Comparison of virtual monoenergetic imaging between a rapid kilovoltage switching dual-energy computed tomography with deep-learning and four dual-energy CTs with iterative reconstruction
Introduction
Dual-energy spectral imaging is increasingly used in clinical routine for abdominal CT explorations (1-9). Radiologists use different types of images including low-energy levels virtual monoenergetic images (VMIs) to assess the contrast-enhanced lesions and/or tissues and thus improve their detection and/or characterization (1-9). To obtain these VMIs, it is necessary to use two spectra of low and high-energy photons (6,10,11). Various DECT platforms may be used to obtain these two spectra (acquisition or reconstruction) with specific DECT algorithms (projection-based techniques or image-based techniques) (11,12). Each platform has different technological principles and therefore has a different impact on spectral performance (12).
Early in 2021, a study was published by our team comparing the spectral performance of four DECT platforms available from three CT manufacturers: fast kV-switching CT (KVSCT-GE, GE Healthcare), split filter CT (SFCT, Siemens Healthineers), dual-source CT (DSCT; Siemens Healthineers) and dual-layer (DLCT, Philips Healthcare) (12). This comparison was performed on VMIs and iodine maps reconstructed only with Filtered Back Projection (FBP) using parameters and dose levels close to those used in clinical practice for DECT examinations of the abdomen. Spectral performance was studied using a task-based image quality assessment (noise magnitude, noise texture, spatial resolution and detectability of contrast-enhanced lesions) often used in conventional single-energy CT (SECT) (13-22) and increasingly used in DECT (12,23,24). However, this study did not compare the spectral performance of these platforms with the use of IR (hybrid or advanced/partial Model-based IR) algorithms used in clinical practice. Indeed, these reconstruction algorithms are regularly used to reduce the image noise generated in low keV VMIs and thus to improve lesion detectability at these energy levels (23-26). Furthermore, none of the DECT platforms developed by Canon Medical Systems were evaluated as they were not available at the time of the study.
A new DECT platform has recently been developed by Canon Medical Systems on the Aquilion One PRISM Edition CT system (27,28). It is a rapid kV-switching technique to obtain both photon spectra. The system switches quickly and repeatedly (<1 ms) from high (135 kVp) to low kVp (80 kVp) as the tube detector rotates around the patient. The process of material decomposition, essential for generating VMIs in particular, is performed in the raw-data domain itself rather than post-reconstruction in the image domain. On this platform, the images are now reconstructed only with a Deep Learning Spectral Reconstruction (DLSR). As expressed by Boedeker et al. and by Kojima et al., this algorithm takes advantage of the fact that much of the anatomical data in low- and high-kilovolt views, at a specific location, is common to the two views. It is the case for example for the high spatial frequency information. The low- and high-energy views differ by the degree of attenuation of the X-ray beam by the patient or object scanned. DLSR works by transforming views of one energy into the other to create Deep Learning Views (DLVs). There are generated by the neural network using measured data from both the opposite-energy views as well as adjacent same-energy views. The algorithm was trained on complete measured sinograms acquired at each energy for a wide variety of patient and phantom attenuation levels. A complete sinogram for each kV is then generated by the DLVs, for the measured views at each energy level. Last, spectral reconstruction is performed. The established noise reduction capabilities of deep learning are used to create low-noise spectral CT image data (27,28). However, DLSR can only be used for a single slice thickness of 0.5 mm and three reconstruction kernels (including Body Spectral) for all three levels (Mild, Standard and Strong). Furthermore, slices can be thickened remotely using the Vitrea workstation. To the best our knowledge, only one study has ever been conducted on this new spectral platform (28). However, the authors did not perform a task-based image quality assessment, they only evaluated the accuracy of CT number on VMIs and the precision of iodine quantification on iodine images.
The purpose of this phantom study was to assess the spectral performance of the rapid-KVSCT platform with the DLSR algorithm developed by Canon Medical System and to compare its spectral performance with four other DECT platforms equipped with different IR algorithms. To do this, we used the same methodology as previously published by our Institution (12), but focused only on the low keV VMIs clinically used for DECT imaging of the abdomen. We also performed a task-based image quality assessment: the noise-magnitude, noise-texture, and the spatial resolution and detectability of two simulated contrast-enhanced lesions were evaluated.
The following article is presented in accordance with the MDAR checklist (available at https://dx.doi.org/10.21037/qims-21-708).
Methods
Details on phantoms can be found in the Supplementary file (Appendix 1).
Phantoms
An image quality phantom was used to measure physical metrics such as the noise power spectrum (NPS) and the task-based transfer function (TTF) (Figure 1A). A multi-energy CT phantom was used to assess the accuracy of CT numbers and to compute the contrast between the soft tissue and two iodine inserts (Figure 1B). High-density inserts were evenly distributed throughout the phantom to minimize reconstruction artifacts.
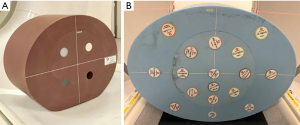
CT scanners and scanning protocols
Acquisitions were performed on the four DECT platforms previously assessed by our team and on an Aquilion One PRISM Edition CT system (KVSCT-Canon, Canon Medical Systems, Japan). With this latter CT system, dual-energy images can be obtained using a rapid kV switching technique such as the Revolution HD (GE Healthcare) CT system but using DLSR to reconstruct spectral images (second version).
Phantom acquisitions were performed using standard clinical parameters of abdomen-pelvic examinations for each DECT platform (Table 1). Five acquisitions were performed for the KVSCT-Canon but only one for the other DECT platforms. For all DECT platforms, the tube current modulation system was disabled to set mAs and obtain a volume CT dose index (CTDIvol) close to 10 mGy (i.e., the “diagnostic guide value” of the national diagnostic reference level; CTDIvol: 11 mGy). However, this dose level can be considered as routinely low in radiologic fields.
