Epicardial and pericardial fat analysis on CT images and artificial intelligence: a literature review
Introduction
The compartments of adipose tissue surrounding the heart are metabolically active organs. In recent years, a relationship has been demonstrated between epicardial adipose tissue (EAT) and heart diseases including coronary artery disease, heart failure with preserved ejection fraction, and atrial fibrillation (1-3). Obesity could cause unbalanced adipogenesis in EAT resulting in secretion of proinflammatory cytokines. Furthermore, the paracrine activity of the adipose tissue seems to be affected by other changes of hormonal status such as diabetes mellitus or menopause (4-7).
The metabolic syndrome (MetS) comprised of obesity, hyperglycemia, hypertension and atherogenic dyslipidemia, can lead to proinflammatory state and is a risk factor for cardiovascular diseases (Figure 1) (8). Moreover, the accumulation of visceral adipose tissue associated with MetS, non-alcoholic fatty liver disease (NAFLD) and EAT has been advocated as biomarker of cardiometabolic risk (6,9-11).
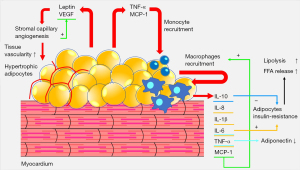
Computed tomography (CT) and magnetic resonance imaging (MRI) allow for non-invasive quantification of adipose tissue depots, including pericardial fat (12-17). However, quantification of pericardial adipose tissue depots is rarely performed outside research setting. Indeed, while CT is in the literature the most validated and reproducible technique for fat quantification, given the higher spatial resolution compared to MRI (18), many practical challenges remain.
When quantification is performed, manual segmentation of epicardial fat volume (EFV) quantification is today the method of choice (18). Nevertheless, this approach requires a laborious stepwise manual segmentation of relevant anatomical structures, being therefore operator-dependent and time-consuming, not suitable for routine clinical practice (18). Therefore, development of computer-assisted tools aimed at tissue segmentation and quantification is of paramount importance to improve consistency of results and reduce processing times (18).
In this context, artificial intelligence (AI) solutions, including machine learning and deep learning, has been recently proposed as solution to obtain fast, automatic, and reliable measures of abdominal adipose tissue on CT and MRI (19-24).
Machine learning is a group of techniques allowing to extrapolate or classify models through computer learning (19-24). These algorithms have the potential to offer robust and feasible solutions to quantify adipose tissue compartments of the human body, including epicardial and pericardial fat, possibly enhancing, in an opportunistic fashion, the value of images acquired for other purposes.
The present literature review will be focused on AI supervised methods for epicardial and pericardial fat analysis using CT Images. Neither other imaging modalities (such as ultrasound or MRI) nor other adipose tissue compartments (such as paracardial adipose tissue) will be addressed in detail.
The aim of this paper is to provide an overview of the available evidence on AI algorithms proposed for the segmentation and quantification of epicardial and pericardial adipose tissues on CT images.
We present the following article in accordance with the Narrative Review reporting checklist (available at https://dx.doi.org/10.21037/qims-21-945).
Anatomy and physiology
The adipose tissue surrounding the heart is divided into several compartments (Figures 2,3). EAT is the adipose tissue localized between the myocardium and the visceral layer of the pericardium. The contiguity between epicardial fat and myocardium is an important anatomical feature, given the epicardial tissue distinct properties. Within the EAT there is the pericoronary adipose tissue (PCAT), consisting of adipose tissue located in a radial distance to the external wall of the vessel equal to the diameter of the coronary vessel (25). PCAT is contiguous with the adventitial layer in large vessels, while adipocytes are present in vascular wall of small vessels and microvessels (3). The pericardial adipose tissue is located outside the EAT. It is lined internally by the visceral layer (located between the EAT and the pericardial adipose tissue) and externally by the parietal layer, located between the pericardial adipose tissue and the paracardial adipose tissue. Hence, from the inner to the outer layer this anatomy can be summarized with the following scheme: ((((((heart) perivascular adipose tissue) EAT) [pericardial adipose tissue = PCAT + EAT + pericardial adipose tissue] pericardial sac) paracardial adipose tissue) mediastinum).
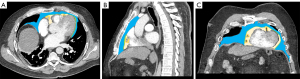
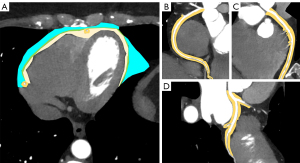
EAT covers 80% of cardiac surface, with a greater concentration in the atrioventricular sulci, interventricular sulci and around the epicardial coronary arteries (26,27). Epicardial adipocytes are smaller in size than adipocytes of the subcutaneous and visceral adipose tissue. EAT also contains immune, stromal, inflammatory, nodal, and nerve tissue cells (4,28) (Figures 2,3).
There are several known physiological functions of EAT related to cardiac physiology. It holds a protective function for the heart and a support for the coronary arteries against the torsion caused by the arterial pulse and cardiac contraction (29). Moreover, EAT derives from the same (splanchnopleuric) mesoderm as brown adipose tissue and omental fat cells.
It is also a barrier against pathogens through immune activity, protects against hypothermia thanks to the activity of adipocytes that have brown or beige characteristics, adipocytes typically involved in thermogenesis, and regulates the energy supply to the myocardium through lipolytic and lipogenic activities (30,31).
Furthermore, it is now known that EAT is an endocrine organ having a unique secrotome. In fact, it exerts a paracrine and vasocrine activity on the myocardium and coronary arteries through the release of adipokines with vasodilator, antioxidant, antifibrotic and anti-inflammatory activities (11,32). Indeed, EAT and pericardial adipose tissue correlate with several markers of oxidative stress, inflammation, and vascular dysfunction, underlining the importance of pericardial adipose tissue segmentation in addition to the other compartments (33).
Taken together, these arguments underline that adipose tissue surrounding the heart is of anatomical and physiological paramount importance.
