Clinical experience of tensor-valued diffusion encoding for microstructure imaging by diffusional variance decomposition in patients with breast cancer
Introduction
Magnetic resonance imaging (MRI) is widely used for evaluating breast cancer because of its non-invasive nature and excellent soft tissue contrast (1-4). Particularly, single diffusion encoding along a single direction per shot has been incorporated as a key imaging technique into routine breast MRI examination to complement dynamic contrast-enhanced (DCE) MRI, since single diffusion encoding can produce contrast in tissues without using gadolinium contrast medium injections and images can be acquired rapidly (5-8).
Single diffusion encoding is a well-known, non-invasive functional MRI technique based on quantitative measure of water diffusion in tissues to provide apparent diffusion coefficient (ADC) which reflects tissue microstructure and tumor cellularity of the breast for lesion characterization; however, previous studies reported inconsistent ADC values with large variability (9-16). One possible explanation for this issue is that the ADC is an average metric that does not capture heterogeneity within individual voxels, meaning that it cannot distinguish variable tumor cellularity, extent of infiltration, and unavoidable overlap between tumorous and non-tumorous tissues (17-20). Therefore, there have been attempts to apply fitting the models of diffusion-tensor invariants based on the diffusion-weighted imaging—such as diffusion tensor imaging (DTI) and diffusion kurtosis imaging (DKI)—to the evaluation of breast tumors (21-25).
Recently, there has been an increasing effort to understand the relation between diffusion parameters and tissue heterogeneity by considering the ‘kurtosis of the diffusion propagator’ or ‘variance of the distribution of diffusivities’ in systems that contain multiple Gaussian components (25). For example, the mean kurtosis (MK) from DKI can quantify the total non-Gaussianity, which may be caused by tissue heterogeneity (26), but it cannot distinguish the specific source of the kurtosis or estimate the microscopic diffusion anisotropy. Recently, tensor-valued diffusion encoding (27), also known as multidimensional diffusion encoding (28), has been made possible at a wide range of clinical MRI systems by efficient experimental design (29). The term ‘tensor-valued’ indicates that diffusion encoding is applied along multiple directions per signal acquisition (27,28), unlike single diffusion encoding where diffusion encoding is applied along one direction at a time. Q-space trajectory imaging (QTI) is an analysis method that uses data encoded by multiple b-values and b-tensor shapes, to decompose the total diffusional variance into two components (27,30,31), namely the ‘anisotropic variance’ caused by microscopic diffusion anisotropy related to eccentric cells and tissue structures (30,32,33), and the ‘isotropic variance’ caused by heterogeneous isotropic diffusivity due to variable cell density or tissue mixtures (30).
To the best of our knowledge, only one recent study has introduced the clinical potential of tensor-valued diffusion sequence in limited number of study patients with breast cancers (34). In the present study, we aimed to investigate the clinical utility of the tensor-valued diffusion sequence by assessing the microstructural detail of breast cancers in a larger number of homogeneous study patients and in a different scanning environment. Also, we present the following article in accordance with the STROBE reporting checklist (available at https://dx.doi.org/10.21037/qims-21-870).
Methods
Study population
The study was conducted in accordance with the Declaration of Helsinki (as revised in 2013). The study was approved by Institutional Review Board of Gyeongsang National University Changwon Hospital (No.: GNUCH 2021-06-013, retrospectively registered on June 30, 2021), and individual consent for this retrospective analysis was waived.
We searched picture archiving and communication system reports and retrospectively reviewed biopsy-proven breast cancer patients who underwent preoperative breast MRI examination from July 2020 to March 2021 at Gyeongsang National University Changwon Hospital (GNUCH). We found 37 female patients with 39 breast cancers. Of them, we excluded 3 patients with invasive lobular carcinomas and 6 patients with ductal carcinoma in situ, because the number of patients was too small to provide appropriate characteristics on MRI. The final analysis included 29 female patients with 30 breast cancers that were pathologically proven as invasive ductal carcinoma (IDC) (mean age, 49±10 years; interval 39–72 years), and one patient had synchronous bilateral breast cancer (Figure 1). Among the 30 lesions, 26 lesions were surgically removed at GNUCH. We also reviewed prognostic cancer stages based on histopathological reports according to the 8th edition of the American Joint Committee on Cancer (AJCC) and the histologic grade with the Nottingham modification of the Scarff-Bloom-Richardson Grading System (35,36).
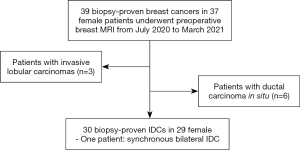
MRI acquisition
Breast MRI was performed using the 3T system (Signa™ Architect; GE Healthcare, Waukesha, WI, USA) with an 8-channel breast coil in prone position. The examination included two morphological sequences: a 3D Dixon-based fat-suppressed T2-weighted sequence with repetition time (TR) =2,000 ms, echo time (TE) =90 ms, axial slices at resolution =0.7×0.7×1.2 mm3, and an acquisition time of 3 min; and a DCE 3D T1-weighted sequence with high temporal and spatial resolution using a dual-echo 3D spoiled gradient echo sequence with Dixon fat-water separation with TR =5.0 ms, TE =1.7 ms, flip angle =10 degrees, resolution =0.7×0.7×0.6 mm3, and an acquisition time of 8 min.
The examination also included single diffusion encoding to acquire data for the regular ADC analysis, and tensor-valued diffusion encoding intended for QTI analysis. The single diffusion encoding was a spin-echo sequence with echo-planar readout with b-values 0 and 1,500 s/mm2, TR =2,970 ms, TE =82 ms, number of excitations =6, number of slices of each b-value =40, axial slices at resolution =1.3×1.3×5.0 mm3, and an acquisition time of 3:27 min. Tensor-valued diffusion encoding was performed by a prototype pulse sequence which is named multidimensional diffusion provided by the vendor. It used gradient waveforms optimized for the MRI hardware, including compensation for concomitant gradients (37,38). Two waveforms were used to yield linear and spherical b-tensor encoding (LTE and STE) at three b-values 100, 1,000, and 2,000 s/mm2, where the LTE was directed along [4 10 15] directions and the STE was repeated [6 10 10] times for each b-value, respectively. We used TR =9,370 ms, TE =120 ms, resolution =4.3×4.3×5.0 mm3 with a total acquisition time of 9:47 min. The two waveforms are depicted in Figure 2. Detailed technical information on the tensor-valued diffusion sequence, sampling scheme, and gradient waveforms are available at https://github.com/filip-szczepankiewicz/fwf_seq_resources. In addition, the following GitHub repository also contains auxiliary routines for setup of acquisition protocols and image visualization: https://github.com/markus-nilsson/md-dmri.