Table 1
Dual-energy CT (DECT) platform | Rapid kVp switching (KVSCT-Canon) | Fast kVp switching (KVSCT-GE) | Split filter (SFCT) | Dual-source (DSCT) | Dual-layer detector (DLCT) |
---|---|---|---|---|---|
Manufacturer | Canon Medical Systems | GE Healthcare | Siemens Healthineers | Siemens Healthineers | Philips Medical system |
CT scan model | Aquilion | Revolution | Somatom | Somatom | IQon Spectral CT |
One PRISM | GSI | Edge | Force | ||
Tube voltage (kVp) | 80/135 | 80/140 | AuSn120 | 80/Sn150 | 120 |
Pitch | 0.813 | 1.375 | 0.3 | 0.6 | 1.234 |
Tube current (mAs) | 140 | 191 (GSI 22) | 468 | 278/139 | 111 |
Rotation time (s) | 0.5 | 0.7 | 0.33 | 0.5 | 0.5 |
CTDIvol (mGy) | 10.10 | 10.76 | 10.00 | 9.93 | 10.00 |
Beam collimation | 80×0.5 mm | 64×0.625 mm | 64×0.6 mm | 128×0.6 mm | 64×0.625 mm |
Slice thickness/overlapped (mm) | 0.5/0.5 mm; 1.5/1.5 mm* | 1.25/1.25 mm | 1.5/1.25 mm | 1.5/1.25 mm | 1.5/1.25 mm |
Reconstruction algorithm | DLSR - Standard | ASIR 50% | ADMIRE 3 | ADMIRE 3 | iDose4 4 |
Reconstruction kernel | Body Spectral | Standard | Br38 | Br40 | B |
Workstation | Vitrea | Advantage Window | Syngo.Via | Syngo.Via | IntelliSpace |
Viewing mode | – | Mono | Monoenergetic+ | Monoenergetic+ | MonoE |
VMI range | 40–135 keV | 40–140 keV | 40–190 keV | 40–190 keV | 40–200 keV |
*, the slices with a thickness of 1.5/1.5 mm were generated after the reconstruction process using the Vitrea workstation. ADMIRE, advanced modeled iterative reconstruction; ASIR, adaptive statistical iterative reconstruction; CTDIvol, volume CT dose index; DLSR, deep learning spectral reconstruction; iDose4, intelligent dose; VMI, virtual monoenergetic images.
For the four DECT platforms, the same reconstruction parameters as those previously published were used, except for the reconstruction algorithms (12). Indeed, the IR (hybrid or Model-based IR) algorithm used for each DECT platform was selected to reconstruct raw data using the respective intermediate IR level.
For the Aquilion One PRISM Edition, raw data were reconstructed using the intermediate level (Standard) of the DLSR algorithm, the spectral reconstruction kernel “Body Spectral” and a slice thickness of 0.5 mm (0.5 mm increment). For this system, no other slice thickness and reconstruction algorithm could be selected. For the Multi-energy CT phantom, a 420-mm field of view (FOV) was used to visualize all the inserts present in the phantom. For the ACR phantom, a 250-mm FOV was used to fit closer to the phantom modules used in order to improve task-based image quality assessment.
For each acquisition, VMIs were reconstructed using the Vitrea Workstation (Canon Medical Systems, Minnetonka, Minnesota) for the 5 lowest energy levels (40/50/60/70/80 keV) usually used in clinical practice to detect or characterize abdominal lesions (1,2,4-6). VMIs were reconstructed for a slice thickness of 0.5 mm (0.5 mm increment) usually used in clinical practice and for a slice thickness of 1.5 mm (1.5 mm increment) to be close to other DECT platforms.
Assessment of CT numbers on VMIs
Measurement of CT numbers (NCT) was performed in a single slice, at the center of the Multi-energy phantom. One circular region of interest (ROI; diameter of 2 cm) was placed semi-automatically in the three selected inserts. The mean pixel attenuation in each ROI was computed for 5 energy levels on VMIs. The contrast between the soft tissue and each simulated contrast-enhanced blood insert was computed for each energy level (Figure 2). These inserts were used to approach the contrast of a hepatocellular carcinoma during arterial phase or that of a hyper vascularized metastasis (23).
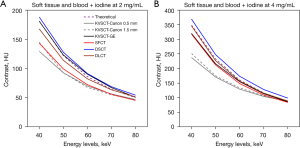
The accuracy of the mean attenuation was obtained for each insert using the root-mean-square deviation (RMSDHU) between measured and theoretical CT numbers over 5 energy levels on VMIs using the following formula:
where, corresponds to the CT number measured in each ROI for energy level Ei, is the respective theoretical value and L refers to the number of energy levels.
Task-based image quality assessment on VMIs
Task-based image quality was assessed using the imQuest software (version 7.1, Duke University). Noise magnitude and texture were assessed using the NPS and spatial resolution using the TTF (13,14). The detectability index (d’) estimated the radiologist’s capacity to detect the lesions.
NPS
To compute the NPS on the same volume in the z-axis (30 mm), 60 consecutive axial slices for the KVSCT-Canon at 0.5 mm, 20 for the KVSCT-Canon at 1.5 mm and 24 for the four other DECT platforms were used. Two square regions of interest (ROIs) of 64×64 pixels in the uniform section (module 3) of the ACR phantom (Figure 1A) were used and the NPS was calculated as follows:
where Δx and Δy are the pixel size in the x- and y-directions, respectively; FFT is the Fast Fourier Transform; Lx and Ly are the lengths of the ROIs in the x- and y-directions; NROI is the number of ROIs; ROIi(x,y) is the mean pixel value measured of a ROI at the position (x,y) and FITi(x,y) is a 2nd order polynomial fit of ROIi(x,y).