AI
Machine learning
Machine learning is based in algorithms that enable computers to learn and predict, possibly improving according to experience (19). These sophisticated algorithms operate on large-scale heterogeneous data sets with the aim of discovering helpful patterns that would be hard or impossible to detect even for well-trained individuals (34).
Machine learning is subdivided into three categories according to the level of human supervision: supervised, unsupervised and reinforcement learning (19).
In supervised machine learning, training is supervised. In this category the outputs are labeled by human experts, while the algorithm associates the inputs to the outputs. In unsupervised machine learning, the computer finds hidden structures of data and separates it into groups. The first subcategory provides data labels to the algorithm, while the second does not (19). In reinforcement machine learning, interactions with the environment provide computer learning that receives negative or positive reinforcement feedback (19).
These approaches can have profound implications for the diagnosis and treatment of diseases (34). The collection of specific data such as genomic testing for personalized cancer therapies, high-resolution two- (2D) and three-dimensional (3D) anatomical imaging or histological analyses, provide basis for future medicine based on early and precise diagnoses, personalized treatments and improve health assessment (34,35).
Artificial neural networks
Artificial neural networks are mathematical and statistical models that process information through elements called neurons connected to each other by multiple connections (i.e., nodes and edges), with a structure inspired by the biological nervous system (19). This network is made up of input layers, hidden layers, and an output layer. Through these three levels the information is respectively entered, processed, and issued (19,36). This type of network can be achieved by three categories of learning: supervised, partially supervised, or unsupervised (19).
Deep learning
Deep learning consists of a subset of neural network algorithms in which there are several hidden levels. The different deep learning models are 1D convolutional neural networks (CNNs) in DL framework, such as pytorch and tensorflow, 2D model or 3D model. These two models are fully connected neural network and CNNs respectively, being the latter learning model the most used technique in medical imaging analyses.
Deep learning applies a different learning method of raw data than machine learning, in fact, the computational models of deep learning are composed by multiple processing layers that learn data representation with multiple levels of abstraction (21).
Deep learning also differs from artificial neural networks according to the number of hidden layers, connections, and ability to learn significative abstractions of the inputs. Indeed, artificial neural networks consist of three layers and are trained to gain supervised representations that are improved for specific assignments (37). The deep learning system instead receives data from an underlying layer and creates a representation of the observed models by improving a local unsupervised criterion (38). A characteristic of deep learning is that these layers of features are learned from the data using a learning procedure (39).
Methods
The literature research was carried out on March 2021 using MEDLINE PubMed Central. Only articles written in English, without limits of time span, were included. The keywords used for articles search were: “adipose tissue artificial intelligence”, “adipose tissue deep learning” or “adipose tissue machine learning”. Relevant articles related to EAT, pericardial adipose tissue and AI were selected also from the reference list of each identified article.
The title and abstract of all the articles of potential interest to our topic were screened, without any specific exclusion criteria. After this screening we filtered the literature search and selected 11 appropriate original articles for further review.
The articles investigating EAT, pericardial adipose tissue together with mediastinal adipose tissue were included in this manuscript, focusing our analysis on the former two.
AI: epicardial and pericardial adipose tissues
In recent years, several research studies investigated the automatic segmentation of EAT on CT (Figures 2,3) using machine learning and deep learning algorithms. Most of these studies showed a level of evidence of 3. The major characteristics of studies are summarized in Tables 1,2.
Table 1
Authors | AI category | Adipose tissue compartments | Algorithm | Accuracy | Number of patients | Dice score | Level of evidence* |
---|---|---|---|---|---|---|---|
Rodrigues et al. [2015] (40) | Machine learning | EAT, mediastinal adipose tissue | J84Graft | 99.00% | 20 | 96.80% | 3 |
Random Forest | 98.90% | ||||||
REPTree | 98.90% | ||||||
J84 | 98.90% | ||||||
SimpleCart | 98.90% | ||||||
SMO | 98.30% | ||||||
RandomTree | 97.50% | ||||||
RBFNetwork | 96.80% | ||||||
Spegasos | 96.80% | ||||||
DecisionStump | 96.80% | ||||||
HyperPipes | 94.80% | ||||||
NaiveBayes | 86.00% | ||||||
Rodrigues et al. [2017] (41) | Machine learning | EAT, mediastinal adipose tissue | Rotation Forest + | – | 20 | – | 3 |
MLP Regressor | 98.70% | ||||||
RBF Regressor | 98.60% | ||||||
MLP Regressor | 98.50% | ||||||
SMO Regressor | 98.50% | ||||||
Rotation Forest + | – | ||||||
Random Forest | 98.20% | ||||||
Additive Regressor + Random Forest | 98.10% | ||||||
k-NN/IBk | 98.00% | ||||||
Random Forest | 97.60% | ||||||
M5P | 96.90% | ||||||
Alternating | – | ||||||
Model Tree | 96.90% | ||||||
M5 Rules | 96.80% | ||||||
Linear Regression | 95.30% | ||||||
Extra Tree | 95.10% | ||||||
LeastMedSq | 94.90% | ||||||
Elastic Net | 94.90% | ||||||
REP Tree | 94.30% | ||||||
Random Tree | 93.40% | ||||||
Priyaet al. [2019] (42) | Machine learning | EAT, mediastinal adipose tissue, pericardial adipose tissue | Proposed methodology | EAT 98.5% | 20 | EAT 98.7% | 3 |
Mediastinal adipose tissue 98.4% | Mediastinal adipose tissue 98.2% | ||||||
Pericardial adipose tissue 96.4% | Pericardial adipose tissue 98.5% | ||||||
Commandeuret al. [2018] (43) | Deep learning | EAT, thoracic adipose tissue | ConvNets | EAT 82.3%; thoracic adipose tissue 90.5% | 250 | 95.3%±0.5% obtained for a threshold ts =53.1%±5.1% | 3 |
Commandeuret al. [2019] (44) | Deep learning | EAT | ConvNets | – | 70 | 87.30% | 3 |
Bandekaret al. [2006] (45) | Machine learning | Pericardial adipose tissue | Fuzzy affinity-based framework | Pericardial adipose tissue 99.13%±0.38% | 23 | – | 3 |
*, SIGN100: Scottish Intercollegiate Guidelines Network 2019. CT, computed tomography; AI, artificial intelligence; EAT, epicardial adipose tissue.
