An automated quantification method for the Agatston coronary artery calcium score on coronary computed tomography angiography
Introduction
Cardiovascular diseases (CVDs) are a large contributor to the global mortality rate. A total of 17.9 million people die from CVDs every year (1). The coronary artery calcium (CAC) score serves as a reliable diagnostic tool for CVD and is recommended by several guidelines (2-4) for risk assessment. A high CAC score reflects an increased relative risk for cardiovascular events (5). CAC is a representative marker of the overall coronary sclerosis burden (6). The amount of CAC is commonly detected on a non-contrast cardiac computed tomography (CT) scan and quantified according to the Agatston scoring method (7,8). The Agatston CAC score has been shown to have prognostic value for cardiovascular events, regardless of age, race, or sex (9-13). However, to assess the severity and degree of coronary artery stenosis, coronary CT angiography (CCTA) must be performed (14). Compared with invasive coronary angiography, CCTA can more accurately assess coronary artery stenosis (15-17). Moreover, it can provide accurate visualization of the coronary vessel wall and facilitate the analysis of coronary plaque constitution.
Since CCTA can distinguish calcified plaques, it is plausible that it can be used to identify CAC and calculate the Agatston CAC score. Because non-contrast CT scans and CCTA are performed separately and both contribute to radiation exposure (18), it would be beneficial to determine whether the Agatston CAC score can be quantified from CCTA scans alone. Previous studies, in which specific Hounsfield unit (HU) thresholds (19-22) and deep learning algorithms (23-25) were applied, have evaluated the potential to quantify CAC from CCTA datasets. Both the HU threshold-based method and deep learning algorithms directly detected potential calcified plaques. However, false-positive areas were sometimes detected outside the coronary artery, such as calcification on the aorta, which led to inaccurate calculation of Agatston CAC scores and CVD risk categorization. Given these inaccuracies, a precise CAC score quantification method warrants investigation.
The present study had three main aims: (I) to propose a method for full automatic detection and quantification of CAC on CCTA scans and quantification of Agatston CAC score; (II) to compare the derived CCTA-CAC score with the standard Agatston CAC score and evaluate the correlation between them; and (III) to define the optimal threshold for CCTA-CAC calculation.
We present the following article in accordance with the STARD reporting checklist (available at https://dx.doi.org/10.21037/qims-21-775).
Methods
Study design
This retrospective study was conducted in accordance with the Declaration of Helsinki (as revised in 2013). There were two patient datasets, one for coronary artery segmentation and one for CAC calculation.
Patients for coronary artery segmentation
A coronary artery segmentation algorithm was trained and tested on 134 CCTA scans collected between 1 August, 2014 and 30 March, 2019 at three Chinese medical centers: the Beijing Anzhen Hospital, Capital Medical University (n=90); the Sir Run Run Shaw Hospital, Zhejiang University School of Medicine (n=28); and the General Hospital of Eastern Theater Command (n=16). Ground truth was achieved through manual segmentation by a clinical expert. The study population comprised 80 males and 54 females, whose ages ranged from 41 to 80 years (mean age: 60.6±8.8 years). The requirement for informed patient consent was waived by the ethics committees of the three medical centers. This multicenter dataset was independent from the dataset used for calculation of the Agatston CAC score.
In this study, a deep-learning approach was used for the segmentation of coronary arteries. Training (n=94) and testing (n=40) datasets were used for the pretrained segmentation model. There were no standard Agatston CAC scores included in this dataset, so we did not use this dataset for quantification of the CCTA-CAC score.
Patients for CAC quantification
The Independent Ethics Committee of the Anzhen Hospital approved this retrospective study. Seventy-five patients from Anzhen Hospital who had CVD symptoms, such as chest pain, underwent non-contrast cardiac CT followed by CCTA between 26 December, 2017 and 25 January, 2019. Patients with a history of percutaneous coronary intervention (n=4), obvious artifacts (n=5), or who underwent coronary artery bypass graft (CABG) surgery (n=2), were excluded, as were those with poor quality scans from which the coronary artery could not be segmented (n=2). The resulting cohort consisted of 22 women (mean age: 64.0±7.52 years) and 40 men (mean age: 60.0±8.1 years).
Imaging for coronary artery segmentation
The CCTA scans for coronary artery segmentation were performed on four different CT scanners: a 256-detector row CT scanner (Revolution CT, GE Healthcare, Milwaukee, WI, USA), a 320-detector row CT scanner (Aquilion One; Toshiba, Otawara, Japan), and two dual-source CT scanners with ≥ 64-detector rows (Somatom Definition Flash/Force; Siemens, Forchheim, Germany), which all met the requirements set out in the Society of Cardiovascular Computed Tomography (SCCT) guidelines (26). For all CCTA scans, sublingual nitroglycerin (0.5 mg per dose; nitroglycerin spray, Jingwei Pharmacy, Jinan, China) was administered to all patients 5 min before scanning. Beta-blockers were not administered to any of the patients. Similar to a previous study (27), the CCTA data were acquired after the injection of 50–60 mL of contrast agent (350 mg iodine/mL, Omnipaque, GE Healthcare; or 370 mg iodine/mL, Ultravist, Bayer Schering Pharma, Berlin, Germany) at a rate of 4.5–5 mL/s followed by a saline bolus chaser IV injection of 30–35 mL at a rate of 5 mL/s. The tube voltage of the CCTA scan was 80–110 kV, depending on the CT scanner. Furthermore, three modes were utilized for data acquisition. Prospective electrocardiogram (ECG)—triggered axial-mode single heartbeat acquisition was used on the scanners with a wide Z-axis coverage, and prospective ECG-gated high-pitch single heartbeat helical acquisition or multiple heartbeat axial-mode acquisition was performed on the dual-source CT platforms. A bolus tracking technique was used for scan triggering CCTA acquisition. The gantry rotation time was in the range of 0.28–0.35 s per rotation, depending on the CT scanner. Axial images were reconstructed with a slice thickness of 0.5, 0.6, or 0.625 mm.
