High resolution computed tomography texture analysis identifies patients at risk of pulmonary fibrosis after COVID-19 pneumonia
Introduction
High resolution computed tomography (HRCT) is an excellent tool for diagnosing COVID-19 pneumonia and following up patients who develop long-term respiratory complications, including pulmonary fibrosis (1,2). While previous studies showed that texture analysis (TA) on lung computed tomography (CT) images could effectively predict clinical outcomes in patients with COVID-19 pneumonia in the acute setting (3,4), its role in identifying patients at risk of developing pulmonary fibrosis has never been investigated. The aim of this study was to assess the accuracy of HRCT TA in predicting the onset of pulmonary fibrosis in patients after COVID-19 pneumonia.
Materials and methods
This study was conducted in accordance with the Declaration of Helsinki (as revised in 2013). The study was approved by Ethics Committee of Padova University Hospital (protocol number: 0042076 of the 29th of June 2021) and individual consent for this retrospective analysis was waived.
Our retrospective study included all consecutive patients with COVID-19 pneumonia who accessed the emergency department of our third-level hospital between February and May 2020. Inclusion criteria were: COVID-19 pneumonia confirmed by real-time polymerase chain reaction (RT-PCR) and HRCT; no concomitant pulmonary disease; at least one follow-up HRCT performed no less than one month after diagnosis. All scans were performed with a 64-slice CT-scanner (Somatom Sensation, Siemens Healthineers, Erlangen, Germany) with a 1-mm slice thickness in breath-hold.
Two radiologists specialized in chest imaging analyzed follow-up HRCTs to identify signs of pulmonary fibrosis (2), blinded to the clinical and imaging data of the patients. In cases with multiple follow-up HRCTs, the latest scan was considered. Each lung was classified separately according to the presence/absence of fibrotic changes, including fibrotic strips, traction bronchiectasis, architectural distortion, and inter-lobular septal thickening. HRCTs performed when COVID-19 pneumonia was diagnosed were analyzed on a dedicated workstation with LIFEx software (5) after image resampling to 3×1×1 mm voxels. The two radiologists agreed on a manually drawn region of interest in each axial slice along the boundaries of the lungs from apex to base, obtaining a volume of interest for each lung (Figure 1A,1B), blinded to the clinical and follow-up HRCT data of the patients. The main bronchi and hilar vessels were excluded from the segmented volume. Fifty-eight textural parameters were extracted for each lung.
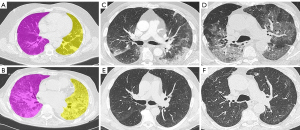
Student’s independent unpaired t-test was used to compare normally distributed continuous variables, and the Mann-Whitney test was used for nonparametric data. Textural parameters were compared in terms of the absence (Group 0) or presence (Group 1) of lung fibrosis. A logistic regression analysis was performed in order to assess the prediction capability of the features. Features with highest importance were then used to create a prediction model. A multivariate receiver operating characteristic (ROC) curve was drawn from this model. Significance was set at P<0.05.
Results
Twenty-eight lungs from 14 patients (12 M; median age:64 years, range, 38–85 years) were analyzed. The median time elapsing from the diagnosis of COVID-19 pneumonia to the follow-up HRCT scan was 207 days (range, 87–491 days). Fibrotic changes were present in 12 lungs. Five patients showed bilateral fibrotic changes and two showed unilateral lung involvement. Four textural parameters—maximum conventional Hounsfield unit (HU) {1,478.984 [interquartile range (IQR), 1,294.819–1,716.333] vs. [1,781.102 (IQR, 1,483.384–2,106.742)]; P=0.041}, maximum discretized HU [265.500 (IQR, 249.000–281.500) vs. 292.000 (IQR, 265.500–321.000); P=0.036], gray level co-occurrence matrix (GLCM) energy angular second moment [4.382 (IQR, 2.766–4.890) vs. 0.752 (IQR, 0.001–2.294); P=0.010], and GLCM correlation [0.623 (IQR, 0.563–0.709) vs. 0.509 (IQR, 0.421–0.598); P=0.022]—differed significantly between the fibrotic and non-fibrotic groups (Table S1). From the logistic regression analysis on the 58 features we considered different models, obtained adding one feature at the time on the base of the P value of the individual logistic regression model. We noticed that using the first 4 of them [GLCM energy angular second moment, GLCM correlation, neighbourhood grey-level difference matrix (NGLDM) contrast, and grey-level zone length matrix (GLZLM), gray-level non-uniformity (GLNU)] we reached a pretty high accuracy of the multivariate ROC curve.
The multivariate ROC showed an area under the curve (AUC) of 0.938 (95% CI: 0.855–1.000; P<0.001) with 92% sensitivity and 81% specificity. This accuracy increases adding more features, reaching an AUC of 1.000 with 7 features (GLCM energy angular second moment, GLCM correlation, NGLDM contrast, GLZLM GLNU, conventional HU excess kurtosis, discretized HU max, and discretized HU kurtosis).
Discussion
Our study demonstrates that performing HRCT TA when COVID-19 pneumonia is diagnosed can be effective in identifying patients at risk of developing lung fibrotic changes.
