded by the Natural Science Foundation of China (No. 82102012, No. 82102006).
Footnote
Reporting Checklist: The authors have completed the TREND reporting checklist. Available at https://qims.amegroups.com/article/view/10.21037/qims-21-915/rc
Conflicts of Interest: All authors have completed the ICMJE uniform disclosure form (available at https://qims.amegroups.com/article/view/10.21037/qims-21-915/coif). The authors have no conflicts of interest to declare.
Ethical Statement: The authors are accountable for all aspects of the work in ensuring that questions related to the accuracy or integrity of any part of the work are appropriately investigated and resolved. The study was conducted in accordance with the Declaration of Helsinki (as revised in 2013). The current study was approved by the Institutional Review Board of Nanjing Medical University. Written informed consent was provided by all participants before their participation in the study protocol.
Open Access Statement: This is an Open Access article distributed in accordance with the Creative Commons Attribution-NonCommercial-NoDerivs 4.0 International License (CC BY-NC-ND 4.0), which permits the non-commercial replication and distribution of the article with the strict proviso that no changes or edits are made and the original work is properly cited (including links to both the formal publication through the relevant DOI and the license). See: https://creativecommons.org/licenses/by-nc-nd/4.0/.
References
- van Gils A, Stone J, Welch K, Davidson LR, Kerslake D, Caesar D, McWhirter L, Carson A. Management of mild traumatic brain injury. Pract Neurol 2020;20:213-21. [Crossref] [PubMed]
- Richter S, Winzeck S, Kornaropoulos EN, Das T, Vande Vyvere T, Verheyden J, Williams GB, Correia MM, Menon DK, Newcombe VFJ. Collaborative European NeuroTrauma Effectiveness Research in Traumatic Brain Injury Magnetic Resonance Imaging (CENTER-TBI MRI) Substudy Participants and Investigators. Neuroanatomical Substrates and Symptoms Associated With Magnetic Resonance Imaging of Patients With Mild Traumatic Brain Injury. JAMA Netw Open 2021;4:e210994. [Crossref] [PubMed]
- Allen CM, Halsey L, Topcu G, Rier L, Gascoyne LE, Scadding JW, Furlong PL, Dunkley BT. das Nair R, Brookes MJ, Evangelou N. Magnetoencephalography abnormalities in adult mild traumatic brain injury: A systematic review. Neuroimage Clin 2021;31:102697. [Crossref] [PubMed]
- Huang M, Lewine JD, Lee RR. Magnetoencephalography for Mild Traumatic Brain Injury and Posttraumatic Stress Disorder. Neuroimaging Clin N Am 2020;30:175-92. [Crossref] [PubMed]
- Oldham S, Ball G, Fornito A. Early and late development of hub connectivity in the human brain. Curr Opin Psychol 2021; Epub ahead of print. [Crossref] [PubMed]
- Griffa A, Van den Heuvel MP. Rich-club neurocircuitry: function, evolution, and vulnerability. Dialogues Clin Neurosci 2018;20:121-32. [Crossref] [PubMed]
- Dumkrieger G, Chong CD, Ross K, Berisha V, Schwedt TJ. Static and dynamic functional connectivity differences between migraine and persistent post-traumatic headache: A resting-state magnetic resonance imaging study. Cephalalgia 2019;39:1366-81. [Crossref] [PubMed]
- Killgore WDS, Vanuk JR, Shane BR, Weber M, Bajaj S. A randomized, double-blind, placebo-controlled trial of blue wavelength light exposure on sleep and recovery of brain structure, function, and cognition following mild traumatic brain injury. Neurobiol Dis 2020;134:104679. [Crossref] [PubMed]
- Iraji A, Chen H, Wiseman N, Welch RD, O'Neil BJ, Haacke EM, Liu T, Kou Z. Compensation through Functional Hyperconnectivity: A Longitudinal Connectome Assessment of Mild Traumatic Brain Injury. Neural Plast 2016;2016:4072402. [Crossref] [PubMed]