Imaging for CAC quantification
The CCTA scans for CAC calculation were all performed on a 256-detector row CT scanner (Revolution CT, GE Healthcare). The CCTA data were acquired after the injection of 50–60 mL of contrast agent (350 mg iodine/mL, Omnipaque, GE Healthcare) at a rate of 4.5–5 mL/s, followed by a saline bolus chaser IV injection of 30–35 mL at a rate of 5 mL/s. Prospective ECG-gated CCTA was used as the CCTA examination protocol. The scanning range was set from the tracheal bifurcation to the diaphragm. The scan parameters for CCTA were as follows: 256×0.625 mm collimation, 0.28s rotation time, 512×512 pixel matrix. The tube voltage was 100 kV, and the Smart mA (GE’s proprietary name for the more general automatic exposure control term) was applied. The slice thickness was 0.625mm. In the study group, images were reconstructed using the state-of-the-art adaptive statistical iterative reconstruction (ASiR)-V algorithm with a weight of 50% (50% ASiR-V).
Non-contrast cardiac CT was performed before the CCTA examination. Prospective ECG-triggered CT acquisition was used for the non-contrast CT. Scan parameters were obtained as follows: the tube voltage was 100 kV, and the Smart mA was applied. The pixel matrix size was 512×512. The slice thickness for the Agatston CAC score quantification was 2.5 mm. Regarding the CAC quantification method, we first obtained the coronary artery segmentation on CCTA images with a slice thickness of 0.625 mm. We then reconstructed both the original image and the segmentation label to 0.5×0.5×2.5 mm for further comparison with the non-contrast CT images.
Quantification of the standard Agatston CAC score on non-contrast CT scans
The collected scans were transferred from the CT equipment to a workstation (AW 15.0, General Electric, Boston, MA, USA) to determine the CAC score using the dedicated postprocessing software “Smartscore”. The standard Agatston CAC score was calculated using the Agatston method (7). A threshold of 130 HU was applied for the non-contrast CT scans. Calcification was defined as more than two adjacent pixels with values of >130 HU. An Agatston CAC score was calculated for the right coronary artery (RCA), left main and left circumflex arteries (LMCx), and left anterior descending (LAD) artery, and this score was used as the reference standard. Assessment of the standard Agatston CAC score was carried out automatically, without consideration of any clinical information.
Quantification of CCTA-CAC score on CCTA scans
An end-to-end detection and quantification CCTA-CAC score method was developed. Models were created using Nvidia Corporation’s deep learning GPU training system. Training and testing were both done on a 2 Intel(R) Xeon Sliver 4110 2.1 GHz, 16 GB processor equipped with an Nvidia Tesla V100 graphic card on a Windows Server 2016 Standard 64 bits operating system. The flow diagram for this process is shown in Figure 1. The quantification method was created as described below.
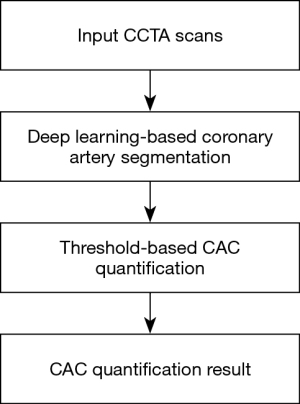
A pretrained dense V-net fully convolutional neural network (28) created from the 134 multicenter CCTA scans was used for coronary artery segmentation. Subsequently, the main coronary branches (RCA, LMCx, and LAD) were identified. The diversity of the data ensured good generalization of the model, and cases were categorized into two groups: the training set (n=94) and the test set (n=40). This quantification method was validated using data from all the patients in the CAC dataset (n=62).
The proposed architecture (Figure 2) used a fully convolutional neural network based on convolutional units. The architectural design can be understood in terms of the following five key features: batch-wise spatial dropout, dense feature stacks (DFSs), V-network down-sampling and up-sampling, dilated convolutions, and an explicit spatial prior. We computed 1003 feature maps using a strided convolution. Then, a cascade of DFSs and strided convolutions generated activation maps at three resolutions. A convolution unit was applied at each resolution, reducing the number of features. After bilinear up-sampling back to 1003, the maps were concatenated, and a final convolution generated the likelihood logits, which were subsequently added to the up-sampled spatial before the segmentation logit was generated. The input was a volume of interest (VOI) (200×200×200). The segmentation result was given in the output (200×200×200) of the dense V-net. Table 1 lists the detailed parameters for the dense V-net. Individual components, such as input, output, kernel, stride, and subunits of each layer, are given.