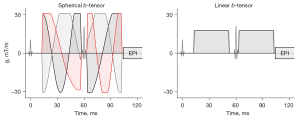
Image processing and analysis
Motion correction and eddy-current correction were not performed, but data was inspected visually to exclude any data with gross errors. At the stage of the visual inspection, there was no patient to exclude due to gross error. We performed QTI according to the method described in Westin et al. (27). We adopted the parameter nomenclature in Szczepankiewicz et al. (30), including the mean diffusivity (MD), the anisotropic and isotropic mean kurtosis (MKA and MKI), and total mean kurtosis (MKT = MKA + MKI), as well as the fractional anisotropy (FA), which is equivalent to FA from DTI, and microscopic fractional anisotropy (µFA) which reflects the diffusion anisotropy without the effects of orientation dispersion. These parameters are similar to the ones employed by Naranjo et al. (34), however, they are derived from a cumulant expansion signal representation rather than an inverse Laplace transform. The main benefit of this is that the fitting is markedly faster and robust to the signal sampling scheme; however, it does not allow estimation of specific compartment signal fractions. The parameter estimation was performed using the open-source toolbox for multidimensional diffusion MRI (39,40).
The analysis was performed in MATLAB (R2019a, The Mathworks, Natick, MA, USA), and detail information for data analysis using MATLAB software is available at https://github.com/markus-nilsson/md-dmri. The regions-of-interest (ROIs) of the tumor were manually drawn on S0 maps calculated by QTI parameters, and the DCE findings were used to confirm the delineation of the tumor. All ROIs were delineated by one breast radiologist with 6 years of experience in breast MRI. Note that we avoided the inclusion of peritumoral edema, intratumoral necrosis, and normal breast parenchymal tissue. The mean values for each QTI parameter across all voxels in the ROI were calculated for each tumor. The same values were also calculated for normal fibroglandular breast tissue (FGBT) at the contralateral healthy breast.
Histopathological analysis
A board-certified pathologist with 7 years of experience retrospectively reviewed the results of the immunohistochemical analysis of the hematoxylin-and-eosin-stained surgical specimens. These results were used to evaluate tumor size, grade, and pathologic type; estrogen receptor, progesterone receptor, c-erb-B2, and Ki-67 expression; and axillary lymph node (LN) metastasis, which was assessed through fine needle aspiration biopsy, sentinel LN biopsy, or axillary LN dissection. We used a cutoff value of 14% classify the participants into low Ki-67 expression and high Ki-67 expression groups according to the St Gallen International Expert Consensus (41). We also classified the patients into groups based on hormonal receptors, c-erb-B2 expression (negative or positive), histologic grade (1, 2, or 3), and AJCC prognostic stage (1, 2, or 3).
Statistical analysis
The distribution of the data was tested using the Kolmogorov-Smirnov test. For parameters with a non-normal distribution, the Mann-Whitney U-test was used to compare diffusion metrics between the IDC and FGBT and those between the IDC subgroups, and the Kruskal-Wallis test was used to compare diffusion metrics among the subgroups according to the AJCC prognostic cancer stage and IDC histologic grade. Cohen’s d effect sizes (ES) were calculated to determine the standardized mean differences between the groups (42,43). We analyzed inter-method reliability for tumor size measurement between breast MRI and pathological examination using intraclass correlation coefficient (ICC) analysis. Spearman’s correlation analysis was also used to evaluate the correlations between the diffusion metrics and tumor size. All statistical analyses were conducted using SPSS, version 24.0 (IBM Corp., Armonk, NY, USA), and P<0.05 was considered significant.
Results
Clinicopathological characteristics of patients and tumors
We have summarized the clinicopathological characteristics of the 30 IDC patients in Table 1. The mean tumor size was 28±23 mm on DCE T1-weighted MRI (range, 7–87 mm) and 25±16 mm on pathological examination (range, 10–54 mm). Additionally, we observed good reliability between the tumor size measured on MRI and that on pathological examination (ICC =0.80, 95% confidence interval: 0.54–0.91, P<0.001).
Table 1
Variables | Total (n=30) |
---|---|
Age at diagnosis (year) | 49±10 |
Amount of fibroglandular tissue | |
Almost entirely fatty | 1 |
Scattered fibroglandular tissue | 10 |
Heterogeneous fibroglandular tissue | 12 |
Extreme fibroglandular tissue | 7 |
Background parenchymal enhancement | |
Minimal enhancement | 15 |
Mild enhancement | 7 |
Moderate enhancement | 5 |
Marked enhancement | 3 |
Tumor size on MRI (mm)† | |
≤10 | 4 |
>10–20 | 14 |
>20–40 | 8 |
>40 | 4 |
AJCC prognostic cancer stage‡ | |
Stage I | 18 |
Stage II | 3 |
Stage III | 5 |
Lesion type | |
Mass | 25 |
Non-mass enhancement | 5 |
Hormonal subtypes | |
Luminal type | 25 |
C-erb-B2 positive type | 3 |
Triple negative type | 2 |
†, tumor size defined as longest diameter on dynamic contrast-enhanced images; ‡, pathologic cancer stage was determined only in the 26 patients underwent surgery at Gyeongsang National University Changwon Hospital. MRI, magnetic resonance imaging; AJCC, American Joint Committee on Cancer.
QTI parameters in IDC and normal FGBT
The QTI parameters and ADC value obtained from ROI analysis of IDC and normal FGBT are summarized in Table 2, and a representative example of diffusion parameter maps obtained through postprocessing is depicted in Figures 3-5. All diffusion parameters showed significant differences between IDCs and normal FGBT. The MD and the mean ADC of IDC were significantly lower than those of normal FGBT (P<0.001, respectively), and there was a strong correlation between the MD and the mean ADC of IDC (rs=0.75, P<0.001). However, all other QTI parameters, including MKT, MKA, MKI, FA, and µFA, were significantly higher in IDC than in normal FGBT (ES range, 0.4–2.2, all P<0.05). Among the parameters, MKT showed a higher difference in mean value than MD between IDC and FGBT, and, there was a greater elevation of MKT, which consisted of a high MKA and low MKI, in IDC than in FGBT. On qualitative histopathological review, IDC tended to have a higher cell density and lower cell density variance with eccentric growth and replacement along the radiating ducts and lobular structures with segmental distribution than FGBT (Figures 3K,3L,4K,4L,5K,5L). Additionally, the largest ES between IDC and FGBT was seen for µFA (Table 2), which suggests that it outperforms conventional diffusion parameters in distinguishing the two tissue types.