Table 2
Authors | AI category | Algorithm and/or software | Adipose tissue compartments | Data | Correlation | Number of patients | Results | Level of evidence* |
---|---|---|---|---|---|---|---|---|
Kroll et al. [2021] (46) | Deep learning | U-Net 3D | EAT | EAT Volume | Calcium artery score | 966 | r=0.18 | 3 |
Paracardialadipose tissue | EAT Attenuation | r=−0.09 | ||||||
Visceral adipose tissue | ||||||||
Intermuscularadipose tissue | ||||||||
Subcutaneous adipose tissue | ||||||||
Eisenberg et al. [2020] (47) | Deep learning | Fully automated DL algorithm incorporated into QFAT research software (version 2.0) | EAT | EAT Volume | Major adverse cardiovascular events | 2,068 | P<0.01 | 1 |
EAT Attenuation | P=0.01 | |||||||
Oikonomouet al. [2019] (48) | Machine learning | Aquarius Workstation V.4.4.11-13, TeraRecon Inc., Foster City, CA, USA for basic segmentation and CaRi-HEART proprietary algorithms | Perivascular adipose tissue | Fat attenuation index | Inflammation | 167, 1,575, 44 | See Figure 4 for inflammation, vascularity and fibrosis | 1, 3, 3 |
Caristo Diagnostics Ltd, Oxford, UK | Fat radiomic profile | Vascularity | ||||||
Slicer Radiomics extension which incorporates the Pyradiomics library into 3D Slicer (v.4.9.0-2017-12-18 r26813) | Fibrosis | |||||||
Major adverse cardiovascular events | P<0.001 | |||||||
Perivascular changes related to acute myocardial infarction | P<0.001 | |||||||
Lin et al. [2021] (49) | Deep learning | QFAT v2.0, CSMC, Los Angeles, CA, USA | EAT | EAT volume | Major adverse cardiovascular events | 2,068 | P<0.001 | 2 |
NAFLD | EAT attenuation | P<0.001 | ||||||
Hepatic attenuation | P=0.003 | |||||||
Tamarappooet al. [2021] (50) | Deep learning | QFAT v2.0, CSMC, Los Angeles, CA, USA | EAT | EAT volume | Long-term risk prediction for cardiac events | 1,069 | P<0.0001 | 1 |
EAT attenuation | P=0.002 |
*, SIGN100: Scottish Intercollegiate Guidelines Network 2019. CT, computed tomography; AI, artificial intelligence; EAT, epicardial adipose tissue; NAFLD, non-alcoholic fatty liver disease.
A common path across studies is that AI-derived algorithms are often validated using the Dice similarity coefficient. This indicator is a statistical method that is used to reliably measure the similarity between two sets of data (Figure 4). Another indicator commonly applied is the accuracy, a fraction of true positive and true negative predicted instances among all instances (51) (Figure 4).
One of the first studies assessing a technique for the segmentation of the fat surrounding the heart compartments was reported by Rodrigues et al. (40). They divided the adipose tissue surrounding the heart into two compartments: the EAT (internal compartment) and the mediastinal adipose tissue (external compartment). The authors proposed an intersubjective registration consisting of multiple steps and based on the creation of an atlas that is subsequently moved over any cardiac CT image with range of Hounsfield unit (HU) of interest. Consequently, the similarity score was calculated and confirmation by heuristic method was added to reinforce the chosen position. For training the algorithm, a series of reliable data was provided, obtained from the manual segmentation of adipose tissue compartments of 10 male and 10 female patients. The model of automatic segmentation proposed by Rodrigues et al. showed a mean epicardial and mediastinal adipose tissue quantification accuracy of 98.4% and a mean true positive rate of 96.2%. Furthermore, for to the quantification of EAT, the model demonstrated a rate of successful automatic segmentations of 100%, a Dice score of 97.9%, and a true positive rate of 98.3% (40). However, this study was performed with an atlas-based, and not AI-based, method.
Rodrigues et al. proposed another method of epicardial, and mediastinal adipose tissue segmentation based on regression algorithms (41). The authors analyzed images according to the quantity of red pixels (i.e., epicardial fat), green pixels (i.e., mediastinal fat), blue pixels (i.e., pericardium), gray pixels (i.e., areas of interest to be segmented), black pixels (i.e., areas not to be segmented), the quantity of scan images and the index of the slice to be segmented. For the prediction of mediastinal adipose tissue, they reported a correlation coefficient of 0.9876, calculated with Rotation Forest algorithm with Multilayer Perceptron (MLP) Regressor, with a relative absolute error of 14.4% (root relative squared error of 15.7%) (41).
Priya et al. proposed an accurate method of adipose tissue segmentation using a Fruitfly Algorithm based on Modified region growing algorithm applied on CT images (42). Ground truth images of fat depots was provided as input to the neural network classifier, trained to distinguish a fat-infested image from a non-fat one image. Each pixel of the image was classified as epicardial or mediastinal. Finally, the segmented image was used as a source to distinguish epicardial or mediastinal adipose tissue based on the interpolation between pixels. This segmentation methodology based on the optimal neural network showed excellent results presenting with a Dice cross-correlation of 98.7 for epicardial fat, 98.2 for mediastinal fat and 98.5 for pericardium (42).