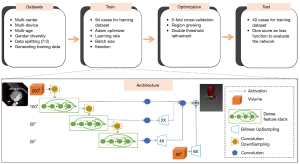
Table 1
Layer | Input | Output | Kernel | Stride | Subunits m*n |
---|---|---|---|---|---|
Feature | 2003×1 | 1003×24 | 53 | 2 | |
DFS 1 | 1003×24 | 1003×20 | 33 | 1 | 5×4 |
Skip 1 | 1003×20 | 1003×12 | 33 | 1 | |
Down 1–2 | 1003×20 | 503×24 | 33 | 2 | |
DFS 2 | 503×24 | 503×80 | 33 | 1 | 10×8 |
Skip 2 | 503×80 | 503×24 | 33 | 1 | |
Up 2 | 503×24 | 1003×24 | |||
Down 2–3 | 503×80 | 253×24 | 33 | 2 | |
DFS 3 | 253×24 | 253×160 | 33 | 1 | 10×16 |
Skip 3 | 253×24 | 253×24 | 33 | 1 | |
Up 3 | 253×24 | 723×24 | |||
Up prior | 203×1 | 1003×1 |
DFS, dense feature stack.
In the preprocessing stage, image quality control was conducted, and improvements such as median filtering and Laplacian enhancement were applied to reduce unwanted noise. During the training stage, data augmentation was realized by flipping and rotating the VOI of the vessel region. The network was trained using the Adam optimizer algorithm, with a learning rate of 0.001 and mini-batch size 6 for 10,000 iterations. Because of the limited number of available annotated datasets, a 5-fold cross-validation approach was employed. The trained model which had the best performance (i.e., with the highest Dice score) for the test dataset was kept. Training each instance of the network took approximately 6 h. In the postprocessing stage, region growing (29) and two-threshold refinement (30) were used for further optimization of the segmentation results. The network loss function was based on the concept of the similarity between the output image and the ground-truth image. Thus, the Dice similarity coefficient was chosen as a loss function for the network. The Dice values for the training (n=94) and testing (n=40) images were 0.92 and 0.90, respectively.
The CCTA-CAC score was automatically detected and quantified using different thresholds. The total CCTA-CAC score was calculated using the following formula, defined as Eq. [1]:
There were some similarities between our quantification method and the standard Agatston CAC score quantification (7). The selected thresholds for calcified plaque were 450, 500, 550, 600, 650, 700, 750, and 800. For each slice, all pixels with a CT value ≥ threshold were displayed. A region of interest marker was placed around all lesions detected in the coronary artery. The weight coefficient α in Eq. [1] is related to the threshold value.
The following formula was defined as Eq. [2]:
For Eq. [2], the thresholds ranged from 450 to 800. The higher the CT value, the larger the weight coefficient α. For instance, if 650 HU was chosen as the threshold, α was determined based on the CT value in the following manner: 1=650 to 749, 2=750 to 849, 3=850 to 949, and 4≥50 HU. A score for each region of interest was calculated by multiplying the CT value score by the area. The total CCTA-CAC score was calculated using each of these scores for all slices. An example of the automatic CAC assessment on CCTA is given in Figure 3. Calcification was automatically identified according to the thresholds after coronary artery segmentation without consideration of clinical information or the reference standard.
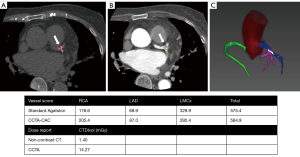
Statistical analysis
Categorical variables were presented as absolute numbers and percentages. Continuous variables were expressed as means ± standard deviation (SD) or median [interquartile range (IQR)]. A comparison was made between the standard Agatston CAC score and the CCTA-CAC score. The non-parametric Spearman’s correlation and intra-class correlation (ICC) were used to evaluate the correlation and consistency between the two methods. An ICC of less than 0.4 indicated poor correlation; an ICC of 0.4 to 0.75 indicated fair to good correlation; and an ICC of more than 0.75 indicated excellent correlation (31). Thereafter, Bland-Altman analysis was applied to assess the limits of agreement between the methods. A two-tailed P value <0.05 indicated statistical significance. Also, the scores were divided into four risk categories (0, 0–99, 100–399, and ≥400), and the agreement between the two methods was calculated. Statistical analyses were performed using Python (version 3.5.6).
Results
Patient population
In this study, the CAC cohort comprised 62 patients with a mean age of 61.4±8.1 years and a body mass index (BMI) of 25.6±3.1 kg/m2. There were 40 male patients and 22 female patients. Non-contrast cardiac CT was performed at the time of the CCTA examination without any clinical interventions. Based on the standard Agatston CAC score, 12 patients were in calcification category 0, 22 patients were in calcification category 1 to 99, 22 patients were in calcification category 100 to 399, and 6 patients were in calcification category >400. The baseline characteristics of the testing patients are shown in Table 2.
Table 2
Characteristic | Value |
---|---|
Clinical data (n=62) | |
Age, mean ± SD (years) | 61.4±8.1 |
Male | 40 (65%) |
Female | 22 (35%) |
BMI, mean ± SD (kg/m2) | 25.6±3.1 |
Obesity (BMI ≥30 kg/m2) | 6 (9%) |
Family history of CAD | 17 (27%) |
Angina pectoris | 9 (15%) |
Myocardial infarction | 4 (6%) |
Current smoking | 30 (48%) |
Current drinking | 17 (27%) |
High pressure | 36 (58%) |
Hyperlipidemia | 33 (53%) |
Data for coronary artery segmentation (n=134) | |
Age, mean ± SD (years) | 60.6±8.8 |
BMI, mean ± SD (kg/m2) | 25.2±3.0 |
Male number | 80 (60%) |
Female number | 54 (40%) |
BMI, body mass index; CAD, coronary artery disease; SD, standard deviation.