The area under the NPS curve was used to assess the modifications of the image noise, i.e.,. The average spatial frequency (fav) of the NPS curve was used to evaluate the noise texture by describing the overall frequency content of the NPS, as follows:
where f is the radial spatial frequency and NPS(f) is the radially re-binned/average 1D NPS (14).
TTF
The TTF was assessed using the acrylic insert of the ACR phantom Module 1 (Figure 1B) as reported by Richard et al. (20). This insert has contrast close to the contrasts of the clinical tasks simulated in this study.
A circular ROI was positioned around the insert. To measure the edge spread function (ESF), the circular-edge technique was used. The ESF was obtained by calculating the radius of each pixel from the center of the insert. The line spread function (LSF) was obtained by derivation of the ESF, and the TTF was generated from the LSF normalized Fourier transformation. The TTF was computed on the same volume in the z-axis (30 mm): 60 consecutive axial slices for the KVSCT-Canon at 0.5 mm, 20 for the KVSCT-Canon at 1.5 mm and 24 for the four other DECT platforms, as to limit the image-noise effect.
Detectability index
The detectability index (d’) was computed using a non-prewhitening observer model with eye filter (), as follows:
where u and v are the spatial frequencies in the x- and y-directions, E the eye filter that models the human visual system sensitivity to different spatial frequencies, and W(u,v) the task function defined as:
where h1(x,y) and h2(x,y) correspond to the object present and the object absent hypotheses, respectively.
The eye filter was modeled according to the visual response function (29).
The TTF on the acrylic insert and the NPS were computed and combined with a task function to compute a d’ estimating the detectability of two circular contrast-enhanced lesions of 10-mm diameter. The contrast of each clinical task was defined directly from the NCT variations between the soft tissue insert and the blood-mimicking material plus iodine at 2.0 mg/mL (theoretical contrast value at 70 keV: 64 HU) or 4.0 mg/mL (theoretical contrast value at 70 keV: 116 HU) inserts. It allowed taking into account the NCT variations depending on the keV (Figure 2).
A zoom factor of 1.5, a 500-mm viewing distance and a 500-mm field of view were used to calculate d’.
Results
Assessment of CT numbers on VMIs
The measured and theoretical CT numbers for the five lowest energy levels and the RMSDHU for all inserts and DECT platforms are depicted in Table 2. For each insert and DECT platform, the CT numbers decreased as the energy levels increased, except for SFCT for the soft tissue insert (on average 30.8±1.9 HU for all keV).
Table 2
Inserts | Energy levels (keV) | Theoretical values | KVSCT-Canon | KVSCT-GE | SFCT | DSCT | DLCT | |
---|---|---|---|---|---|---|---|---|
0.5 mm/0.5 mm | 1.5 mm/1.5 mm | |||||||
Soft tissue | 40 keV | 49 | 52 | 50 | 38 | 28 | 59 | 62 |
50 keV | 39 | 45 | 44 | 32 | 30 | 46 | 47 | |
60 keV | 32 | 41 | 41 | 26 | 31 | 38 | 39 | |
70 keV | 28 | 36 | 39 | 25 | 32 | 34 | 35 | |
80 keV | 26 | 36 | 37 | 25 | 33 | 31 | 32 | |
RMSDHU | – | 7.6 | 8.2 | 6.6 | 10.8 | 7.0 | 8.6 | |
Blood mimicking material plus iodine at 2.0 mg/mL | 40 keV | 230 | 181 | 195 | 219 | 171 | 247 | 230 |
50 keV | 161 | 137 | 137 | 155 | 129 | 174 | 161 | |
60 keV | 118 | 110 | 108 | 116 | 103 | 130 | 121 | |
70 keV | 92 | 92 | 94 | 92 | 88 | 102 | 98 | |
80 keV | 76 | 83 | 83 | 76 | 78 | 85 | 83 | |
RMSDHU | – | 24.9 | 19.9 | 5.7 | 30.8 | 12.5 | 4.3 | |
Blood mimicking material plus iodine at 4.0 mg/mL | 40 keV | 397 | 289 | 301 | 358 | 345 | 427 | 406 |
50 keV | 271 | 215 | 219 | 248 | 242 | 293 | 274 | |
60 keV | 192 | 169 | 174 | 181 | 179 | 212 | 196 | |
70 keV | 144 | 142 | 145 | 138 | 141 | 161 | 150 | |
80 keV | 114 | 123 | 124 | 111 | 116 | 129 | 120 | |
RMSDHU | – | 55.5 | 49.8 | 21.1 | 27.3 | 21.4 | 6.0 |
KVSCT-Canon, rapid kV switching CT; DLCT, dual-layer CT; DSCT, dual-source CT; KVSCT-GE, fast kV switching CT; RMSD, root-mean square deviation; SFCT, split filter CT.
The CT numbers measured were higher than the theoretical values for all inserts for DLCT and DSCT. For KVSCT-GE and SFCT, measured CT numbers were lower than or similar theoretical values, except at 70 and 80 keV for all inserts with SFCT. For the KVSCT-Canon, measured CT numbers were higher than theoretical values for the soft tissue insert but the opposite for both other inserts (except at 80 keV). Similar CT numbers were found between both slice thicknesses for KVSCT-Canon (2%±3%).