Moreover, Commandeur et al. proposed a deep learning method for the quantification of EAT and thoracic adipose tissue on coronary calcium scoring CT scans based on CNN ConvNets (43). The segmentation of the adipose tissue was performed on axial slices, provided as input in a first network Net1. Then, the hidden convolutional unit was used to define the thoracic mask and differentiate pericardial and epicardial masks. A second Net2 network detected the pericardium. The epicardial mask obtained from Net1 was used to perform a radial sampling and modify the input CT slice from Cartesian coordinates to cylindrical coordinates. The transformed input was then supplied to Net2 which resulted in an output probability map of the pericardial sac. Finally, post-processing including HU threshold values (e.g., −190, −30 HU) to remove non-fat pixels and median filtering, provided binary images for quantification of adipose tissue. The authors reported a strong correlation between automatic and manual segmentation, with Dice scores of 0.924 and 0.945 for EAT and thoracic adipose tissue volumes, respectively (43).
Subsequently, Commandeur et al. conducted a multicenter study aimed to the quantification of EAT on non-contrast calcium-scoring CT scans using deep learning method from multiple cohorts, scanners, and protocols (n=850) (44). This deep learning model was implemented by using CNN with the TensorFlow framework, version 1.10.1 (http://www.Tensorflow.org), and the Keras library, version 2.2.2 (https://keras.io). Using this network, the lower and upper limits of the heart are recognized, and the pericardium is segmented. The EAT is quantified through three axial sections: the classification and segmentation section and the previous and subsequent sections. In addition, post-processing consisting of median filtering and the HU threshold for adipose tissue is applied. This study reported high correlation between deep learning quantification and manual segmentations of two experienced tracers (r=0.973 and r=0.979; P<0.001). Furthermore, a strong correlation was also demonstrated between deep learning EAT progression and manual EAT progression in 70 patients (r=0.905; P<0.001) (44).
Fully automatic deep learning method was adopted by Eisenberg et al. to quantify EAT volume and attenuation measures (HU) from non-contrast coronary CT to predict major adverse cardiovascular events, considered as myocardial infarction, late revascularization (>180 days) and cardiac death (47). Interestingly, they showed a correlation of major adverse cardiovascular events with the increased EAT volumes [hazard ratio, 1.03 (95% CI: 1.01–1.04), P<0.01] and with decreased EAT mitigation measures [hazard ratio, 0.83 (95% CI: 0.72–0.96); P=0.01]. Moreover, EAT volume correlated with serum levels of C-reactive protein, D-dimer, adiponectin, myoglobin, plasminogen activator inhibitor-1 and myeloperoxidase (P<0.01). These serum biomarkers together with interleukin-6, endothelial cell-selective adhesion molecule and matrix metalloproteinase-9 showed an inverse correlation with EAT attenuation (P<0.05). This study robustly demonstrated how fully automated deep learning analysis of EAT volume and EAT attenuation can provide important clinical information in asymptomatic patients (47).
Kroll et al. examined a method for fully automatic quantification of epicardial and paracardial adipose tissues for possible use in cardiovascular risk assessment (46). A deep learning network was used for quantification of adipose tissue from coronary CT in 966 patients with intermediate Framingham risk scores. The deep learning architecture used was an evolution of the system described by Koitka et al. (52). The body was segmented into semantic regions using a multi-resolution U-Net 3D network. HU thresholds ranging from −190 to −30 and −29 to −150 were used to segment adipose tissue (subcutaneous adipose tissue, visceral adipose tissue, intermuscular adipose tissue, EAT and paracardial adipose tissue, respectively) and muscle tissue. The Dice score on the test set showed the following values: subcutaneous tissue, 0.97; muscle, 0.96; thoracic cavity, 0.98; mediastinum, 0.90 and pericardium, 0.96. The results of this study showed no correlation between the volume and density of EAT and paracardial adipose tissue with the coronary artery calcium score. EAT and pericardial adipose tissue volume and attenuation were not significantly correlated with Agatston score: EAT volume r=0.19, EAT attenuation r=−0.09, pericardial adipose tissue volume r=0.25 and pericardial adipose tissue attenuation r=−0.14. Because EAT and pericardial adipose tissue did not show a significant correlation when compared with coronary artery calcium, these parameters should not be used as a surrogate marker for coronary artery calcium.
No cut-off scores were identified in EAT and pericardial adipose tissue features for high-risk coronary artery disease (46).
Bandekar et al. presented another method for the automatic quantification and classification of pericardial adipose tissue (45). The study performed on 23 subjects was divided into two phases: training phase and deployment phase. Using a training dataset in the first phase, object-specific distributions (fat, non-fat, background classes) were estimated. The second phase consisted of multiple steps mainly to remove artifacts, automatically detect the body contour to process data within the contour, segment and label the different organs/tissues. The results of this study were compared with manual segmentation and showed a mean accuracy for pericardial adipose tissue of 99.13%±0.38%, a mean true negative rate of 99.28%±0.33% and a mean true positive rate of 85.63%±7.42% (45).
Oikonomou et al. analyzed the radiomic profile of the perivascular adipose tissue using coronary CT through a machine learning-derived radiotranscriptomic signature (48). The authors performed a fundamental work composed by three different analyses:
- Study 1: in this study, 167 patients performed coronary CT and underwent cardiac surgery. Adipose tissue biopsies were performed during surgery to look for a relationship between the expression of genes that characterized inflammation, fibrosis, and vascularity and radiotranscriptomic evaluation. Gene expression was evaluated by tumor necrosis factor α (TNF-α) for inflammation, COL1A1 for fibrosis and endothelial-marker platelet and endothelial cell adhesion marker CD31 (PECAM1) for vascularity.