Coronary artery segmentation and CCTA-CAC quantification results
The proposed pretrained deep learning-based coronary artery segmentation network was used to segment the LAD, LMCx, and RCA in our dataset. The CCTA-CAC score was automatically quantified according to different thresholds. The total analysis time was 11 s per case on an Nvidia Tesla V100 graphic card.
Correlation between the standard Agatston CAC and CCTA-CAC scores
A normality evaluation of the datasets was performed first, and the results are shown in Table 3. The median CCTA-CAC score from CCTA scans [45.8 (IQR, 55.0–67.0)] was lower than the standard Agatston CAC score from non-contrast CT scans [69 (IQR, 60.5–67.5)]. Spearman’s correlation and ICC were used to calculate the correlation between the standard Agatston CAC and CCTA-CAC scores. The results are displayed on a per-patient basis in Table 4 and on a per-vessel basis in Table 5. Considering the Spearman’s correlation and ICC values, 650 HU was chosen as the optimal lower threshold for calculating the CCTA-CAC score. On a per-patient basis, the Spearman’s correlation (R =0.959; P<0.001) and ICC (ICC =0.822; P<0.001) values indicated an almost perfect correlation. The Spearman’s correlation (R =0.889; P<0.001) and ICC (ICC =0.717; P<0.001) values also showed good correlation on a per-vessel basis.
Table 3
CCTA threshold (HU) | Per-patient normality ρ | Per-vessel normality ρ |
---|---|---|
450 | 6.956e-11 | 2.540e-36 |
500 | 4.715e-13 | 2.383e-35 |
550 | 1.429e-16 | 2.243e-33 |
600 | 1.455e-18 | 2.989e-39 |
650 | 2.865e-22 | 6.345e-45 |
700 | 1.149e-22 | 1.154e-45 |
750 | 3.580e-23 | 2.119e-46 |
800 | 1.794e-23 | 4.381e-47 |
Standard-Agatston | 1.879e-23 | 3.379e-60 |
CAC, coronary artery calcium; CCTA-CAC score, CCTA Agatston CAC score; CCTA, coronary computed tomography angiography.
Table 4
CCTA threshold (HU) | Spearman’s ρ | ICC |
---|---|---|
450 | 0.644 (P<0.01) | 0.316 (P<0.01) |
500 | 0.792 (P<0.01) | 0.589 (P<0.01) |
550 | 0.857 (P<0.01) | 0.850 (P<0.01) |
600 | 0.897 (P<0.01) | 0.862 (P<0.01) |
650 | 0.959 (P<0.01) | 0.822 (P<0.01) |
700 | 0.961 (P<0.01) | 0.574 (P<0.01) |
750 | 0.958 (P<0.01) | 0.468 (P<0.01) |
800 | 0.954 (P<0.01) | 0.245 (P=0.03) |
ICC, intra-class correlation; CCTA-CAC score, CCTA Agatston CAC score; CCTA, coronary computed tomography angiography; CAC, coronary artery calcium.
Table 5
CCTA threshold (HU) | Spearman’s ρ | ICC |
---|---|---|
450 | 0.606 (P<0.01) | 0.240 (P<0.01) |
500 | 0.750 (P<0.01) | 0.489 (P<0.01) |
550 | 0.815 (P<0.01) | 0.750 (P<0.01) |
600 | 0.845 (P<0.01) | 0.767 (P<0.01) |
650 | 0.889 (P<0.01) | 0.717 (P<0.01) |
700 | 0.883 (P<0.01) | 0.489 (P<0.01) |
750 | 0.883 (P<0.01) | 0.396 (P<0.01) |
800 | 0.876 (P<0.01) | 0.207 (P=0.03) |
ICC, intra-class correlation; CCTA-CAC score, CCTA Agatston CAC score; CCTA, coronary computed tomography angiography; CAC, coronary artery calcium.
Figure 4 displays the regression lines for the correlation of the various scores based on their averages, including the slope and 95% prediction interval. Figure 4A presents the full-range scatter plot. Figure 4B presents a magnified view of standard Agatston CAC scores up to 1,000. Bland-Altman analysis of the standard Agatston CAC score as assessed by CCTA-CAC score (650 HU threshold) is shown in Figure 5.
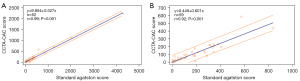
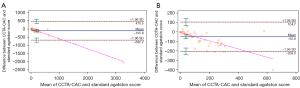
Agreement between score risk categories
The agreement between the two methods within the standard Agatston CAC score risk categories is shown in Table 6. The underlined numbers indicate points of agreement between standard Agatston CAC scores and CCTA-CAC scores (650 HU threshold). The proposed CCTA-CAC score quantification method classified 47 of 62 patients (76%) into the same cardiovascular risk category as did the standard Agatston CAC score, and 15 patients shifted to a lower category. Four (6.5%) CAC scores of 0.2, 1, 2.5, and 6.4 were miscalculated to zero. Importantly, all 12 patients in category 0 stayed in the same category after being given a CAC score from their CCTA scans. Overall, the agreement between the standard Agatston CAC score risk categories was good (kappa =0.756).