The four parameters identified by the logistic regression analysis that together showed an AUC of the multivariate ROC of 0.938 were respectively GLCM energy angular second moment, that represents the uniformity of distribution of grey level in the image, GLCM correlation that reflects the joint probability occurrence of the specified pixel pairs, NGLDM contrast that shows the spatial rate change of intensity, and GLZLM GLNU that measures the non-uniformity of the grey-levels in the volume of interest (5).
Noteworthy, adding other three features to the model the AUC rose to 1.000. These parameters were respectively conventional HU excess kurtosis and discretized HU kurtosis, that reflect the shape of the grey-level distribution relative to a normal distribution and discretized HU max that represents the maximum intensity value in the volume of interest (5).
A hypothesis that can be postulated about the mechanisms involved in lung fibrosis is that the more parenchymal consolidations are wide and inhomogeneous at baseline HRCT, the more lung parenchyma is prone to develop fibrosis at follow-up. Indeed, patients who developed fibrosis had higher maximum conventional and discretized HU values and lower GLCM energy angular second moment and correlation values compared to patients who completely recovered at the follow-up HRCT (Figure 1C-1F). It is well known that consolidations occurring when the alveolar spaces are filled by erythrocytes, leukocytes and fibrin, increase the intrinsic radiological density of the lung parenchyma (6,7) and it has recently been suggested that the intra-alveolar fibrosis and collapse of alveolar capillary units represent the initial “Noxa” that later lads to fibrosis (8). This collapse of the alveolar capillary units results in higher densitometric values compared to normal parenchyma, hence, we can speculate that the higher maximum density values of consolidations in patients who develop fibrosis could be related to this physio-pathological mechanism (7). Moreover, consolidation and inhomogeneous density pattern like “crazy paving” characterize the later stage of COVID-19 pneumonia, when lung parenchyma is more prone to develop fibrosis (7).
Despite its relevant limitations (e.g., sample size), our study may prompt larger follow-up studies to investigate the role of TA in the early detection of COVID-19 patients at risk of developing pulmonary fibrosis.
Acknowledgments
Funding: None.
Footnote
Conflicts of Interest: All authors have completed the ICMJE uniform disclosure form (available at https://dx.doi.org/10.21037/qims-21-769). The authors have no conflicts of interest to declare.
Ethical Statement: The authors are accountable for all aspects of the work in ensuring that questions related to the accuracy or integrity of any part of the work are appropriately investigated and resolved. This study was conducted in accordance with the Declaration of Helsinki (as revised in 2013). The study was approved by Ethics Committee of Padova University Hospital (protocol number: 0042076 of the 29th of June 2021) and individual consent for this retrospective analysis was waived.
Open Access Statement: This is an Open Access article distributed in accordance with the Creative Commons Attribution-NonCommercial-NoDerivs 4.0 International License (CC BY-NC-ND 4.0), which permits the non-commercial replication and distribution of the article with the strict proviso that no changes or edits are made and the original work is properly cited (including links to both the formal publication through the relevant DOI and the license). See: https://creativecommons.org/licenses/by-nc-nd/4.0/.
References
- Rubin GD, Ryerson CJ, Haramati LB, Sverzellati N, Kanne JP, Raoof S, et al. The Role of Chest Imaging in Patient Management During the COVID-19 Pandemic: A Multinational Consensus Statement From the Fleischner Society. Chest 2020;158:106-16. [Crossref] [PubMed]
- Han X, Fan Y, Alwalid O, Li N, Jia X, Yuan M, Li Y, Cao Y, Gu J, Wu H, Shi H. Six-month Follow-up Chest CT Findings after Severe COVID-19 Pneumonia. Radiology 2021;299:E177-86. [Crossref] [PubMed]
- Wu Z, Li L, Jin R, Liang L, Hu Z, Tao L, Han Y, Feng W, Zhou D, Li W, Lu Q, Liu W, Fang L, Huang J, Gu Y, Li H, Guo X. Texture feature-based machine learning classifier could assist in the diagnosis of COVID-19. Eur J Radiol 2021;137:109602. [Crossref] [PubMed]
- Wu Q, Wang S, Li L, Wu Q, Qian W, Hu Y, Li L, Zhou X, Ma H, Li H, Wang M, Qiu X, Zha Y, Tian J. Radiomics Analysis of Computed Tomography helps predict poor prognostic outcome in COVID-19. Theranostics 2020;10:7231-44. [Crossref] [PubMed]
- Nioche C, Orlhac F, Boughdad S, Reuzé S, Goya-Outi J, Robert C, Pellot-Barakat C, Soussan M, Frouin F, Buvat I. LIFEx: A Freeware for Radiomic Feature Calculation in Multimodality Imaging to Accelerate Advances in the Characterization of Tumor Heterogeneity. Cancer Res 2018;78:4786-9. [Crossref] [PubMed]
- Lei Q, Li G, Ma X, Tian J, Wu YF, Chen H, Xu W, Li C, Jiang G. Correlation between CT findings and outcomes in 46 patients with coronavirus disease 2019. Sci Rep 2021;11:1103. [Crossref] [PubMed]
- Edwards RM, Godwin JD, Hippe DS, Kicska G. A Quantitative Approach to Distinguish Pneumonia From Atelectasis Using Computed Tomography Attenuation. J Comput Assist Tomogr 2016;40:746-51. [Crossref] [PubMed]
- Ward PA, Hunninghake GW. Lung inflammation and fibrosis. Am J Respir Crit Care Med 1998;157:S123-9. [Crossref] [PubMed]