- Study 2: then, a case-control study was designed to distinguish the perivascular adipose tissue of individuals with increased risk for major adverse cardiac events and compare it with radiotranscriptomic signature of inflammation, fibrosis, and vascularity. The groups consisted of patients with a major adverse cardiac event within 5 years and patients who had no cardiac events in the same period. The radiomic signature of high-risk perivascular adipose tissue [fat radiomic profile (FRP)] was trained and validated by scans randomly divided into training/internal validation (80%) and an external validation set (20%), to discriminate high-risk cases from low-risk cases. Consequently, FRP was explored in 1,575 patients to verify if it could add value in cardiac risk prediction beyond traditional risk factors.
- Study 3: finally, a prospective evaluation was performed to assess the ability of FRP to monitor perivascular changes related to acute myocardial infarction. Forty-four patients with coronary CT performed 96 hours before and 6 months after the ischemic event were evaluated. A total of 843 radiomic features were calculated for each adipose tissue segmentation using the Slicer Radiomics extension which incorporates the Pyradiomics library into 3D Slicer (v.4.9.0-2017-12-18 r26813).
The results of the three studies are summarized below:
- Study 1: the fat attenuation index (FAI) was a performing metric for the evaluation of adipose tissue inflammation. The radiomic plot showed a comparable or greater performance than FAI in detecting fibrosis and vascularity, but not inflammation, suggesting that adipose tissue phenotyping constitutes a non-invasive model for detecting fibrosis and microvascular remodeling of the adipose tissue (Figure 5).
- Study 2: radiomic phenotyping of perivascular adipose tissue produced 1,686 radiomic features (843 features around each vessel). The stability analysis identified 1,391 features with an intra-class correlation coefficient of 0.9. In the case-control evaluation the univariable receiver operating characteristic (ROC) analysis highlighted a total of 22, 86 and 241 of the 1,391 stable radiomic characteristics discriminating cases with major adverse cardiac events from non-major adverse cardiac events (P<0.001, P<0.01, and P<0.05, respectively). Moreover, FRP showed association with the adjusted risk of major adverse cardiac events [per 0.01 increments: adjusted hazard ratio (HR) 1.12 (95% CI: 1.08–1.15), P<0.001].
- Study 3: higher FRP (i.e., adverse perivascular adipose tissue remodeling) values were found in 44 acute myocardial infarction patients undergoing coronary CT within 96 hours of admission compared to 44 matched controls undergoing coronary CT. Dynamic changes in perivascular FAI around the right coronary artery were seen at 6 months. Furthermore, in a subclass of 10 patients with ST elevation myocardial infarction these changes were pronounced in the region adjacent to the culprit lesion (50). These studies applied an atlas-based segmentation and not AI-based segmentation.
Recently, Lin et al. evaluated the association of MetS with CT-derived cardiometabolic biomarker (NAFLD and EAT) with long-term risk of major adverse cardiovascular events in asymptomatic subjects (49). Volumetry and attenuation of EAT were measured using a fully automated deep learning algorithm included into research software (QFAT v2.0, CSMC, Los Angeles, CA, USA). The final population consisted of 2,068 participants. Subjects with MetS had a higher prevalence of NAFLD (26.0% vs. 9.9%), a greater amount of EAT (114.1 vs. 73.7 cm3), and low attenuation values of EAT (−76.9 vs. −73.4 HU, all P<0.001) compared to subjects without MetS. At 14±3 years, major adverse cardiovascular events occurred in 10.8% of subjects. Multivariable Cox analysis highlighted that NAFLD was predictive for major adverse cardiovascular events independent of EAT measures, coronary artery calcium score and MetS [HR 1.78 (95% CI: 1.21–2.61), P=0.003]. The addition of the EAT volume significantly improved the reclassification for major adverse cardiovascular events (22% over atherosclerotic cardiovascular disease risk score; 17% over atherosclerotic cardiovascular disease risk score plus coronary artery calcium score) (50).
Lastly, Tamarappoo et al. proposed a machine learning model integrating quantitative CT measures, clinical risk factors, and circulating biomarkers to provide a better long-term prediction of cardiac events than current risk assessment. As in the study by Lin et al., EAT was quantified by non-contrast CT using a fully automated deep learning algorithm (QFAT version 2.0, CSMC, Los Angeles, CA). The results of this study demonstrated a statistically significant difference between calcium artery score, EAT volume and EAT attenuation between patients without and with cardiac events (P<0.0001, P<0.0001 and P=0.002 respectively) (50).
Taken together, studies on the topic underline that investigating adipose tissue surrounding the heart with AI-based methods can be a robust and reliable approach, offering novel metrics that should be further tested to understand whether they can contribute to improve the current cardiovascular risk-assessment, follow-up, and treatments monitoring.
AI and body composition on cardiovascular imaging: perspectives
Non-invasive cardiac imaging using CT and MR has significantly evolved in the last decade, delivering anatomical and functional information at increasing superior levels. This is at least partially due to technological advances pairing advanced imaging methods with software solutions to extract more information from acquired images. As such, AI has already led to the introduction of potential new biomarkers like perivascular fat infiltration (48). This shows the potential of software techniques to extract from every CT or MRI scan “hidden” data regarding tissue characteristics that can be measured, including muscle mass and density, visceral and subcutaneous fat, liver fat, and vascular calcifications (53,54). Moreover, advanced analysis of medical images might provide insights into the interplay between functional phenotypes and genetic fingerprints in health and disease. In this respect AI solutions, such as machine learning and deep learning, can be helpful for researchers and physicians to improve classic disease definitions, genomic discovery, deep phenotyping, predict outcomes more precisely, and enhance our understanding of genetic and environmental basis of several disorders, including cardiovascular, with a potential positive impact on patient-tailored drug development (55).
There is growing interest in applying AI solutions in the context of opportunistic use of imaging data, to make this application feasible, fast, reproducible, and reliable across patients. It is likely that soon the value of epicardial and pericardial fat analysis on CT images will be empowered by AI, possibly becoming a clinical routine approach.