- Chong CD, Schwedt TJ. Research Imaging of Brain Structure and Function After Concussion. Headache 2018;58:827-35. [Crossref] [PubMed]
- Li F, Lu L, Shang S, Hu L, Chen H, Wang P, Zhang H, Chen YC, Yin X. Disrupted functional network connectivity predicts cognitive impairment after acute mild traumatic brain injury. CNS Neurosci Ther 2020;26:1083-91. [Crossref] [PubMed]
- Lu L, Zhang J, Li F, Shang S, Chen H, Yin X, Gao W, Chen YC. Aberrant Static and Dynamic Functional Network Connectivity in Acute Mild Traumatic Brain Injury with Cognitive Impairment. Clin Neuroradiol 2022;32:205-14. [Crossref] [PubMed]
- Zhou Y, Milham MP, Lui YW, Miles L, Reaume J, Sodickson DK, Grossman RI, Ge Y. Default-mode network disruption in mild traumatic brain injury. Radiology 2012;265:882-92. [Crossref] [PubMed]
- Xiong KL, Zhang JN, Zhang YL, Zhang Y, Chen H, Qiu MG. Brain functional connectivity and cognition in mild traumatic brain injury. Neuroradiology 2016;58:733-9. [Crossref] [PubMed]
- Amir J, Nair JKR, Del Carpio-O'Donovan R, Ptito A, Chen JK, Chankowsky J, Tinawi S, Lunkova E, Saluja RS. Atypical resting state functional connectivity in mild traumatic brain injury. Brain Behav 2021;11:e2261. [Crossref] [PubMed]
- van der Horn HJ, Liemburg EJ, Scheenen ME, de Koning ME, Spikman JM, van der Naalt J. Graph Analysis of Functional Brain Networks in Patients with Mild Traumatic Brain Injury. PLoS One 2017;12:e0171031. [Crossref] [PubMed]
- Zhou Y. Small world properties changes in mild traumatic brain injury. J Magn Reson Imaging 2017;46:518-27. [Crossref] [PubMed]
- Antonakakis M, Dimitriadis SI, Zervakis M, Papanicolaou AC, Zouridakis G. Altered Rich-Club and Frequency-Dependent Subnetwork Organization in Mild Traumatic Brain Injury: A MEG Resting-State Study. Front Hum Neurosci 2017;11:416. [Crossref] [PubMed]
- van den Heuvel MP, Kahn RS, Goñi J, Sporns O. High-cost, high-capacity backbone for global brain communication. Proc Natl Acad Sci U S A 2012;109:11372-7. [Crossref] [PubMed]
- Wang B, Zhan Q, Yan T, Imtiaz S, Xiang J, Niu Y, Liu M, Wang G, Cao R, Li D. Hemisphere and Gender Differences in the Rich-Club Organization of Structural Networks. Cereb Cortex 2019;29:4889-901. [Crossref] [PubMed]
- van den Heuvel MP, Sporns O. An anatomical substrate for integration among functional networks in human cortex. J Neurosci 2013;33:14489-500. [Crossref] [PubMed]
- Schirmer MD, Chung AW, Grant PE, Rost NS. Network structural dependency in the human connectome across the life-span. Netw Neurosci 2019;3:792-806. [Crossref] [PubMed]
- van den Heuvel MP, Sporns O, Collin G, Scheewe T, Mandl RC, Cahn W, Goñi J, Hulshoff Pol HE, Kahn RS. Abnormal rich club organization and functional brain dynamics in schizophrenia. JAMA Psychiatry 2013;70:783-92. [Crossref] [PubMed]
- Yan T, Wang W, Yang L, Chen K, Chen R, Han Y. Rich club disturbances of the human connectome from subjective cognitive decline to Alzheimer's disease. Theranostics 2018;8:3237-55. [Crossref] [PubMed]
- Cao R, Wang X, Gao Y, Li T, Zhang H, Hussain W, Xie Y, Wang J, Wang B, Xiang J. Abnormal Anatomical Rich-Club Organization and Structural-Functional Coupling in Mild Cognitive Impairment and Alzheimer's Disease. Front Neurol 2020;11:53. [Crossref] [PubMed]
- Cui LB, Wei Y, Xi YB, Griffa A, De Lange SC, Kahn RS, Yin H, Van den Heuvel MP. Connectome-Based Patterns of First-Episode Medication-Naïve Patients With Schizophrenia. Schizophr Bull 2019;45:1291-9. [Crossref] [PubMed]