For the soft tissue insert, lower RMSDHU was found for KVSCT-GE and higher for SFCT (Table 2). RMSDHU ranged from 7.0 to 8.6 HU for all other DECT platforms. For the insert with blood-mimicking material plus iodine at 2.0 mg/mL, the RMSDHU was lower for KVSCT-GE and DLCT and higher for KVSCT-Canon and SFCT. For the insert with blood-mimicking material plus iodine at 4.0 mg/mL, the RMSDHU was lower for DLCT and higher for KVSCT-Canon. RMSDHU ranged from 21.1 to 27.3 HU for other DECT platforms. RMSDHU for both blood-mimicking material plus iodine insert were reduced with the increase of the slice thickness for KVSCT-Canon and the opposite for the soft tissue insert.
Figure 2 shows the contrast variations between the soft tissue insert and the blood-mimicking material plus iodine insert. For both inserts, the measured contrast values were lower than the theoretical values for KVSCT-Canon, SFCT and DLCT but the opposite for the DSCT platform. For KVSCT-GE, the measured contrast values between the soft tissue and the blood-mimicking material plus iodine at 2 mg/mL inserts were higher than theoretical values (Figure 2A), but the opposite for the contrast between the soft tissue and the blood-mimicking material plus iodine at 4 mg/mL inserts (Figure 2B).
NPS
Figure 3 depicts the noise magnitude and the average NPS spatial frequency (fav) for the five energy levels and for all DECT platforms.
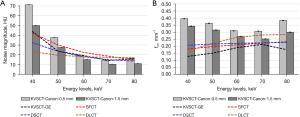
Noise magnitude
For KVSCT-Canon, the noise magnitude decreased from 40 to 70 keV (−80% with 0.5 mm and −79% with 1.5 mm) and increased from 70 to 80 keV (15% and 4%, respectively; Figure 3A). Similar outcomes were found for KVSCT-GE with a decrease of −67% from 40 to 70 keV and an increased afterwards (10%). For other DECT platforms, the noise magnitude decreased as the keV increased. From 40 to 70 keV, a similar decrease of noise magnitude was reported for SFCT (56%) and DSCT (−54%), and a lower decrease for DLCT (−25%). For KVSCT-Canon, the noise magnitude was reduced by −28%±4% with the slice thickness of 1.5 mm compared to 0.5 mm.
At 40 and 50 keV, the highest noise magnitude values were found for KVSCT-Canon at 0.5 mm. Lowest image noise magnitude were found for KVSCT-Canon at 1.5 mm for all keV, except at 40 and 50 keV where image noise were lower with DLCT. At 60 keV, similar noise magnitude values were found for KVSCT-Canon at 0.5 mm, DLCT, DSCT and KVSCT-GE (19.5±1.8 HU).
Noise texture
Regardless of the energy level, the highest fav values were found for KVSCT-Canon at 0.5 mm and the lowest for KVSCT-GE (Figure 3B).
For KVSCT-Canon at 0.5 mm, the fav values shifted towards lower frequencies from 40 to 70 keV (0.39 to 0.31 mm−1) but shifted towards higher frequencies thereafter (0.38 mm−1). A similar pattern was found for KVSCT-Canon at 1.5 mm with lower fav values (0.34 to 0.25 and 0.30 mm−1). The opposite pattern was found for KVSCT-GE, fav values increased from 40 to 70 keV (0.13 to 0.21 mm−1) and decreased thereafter (0.18 mm−1). For SFCT, DSCT and DLCT, the fav values increased from 40 to 80 keV (0.18 to 0.23 mm−1 for SFCT, 0.21 to 0.23 mm−1 for DSCT and 0.16 to 0.28 mm−1 for DLCT).
The peak-normalized NPS curves and the spatial frequency of NPS peaks are presented in Figure 4 for each platform at different keVs. For KVSCT-Canon, NPS peaks were found at low frequency (0.05 mm−1) but a second peak was found for the highest spatial frequency (0.32 mm−1 at 0.5 mm and 0.28 mm−1 at 1.5 mm). Similar patterns were found at 40 and 50 keV for SFCT with a spatial frequency of 0.05 and 0.21 mm−1.
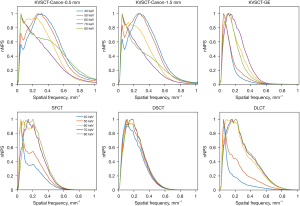
TTF
Figure 5 depicts the values of TTF at 50% (f50) and at 10% (f10) for the five energy levels and for all DECT platforms. For all DECT platforms, the TTF values at 50% (f50) decreased as the energy level increased (Figure 5A). From 40 to 80 keV, the f50 values decreased from 0.36 to 0.29 mm−1 for KVSCT-Canon at 0.5 mm, from 0.32 to 0.27 mm−1 for KVSCT-Canon at 1.5 mm, from 0.68 to 0.22 mm−1 for KVSCT-GE, from 0.58 to 0.37 mm−1 for SFCT, from 0.49 to 0.29 mm−1 for DSCT and from 0.86 to 0.29 mm−1 for DLCT. The highest f50 values were found for SFCT (except at 40 keV) and the lowest values for KVSCT-Canon (except at 70 and 80 keV, KVSCT-GE).
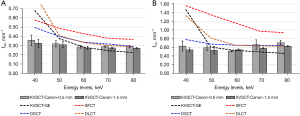
Values of TTF at 10% (f10) decreased as the energy level increased (Figure 5B), except for the KVSCT-Canon. For KVSCT-Canon at 0.5 mm, f10 decreased from 40 to 60 keV but similar values were found for KVSCT-Canon at 1.5 mm. For both slice thicknesses, f10 values increased from 60 to 80 keV.