Table 2
Variables | MD | MKT | MKA | MKI | FA | μFA | ADC |
---|---|---|---|---|---|---|---|
IDCs | 1.07±0.27 | 1.07±0.45 | 0.84±0.46 | 0.26±0.28 | 0.26±0.07 | 0.69±0.14 | 0.94±0.20 |
FGT | 1.34±0.29 | 0.38±0.36 | 0.22±0.21 | 0.16±0.19 | 0.21±0.08 | 0.40±0.13 | 1.38±0.30 |
P value | <0.001 | <0.001 | <0.001 | 0.018 | 0.008 | <0.001 | <0.001 |
ES | 1.0 | 1.7 | 1.8 | 0.4 | 0.7 | 2.2 | 1.7 |
Data are mean ± standard deviation. P values were calculated using Mann-Whitney’s U test. QTI, Q-space trajectory imaging; IDC, invasive ductal carcinoma; FGBT, fibroglandular breast tissue; ES, effect sizes; MD, mean diffusivity; ADC, apparent diffusion coefficient; MKT, total mean kurtosis; MKA, anisotropic mean kurtosis; MKI, isotropic mean kurtosis; FA, macroscopic fractional anisotropy; μFA, microscopic fractional anisotropy.
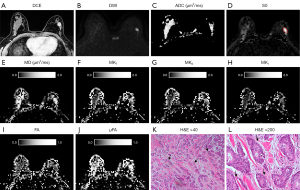
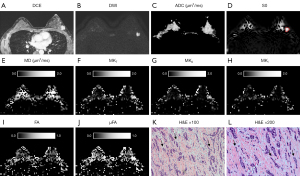
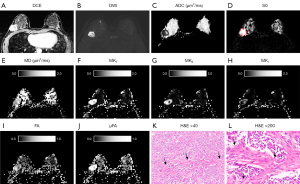
Correlation between QTI parameters and clinicopathological characteristics of IDC
Table 3 demonstrates the correlation between tumor size and diffusion parameters. MKI was positively correlated with tumor size, both on MRI and pathological examination (rs=0.38, P=0.04 vs. rs=0.54, P=0.005, respectively), whereas MKT and ADC only demonstrated moderate correlations with the tumor size on pathological examination (rs=0.46, P=0.02 vs. rs=−0.48, P=0.02).
Table 3
Variables | MD | MKT | MKA | MKI | FA | μFA | ADC |
---|---|---|---|---|---|---|---|
MRI tumor size | |||||||
rs | −0.23 | 0.36 | 0.33 | 0.38 | −0.02 | 0.32 | −0.35 |
P value | 0.23 | 0.05 | 0.07 | 0.039 | 0.92 | 0.09 | 0.056 |
Pathological tumor size | |||||||
rs | −0.31 | 0.46 | 0.38 | 0.54 | 0.06 | 0.37 | −0.48 |
P value | 0.12 | 0.019 | 0.06 | 0.005 | 0.78 | 0.07 | 0.015 |
QTI, Q-space trajectory imaging; MKI, isotropic mean kurtosis; MD, mean diffusivity; ADC, apparent diffusion coefficient; MKT, total mean kurtosis; MKA, anisotropic mean kurtosis; FA, macroscopic fractional anisotropy; μFA, microscopic fractional anisotropy; rs, Spearman’s rank correlation coefficient. P values were calculated using Spearman’s correlation analysis.
Table 4 demonstrates the comparison of diffusion parameters in IDC according to prognostic factors. IDCs with LN metastasis showed significantly higher MKT, MKA, and µFA than those without LN metastasis (ES: 0.99, 0.96, and 0.81, respectively). Although no significant difference was seen in any diffusion parameter between the groups according to hormonal receptor, c-erb-B2, and Ki-67 expression, the positive c-erb-B2 expression group had a higher µFA, with a large ES, than the negative c-erb-B2 expression group (ES: 0.83, P=0.23). According to the histologic grades, QTI parameters—MD, MKT, MKA, MKI, FA, and µFA—showed no significant differences among the grade 1, grade 2, and grade 3 groups. Furthermore, there were no significant differences in the six QTI parameters among the AJCC stage 1, stage 2, and stage 3 groups.
Table 4
Factor | No. of tumors | MD | MKT | MKA | MKI | FA | μFA | ADC |
---|---|---|---|---|---|---|---|---|
Hormone receptor | ||||||||
Positive | 25 | 1.09±0.30 | 1.05±0.50 | 0.80±0.47 | 0.28±0.26 | 0.26±0.08 | 0.67±0.15 | 0.94±0.21 |
Negative | 5 | 1.05±0.12 | 0.98±0.26 | 0.91±0.49 | 0.06±0.35 | 0.24±0.05 | 0.73±0.14 | 0.92±0.12 |
P value | 0.96 | 0.83 | 0.55 | 0.23 | 0.83 | 0.48 | 0.87 | |
ES | 0.17 | 0.18 | 0.23 | 0.71 | 0.30 | 0.41 | 0.12 | |
C-erb-B2 | ||||||||
Positive | 3 | 0.97±0.06 | 1.13±0.16 | 1.10±0.55 | 0.03±0.50 | 0.23±0.06 | 0.79±0.15 | 0.84±0.02 |
Negative | 27 | 1.10±0.29 | 1.03±0.49 | 0.78±0.45 | 0.27±0.25 | 0.26±0.08 | 0.67±0.14 | 0.95±0.20 |
P value | 0.28 | 0.60 | 0.25 | 0.70 | 0.65 | 0.23 | 0.25 | |
ES | 0.62 | 0.27 | 0.63 | 0.61 | 0.42 | 0.83 | 0.77 | |
Ki-67† | ||||||||
<14% | 7 | 1.14±0.36 | 0.89±0.58 | 0.68±0.53 | 0.21±0.14 | 0.27±0.09 | 0.63±0.18 | 1.07±0.16 |
≥14% | 19 | 1.09±0.27 | 1.08±0.46 | 0.77±0.39 | 0.34±0.24 | 0.26±0.07 | 0.67±0.12 | 0.90±0.20 |
P value | 0.82 | 0.36 | 0.53 | 0.23 | 0.96 | 0.69 | 0.05 | |
ES | 0.16 | 0.36 | 0.19 | 0.66 | 0.12 | 0.26 | 0.94 | |
Histologic grades‡ | ||||||||
Grade 1 | 10 | 1.03±0.26 | 1.19±0.55 | 0.85±0.50 | 0.36±0.28 | 0.28±0.10 | 0.70±0.13 | 0.95±0.21 |
Grade 2 | 8 | 1.23±0.39 | 0.81±0.47 | 0.69±0.54 | 0.12±0.22 | 0.24±0.06 | 0.63±0.20 | 1.05±0.19 |
Grade 3 | 8 | 1.02±0.18 | 1.10±0.33 | 0.87±0.42 | 0.28±0.36 | 0.23±0.07 | 0.70±0.13 | 0.81±0.10 |
P value | 0.46 | 0.27 | 0.61 | 0.14 | 0.61 | 0.80 | 0.09 | |
ES | 0.28 | 0.34 | 0.42 | 0.58 | 0.42 | 0.53 | 0.23 | |
AJCC prognostic stages§ | ||||||||
Stage I | 18 | 1.12±0.27 | 1.00±0.53 | 0.76±0.50 | 0.25±0.27 | 0.26±0.09 | 0.67±0.16 | 0.98±0.21 |
Stage II | 3 | 0.91±0.46 | 1.29±0.17 | 1.26±0.35 | 0.03±0.49 | 0.27±0.10 | 0.84±0.08 | 1.29±0.18 |
Stage III | 5 | 1.04±0.16 | 1.11±0.41 | 0.77±0.38 | 0.42±0.24 | 0.24±0.04 | 0.67±0.11 | 0.90±0.13 |
P value | 0.29 | 0.37 | 0.15 | 0.28 | 0.93 | 0.11 | 0.20 | |
ES | 0.29 | 0.03 | 0.58 | 0.30 | 0.59 | 0.68 | 0.29 | |
Lymph node metastasis | ||||||||
Positive | 11 | 0.98±0.24 | 1.30±0.37 | 1.08±0.49 | 0.28±0.30 | 0.26±0.09 | 0.75±0.12 | 0.90±0.08 |
Negative | 19 | 1.15±0.28 | 0.89±0.45 | 0.66±0.38 | 0.23±0.28 | 0.25±0.07 | 0.64±0.15 | 0.97±0.22 |
P value | 0.27 | 0.033 | 0.026 | 0.49 | 0.55 | 0.047 | 0.31 | |
ES | 0.26 | 0.99 | 0.96 | 0.17 | 0.12 | 0.81 | 0.42 |
P values for tests for equal mean values from Mann-Whitney’s U test or Kruskal-Wallis test. ES are provided to document the standardized mean differences between the groups. †, Ki-67 index was measured only in the patients underwent surgery at our hospital; ‡, §, histologic grade and AJCC cancer stage were determined in the only 26 patients who underwent surgery at Gyeongsang National University Changwon Hospital. QTI, Q-space trajectory imaging; ES, effect size; MD, mean diffusivity; MKT, total mean kurtosis; MKA, anisotropic mean kurtosis; MKI, isotropic mean kurtosis; FA, macroscopic fractional anisotropy; μFA, microscopic fractional anisotropy; ADC, apparent diffusion coefficient.