- Wu Y, Zhou Z, Fu S, Zeng S, Ma X, Fang J, Yang N, Li C, Yin Y, Hua K, Liu M, Li G, Yu K, Jiang G. Abnormal Rich Club Organization of Structural Network as a Neuroimaging Feature in Relation With the Severity of Primary Insomnia. Front Psychiatry 2020;11:308. [Crossref] [PubMed]
- Ktena SI, Schirmer MD, Etherton MR, Giese AK, Tuozzo C, Mills BB, Rueckert D, Wu O, Rost NS. Brain Connectivity Measures Improve Modeling of Functional Outcome After Acute Ischemic Stroke. Stroke 2019;50:2761-7. [Crossref] [PubMed]
- Hillary FG, Rajtmajer SM, Roman CA, Medaglia JD, Slocomb-Dluzen JE, Calhoun VD, Good DC, Wylie GR. The rich get richer: brain injury elicits hyperconnectivity in core subnetworks. PLoS One 2014;9:e104021. [Crossref] [PubMed]
- de Guise E, Alturki AY, LeBlanc J, Champoux MC, Couturier C, Lamoureux J, Desjardins M, Marcoux J, Maleki M, Feyz M. The Montreal Cognitive Assessment in persons with traumatic brain injury. Appl Neuropsychol Adult 2014;21:128-35. [Crossref] [PubMed]
- Yan CG, Wang XD, Zuo XN, Zang YF. DPABI: Data Processing & Analysis for (Resting-State) Brain Imaging. Neuroinformatics 2016;14:339-51. [Crossref] [PubMed]
- Kopal J, Pidnebesna A, Tomeček D, Tintěra J, Hlinka J. Typicality of functional connectivity robustly captures motion artifacts in rs-fMRI across datasets, atlases, and preprocessing pipelines. Hum Brain Mapp 2020;41:5325-40. [Crossref] [PubMed]
- Wang S, Li Y, Qiu S, Zhang C, Wang G, Xian J, Li T, He H. Reorganization of rich-clubs in functional brain networks during propofol-induced unconsciousness and natural sleep. Neuroimage Clin 2020;25:102188. [Crossref] [PubMed]
- Collin G, Sporns O, Mandl RC, van den Heuvel MP. Structural and functional aspects relating to cost and benefit of rich club organization in the human cerebral cortex. Cereb Cortex 2014;24:2258-67. [Crossref] [PubMed]
- Dosenbach NU, Nardos B, Cohen AL, Fair DA, Power JD, Church JA, Nelson SM, Wig GS, Vogel AC, Lessov-Schlaggar CN, Barnes KA, Dubis JW, Feczko E, Coalson RS, Pruett JR Jr, Barch DM, Petersen SE, Schlaggar BL. Prediction of individual brain maturity using fMRI. Science 2010;329:1358-61. [Crossref] [PubMed]
- Choe AS, Nebel MB, Barber AD, Cohen JR, Xu Y, Pekar JJ, Caffo B, Lindquist MA. Comparing test-retest reliability of dynamic functional connectivity methods. Neuroimage 2017;158:155-75. [Crossref] [PubMed]
- Wang J, Wang X, Xia M, Liao X, Evans A, He Y. GRETNA: a graph theoretical network analysis toolbox for imaging connectomics. Front Hum Neurosci 2015;9:386. [PubMed]
- Wen H, Liu Y, Rekik I, Wang S, Zhang J, Zhang Y, Peng Y, He H. Disrupted topological organization of structural networks revealed by probabilistic diffusion tractography in Tourette syndrome children. Hum Brain Mapp 2017;38:3988-4008. [Crossref] [PubMed]
- Rubinov M, Sporns O. Complex network measures of brain connectivity: uses and interpretations. Neuroimage 2010;52:1059-69. [Crossref] [PubMed]
- Rubinov M, Sporns O. Weight-conserving characterization of complex functional brain networks. Neuroimage 2011;56:2068-79. [Crossref] [PubMed]
- Daianu M, Jahanshad N, Nir TM, Jack CR Jr, Weiner MW, Bernstein MA, Thompson PMAlzheimer's Disease Neuroimaging Initiative. Rich club analysis in the Alzheimer's disease connectome reveals a relatively undisturbed structural core network. Hum Brain Mapp 2015;36:3087-103. [Crossref] [PubMed]
- Collin G, Kahn RS, de Reus MA, Cahn W, van den Heuvel MP. Impaired rich club connectivity in unaffected siblings of schizophrenia patients. Schizophr Bull 2014;40:438-48. [Crossref] [PubMed]
- van Leijsen EMC, van Uden IWM, Bergkamp MI, van der Holst HM, Norris DG, Claassen JAHR, Kessels RPC, de Leeuw FE, Tuladhar AM. Longitudinal changes in rich club organization and cognition in cerebral small vessel disease. Neuroimage Clin 2019;24:102048. [Crossref] [PubMed]