TTF curves for all DECT platforms according to energy levels are depicted in the Supplementary file (Appendix 1).
Detectability indexes
The detectability indexes (d’) obtained for the two simulated contrast-enhanced lesions for each DECT platform and the 5 energy levels are depicted in Figure 6. d’ values increased as the contrast of the simulated lesions increased (Figure 6A,6B).
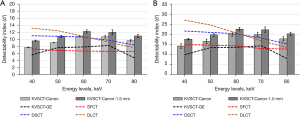
For KVSCT-Canon and both lesions, d’ increased from 40 to 60 keV (on average by 41%±1% at 0.5 mm and 29%±1% at 1.5 mm), then stabilized from 60 to 70 keV and decreased afterwards (on average by −11%±1% and −9%±1%, respectively). For both lesions, d’ values were increased by 1.2 times with 1.5 mm compared with 0.5 mm. For KVSCT-GE, d’ values increased from 40 to 50 keV (on average by 36%±1%), then stabilized between 50 and 70 keV and decreased thereafter (on average by −44%±1%). d’ decreased between 40 and 70 keV for DSCT (−12% at 2 mg/mL and −16% at 4 mg/mL) and DLCT (−30% and −38%, respectively). For SFCT, d’ decreased from 40 to 80 keV (−9% at 2 mg/mL and −15% at 4 mg/mL).
For both lesions, highest d’ values were found for DLCT followed with DSCT at 40 and 50 keV and for KVSCT-Canon at 1.5 mm for other keV. At 60 keV, similar d’ values were found for KVSCT-Canon at 0.5 mm, DSCT and DLCT. At 70 and 80 keV, the highest d’ values were found for KVSCT-Canon at 0.5 and 1.5 mm followed with DSCT. Overall, the lowest d’ values were found for SFCT and KVSCT-GE.
Discussion
For the first time, we assessed the performance of the rapid kV-switching CT (KVSCT-Canon) equipped with the deep learning spectral reconstruction algorithm (DLSR) developed by Canon Medical Systems using two slice thicknesses: the usual clinical slice thickness (0.5 mm) and a slice thickness of 1.5 mm. We performed a task-based image quality assessment to evaluate the noise characteristics, spatial resolution and detectability of two contrast-enhanced lesions on virtual monoenergetic images (VMIs) at low energy levels. These outcomes were compared to those of 4 DECT platforms each equipped with an iterative reconstruction algorithm (hybrid or model-based IR). For the slice thickness of 0.5 mm, we found that, this new platform at 40 and 50 keV, not only had one of the highest noise magnitude and lowest spatial resolution but also the best noise texture for all keV compared to other DECT platforms. Compared to 0.5 mm, the use of a slice thickness of 1.5 mm reduced the noise magnitude but reduced also the spatial resolution and degraded the noise texture. For both slice thicknesses, highest d’ values were found at 60 keV. The best lesion detectability was found for DLCT at 40 and 50 keV and KVSCT-Canon at 1.5 mm for other keV. For KVSCT-Canon at 0.5 mm, detectability was higher than other DECT platforms at 60, 70, and 80 keV and but lower than DLCT and DSCT at 40 and 50 keV.
The results obtained with KVSCT-Canon for both slice thicknesses showed that for the two inserts containing iodine and blood, the NCT values measured were lower compared to the theoretical values. This led to a lower accuracy of the HU for these two inserts and a lower contrast between the soft tissue and blood-mimicking iodine inserts compared to other DECT platforms. Regarding the noise magnitude, we found that, as for KVSCT-GE, it sharply decreased from 40 to 70 keV and weakly increased between 70 and 80 keV. Similar results were found between 50 and 100 keV by Kojima et al. on the Multi-energy CT phantom for a CTDIvol of 12.3 mGy, using the first version of DLSR and a slice thickness of 0.5 mm (28). The use of a slice thickness of 1.5 mm reduced the noise magnitude compared to 0.5 mm. Compared to the other platforms, the noise magnitude was the lowest for 60, 70 and 80 keV at 1.5 mm. At 0.5 mm, the noise magnitude was the highest at 40 and 50 keV and similar to other platform for the other keV. Furthermore, we found that the fav values decreased from 40 to 70 keV and increased from 70 to 80 keV. These fav variations along with keV were not found on any other DECT platform. Furthermore, KVSCT-Canon at 0.5 mm had the highest fav values inducing a less modified image texture than the other platforms. This result could be explained by the fact that a DLSR algorithm is compared with IR algorithms. Indeed, IR algorithms are known to result in a more or less important image smoothing (17-19). Although different, the first results of Deep learning image reconstruction in SECT showed that this algorithm allowed to reduce the noise while preserving the image texture compared to IR and model-based IR algorithms (30-33). Furthermore, the increase in slice thickness led to decrease the fav values but these remain higher or similar to those of other platforms. Regarding spatial resolution, we found that, as for other DECT platforms, the f50 decreased as the keV increased. As for fav values, the increase of slice thickness led to decrease f50 values and reducing the spatial resolution. Compared to other platforms, the values of f50 for both slice thicknesses were among the lowest, especially from 40 to 60 keV. For other keV, the f50 values were close to those with KVSCT-GE, DLCT and DSCT using the 0.5 mm slice thickness. Furthermore, the NPS and TTF results obtained must be toned down. Indeed, the positioning and size of the ROIs used for the NPS may have impacted the NPS curves and favored the appearance of a second peak at low keV. In this study, the methodology proposed by Samei et al. was used and two NPS peaks appeared at low keV for SFCT and KVSCT-Canon (14). For the TTF, the ESF was conditioned for a CNRTotal of less than 15, i.e., at 40, 50 keV for all platforms and 60 keV for some (14,34). This conditioning tends to overestimate the TTF values and could explain the large differences in f50 and f10 obtained between these keVs. To improve the TTF calculation, the number of slices (not possible for the ACR phantom) and/or the number of acquisitions should be increased. Another solution would be to calculate the TTF in the Multi-energy phantom on the two inserts used to calculate d’. However, the positioning of ROIs equal to twice the size of the insert taking into account only the insert and the phantom background is hardly feasible. The use of a phantom containing a homogeneous module to calculate the NPS; and another module containing inserts with iodine concentrations making it possible to get closer to the simulated lesions and to increase the HU of the insert and thus the CNR, would be a good solution.