Table 6
Category | Standard-Agatston score | Total | |||
---|---|---|---|---|---|
0 | 1–99 | 100–399 | ≥400 | ||
CCTA-CAC score (650 HU) | |||||
0 | 12* | 4 | 0 | 0 | 16 |
0–99 | 0 | 18* | 10 | 0 | 28 |
100–399 | 0 | 0 | 12* | 1 | 13 |
≥400 | 0 | 0 | 0 | 5* | 5 |
Total | 12 | 22 | 22 | 6 | 62 |
Same | 12* | 18* | 12* | 5* | 47* |
Shift up | 0 | 0 | 0 | 0 | 0 |
Shift down | 0 | 4 | 10 | 1 | 15 |
*, the numbers indicate agreement between both methods. CAC, coronary artery calcium; CCTA-CAC score, CCTA Agatston CAC score; CCTA, coronary computed tomography angiography.
Discussion
In this study, an automated method for the quantification of a CAC score from CCTA was presented and compared with the standard Agatston CAC score from non-contrast CT. The overall processing time of our method was approximately 11 s per case on an Nvidia Tesla V100 GPU. This study shows that a CAC score can be obtained from CCTA scans, and that it is strongly correlated with the Agatston CAC score from non-contrast CT, both on a per-patient basis (R =0.959, P<0.01; ICC =0.822, P<0.01) and a per-vessel basis (R =0.889, P<0.01; ICC =0.717, P<0.01). Of the patients, 76% were classified in the same CAC risk category when the CCTA-CAC score was used.
The main challenge in quantifying Agatston CAC scores is designing an accurate method to differentiate between calcified plaque and coronary artery luminal contrast from CCTA scans. Despite the need for manual segmentation of CAC from CCTA scans, there are methods for the automated quantification of CAC scores using fixed or patient-specific HU thresholds. Due to the effects of contrast agents, it is natural to increase the HU threshold. Previous studies have indicated that the HU threshold for calcification quantification is dependent on CT scan protocols and the luminal contrast intensity (21,32). Glodny et al. (19) used 600 HU as the calcification detection threshold and observed an excellent correlation.
As threshold definitions have proved inadequate for some patients, patient-specific HU threshold strategies have been proposed. Mylonas et al. (20) set aortic attenuation +2 SD as the lower threshold for calcification detection and observed an excellent correlation, with 83% of their patients being classified into the same CAC risk category. Bischoff et al. (22) used 150% of the mean attenuation (HU) in the ascending aorta as the calcification detection threshold, and 90% of their patients were classified into the same CAC risk category. However, although these methods achieved excellent correlation with the standard Agatston CAC score from non-contrast CT scans, they both required semi-automated segmentation for coronary arteries.
In this study, we used a pretrained dense V-Net fully convolutional neural network for coronary artery segmentation. The accurate visualization of the coronary artery vessel wall provided a good premise for our final CAC quantification. Previous deep learning methods, such as pairs of ConvNets (Convolutional neural networks) (33), have directly identified calcified plaques without requiring coronary artery extraction. However, annotation of calcified lesions from CCTA images required a certain degree of clinical experience. Another problem was false positives caused by, for example, calcified lymph nodes or calcified lesions in the aorta, which had a similar intensity and shape as calcification plaques in the coronary arteries. False positives were less likely using our method, since we performed coronary artery segmentation first and extracted calcified plaques from the segmentation result.
The prognostic significance of the CAC score has been extensively investigated (34). One of the most important purposes of the CAC score is to determine the overall coronary atherosclerosis burden. It is also useful for the risk classification of patients and clinical decision-making. In our study, good agreement was observed between the standard Agatston CAC and CCTA-CAC risk categories. Besides the clinical significance of CAC scores, the prognostic value of CAC progression has also been established (35).
Like previous methods for CAC quantification from CCTA (22-24), our method might have the potential to reduce radiation exposure resulting from separate non-contrast and CCTA images. The radiation doses from CAC scoring with a multi-detector row CT scanner were 1.5–5.2 mSv for male patients and 1.8–6.2 mSv for female patients (36). We used 100 kV, Smart mA technology, and ASiR-V reconstruction (37) simultaneously to achieve the goal of significantly reducing the radiation dose without affecting the quantification of the CAC score, as demonstrated as demonstrated in Vonder et al. (38). One previous study (39) evaluated the effects of different iterative reconstruction algorithms on the CAC score using a reduced radiation dose protocol and proved the clinical feasibility of such a protocol. One of the main drawbacks of using CT equipment is the high exposure to radiation, which has been linked to the risk of tumors (40). Therefore, it should not be overlooked that omitting the need for non-contrast CT would also reduce the total radiation dosage. We hoped that while obtaining the anatomical structure to analyze stenosis from the CCTA scans, we might also analyze the calcified plaque, which greatly affects the prognosis of the patient. Agatston CAC score quantification from CCTA scans is an important part of coronary artery disease (CAD)—related assessments. A fully automated analysis could increase workflow efficiency and aid clinicians in dealing with the increasing number of acquisitions to be processed and evaluated.
There were several limitations to this study. First, we found an overall underestimation of the calcium score from CCTA images when analyzing the agreement with the Agatston CAC score: our proposed method underestimated the scores of 24% of the patients. Considering the Spearman’s correlation and ICC values, we chose 650 HU as the optimal lower threshold for CCTA-CAC score calculation, as its efficacy was superior to that of other thresholds. While using lower thresholds may have led to more false-positive areas and a severe overestimation of the calcification severity, using a fixed threshold of 650 HU might have reduced the calcification areas per slice. Lower density calcium, which is associated with a higher risk of future cardiovascular events, was ignored at 650 HU (41), which was a possible reason for the underestimations. Another reason was the acquisition parameters of the CCTA and non-contrast CT, which resulted in a difference in CT values between the two modalities. The underestimation of calcification was therefore inevitable. In future, more external data will be used for verification.