Conclusions
AI is a revolutionary innovation allowing for quantitative and qualitative analysis of EAT and pericardial adipose tissue. These algorithms enable researchers and physicians to obtain important data at minimal or no additional cost to the healthcare system and almost no additional workload to physicians. The current literature showed that investigating adipose tissue surrounding the heart with AI-based methods can be a feasible, robust and reliable approach to obtain novel metrics that will be used in clinical practice. Information including quantitative and qualitative data of EAT and pericardial adipose tissue can have an impact on cardiovascular disorders, possibly enhancing risk stratification, pathogenesis understanding, prediction of clinical outcomes and overall morbidity and mortality. Application of AI to cardiovascular images might help to prevent future adverse events, and support predictive and precision medicine strategies to improve patients’ quality of life.
Acknowledgments
Funding: None.
Footnote
Reporting Checklist: The authors have completed the Narrative Review reporting checklist. Available at https://dx.doi.org/10.21037/qims-21-945
Conflicts of Interest: All authors have completed the ICMJE uniform disclosure form (available at https://dx.doi.org/10.21037/qims-21-945). CAM serves as an unpaid editorial board member of Quantitative Imaging in Medicine and Surgery. The other authors have no conflicts of interest to declare.
Ethical Statement: The authors are accountable for all aspects of the work in ensuring that questions related to the accuracy or integrity of any part of the work are appropriately investigated and resolved.
Open Access Statement: This is an Open Access article distributed in accordance with the Creative Commons Attribution-NonCommercial-NoDerivs 4.0 International License (CC BY-NC-ND 4.0), which permits the non-commercial replication and distribution of the article with the strict proviso that no changes or edits are made and the original work is properly cited (including links to both the formal publication through the relevant DOI and the license). See: https://creativecommons.org/licenses/by-nc-nd/4.0/.
References
- Le Jemtel TH, Samson R, Ayinapudi K, Singh T, Oparil S. Epicardial Adipose Tissue and Cardiovascular Disease. Curr Hypertens Rep 2019;21:36. [Crossref] [PubMed]
- Mahabadi AA, Lehmann N, Kälsch H, Bauer M, Dykun I, Kara K, Moebus S, Jöckel KH, Erbel R, Möhlenkamp S. Association of epicardial adipose tissue and left atrial size on non-contrast CT with atrial fibrillation: the Heinz Nixdorf Recall Study. Eur Heart J Cardiovasc Imaging 2014;15:863-9. [Crossref] [PubMed]
- Goeller M, Achenbach S, Marwan M, Doris MK, Cadet S, Commandeur F, Chen X, Slomka PJ, Gransar H, Cao JJ, Wong ND, Albrecht MH, Rozanski A, Tamarappoo BK, Berman DS, Dey D. Epicardial adipose tissue density and volume are related to subclinical atherosclerosis, inflammation and major adverse cardiac events in asymptomatic subjects. J Cardiovasc Comput Tomogr 2018;12:67-73. [Crossref] [PubMed]
- Mazurek T, Zhang L, Zalewski A, Mannion JD, Diehl JT, Arafat H, Sarov-Blat L, O'Brien S, Keiper EA, Johnson AG, Martin J, Goldstein BJ, Shi Y. Human epicardial adipose tissue is a source of inflammatory mediators. Circulation 2003;108:2460-6. [Crossref] [PubMed]
- Packer M. Epicardial Adipose Tissue May Mediate Deleterious Effects of Obesity and Inflammation on the Myocardium. J Am Coll Cardiol 2018;71:2360-72. [Crossref] [PubMed]
- Villasante Fricke AC, Iacobellis G. Epicardial Adipose Tissue: Clinical Biomarker of Cardio-Metabolic Risk. Int J Mol Sci 2019;20:5989. [Crossref] [PubMed]
- El Khoudary SR, Shields KJ, Janssen I, Budoff MJ, Everson-Rose SA, Powell LH, Matthews KA. Postmenopausal Women With Greater Paracardial Fat Have More Coronary Artery Calcification Than Premenopausal Women: The Study of Women's Health Across the Nation (SWAN) Cardiovascular Fat Ancillary Study. J Am Heart Assoc 2017;6:004545. [Crossref] [PubMed]
- Eckel RH, Grundy SM, Zimmet PZ. The metabolic syndrome. Lancet 2005;365:1415-28. [Crossref] [PubMed]
- Yki-Järvinen H. Non-alcoholic fatty liver disease as a cause and a consequence of metabolic syndrome. Lancet Diabetes Endocrinol 2014;2:901-10. [Crossref] [PubMed]
- Stahl EP, Dhindsa DS, Lee SK, Sandesara PB, Chalasani NP, Sperling LS. Nonalcoholic Fatty Liver Disease and the Heart: JACC State-of-the-Art Review. J Am Coll Cardiol 2019;73:948-63. [Crossref] [PubMed]
- Lin A, Dey D, Wong DTL, Nerlekar N. Perivascular Adipose Tissue and Coronary Atherosclerosis: from Biology to Imaging Phenotyping. Curr Atheroscler Rep 2019;21:47. [Crossref] [PubMed]
- Greco F, Quarta LG, Grasso RF, Beomonte Zobel B, Mallio CA. Increased visceral adipose tissue in clear cell renal cell carcinoma with and without peritumoral collateral vessels. Br J Radiol 2020;93:20200334. [Crossref] [PubMed]
- Huber FA, Del Grande F, Rizzo S, Guglielmi G, Guggenberger R. MRI in the assessment of adipose tissues and muscle composition: how to use it. Quant Imaging Med Surg 2020;10:1636-49. [Crossref] [PubMed]