For both simulated contrast-enhanced lesions, the d’ values increased from 40 to 50 keV, peaked at 60 keV then 70 keV and decreased at 80 keV. Compared to other DECT platforms, d’ values were higher for KVSCT-Canon at 1.5 mm for 60, 70 and 80 keV and for DLCT for 40 and 50 keV. For KVSCT-Canon at 0.5 mm, d’ values were higher than for SFCT and KVSCT-GE for all keV (except SFCT at 40 keV). However, d’ values were lower than for DLCT and DSCT at 40 keV and 50 keV and higher at 70 and 80 keV. For each DECT platform, d’ variations were mainly related to the variation in the noise magnitude and contrast according to keV.
Several studies have shown the advantage of using 50–60 keV energy levels for the detection and characterization of hepatic, pancreatic, or renal lesions (1,2,4-6). The results obtained with the different DECT platforms showed that for these energy levels, d’ values are similar or higher compared to those obtained at 70 keV (approximately corresponding to a SECT acquisition at 120 kVp). Indeed, for KVSCT-Canon, we found that the d' values peaked at 60 keV. For SFCT, DSCT and DLCT, the highest values of d’ were obtained at 40 keV. For KVSCT-GE, the use of ASIR resulted in the highest d’ values between 50 and 60 keV. For all DECT platforms, the detectability of contrast-enhanced lesions should therefore be higher with low keV on VMIs compared to conventional SECT acquisitions. Various hypotheses to explain the differences found between the DECT platforms were listed by Greffier et al. (12). First, they can be explained by technological differences between the DECT platforms to obtain the two spectra of low and high energy photons. Indeed, a high overlap between the two spectra and poor spectral separation lead to an altered spectral performance. SFCT, which uses two filters to split the energy spectra into low (gold filter), and high-energy spectra (tin filter) and KVSCT, which switches between low and high-kVp, both show a non-homogeneous and limited spectral separation which could explain the poor results obtained compared to other techniques (11). Second, these differences can also be explained by differences in the way the spectra of high and low-energy sinograms are decomposed into basic sinograms. Indeed, this is done in the projection domain for KVSCT-GE, KVSCT-Canon and DLCT and in the image domain for SFCT and DSCT. In addition to these explanations, the differences in the operation between the different IR algorithms used or between IR and DLR algorithms should be considered. For the all IR algorithms used, these differences were previously studied in conventional SECT (19). However, to our knowledge, the differences between DLSR and IR algorithms on DECT images were not studied. In all cases, further patient studies should be performed to validate the quality of the low keV VMIs obtained with each DECT platform in clinical practice.
The outcomes of our study show that the use of IR algorithms makes it possible to reduce image noise on VMIs at low keV and facilitate their use for abdominal spectral CT examination. Indeed, an image with low noise and high contrast is essential to detect and characterize abdominal lesions. However, the use of these algorithms also changes the texture of the image and degrades the spatial resolution. These modifications can also perturb the radiologist in her interpretation. For KVSCT-Canon, although not available for the reconstruction process, the results of our study showed that there was a strong need to increase the slice thickness of VMIs directly on the dedicated workstation. This greatly reduces noise and improves lesion detectability. Using a slice thickness equivalent to other platforms shows that this KVSCT-Canon technology with DLSR has the lowest noise levels at 60, 70 and 80 keV and greatly increases detectability. These differences are certainly related to the use of this spectral reconstruction algorithm compared to IR algorithms for other platforms.
This study has several limitations. First, acquisitions were performed for only one dose level, for one level of DLR/IR, and with a single standard soft tissue reconstruction kernel. Different outcomes may be obtained if other combinations of parameters were used. Second, we compared the spectral performance with spectral images reconstructed with a DLR algorithm for a slice thickness of 0.5 mm and iterative algorithms for slice thicknesses close to 1.5 mm. In addition, for KVSCT-GE, the results should also be improved with the new GSI Xtream platform with the DLR TrueFidelity algorithm. However, this platform was also unavailable at the time of the study. Last, we defined two task functions to simulate the detection of contrast-enhanced lesions, according to the NCT variations as a function of keV for the two inserts available in the Multi-energy CT phantom. This choice allowed us to ensure the reproducibility of the results between the DECT platforms. Also, the contrast variations of both simulated lesions were taken into account according to the DECT performance of the platforms. It is now necessary to perform a clinical study on specific sized, contrast-enhanced lesions to confirm these results.
Conclusions
For the rapid kV-switching DECT with deep learning, the highest detectability of contrast-enhanced lesions was reported at 60 keV. For KVSCT-Canon, the highest detectability of contrast-enhanced lesions was found at 60 keV. The highest d’ values were found for DLCT at 40 and 50 keV and for KVSCT-Canon at 1.5 mm for other keV. For KVSCT-Canon at 0.5 mm, d’ values were lower than DSCT and DLCT at 40 and 50 keV but similar or higher afterwards.. The results found on phantoms should now be validated in clinical practice for the detection and/or characterization of abdominal lesions on VMIs at low keV.