Furthermore, few patients with severe calcification were included in the study (only 5 patients had a standard Agatston score of ≥400). Another weakness of this study was the fact that the evaluation was performed on good-quality images which did not contain artifacts, unusual lesions, or abnormalities. Also, the dataset was small. For this research, we were committed to obtaining plaque information on the premise of accurate coronary artery segmentation. We combined a deep learning method and conventional threshold method to establish a whole-process, automated, fast method for CAC quantification. We plan to evaluate our method using a larger number of multicenter datasets in the future. We intend to develop a more advanced self-adaptive calcification detection and quantification method to contrast attenuation. For the small amounts of coronary calcification that might be overlooked by the method proposed herein, we are now testing a deep learning-based extraction method while maintaining vessel segmentation as a prerequisite. In future, we will divide data into different groups according to calcification severity and image quality
Conclusions
In conclusion, we proposed an automated method for calcification quantification on CCTA, and it shows excellent correlation with standard Agatston CAC scores from non-contrast CT. Further development of this method might have the potential to reduce radiation exposure from separate non-contrast CT and CCTA images. We hope that it will be possible to automatically obtain the anatomical structure and analyze stenosis and plaque from CCTA scans in CAD-related assessments. A fully automated analysis could increase workflow efficiency and help clinicians deal with the increasing number of acquisitions to be processed and evaluated.
Acknowledgments
Funding: This study was supported by a grant from National Natural Science Foundation of China (U1908211), a grant from the Capital’s Funds for Health Improvement and Research (PXM2020_026272_000013), and a grant from National Key Research and Development Program of China (2016YFC1300300) for LX.
Footnote
Reporting Checklist: The authors have completed the STARD reporting checklist. Available at https://dx.doi.org/10.21037/qims-21-775
Conflicts of Interest: All authors have completed the ICMJE uniform disclosure form (available at https://dx.doi.org/10.21037/qims-21-775). WW and SW are employees of GE Healthcare China and provided data analysis support. The other authors have no conflicts of interest to declare.
Ethical Statement: The authors are accountable for all aspects of the work in ensuring that questions related to the accuracy or integrity of any part of the work are appropriately investigated and resolved. The study was conducted in accordance with the Declaration of Helsinki (as revised in 2013). The study protocol was approved by the Independent Ethics Committee of the Anzhen Hospital and the requirement for informed patient consent was waived for this retrospective study.
Open Access Statement: This is an Open Access article distributed in accordance with the Creative Commons Attribution-NonCommercial-NoDerivs 4.0 International License (CC BY-NC-ND 4.0), which permits the non-commercial replication and distribution of the article with the strict proviso that no changes or edits are made and the original work is properly cited (including links to both the formal publication through the relevant DOI and the license). See: https://creativecommons.org/licenses/by-nc-nd/4.0/.
References
- World Health Organization. Key facts Cardiovascular Diseases (CVDs), 2017. Available online: https://www.who.int/cardiovascular_diseases/en/
- Fihn SD, Gardin JM, Abrams J, Berra K, Blankenship JC, Dallas AP, et al. 2012 ACCF/AHA/ACP/AATS/PCNA/SCAI/STS Guideline for the diagnosis and management of patients with stable ischemic heart disease: a report of the American College of Cardiology Foundation/American Heart Association Task Force on Practice Guidelines, and the American College of Physicians, American Association for Thoracic Surgery, Preventive Cardiovascular Nurses Association, Society for Cardiovascular Angiography and Interventions, and Society of Thoracic Surgeons. J Am Coll Cardiol 2012;60:e44-e164. [Crossref] [PubMed]
- Arnett DK, Blumenthal RS, Albert MA, Buroker AB, Goldberger ZD, Hahn EJ, Himmelfarb CD, Khera A, Lloyd-Jones D, McEvoy JW, Michos ED, Miedema MD, Muñoz D, Smith SC Jr, Virani SS, Williams KA Sr, Yeboah J, Ziaeian B. 2019 ACC/AHA Guideline on the Primary Prevention of Cardiovascular Disease: Executive Summary: A Report of the American College of Cardiology/American Heart Association Task Force on Clinical Practice Guidelines. J Am Coll Cardiol 2019;74:1376-414. [Crossref] [PubMed]
- Knuuti J, Wijns W, Saraste A, Capodanno D, Barbato E, Funck-Brentano C, et al. 2019 ESC Guidelines for the diagnosis and management of chronic coronary syndromes. Eur Heart J 2020;41:407-77. [Crossref] [PubMed]