- Greco F, Mallio CA. Relationship between visceral adipose tissue and genetic mutations (VHL and KDM5C) in clear cell renal cell carcinoma. Radiol Med 2021;126:645-51. [Crossref] [PubMed]
- Gohmann RF, Temiz B, Seitz P, Gottschling S, Lücke C, Krieghoff C, Blume C, Horn M, Gutberlet M. Segmentation and characterization of visceral and abdominal subcutaneous adipose tissue on CT with and without contrast medium: influence of 2D- and 3D-segmentation. Quant Imaging Med Surg 2021;11:4258-68. [Crossref] [PubMed]
- Greco F, Quarta LG, Carnevale A, Giganti M, Grasso RF, Beomonte Zobel B, Mallio CA. Subcutaneous Adipose Tissue Reduction in Patients with Clear Cell Renal Cell Carcinoma and Peritumoral Collateral Vessels: A Retrospective Observational Study. Appl Sci 2021;11:6076. [Crossref]
- Sun J, Lv H, Li M, Zhao L, Liu Y, Zeng N, Wei X, Chen Q, Ren P, Liu Y, Zhang P, Yang Z, Zhang Z, Wang Z. How much abdominal fat do obese patients lose short term after laparoscopic sleeve gastrectomy? A quantitative study evaluated with MRI. Quant Imaging Med Surg 2021;11:4569-82. [Crossref] [PubMed]
- Militello C, Rundo L, Toia P, Conti V, Russo G, Filorizzo C, Maffei E, Cademartiri F, La Grutta L, Midiri M, Vitabile S. A semi-automatic approach for epicardial adipose tissue segmentation and quantification on cardiac CT scans. Comput Biol Med 2019;114:103424. [Crossref] [PubMed]
- Choy G, Khalilzadeh O, Michalski M, Do S, Samir AE, Pianykh OS, Geis JR, Pandharipande PV, Brink JA, Dreyer KJ. Current Applications and Future Impact of Machine Learning in Radiology. Radiology 2018;288:318-28. [Crossref] [PubMed]
- Wang S, Summers RM. Machine learning and radiology. Med Image Anal 2012;16:933-51. [Crossref] [PubMed]
- LeCun Y, Bengio Y, Hinton G. Deep learning. Nature 2015;521:436-44. [Crossref] [PubMed]
- Quattrocchi CC, Mallio CA, Presti G, Beomonte Zobel B, Cardinale J, Iozzino M, Della Sala SW. The challenge of COVID-19 low disease prevalence for artificial intelligence models: report of 1,610 patients. Quant Imaging Med Surg 2020;10:1891-3. [Crossref] [PubMed]
- Mallio CA, Quattrocchi CC, Beomonte Zobel B, Parizel PM. Artificial intelligence, chest radiographs, and radiology trainees: a powerful combination to enhance the future of radiologists? Quant Imaging Med Surg 2021;11:2204-7. [Crossref] [PubMed]
- Mallio CA, Napolitano A, Castiello G, Giordano FM, D'Alessio P, Iozzino M, Sun Y, Angeletti S, Russano M, Santini D, Tonini G, Zobel BB, Vincenzi B, Quattrocchi CC. Deep Learning Algorithm Trained with COVID-19 Pneumonia Also Identifies Immune Checkpoint Inhibitor Therapy-Related Pneumonitis. Cancers (Basel) 2021;13:652. [Crossref] [PubMed]
- Antonopoulos AS, Sanna F, Sabharwal N, Thomas S, Oikonomou EK, Herdman L, et al. Detecting human coronary inflammation by imaging perivascular fat. Sci Transl Med 2017;9:eaal2658. [Crossref] [PubMed]
- Shirani J, Berezowski K, Roberts WC. Quantitative measurement of normal and excessive (cor adiposum) subepicardial adipose tissue, its clinical significance, and its effect on electrocardiographic QRS voltage. Am J Cardiol 1995;76:414-8. [Crossref] [PubMed]
- Sacks HS, Fain JN. Human epicardial adipose tissue: a review. Am Heart J 2007;153:907-17. [Crossref] [PubMed]
- Bambace C, Telesca M, Zoico E, Sepe A, Olioso D, Rossi A, Corzato F, Di Francesco V, Mazzucco A, Santini F, Zamboni M. Adiponectin gene expression and adipocyte diameter: a comparison between epicardial and subcutaneous adipose tissue in men. Cardiovasc Pathol 2011;20:e153-6. [Crossref] [PubMed]
- Rabkin SW. Epicardial fat: properties, function and relationship to obesity. Obes Rev 2007;8:253-61. [Crossref] [PubMed]
- Gaborit B, Sengenes C, Ancel P, Jacquier A, Dutour A. Role of Epicardial Adipose Tissue in Health and Disease: A Matter of Fat? Compr Physiol 2017;7:1051-82. [Crossref] [PubMed]
- Chechi K, Richard D. Thermogenic potential and physiological relevance of human epicardial adipose tissue. Int J Obes Suppl 2015;5:S28-34. [Crossref] [PubMed]
- Iacobellis G. Epicardial adipose tissue in endocrine and metabolic diseases. Endocrine 2014;46:8-15. [Crossref] [PubMed]
- Tadros TM, Massaro JM, Rosito GA, Hoffmann U, Vasan RS, Larson MG, Keaney JF Jr, Lipinska I, Meigs JB, Kathiresan S, O'Donnell CJ, Fox CS, Benjamin EJ. Pericardial fat volume correlates with inflammatory markers: the Framingham Heart Study. Obesity (Silver Spring) 2010;18:1039-45. [Crossref] [PubMed]
- Goecks J, Jalili V, Heiser LM, Gray JW. How Machine Learning Will Transform Biomedicine. Cell 2020;181:92-101. [Crossref] [PubMed]
- Shilo S, Rossman H, Segal E. Axes of a revolution: challenges and promises of big data in healthcare. Nat Med 2020;26:29-38. [Crossref] [PubMed]
- Renganathan V. Overview of artificial neural network models in the biomedical domain. Bratisl Lek Listy 2019;120:536-40. [Crossref] [PubMed]
- Rumelhart DE, Hinton GE, Williams RJ. Learning representations by back-propagating errors. Nature 1986;323:533-6. [Crossref]
- Bengio Y. Learning deep architectures for AI. Foundations and Trends® in Machine Learning 2009;2:1-127.