Discussion
In this study on patients with pathologically proven IDC, we observed that IDC had a significantly lower mean value of MD than normal FGBT. Furthermore, the mean values of QTI parameters were significantly higher in IDC than in normal FGBT. The mean MKA was significantly higher than the mean MKI in IDC, which contributed to a high MKT. Among all QTI parameters, µFA had the largest ES for differentiating IDC from FGBT. Additionally, IDCs with LN metastasis had higher MKT, MKA, and µFA than those without LN metastasis.
In real-world practice, single diffusion encoding plays a key role in differentiating between benign and malignant lesions on breast MRI by using ADC as a common biomarker to indicate the average cell density in each imaging voxel (44). However, single diffusion encoding cannot provide detailed information on tumor microstructure. Therefore, DTI is performed with conventional breast MRI, and this enables the estimation of MD and FA, which is used to determine whether a breast lesion is benign or malignant (44-46). Furthermore, MK can be estimated using DKI. MK can provide information on non-Gaussian diffusion, which may be related to microstructural tissue heterogeneity (26,47,48). Also, MK has been found to improve the accuracy of differentiation of breast lesions and have a higher diagnostic potential than ADC (49,50). However, the interpretation of MK could not be linked to the two components of diffusional variance, which decreased the specificity. To overcome this limitation, tensor-valued diffusion encoding, i.e., b-tensors with different shapes, may be used, as it provides detailed microstructural information by decomposing MK into its anisotropic and isotropic components (30,31).
In the current study, the MD values in IDC were significantly lower than those in FGBT, which is consistent with the results of previous studies that have demonstrated a negative correlation of MD with tissue cellularity (44). Additionally, the MD value of IDC in our study was 1.07 µm2/ms, which was within the range reported (0.71–1.62 µm2/ms) in previous studies (24). In addition, there was a strong correlation between the MD and mean ADC of IDC which could be interpreted that the two parameters being influenced by cellular density within the voxel as expected (51). Although these two parameters reflect the average diffusivity, the MD has the major difference and advantage because it is free of bias from non-Gaussian diffusion and b-value dependence (25).
We also found that microscopic anisotropy was a dominant component of diffusion restriction in IDC, which was consistent with the findings of a recent study (34). Interestingly, our findings were similar to those of a previous study that used tensor-valued diffusion encoding in brain tumors and found high MKA values in meningiomas due to an abundance of eccentric cells and cell structures (30). Based on the histopathological correlation, we speculate that, regardless of the organ studied, the consistency of the result may be related to the similar tumor growth patterns of IDCs and meningiomas; IDC has a growth pattern that extends along the ductal system, with penetration into the adjacent stroma and high cellularity, prominent nucleoli, and numerous mitoses (52). These histopathological features can indicate directionality and eccentricity based on the anatomical characteristics of tumor growth. IDCs may have features similar to meningiomas that grow along the dura mater and show hypercellularity, relatively uniform tumor cells, and a tendency to encircle adjacent tumor cells (53). Our results were also consistent to the recent study in prostate cancer that demonstrated higher MKI and MKA values in the tumor (54). Interestingly, this recent study also showed these two QTI parameters had significant differences within the different location of normal prostatic tissues that might be related to an increase in within-voxel diffusion heterogeneity according to its zonal anatomy, reflecting the different compositions of various tissues (54). Unlike the prostate, the breast is not an organ with a detailed anatomical division, and the non-glandular tissues such stroma or fat tissue also inherently exist along the driving direction of the ductal distribution. Therefore, we could not find these differences in QTI parameters according to the detailed anatomical division.
In this study, we evaluated the correlation between QTI parameters and tumor size. However, only isotropic heterogeneity (MKI) showed a moderate positive correlation with tumor size on both MRI and pathological examination. The interpretation of this observation remains unclear. A potential explanation based on our histopathologic findings is that large tumors are more likely to contain poorly perfused regions with variable cell density than small tumors due to aggressive cell growth or necrosis. It is well-known that intratumoral necrosis is contributed to intratumoral heterogeneity of breast cancer which indicates its ability to adapt to a new microenvironment, leading to chemotherapy resistance or tumor progression (55-59). Considering this issue, MKI may be a useful non-invasive parameter to estimate the intratumoral heterogeneity of breast cancer. Further studies of QTI parameters with histopathological correlation are required to clarify the relationship between intratumoral heterogeneity and treatment response in different types of breast cancers.