- Xia M, Wang J, He Y. BrainNet Viewer: a network visualization tool for human brain connectomics. PLoS One 2013;8:e68910. [Crossref] [PubMed]
- Kaplan CM, Schrepf A, Vatansever D, Larkin TE, Mawla I, Ichesco E, Kochlefl L, Harte SE, Clauw DJ, Mashour GA, Harris RE. Functional and neurochemical disruptions of brain hub topology in chronic pain. Pain 2019;160:973-83. [Crossref] [PubMed]
- Kim J, Criaud M, Cho SS, Díez-Cirarda M, Mihaescu A, Coakeley S, Ghadery C, Valli M, Jacobs MF, Houle S, Strafella AP. Abnormal intrinsic brain functional network dynamics in Parkinson's disease. Brain 2017;140:2955-67. [Crossref] [PubMed]
- Fagerholm ED, Hellyer PJ, Scott G, Leech R, Sharp DJ. Disconnection of network hubs and cognitive impairment after traumatic brain injury. Brain 2015;138:1696-709. [Crossref] [PubMed]
- De Vico Fallani F, Richiardi J, Chavez M, Achard S. Graph analysis of functional brain networks: practical issues in translational neuroscience. Philos Trans R Soc Lond B Biol Sci 2014;369:20130521. [Crossref] [PubMed]
- Popescu M, Hughes JD, Popescu EA, Riedy G, DeGraba TJ. Reduced prefrontal MEG alpha-band power in mild traumatic brain injury with associated posttraumatic stress disorder symptoms. Clin Neurophysiol 2016;127:3075-85. [Crossref] [PubMed]
- Mishra VR, Sreenivasan KR, Zhuang X, Yang Z, Cordes D, Banks SJ, Bernick C. Understanding white matter structural connectivity differences between cognitively impaired and nonimpaired active professional fighters. Hum Brain Mapp 2019;40:5108-22. [Crossref] [PubMed]
- de Reus MA, van den Heuvel MP. The parcellation-based connectome: limitations and extensions. Neuroimage 2013;80:397-404. [Crossref] [PubMed]
- Xu XK, Zhang J, Small M. Rich-club connectivity dominates assortativity and transitivity of complex networks. Phys Rev E Stat Nonlin Soft Matter Phys 2010;82:046117. [Crossref] [PubMed]
- Crossley NA, Mechelli A, Scott J, Carletti F, Fox PT, McGuire P, Bullmore ET. The hubs of the human connectome are generally implicated in the anatomy of brain disorders. Brain 2014;137:2382-95. [Crossref] [PubMed]
- Shine JM, Bissett PG, Bell PT, Koyejo O, Balsters JH, Gorgolewski KJ, Moodie CA, Poldrack RA. The Dynamics of Functional Brain Networks: Integrated Network States during Cognitive Task Performance. Neuron 2016;92:544-54. [Crossref] [PubMed]
- de Pasquale F, Corbetta M, Betti V, Della Penna S. Cortical cores in network dynamics. Neuroimage 2018;180:370-82. [Crossref] [PubMed]
- McInnes K, Friesen CL, MacKenzie DE, Westwood DA, Boe SG. Mild Traumatic Brain Injury (mTBI) and chronic cognitive impairment: A scoping review. PLoS One 2017;12:e0174847. [Crossref] [PubMed]
- Xiong K, Zhu Y, Zhang Y, Yin Z, Zhang J, Qiu M, Zhang W. White matter integrity and cognition in mild traumatic brain injury following motor vehicle accident. Brain Res 2014;1591:86-92. [Crossref] [PubMed]
- Polinder S, Cnossen MC, Real RGL, Covic A, Gorbunova A, Voormolen DC, Master CL, Haagsma JA, Diaz-Arrastia R, von Steinbuechel N. A Multidimensional Approach to Post-concussion Symptoms in Mild Traumatic Brain Injury. Front Neurol 2018;9:1113. [Crossref] [PubMed]
- van den Heuvel MP, de Lange SC, Zalesky A, Seguin C, Yeo BTT, Schmidt R. Proportional thresholding in resting-state fMRI functional connectivity networks and consequences for patient-control connectome studies: Issues and recommendations. Neuroimage 2017;152:437-49. [Crossref] [PubMed]
- Hallquist MN, Hillary FG. Graph theory approaches to functional network organization in brain disorders: A critique for a brave new small-world. Netw Neurosci 2019;3:1-26. [Crossref] [PubMed]