- Oudkerk M, Stillman AE, Halliburton SS, Kalender WA, Möhlenkamp S, McCollough CH, Vliegenthart R, Shaw LJ, Stanford W, Taylor AJ, van Ooijen PM, Wexler L, Raggi P. Coronary artery calcium screening: current status and recommendations from the European Society of Cardiac Radiology and North American Society for Cardiovascular Imaging. Int J Cardiovasc Imaging 2008;24:645-71. [Crossref] [PubMed]
- Genders TS, Pugliese F, Mollet NR, Meijboom WB, Weustink AC, van Mieghem CA, de Feyter PJ, Hunink MG. Incremental value of the CT coronary calcium score for the prediction of coronary artery disease. Eur Radiol 2010;20:2331-40. [Crossref] [PubMed]
- Agatston AS, Janowitz WR, Hildner FJ, Zusmer NR, Viamonte M Jr, Detrano R. Quantification of coronary artery calcium using ultrafast computed tomography. J Am Coll Cardiol 1990;15:827-32. [Crossref] [PubMed]
- Greenland P, Bonow RO, Brundage BH, Budoff MJ, Eisenberg MJ, Grundy SM, et al. ACCF/AHA 2007 clinical expert consensus document on coronary artery calcium scoring by computed tomography in global cardiovascular risk assessment and in evaluation of patients with chest pain: a report of the American College of Cardiology Foundation Clinical Expert Consensus Task Force (ACCF/AHA Writing Committee to Update the 2000 Expert Consensus Document on Electron Beam Computed Tomography). Circulation 2007;115:402-26. [Crossref] [PubMed]
- Folsom AR, Kronmal RA, Detrano RC, O'Leary DH, Bild DE, Bluemke DA, Budoff MJ, Liu K, Shea S, Szklo M, Tracy RP, Watson KE, Burke GL. Coronary artery calcification compared with carotid intima-media thickness in the prediction of cardiovascular disease incidence: the Multi-Ethnic Study of Atherosclerosis (MESA). Arch Intern Med 2008;168:1333-9. [Crossref] [PubMed]
- Budoff MJ, Gul KM. Expert review on coronary calcium. Vasc Health Risk Manag 2008;4:315-24. [Crossref] [PubMed]
- Detrano R, Guerci AD, Carr JJ, Bild DE, Burke G, Folsom AR, Liu K, Shea S, Szklo M, Bluemke DA, O'Leary DH, Tracy R, Watson K, Wong ND, Kronmal RA. Coronary calcium as a predictor of coronary events in four racial or ethnic groups. N Engl J Med 2008;358:1336-45. [Crossref] [PubMed]
- Polonsky TS, McClelland RL, Jorgensen NW, Bild DE, Burke GL, Guerci AD, Greenland P. Coronary artery calcium score and risk classification for coronary heart disease prediction. JAMA 2010;303:1610-6. [Crossref] [PubMed]
- Greenland P, LaBree L, Azen SP, Doherty TM, Detrano RC. Coronary artery calcium score combined with Framingham score for risk prediction in asymptomatic individuals. JAMA 2004;291:210-5. [Crossref] [PubMed]
- Hamon M, Biondi-Zoccai GG, Malagutti P, Agostoni P, Morello R, Valgimigli M, Hamon M. Diagnostic performance of multislice spiral computed tomography of coronary arteries as compared with conventional invasive coronary angiography: a meta-analysis. J Am Coll Cardiol 2006;48:1896-910. [Crossref] [PubMed]
- Mowatt G, Cook JA, Hillis GS, Walker S, Fraser C, Jia X, Waugh N. 64-Slice computed tomography angiography in the diagnosis and assessment of coronary artery disease: systematic review and meta-analysis. Heart 2008;94:1386-93. [Crossref] [PubMed]
- Budoff MJ, Dowe D, Jollis JG, Gitter M, Sutherland J, Halamert E, Scherer M, Bellinger R, Martin A, Benton R, Delago A, Min JK. Diagnostic performance of 64-multidetector row coronary computed tomographic angiography for evaluation of coronary artery stenosis in individuals without known coronary artery disease: results from the prospective multicenter ACCURACY (Assessment by Coronary Computed Tomographic Angiography of Individuals Undergoing Invasive Coronary Angiography) trial. J Am Coll Cardiol 2008;52:1724-32. [Crossref] [PubMed]
- Miller JM, Rochitte CE, Dewey M, Arbab-Zadeh A, Niinuma H, Gottlieb I, Paul N, Clouse ME, Shapiro EP, Hoe J, Lardo AC, Bush DE, de Roos A, Cox C, Brinker J, Lima JA. Diagnostic performance of coronary angiography by 64-row CT. N Engl J Med 2008;359:2324-36. [Crossref] [PubMed]
- Einstein AJ. Effects of radiation exposure from cardiac imaging: how good are the data? J Am Coll Cardiol 2012;59:553-65. [Crossref] [PubMed]
- Glodny B, Helmel B, Trieb T, Schenk C, Taferner B, Unterholzner V, Strasak A, Petersen J. A method for calcium quantification by means of CT coronary angiography using 64-multidetector CT: very high correlation with Agatston and volume scores. Eur Radiol 2009;19:1661-8. [Crossref] [PubMed]
- Mylonas I, Alam M, Amily N, Small G, Chen L, Yam Y, Hibbert B, Chow BJ. Quantifying coronary artery calcification from a contrast-enhanced cardiac computed tomography angiography study. Eur Heart J Cardiovasc Imaging 2014;15:210-5. [Crossref] [PubMed]
- Dalager MG, Bøttcher M, Andersen G, Thygesen J, Pedersen EM, Dejbjerg L, Gøtzsche O, Bøtker HE. Impact of luminal density on plaque classification by CT coronary angiography. Int J Cardiovasc Imaging 2011;27:593-600. [Crossref] [PubMed]