In this study, we also observed higher mean values of FA and µFA due to the disorganized growth and increased cellularity of tumors in IDC than in FGBT, and these findings are consistent with the results of previous studies of brain tumors and breast tumors (30,34,60). However, our findings are inconsistent with those of other DTI studies that have reported lower or similar values of FA in breast cancer compared with FGBT (21,44,46,61-63). These discordant results suggest that FA has a limited role as a parameter for evaluating breast cancers. Interestingly, we observed that µFA was better than FA at differentiating IDC from FGBT, therefore, we believe that µFA can be a more reliable parameter than FA for assessing breast cancers because of the following reasons: (I) µFA is a straightforward microscopic analogue to FA and is closely related to MKA (30); therefore, this parameter could be a useful biomarker for demonstrating the dominant microstructural changes in breast cancer, and (II) brain imaging studies have shown that FA as a parameter of voxel scale anisotropy is confounded by the orientation coherence of the tissue (i.e., cell elongation and cell orientation) and voxel size (60,64-66).
In addition, we found that the LN metastasis group had higher MKT, MKA, and µFA than the metastasis negative group. These results suggest that QTI parameters could be useful non-invasive biomarkers for evaluating LN metastasis in breast cancers, because preoperative identification of LN metastasis is important for determining pretreatment decisions, such as surgical planning and adjuvant therapy (67,68).
Despite of the promising results, this study has several limitations which should be considered when interpreting the results. First, tensor-valued diffusion encoding tends to have a longer acquisition time than single diffusion encoding, and a reduced resolution may be required to maintain a high signal-to-noise ratio. This may introduce biases from dissimilar partial volume effects, especially for small lesions. Therefore, careful evaluation using this novel sequence with conventional breast MRI, including high-resolution single diffusion encoding, should be performed in clinical practice. Alternatively, tensor-valued diffusion encoding can be performed using MRI systems with high gradient performance (29) or by exploiting methods, such as simultaneous multi-slice imaging, to decrease scan time and yield high-resolution images in a limited amount of time. Second, we did not apply correction for motion, eddy-currents, or geometric distortion. We expect that such post-processing may improve spatial sharpness, and parameter accuracy, especially in regions of high contrast, however, implementing such methods was outside the scope of the present study. Third, the interpretation of MKI and MKA in terms of diffusional variance caused by anisotropic and isotropic variance is based on the assumption that the diffusion in each voxel has an approximately multi-Gaussian distribution (30). Since the experiment may also encode time-dependent diffusion (69), the metrics may be confounded by the size of the restrictions, intra-compartment kurtosis, and exchange (69,70). In addition, we did not perform quantitative microscopy, instead, radiological and pathological correlation was qualitative in the same manner of the recent previous studies (54,71). Therefore, further studies should corroborate our interpretation of the parameters by evaluating the association of QTI parameters with quantitative histopathology.
In conclusion, we found that QTI parameters reflected changes in tissue microstructures in IDC. The predominant histopathological features of IDC were high MKA and µFA, which represent the eccentricity of tumor cells and cell structures. µFA was superior to other parameters for differentiating IDC from FGBT; therefore, it could be a potential imaging biomarker for evaluating breast cancers. QTI is not affected by tissue organization as DTI is, hence, tensor-valued diffusion encoding can provide additional information about microstructural compartments in the breast cancer where DTI is limited (72,73). Therefore, QTI parameters based on tensor-valued diffusion encoding may facilitate a deeper understanding of tissue microstructure and intratumoral heterogeneity in patients with breast cancer who are prior to undergo surgery or received chemotherapy.
Acknowledgments
We would like to thank Tim Sprenger at MR Applied Science Laboratory Europe in GE Healthcare for his help to implement the sequence, and we also thank our colleagues in the MRI section, Seong Jin Kim, Myungwook Lee, and Jae Hyun Lim, for their efforts in ensuring that all MR images were obtained optimally.
Funding: None.
Footnote
Reporting Checklist: The authors have completed the STROBE reporting checklist. Available at https://dx.doi.org/10.21037/qims-21-870
Conflicts of Interest: All authors have completed the ICMJE uniform disclosure form (available at https://dx.doi.org/10.21037/qims-21-870). JL and SMG are employees of GE Healthcare. However, GE Healthcare has no role in the design of the study, data analyses, or data interpretation. The other authors have no conflicts of interest to declare.
Ethical Statement: The authors are accountable for all aspects of the work in ensuring that questions related to the accuracy or integrity of any part of the work are appropriately investigated and resolved. The study was conducted in accordance with the Declaration of Helsinki (as revised in 2013). The study was approved by Institutional Review Board of Gyeongsang National University Changwon Hospital (No. GNUCH 2021-06-013, retrospectively registered on June 30, 2021), and individual consent for this retrospective analysis was waived.
Open Access Statement: This is an Open Access article distributed in accordance with the Creative Commons Attribution-NonCommercial-NoDerivs 4.0 International License (CC BY-NC-ND 4.0), which permits the non-commercial replication and distribution of the article with the strict proviso that no changes or edits are made and the original work is properly cited (including links to both the formal publication through the relevant DOI and the license). See: https://creativecommons.org/licenses/by-nc-nd/4.0/.