Acknowledgments
We thank H. de Forges and T. Sawyers for their help in editing the manuscript. We thank C. Balta and A. Thay for their support.
Funding: None.
Footnote
Reporting Checklist: The authors have completed the MDAR checklist. Available at https://dx.doi.org/10.21037/qims-21-708
Conflicts of Interest: All authors have completed the ICMJE uniform disclosure form (available at https://dx.doi.org/10.21037/qims-21-708). The authors have no conflicts of interest to declare.
Ethical Statement: The authors are accountable for all aspects of the work in ensuring that questions related to the accuracy or integrity of any part of the work are appropriately investigated and resolved. Ethical approval and written informed consent are not applicable because it is a phantom study and not a patient study.
Open Access Statement: This is an Open Access article distributed in accordance with the Creative Commons Attribution-NonCommercial-NoDerivs 4.0 International License (CC BY-NC-ND 4.0), which permits the non-commercial replication and distribution of the article with the strict proviso that no changes or edits are made and the original work is properly cited (including links to both the formal publication through the relevant DOI and the license). See: https://creativecommons.org/licenses/by-nc-nd/4.0/.
References
- Agrawal MD, Pinho DF, Kulkarni NM, Hahn PF, Guimaraes AR, Sahani DV. Oncologic applications of dual-energy CT in the abdomen. Radiographics 2014;34:589-612. [Crossref] [PubMed]
- De Cecco CN, Boll DT, Bolus DN, Foley WD, Kaza RK, Morgan DE, Rofsky NM, Sahani DV, Schoepf UJ, Shuman WP, Siegel MJ, Vrtiska TJ, Yeh BM, Berland LL. White Paper of the Society of Computed Body Tomography and Magnetic Resonance on Dual-Energy CT, Part 4: Abdominal and Pelvic Applications. J Comput Assist Tomogr 2017;41:8-14. [Crossref] [PubMed]
- De Cecco CN, Schoepf UJ, Steinbach L, Boll DT, Foley WD, Kaza RK, Bolus DN, Morgan DE, Sahani DV, Shuman WP, Siegel MJ, Vrtiska TJ, Yeh BM, Berland LL. White Paper of the Society of Computed Body Tomography and Magnetic Resonance on Dual-Energy CT, Part 3: Vascular, Cardiac, Pulmonary, and Musculoskeletal Applications. J Comput Assist Tomogr 2017;41:1-7. [Crossref] [PubMed]
- Marin D, Boll DT, Mileto A, Nelson RC. State of the art: dual-energy CT of the abdomen. Radiology 2014;271:327-42. [Crossref] [PubMed]
- Wang Q, Shi G, Qi X, Fan X, Wang L. Quantitative analysis of the dual-energy CT virtual spectral curve for focal liver lesions characterization. Eur J Radiol 2014;83:1759-64. [Crossref] [PubMed]
- Si-Mohamed S, Douek P, Boussel L. Spectral CT: Dual energy towards multienergy CT. Journal d'imagerie diagnostique et interventionnelle 2019;2:32-45.
- Karçaaltıncaba M, Aktaş A. Dual-energy CT revisited with multidetector CT: review of principles and clinical applications. Diagn Interv Radiol 2011;17:181-94. [PubMed]
- Chandarana H, Megibow AJ, Cohen BA, Srinivasan R, Kim D, Leidecker C, Macari M. Iodine quantification with dual-energy CT: phantom study and preliminary experience with renal masses. AJR Am J Roentgenol 2011;196:W693-700. [Crossref] [PubMed]
- Jamali S, Michoux N, Coche E, Dragean CA. Virtual unenhanced phase with spectral dual-energy CT: Is it an alternative to conventional true unenhanced phase for abdominal tissues? Diagn Interv Imaging 2019;100:503-11. [Crossref] [PubMed]
- Alvarez RE, Macovski A. Energy-selective reconstructions in X-ray computerized tomography. Phys Med Biol 1976;21:733-44. [Crossref] [PubMed]
- McCollough CH, Leng S, Yu L, Fletcher JG. Dual- and Multi-Energy CT: Principles, Technical Approaches, and Clinical Applications. Radiology 2015;276:637-53. [Crossref] [PubMed]
- Greffier J, Si-Mohamed S, Dabli D, de Forges H, Hamard A, Douek P, Beregi JP, Frandon J. Performance of four dual-energy CT platforms for abdominal imaging: a task-based image quality assessment based on phantom data. Eur Radiol 2021;31:5324-34. [Crossref] [PubMed]
- Samei E, Richard S. Assessment of the dose reduction potential of a model-based iterative reconstruction algorithm using a task-based performance metrology. Med Phys 2015;42:314-23. [Crossref] [PubMed]
- Samei E, Bakalyar D, Boedeker KL, Brady S, Fan J, Leng S, Myers KJ, Popescu LM, Ramirez Giraldo JC, Ranallo F, Solomon J, Vaishnav J, Wang J. Performance evaluation of computed tomography systems: Summary of AAPM Task Group 233. Med Phys 2019;46:e735-56. [Crossref] [PubMed]
- Rotzinger DC, Racine D, Beigelman-Aubry C, Alfudhili KM, Keller N, Monnin P, Verdun FR, Becce F. Task-Based Model Observer Assessment of A Partial Model-Based Iterative Reconstruction Algorithm in Thoracic Oncologic Multidetector CT. Sci Rep 2018;8:17734. [Crossref] [PubMed]