- Miotto R, Wang F, Wang S, Jiang X, Dudley JT. Deep learning for healthcare: review, opportunities and challenges. Brief Bioinform 2018;19:1236-46. [Crossref] [PubMed]
- Rodrigues ÉO, Cordeiro de Morais FF, Conci A. On the Automated Segmentation of Epicardial and Mediastinal Cardiac Adipose Tissues Using Classification Algorithms. Stud Health Technol Inform 2015;216:726-30. [PubMed]
- Rodrigues ÉO, Pinheiro VHA, Liatsis P, Conci A. Machine learning in the prediction of cardiac epicardial and mediastinal fat volumes. Comput Biol Med 2017;89:520-9. [Crossref] [PubMed]
- Priya C, Sudha S. Adaptive Fruitfly Based Modified Region Growing Algorithm for Cardiac Fat Segmentation Using Optimal Neural Network. J Med Syst 2019;43:104. [Crossref] [PubMed]
- Commandeur F, Goeller M, Betancur J, Cadet S, Doris M, Chen X, Berman DS, Slomka PJ, Tamarappoo BK, Dey D. Deep Learning for Quantification of Epicardial and Thoracic Adipose Tissue From Non-Contrast CT. IEEE Trans Med Imaging 2018;37:1835-46. [Crossref] [PubMed]
- Commandeur F, Goeller M, Razipour A, Cadet S, Hell MM, Kwiecinski J, Chen X, Chang HJ, Marwan M, Achenbach S, Berman DS, Slomka PJ, Tamarappoo BK, Dey D. Fully Automated CT Quantification of Epicardial Adipose Tissue by Deep Learning: A Multicenter Study. Radiol Artif Intell 2019;1:e190045. [Crossref] [PubMed]
- Bandekar AN, Naghavi M, Kakadiaris IA. Automated pericardial fat quantification in CT data. Conf Proc IEEE Eng Med Biol Soc 2006;2006:932-5. [Crossref] [PubMed]
- Kroll L, Nassenstein K, Jochims M, Koitka S, Nensa F. Assessing the Role of Pericardial Fat as a Biomarker Connected to Coronary Calcification-A Deep Learning Based Approach Using Fully Automated Body Composition Analysis. J Clin Med 2021;10:356. [Crossref] [PubMed]
- Eisenberg E, McElhinney PA, Commandeur F, Chen X, Cadet S, Goeller M, Razipour A, Gransar H, Cantu S, Miller RJH, Slomka PJ, Wong ND, Rozanski A, Achenbach S, Tamarappoo BK, Berman DS, Dey D. Deep Learning-Based Quantification of Epicardial Adipose Tissue Volume and Attenuation Predicts Major Adverse Cardiovascular Events in Asymptomatic Subjects. Circ Cardiovasc Imaging 2020;13:e009829. [Crossref] [PubMed]
- Oikonomou EK, Williams MC, Kotanidis CP, Desai MY, Marwan M, Antonopoulos AS, et al. A novel machine learning-derived radiotranscriptomic signature of perivascular fat improves cardiac risk prediction using coronary CT angiography. Eur Heart J 2019;40:3529-43. [Crossref] [PubMed]
- Lin A, Wong ND, Razipour A, McElhinney PA, Commandeur F, Cadet SJ, Gransar H, Chen X, Cantu S, Miller RJH, Nerlekar N, Wong DTL, Slomka PJ, Rozanski A, Tamarappoo BK, Berman DS, Dey D. Metabolic syndrome, fatty liver, and artificial intelligence-based epicardial adipose tissue measures predict long-term risk of cardiac events: a prospective study. Cardiovasc Diabetol 2021;20:27. [Crossref] [PubMed]
- Tamarappoo BK, Lin A, Commandeur F, McElhinney PA, Cadet S, Goeller M, Razipour A, Chen X, Gransar H, Cantu S, Miller RJ, Achenbach S, Friedman J, Hayes S, Thomson L, Wong ND, Rozanski A, Slomka PJ, Berman DS, Dey D. Machine learning integration of circulating and imaging biomarkers for explainable patient-specific prediction of cardiac events: A prospective study. Atherosclerosis 2021;318:76-82. [Crossref] [PubMed]
- Weikert T, Francone M, Abbara S, Baessler B, Choi BW, Gutberlet M, Hecht EM, Loewe C, Mousseaux E, Natale L, Nikolaou K, Ordovas KG, Peebles C, Prieto C, Salgado R, Velthuis B, Vliegenthart R, Bremerich J, Leiner T. Machine learning in cardiovascular radiology: ESCR position statement on design requirements, quality assessment, current applications, opportunities, and challenges. Eur Radiol 2021;31:3909-22. [Crossref] [PubMed]
- Koitka S, Kroll L, Malamutmann E, Oezcelik A, Nensa F. Fully automated body composition analysis in routine CT imaging using 3D semantic segmentation convolutional neural networks. Eur Radiol 2021;31:1795-804. [Crossref] [PubMed]
- Greco F, Mallio CA. Artificial intelligence and abdominal adipose tissue analysis: a literature review. Quant Imaging Med Surg 2021;11:4461-74. [Crossref] [PubMed]
- Pickhardt PJ, Graffy PM, Zea R, Lee SJ, Liu J, Sandfort V, Summers RM. Automated CT biomarkers for opportunistic prediction of future cardiovascular events and mortality in an asymptomatic screening population: a retrospective cohort study. Lancet Digit Health 2020;2:e192-200. [Crossref] [PubMed]
- de Marvao A, Dawes TJW, O'Regan DP. Artificial Intelligence for Cardiac Imaging-Genetics Research. Front Cardiovasc Med 2019;6:195. [Crossref] [PubMed]