References
- Bluemke DA, Gatsonis CA, Chen MH, DeAngelis GA, DeBruhl N, Harms S, Heywang-Köbrunner SH, Hylton N, Kuhl CK, Lehman C, Pisano ED, Causer P, Schnitt SJ, Smazal SF, Stelling CB, Weatherall PT, Schnall MD. Magnetic resonance imaging of the breast prior to biopsy. JAMA 2004;292:2735-42. [Crossref] [PubMed]
- Chiarelli AM, Prummel MV, Muradali D, Majpruz V, Horgan M, Carroll JC, Eisen A, Meschino WS, Shumak RS, Warner E, Rabeneck L. Effectiveness of screening with annual magnetic resonance imaging and mammography: results of the initial screen from the ontario high risk breast screening program. J Clin Oncol 2014;32:2224-30. [Crossref] [PubMed]
- Sardanelli F, Giuseppetti GM, Panizza P, Bazzocchi M, Fausto A, Simonetti G, Lattanzio V, Del Maschio A. Italian Trial for Breast MR in Multifocal/Multicentric Cancer. Sensitivity of MRI versus mammography for detecting foci of multifocal, multicentric breast cancer in Fatty and dense breasts using the whole-breast pathologic examination as a gold standard. AJR Am J Roentgenol 2004;183:1149-57. [Crossref] [PubMed]
- Bailey C, Siow B, Panagiotaki E, Hipwell JH, Mertzanidou T, Owen J, Gazinska P, Pinder SE, Alexander DC, Hawkes DJ. Microstructural models for diffusion MRI in breast cancer and surrounding stroma: an ex vivo study. NMR Biomed 2017;30:e3679. [Crossref] [PubMed]
- Baltzer P, Mann RM, Iima M, Sigmund EE, Clauser P, Gilbert FJ, Martincich L, Partridge SC, Patterson A, Pinker K, Thibault F, Camps-Herrero J, Le Bihan D. EUSOBI international Breast Diffusion-Weighted Imaging working group. Diffusion-weighted imaging of the breast-a consensus and mission statement from the EUSOBI International Breast Diffusion-Weighted Imaging working group. Eur Radiol 2020;30:1436-50. [Crossref] [PubMed]
- Choi BH, Baek HJ, Ha JY, Ryu KH, Moon JI, Park SE, Bae K, Jeon KN, Jung EJ. Feasibility Study of Synthetic Diffusion-Weighted MRI in Patients with Breast Cancer in Comparison with Conventional Diffusion-Weighted MRI. Korean J Radiol 2020;21:1036-44. [Crossref] [PubMed]
- Lee SH, Shin HJ, Moon WK. Diffusion-Weighted Magnetic Resonance Imaging of the Breast: Standardization of Image Acquisition and Interpretation. Korean J Radiol 2021;22:9-22. [Crossref] [PubMed]
- Andreassen MMS, Rodríguez-Soto AE, Conlin CC, Vidić I, Seibert TM, Wallace AM, Zare S, Kuperman J, Abudu B, Ahn GS, Hahn M, Jerome NP, Østlie A, Bathen TF, Ojeda-Fournier H, Goa PE, Rakow-Penner R, Dale AM. Discrimination of Breast Cancer from Healthy Breast Tissue Using a Three-component Diffusion-weighted MRI Model. Clin Cancer Res 2021;27:1094-104. [Crossref] [PubMed]
- Galbán CJ, Ma B, Malyarenko D, Pickles MD, Heist K, Henry NL, Schott AF, Neal CH, Hylton NM, Rehemtulla A, Johnson TD, Meyer CR, Chenevert TL, Turnbull LW, Ross BD. Multi-site clinical evaluation of DW-MRI as a treatment response metric for breast cancer patients undergoing neoadjuvant chemotherapy. PLoS One 2015;10:e0122151. [Crossref] [PubMed]
- Yoshikawa MI, Ohsumi S, Sugata S, Kataoka M, Takashima S, Mochizuki T, Ikura H, Imai Y. Relation between cancer cellularity and apparent diffusion coefficient values using diffusion-weighted magnetic resonance imaging in breast cancer. Radiat Med 2008;26:222-6. [Crossref] [PubMed]
- Giannotti E, Waugh S, Priba L, Davis Z, Crowe E, Vinnicombe S. Assessment and quantification of sources of variability in breast apparent diffusion coefficient (ADC) measurements at diffusion weighted imaging. Eur J Radiol 2015;84:1729-36. [Crossref] [PubMed]
- Hatakenaka M, Soeda H, Yabuuchi H, Matsuo Y, Kamitani T, Oda Y, Tsuneyoshi M, Honda H. Apparent diffusion coefficients of breast tumors: clinical application. Magn Reson Med Sci 2008;7:23-9. [Crossref] [PubMed]
- O'Flynn EA, Morgan VA, Giles SL, deSouza NM. Diffusion weighted imaging of the normal breast: reproducibility of apparent diffusion coefficient measurements and variation with menstrual cycle and menopausal status. Eur Radiol 2012;22:1512-8. [Crossref] [PubMed]
- Shin HJ, Kim SH, Lee HJ, Gong G, Baek S, Chae EY, Choi WJ, Cha JH, Kim HH. Tumor apparent diffusion coefficient as an imaging biomarker to predict tumor aggressiveness in patients with estrogen-receptor-positive breast cancer. NMR Biomed 2016;29:1070-8. [Crossref] [PubMed]
- Spick C, Bickel H, Pinker K, Bernathova M, Kapetas P, Woitek R, Clauser P, Polanec SH, Rudas M, Bartsch R, Helbich TH, Baltzer PA. Diffusion-weighted MRI of breast lesions: a prospective clinical investigation of the quantitative imaging biomarker characteristics of reproducibility, repeatability, and diagnostic accuracy. NMR Biomed 2016;29:1445-53. [Crossref] [PubMed]
- Xu J, Jiang X, Li H, Arlinghaus LR, McKinley ET, Devan SP, Hardy BM, Xie J, Kang H, Chakravarthy AB, Gore JC. Magnetic resonance imaging of mean cell size in human breast tumors. Magn Reson Med 2020;83:2002-14. [Crossref] [PubMed]
- Chenevert TL, Stegman LD, Taylor JM, Robertson PL, Greenberg HS, Rehemtulla A, Ross BD. Diffusion magnetic resonance imaging: an early surrogate marker of therapeutic efficacy in brain tumors. J Natl Cancer Inst 2000;92:2029-36. [Crossref] [PubMed]
- Padhani AR, Liu G, Koh DM, Chenevert TL, Thoeny HC, Takahara T, Dzik-Jurasz A, Ross BD, Van Cauteren M, Collins D, Hammoud DA, Rustin GJ, Taouli B, Choyke PL. Diffusion-weighted magnetic resonance imaging as a cancer biomarker: consensus and recommendations. Neoplasia 2009;11:102-25. [Crossref] [PubMed]