- Greffier J, Larbi A, Frandon J, Moliner G, Beregi JP, Pereira F. Comparison of noise-magnitude and noise-texture across two generations of iterative reconstruction algorithms from three manufacturers. Diagn Interv Imaging 2019;100:401-10. [Crossref] [PubMed]
- Greffier J, Boccalini S, Beregi JP, Vlassenbroek A, Vuillod A, Dupuis-Girod S, Boussel L, Douek P, Si-Mohamed S. CT dose optimization for the detection of pulmonary arteriovenous malformation (PAVM): A phantom study. Diagn Interv Imaging 2020;101:289-97. [Crossref] [PubMed]
- Greffier J, Frandon J, Pereira F, Hamard A, Beregi JP, Larbi A, Omoumi P. Optimization of radiation dose for CT detection of lytic and sclerotic bone lesions: a phantom study. Eur Radiol 2020;30:1075-8. [Crossref] [PubMed]
- Greffier J, Frandon J, Larbi A, Beregi JP, Pereira F. CT iterative reconstruction algorithms: a task-based image quality assessment. Eur Radiol 2020;30:487-500. [Crossref] [PubMed]
- Richard S, Husarik DB, Yadava G, Murphy SN, Samei E. Towards task-based assessment of CT performance: system and object MTF across different reconstruction algorithms. Med Phys 2012;39:4115-22. [Crossref] [PubMed]
- Greffier J, Hamard A, Pereira F, Barrau C, Pasquier H, Beregi JP, Frandon J. Image quality and dose reduction opportunity of deep learning image reconstruction algorithm for CT: a phantom study. Eur Radiol 2020;30:3951-9. [Crossref] [PubMed]
- Si-Mohamed SA, Greffier J, Miailhes J, Boccalini S, Rodesch PA, Vuillod A, van der Werf N, Dabli D, Racine D, Rotzinger D, Becce F, Yagil Y, Coulon P, Vlassenbroek A, Boussel L, Beregi JP, Douek P. Comparison of image quality between spectral photon-counting CT and dual-layer CT for the evaluation of lung nodules: a phantom study. Eur Radiol 2021; Epub ahead of print. [Crossref] [PubMed]
- Greffier J, Dabli D, Hamard A, Akessoul P, Belaouni A, Beregi JP, Frandon J. Impact of dose reduction and the use of an advanced model-based iterative reconstruction algorithm on spectral performance of a dual-source CT system: A task-based image quality assessment. Diagn Interv Imaging 2021;102:405-12. [Crossref] [PubMed]
- Greffier J, Frandon J, Hamard A, Teissier JM, Pasquier H, Beregi JP, Dabli D. Impact of iterative reconstructions on image quality and detectability of focal liver lesions in low-energy monochromatic images. Phys Med 2020;77:36-42. [Crossref] [PubMed]
- Greffier J, Frandon J, Sadate A, Akessoul P, Belaouni A, Beregi JP, Dabli D. Impact of four kVp combinations available in a dual-source CT on the spectral performance of abdominal imaging: A task-based image quality assessment on phantom data. J Appl Clin Med Phys 2021;22:243-54. [Crossref] [PubMed]
- Dabli D, Frandon J, Hamard A, Belaouni A, Addala T, Beregi JP, Greffier J. Optimization of image quality and accuracy of low iodine concentration quantification as function of kVp pairs for abdominal imaging using dual-source CT: A phantom study. Phys Med 2021;88:285-92. [Crossref] [PubMed]
- Boedeker K, Hayes M, Zhou J, Zhang R, Z. Y. Deep Learning Spectral CT – Faster, easier and more intelligent. Whitepaper - Canon Medical Systems 2020.
- Kojima T, Shirasaka T, Kondo M, Kato T, Nishie A, Ishigami K, Yabuuchi H. A novel fast kilovoltage switching dual-energy CT with deep learning: Accuracy of CT number on virtual monochromatic imaging and iodine quantification. Phys Med 2021;81:253-61. [Crossref] [PubMed]
- Eckstein M, Bartroff J, Abbey C, Whiting J, Bochud F. Automated computer evaluation and optimization of image compression of x-ray coronary angiograms for signal known exactly detection tasks. Opt Express 2003;11:460-75. [Crossref] [PubMed]
- Akagi M, Nakamura Y, Higaki T, Narita K, Honda Y, Zhou J, Yu Z, Akino N, Awai K. Deep learning reconstruction improves image quality of abdominal ultra-high-resolution CT. Eur Radiol 2019;29:6163-71. [Crossref] [PubMed]
- Higaki T, Nakamura Y, Zhou J, Yu Z, Nemoto T, Tatsugami F, Awai K. Deep Learning Reconstruction at CT: Phantom Study of the Image Characteristics. Acad Radiol 2020;27:82-7. [Crossref] [PubMed]
- Singh R, Digumarthy SR, Muse VV, Kambadakone AR, Blake MA, Tabari A, Hoi Y, Akino N, Angel E, Madan R, Kalra MK. Image Quality and Lesion Detection on Deep Learning Reconstruction and Iterative Reconstruction of Submillisievert Chest and Abdominal CT. AJR Am J Roentgenol 2020;214:566-73. [Crossref] [PubMed]
- Brady SL, Trout AT, Somasundaram E, Anton CG, Li Y, Dillman JR. Improving Image Quality and Reducing Radiation Dose for Pediatric CT by Using Deep Learning Reconstruction. Radiology 2021;298:180-8. [Crossref] [PubMed]
- Maidment AD, Albert M. Conditioning data for calculation of the modulation transfer function. Med Phys 2003;30:248-53. [Crossref] [PubMed]