- Bischoff B, Kantert C, Meyer T, Hadamitzky M, Martinoff S, Schömig A, Hausleiter J. Cardiovascular risk assessment based on the quantification of coronary calcium in contrast-enhanced coronary computed tomography angiography. Eur Heart J Cardiovasc Imaging 2012;13:468-75. [Crossref] [PubMed]
- Ebersberger U, Eilot D, Goldenberg R, Lev A, Spears JR, Rowe GW, Gallagher NY, Halligan WT, Blanke P, Makowski MR, Krazinski AW, Silverman JR, Bamberg F, Leber AW, Hoffmann E, Schoepf UJ. Fully automated derivation of coronary artery calcium scores and cardiovascular risk assessment from contrast medium-enhanced coronary CT angiography studies. Eur Radiol 2013;23:650-7. [Crossref] [PubMed]
- Ahmed W, de Graaf MA, Broersen A, Kitslaar PH, Oost E, Dijkstra J, Bax JJ, Reiber JH, Scholte AJ. Automatic detection and quantification of the Agatston coronary artery calcium score on contrast computed tomography angiography. Int J Cardiovasc Imaging 2015;31:151-61. [Crossref] [PubMed]
- Lee H, Emrich T, Schoepf UJ, Brandt V, Leonard TJ, Gray HN, Giovagnoli VM, Dargis DM, Burt JR, Tesche C. Artificial Intelligence in Cardiac CT: Automated Calcium Scoring and Plaque Analysis. Curr Cardiovasc Imaging Rep 2020;13:1-9. [Crossref]
- Weigold WG, Abbara S, Achenbach S, Arbab-Zadeh A, Berman D, Carr JJ, Cury RC, Halliburton SS, McCollough CH, Taylor AJSociety of Cardiovascular Computed Tomography. Standardized medical terminology for cardiac computed tomography: a report of the Society of Cardiovascular Computed Tomography. J Cardiovasc Comput Tomogr 2011;5:136-44. [Crossref] [PubMed]
- Tang CX, Liu CY, Lu MJ, Schoepf UJ, Tesche C, Bayer RR 2nd, et al. CT FFR for Ischemia-Specific CAD With a New Computational Fluid Dynamics Algorithm: A Chinese Multicenter Study. JACC Cardiovasc Imaging 2020;13:980-90. [Crossref] [PubMed]
- Milletari F, Navab N, Ahmadi SA. V-net: Fully convolutional neural networks for volumetric medical image segmentation. In: 2016 fourth international conference on 3D vision (3DV). Los Alamitos, CA, USA: IEEE, 2016:565-71.
- Adams R, Bischof L. Seeded region growing. IEEE Transactions on pattern analysis and machine intelligence 1994;16:641-7. [Crossref]
- Ramya A, Murugan D, Murugeswari G, Joseph N. Adaptive multi-threshold based de-noising filter for medical image applications. Int J Comput Vis Robot 2019;9:272-92. [Crossref]
- Rosner B. Fundamentals of biostatistics. Boston, MA, USA: Cengage learning, 2015.
- Akram K, Rinehart S, Voros S. Coronary arterial atherosclerotic plaque imaging by contrast-enhanced computed tomography: fantasy or reality? J Nucl Cardiol 2008;15:818-29. [Crossref] [PubMed]
- Wolterink JM, Leiner T, de Vos BD, van Hamersvelt RW, Viergever MA, Išgum I. Automatic coronary artery calcium scoring in cardiac CT angiography using paired convolutional neural networks. Med Image Anal 2016;34:123-36. [Crossref] [PubMed]
- Shareghi S, Ahmadi N, Young E, Gopal A, Liu ST, Budoff MJ. Prognostic significance of zero coronary calcium scores on cardiac computed tomography. J Cardiovasc Comput Tomogr 2007;1:155-9. [Crossref] [PubMed]
- Heng SY, Ho JS, Saffari SE, Huang Z, Cheah FK, Chua SJ, Keng YJ, Baskaran L, Tan SY. Prognostic Value of Coronary Artery Calcium in a Multi-Ethnic Asian Cohort. Cardiovasc Imaging Asia 2021;5:63-71. [Crossref]
- Hunold P, Vogt FM, Schmermund A, Debatin JF, Kerkhoff G, Budde T, Erbel R, Ewen K, Barkhausen J. Radiation exposure during cardiac CT: effective doses at multi-detector row CT and electron-beam CT. Radiology 2003;226:145-52. [Crossref] [PubMed]
- Kwon H, Cho J, Oh J, Kim D, Cho J, Kim S, Lee S, Lee J. The adaptive statistical iterative reconstruction-V technique for radiation dose reduction in abdominal CT: comparison with the adaptive statistical iterative reconstruction technique. Br J Radiol 2015;88:20150463. [Crossref] [PubMed]
- Vonder M, Pelgrim GJ, Meyer M, Henzler T, Oudkerk M, Vliegenthart R. Dose reduction techniques in coronary calcium scoring: The effect of iterative reconstruction combined with low tube voltage on calcium scores in a thoracic phantom. Eur J Radiol 2017;93:229-35. [Crossref] [PubMed]
- Pan YK, Sun MH, Wang JJ, Chen XB, Kan XJ, Ge YH, Guo ZP. Effect of different reconstruction algorithms on coronary artery calcium scores using the reduced radiation dose protocol: a clinical and phantom study. Quant Imaging Med Surg 2021;11:1504-17. [Crossref] [PubMed]
- Berrington de González A, Mahesh M, Kim KP, Bhargavan M, Lewis R, Mettler F, Land C. Projected cancer risks from computed tomographic scans performed in the United States in 2007. Arch Intern Med 2009;169:2071-7. [Crossref] [PubMed]
- Criqui MH, Denenberg JO, Ix JH, McClelland RL, Wassel CL, Rifkin DE, Carr JJ, Budoff MJ, Allison MA. Calcium density of coronary artery plaque and risk of incident cardiovascular events. JAMA 2014;311:271-8. [Crossref] [PubMed]