- Sternberg EJ, Lipton ML, Burns J. Utility of diffusion tensor imaging in evaluation of the peritumoral region in patients with primary and metastatic brain tumors. AJNR Am J Neuroradiol 2014;35:439-44. [Crossref] [PubMed]
- Medved M, Soylu-Boy FN, Karademir I, Sethi I, Yousuf A, Karczmar GS, Oto A. High-resolution diffusion-weighted imaging of the prostate. AJR Am J Roentgenol 2014;203:85-90. [Crossref] [PubMed]
- Partridge SC, Ziadloo A, Murthy R, White SW, Peacock S, Eby PR, DeMartini WB, Lehman CD. Diffusion tensor MRI: preliminary anisotropy measures and mapping of breast tumors. J Magn Reson Imaging 2010;31:339-47. [Crossref] [PubMed]
- Wu D, Li G, Zhang J, Chang S, Hu J, Dai Y. Characterization of breast tumors using diffusion kurtosis imaging (DKI). PLoS One 2014;9:e113240. [Crossref] [PubMed]
- Onaygil C, Kaya H, Ugurlu MU, Aribal E. Diagnostic performance of diffusion tensor imaging parameters in breast cancer and correlation with the prognostic factors. J Magn Reson Imaging 2017;45:660-72. [Crossref] [PubMed]
- Wang K, Li Z, Wu Z, Zheng Y, Zeng S. E L, Liang J. Diagnostic Performance of Diffusion Tensor Imaging for Characterizing Breast Tumors: A Comprehensive Meta-Analysis. Front Oncol 2019;9:1229. [Crossref] [PubMed]
- Jensen JH, Helpern JA, Ramani A, Lu H, Kaczynski K. Diffusional kurtosis imaging: the quantification of non-gaussian water diffusion by means of magnetic resonance imaging. Magn Reson Med 2005;53:1432-40. [Crossref] [PubMed]
- Raab P, Hattingen E, Franz K, Zanella FE, Lanfermann H. Cerebral gliomas: diffusional kurtosis imaging analysis of microstructural differences. Radiology 2010;254:876-81. [Crossref] [PubMed]
- Westin CF, Knutsson H, Pasternak O, Szczepankiewicz F, Özarslan E, van Westen D, Mattisson C, Bogren M, O'Donnell LJ, Kubicki M, Topgaard D, Nilsson M. Q-space trajectory imaging for multidimensional diffusion MRI of the human brain. Neuroimage 2016;135:345-62. [Crossref] [PubMed]
- Topgaard D. Multidimensional diffusion MRI. J Magn Reson 2017;275:98-113. [Crossref] [PubMed]
- Szczepankiewicz F, Sjölund J, Ståhlberg F, Lätt J, Nilsson M. Tensor-valued diffusion encoding for diffusional variance decomposition (DIVIDE): Technical feasibility in clinical MRI systems. PLoS One 2019;14:e0214238. [Crossref] [PubMed]
- Szczepankiewicz F, van Westen D, Englund E, Westin CF, Ståhlberg F, Lätt J, Sundgren PC, Nilsson M. The link between diffusion MRI and tumor heterogeneity: Mapping cell eccentricity and density by diffusional variance decomposition (DIVIDE). Neuroimage 2016;142:522-32. [Crossref] [PubMed]
- Lasič S, Szczepankiewicz F, Eriksson S, Nilsson M, Topgaard D. Microanisotropy imaging: quantification of microscopic diffusion anisotropy and orientational order parameter by diffusion MRI with magic-angle spinning of the q-vector. Front Phys 2014; [Crossref]
- Cory D, Garroway A, Miller J, editors. Applications of spin transport as a probe of local geometry. Abstracts of Papers of the American Chemical Society; 1990: Amer Chemocal Soc 1155 16th ST, NW, Washington, DC.
- Callaghan PT. Translational Dynamics and Magnetic Resonance: Principles of Pulsed Gradient Spin Echo NMR. 2011. doi:
10.1093/acprof:oso/9780199556984.001.0001 .10.1093/acprof:oso/9780199556984.001.0001 - Naranjo ID, Reymbaut A, Brynolfsson P, Lo Gullo R, Bryskhe K, Topgaard D, Giri DD, Reiner JS, Thakur SB, Pinker-Domenig K. Multidimensional Diffusion Magnetic Resonance Imaging for Characterization of Tissue Microstructure in Breast Cancer Patients: A Prospective Pilot Study. Cancers (Basel) 2021;13:1606. [Crossref] [PubMed]
- Elston CW, Ellis IO. Pathological prognostic factors in breast cancer. I. The value of histological grade in breast cancer: experience from a large study with long-term follow-up. Histopathology 1991;19:403-10. [Crossref] [PubMed]
- Giuliano AE, Connolly JL, Edge SB, Mittendorf EA, Rugo HS, Solin LJ, Weaver DL, Winchester DJ, Hortobagyi GN. Breast Cancer-Major changes in the American Joint Committee on Cancer eighth edition cancer staging manual. CA Cancer J Clin 2017;67:290-303.
- Sjölund J, Szczepankiewicz F, Nilsson M, Topgaard D, Westin CF, Knutsson H. Constrained optimization of gradient waveforms for generalized diffusion encoding. J Magn Reson 2015;261:157-68. [Crossref] [PubMed]
- Szczepankiewicz F, Westin CF, Nilsson M. Maxwell-compensated design of asymmetric gradient waveforms for tensor-valued diffusion encoding. Magn Reson Med 2019;82:1424-37. [Crossref] [PubMed]
- Available online: https://github.com/markus-nilsson/md-dmri
- Nilsson M, Szczepankiewicz F, Lampinen B, Ahlgren A, Martins JP, Lasic S, Westin CF, Topgaard D. An open-source framework for analysis of multidimensional diffusion MRI data implemented in MATLAB. Proc Intl Soc Mag Reson Med 2018;26. Paris, France.
- Goldhirsch A, Winer EP, Coates AS, Gelber RD, Piccart-Gebhart M, Thürlimann B, Senn HJ. Panel members. Personalizing the treatment of women with early breast cancer: highlights of the St Gallen International Expert Consensus on the Primary Therapy of Early Breast Cancer 2013. Ann Oncol 2013;24:2206-23. [Crossref] [PubMed]
- Cohen J. Statistical power analysis for the behavioral sciences. Second edition. 1988.
- Olejnik S, Algina J. Measures of Effect Size for Comparative Studies: Applications, Interpretations, and Limitations. Contemp Educ Psychol 2000;25:241-86. [Crossref] [PubMed]
- Cakir O, Arslan A, Inan N, Anık Y, Sarısoy T, Gumustas S, Akansel G. Comparison of the diagnostic performances of diffusion parameters in diffusion weighted imaging and diffusion tensor imaging of breast lesions. Eur J Radiol 2013;82:e801-6. [Crossref] [PubMed]
- Luo J, Hippe DS, Rahbar H, Parsian S, Rendi MH, Partridge SC. Diffusion tensor imaging for characterizing tumor microstructure and improving diagnostic performance on breast MRI: a prospective observational study. Breast Cancer Res 2019;21:102. [Crossref] [PubMed]
- Eyal E, Shapiro-Feinberg M, Furman-Haran E, Grobgeld D, Golan T, Itzchak Y, Catane R, Papa M, Degani H. Parametric diffusion tensor imaging of the breast. Invest Radiol 2012;47:284-91. [Crossref] [PubMed]
- Jensen JH, Helpern JA. MRI quantification of non-Gaussian water diffusion by kurtosis analysis. NMR Biomed 2010;23:698-710. [Crossref] [PubMed]
- Van Cauter S, Veraart J, Sijbers J, Peeters RR, Himmelreich U, De Keyzer F, Van Gool SW, Van Calenbergh F, De Vleeschouwer S, Van Hecke W, Sunaert S. Gliomas: diffusion kurtosis MR imaging in grading. Radiology 2012;263:492-501. [Crossref] [PubMed]
- Zhou WP, Zan XY, Hu XY, Liu X, Sudarshan SKP, Yang SD, Guo YJ, Fang XM. Characterization of breast lesions using diffusion kurtosis model-based imaging: An initial experience. J Xray Sci Technol 2020;28:157-69. [Crossref] [PubMed]
- Huang Y, Lin Y, Hu W, Ma C, Lin W, Wang Z, Liang J, Ye W, Zhao J, Wu R. Diffusion Kurtosis at 3.0T as an in vivo Imaging Marker for Breast Cancer Characterization: Correlation With Prognostic Factors. J Magn Reson Imaging 2019;49:845-56. [Crossref] [PubMed]
- Chen L, Liu M, Bao J, Xia Y, Zhang J, Zhang L, Huang X, Wang J. The correlation between apparent diffusion coefficient and tumor cellularity in patients: a meta-analysis. PLoS One 2013;8:e79008. [Crossref] [PubMed]
- Makki J. Diversity of Breast Carcinoma: Histological Subtypes and Clinical Relevance. Clin Med Insights Pathol 2015;8:23-31. [Crossref] [PubMed]
- Gangadhar K, Santhosh D, Fatterpekar G. Imaging Features of Intracranial Meningiomas with Histopathological Correlation: A Relook into Old Disease. Nep J Radiol 2013;3:14-32. [Crossref]
- Langbein BJ, Szczepankiewicz F, Westin CF, Bay C, Maier SE, Kibel AS, Tempany CM, Fennessy FM. A Pilot Study of Multidimensional Diffusion MRI for Assessment of Tissue Heterogeneity in Prostate Cancer. Invest Radiol 2021; Epub ahead of print. [Crossref] [PubMed]
- Ling YH, Chen JW, Wen SH, Huang CY, Li P, Lu LH, Mei J, Li SH, Wei W, Cai MY, Guo RP. Tumor necrosis as a poor prognostic predictor on postoperative survival of patients with solitary small hepatocellular carcinoma. BMC Cancer 2020;20:607. [Crossref] [PubMed]
- Michor F, Polyak K. The origins and implications of intratumor heterogeneity. Cancer Prev Res (Phila) 2010;3:1361-4. [Crossref] [PubMed]
- Visvader JE. Cells of origin in cancer. Nature 2011;469:314-22. [Crossref] [PubMed]
- Januškevičienė I, Petrikaitė V. Heterogeneity of breast cancer: The importance of interaction between different tumor cell populations. Life Sci 2019;239:117009. [Crossref] [PubMed]
- McGranahan N, Swanton C. Biological and therapeutic impact of intratumor heterogeneity in cancer evolution. Cancer Cell 2015;27:15-26. [Crossref] [PubMed]
- Szczepankiewicz F, Lasič S, van Westen D, Sundgren PC, Englund E, Westin CF, Ståhlberg F, Lätt J, Topgaard D, Nilsson M. Quantification of microscopic diffusion anisotropy disentangles effects of orientation dispersion from microstructure: applications in healthy volunteers and in brain tumors. Neuroimage 2015;104:241-52. [Crossref] [PubMed]
- Baltzer PA, Schäfer A, Dietzel M, Grässel D, Gajda M, Camara O, Kaiser WA. Diffusion tensor magnetic resonance imaging of the breast: a pilot study. Eur Radiol 2011;21:1-10. [Crossref] [PubMed]
- Tsougos I, Svolos P, Kousi E, Athanassiou E, Theodorou K, Arvanitis D, Fezoulidis I, Vassiou K. The contribution of diffusion tensor imaging and magnetic resonance spectroscopy for the differentiation of breast lesions at 3T. Acta Radiol 2014;55:14-23. [Crossref] [PubMed]
- Jiang R, Zeng X, Sun S, Ma Z, Wang X. Assessing Detection, Discrimination, and Risk of Breast Cancer According to Anisotropy Parameters of Diffusion Tensor Imaging. Med Sci Monit 2016;22:1318-28. [Crossref] [PubMed]
- Pierpaoli C, Jezzard P, Basser PJ, Barnett A, Di Chiro G. Diffusion tensor MR imaging of the human brain. Radiology 1996;201:637-48. [Crossref] [PubMed]
- De Santis S, Drakesmith M, Bells S, Assaf Y, Jones DK. Why diffusion tensor MRI does well only some of the time: variance and covariance of white matter tissue microstructure attributes in the living human brain. Neuroimage 2014;89:35-44. [Crossref] [PubMed]
- Lu S, Ahn D, Johnson G, Cha S. Peritumoral diffusion tensor imaging of high-grade gliomas and metastatic brain tumors. AJNR Am J Neuroradiol 2003;24:937-41. [PubMed]
- Tafreshi NK, Kumar V, Morse DL, Gatenby RA. Molecular and functional imaging of breast cancer. Cancer Control 2010;17:143-55. [Crossref] [PubMed]
- Zhou LQ, Wu XL, Huang SY, Wu GG, Ye HR, Wei Q, Bao LY, Deng YB, Li XR, Cui XW, Dietrich CF. Lymph Node Metastasis Prediction from Primary Breast Cancer US Images Using Deep Learning. Radiology 2020;294:19-28. [Crossref] [PubMed]
- Szczepankiewicz F, Westin CF, Nilsson M. Gradient waveform design for tensor-valued encoding in diffusion MRI. J Neurosci Methods 2021;348:109007. [Crossref] [PubMed]
- Henriques RN, Jespersen SN, Shemesh N. Correlation tensor magnetic resonance imaging. Neuroimage 2020;211:116605. [Crossref] [PubMed]
- Li S, Zheng Y, Sun W, Lasič S, Szczepankiewicz F, Wei Q, Han S, Zhang S, Zhong X, Wang L, Li H, Cai Y, Xu D, Li Z, He Q, van Westen D, Bryskhe K, Topgaard D, Xu H. Glioma grading, molecular feature classification, and microstructural characterization using MR diffusional variance decomposition (DIVIDE) imaging. Eur Radiol 2021;31:8197-207. [Crossref] [PubMed]
- Lundell H, Nilsson M, Dyrby TB, Parker GJM, Cristinacce PLH, Zhou FL, Topgaard D, Lasič S. Multidimensional diffusion MRI with spectrally modulated gradients reveals unprecedented microstructural detail. Sci Rep 2019;9:9026. [Crossref] [PubMed]
- Andersen KW, Lasič S, Lundell H, Nilsson M, Topgaard D, Sellebjerg F, Szczepankiewicz F, Siebner HR, Blinkenberg M, Dyrby TB. Disentangling white-matter damage from physiological fibre orientation dispersion in multiple sclerosis. Brain Commun 2020;2:fcaa077.