Using quantitative parameters derived from pretreatment dual-energy computed tomography to predict histopathologic features in head and neck squamous cell carcinoma
Introduction
Head and neck squamous cell carcinoma (HNSCC) is a malignancy developing from mucosal epithelium in the oral cavity, pharynx, larynx, and other sites. There are more than 500,000 new cases of HNSCC reported every year worldwide (1). HNSCC patients with high tumor grade, lymphovascular invasion (LVI), or perineural invasion (PNI) tend to demonstrate a poor prognosis in clinical series. Thus, the identification of histopathological features, including tumor grade, LVI, and PNI, before treatment could be used to stratify the prognosis of patients with HNSCC (2-7) and to identify patients who should receive more aggressive treatments (8).
Invasive biopsy is widely used to determine the histopathological features of HNSCC, but its application is limited due to complications, such as bleeding, and a lack of tumorous histopathological characteristics (9). Single-energy computed tomography (SDCT) shows the morphological features of HNSCC; however, as these morphological features are subjectively and qualitatively evaluated by radiologists, the results partially depend on the observers’ experience (10-12). As SDCT fails to accurately predict the histopathological features of tumors, a noninvasive quantitative analysis of the histopathological features before treatment could be clinically meaningful in treatment planning and consequently improve patient prognosis.
Dual-energy computed tomography (DECT) is an advanced imaging technique that allows 2 series of CT images to be acquired at different energies (13). DECT can provide a series of quantitative parameters, including iodine concentration (IC), attenuation values at different energy levels, such as 40–140 keV, and effective atomic number (Zeff), to represent the essential characteristics of tumors (14-18). These quantitative parameters have been shown to be beneficial in the differential diagnosis, staging, and prognostic prediction of HNSCC (19-22). However, very little is currently known about the value of quantitative parameters derived from DECT in the pretreatment prediction of histopathological features in patients with HNSCC. Thus, this study sought to determine whether quantitative parameters derived from pretreatment DECT could predict these histopathological features in patients with HNSCC.
We present the following article in accordance with the STARD reporting checklist (available at https://dx.doi.org/10.21037/qims-21-650).
Methods
Data collection occurred after the surgical pathological results were collected. The study was conducted in accordance with the Declaration of Helsinki (as revised in 2013). This retrospective study was approved by the Ethics Committee of Chongqing University Cancer Hospital, and written informed consent was waived.
From December 2018 to July 2020, 89 consecutive patients with findings highly suspicious of HNSCC underwent DECT. To be eligible to for study enrollment, patients had to meet the following inclusion criteria: (I) histopathologically confirmed HNSCC, (II) no history of allergy to iodine contrast agents, and (III) no history of antitumor therapy before DECT examination. Patients were excluded from this study if they (I) had severe image artifacts, (II) incomplete clinical and histopathological data, or (III) a primary tumor with a maximum diameter <10 mm on polyenergetic images (PEI).
DECT image acquisitions
All patients underwent dual-phase (nonenhanced phase and contrast agent-enhanced phase) DECT scans with a dual-source CT scanner (SOMATOM Drive, Siemens Healthineers, Forchheim, Germany) and 100 kVp and Sn140 kVp tubes. All the scans were performed with an automatic exposure control (CARE Dose 4D, Siemens Healthineers) under the following settings: a reference tube current time product of 104 mAs for the Sn140-kVp tube and 134 mAs for the 100-kVp tube, a collimation of 32 mm × 0.6 mm, a rotation time of 0.5 s, a pitch of 0.7, a section thickness of 1.5 mm, and a section increment of 1.2 mm.
Patients were then injected with nonionic contrast medium (ioversol, 320 mg/mL iodine; Jiangsu Hengrui Medicine, Jiangsu, China) via antecubital venous access. The iodinated contrast medium was injected at a dose of 1.5 mL/kg and a rate of 2.5 mL/s. A bolus-tracking technique was used to perform the enhanced phase with a delay of 30 s after the arcus aorta at the threshold of 100 HU.
DECT image reconstruction
All the postprocessing of the DECT images was performed at a dedicated workstation (syngo.via VB20A, Dual Energy, Siemens Healthineers). PEIs in both the unenhanced and enhanced phases were reconstructed with a mix factor of 0.4 (M_0.4; 40% of the low kV and 60% of the high-kV spectrum). Virtual monoenergetic images (VMIs) at 40 and 110 keV were reconstructed using contrast-enhanced phase images. The parameters of the reconstructed images were as follows: transverse sections; thickness, 1.5 mm; increment, 1.2 mm; and soft-tissue kernel, J30f.
Qualitative DECT imaging analysis
Two radiologists with 7 and 15 years of experience in head and neck CT imaging interpretation independently assessed the subjective and objective quality of the images using the same approach as that adopted in our previous study (see the Supplementary Materials) (23). The radiologists evaluated the morphological features on the VMIs with optimal image quality. The radiologists were blinded to patient information and the histopathological results. The morphological features included heterogeneous density (presence or absence), ill-defined margins (presence or absence), the infiltration of adjacent structures, such as muscle or cartilage or bone (presence or absence), and the degree of enhancement (high, intermediate, or low). The degree of enhancement was defined as per our previous study (23).
Analysis of quantitative DECT parameters
All the quantitative parameters were independently measured by the above mentioned 2 radiologists, who were blinded to patient information and the histopathological results. To assess intraobserver variability, the first radiologist evaluated the images twice with an interval of 2 weeks. The regions of interest (ROIs) were placed on a single slice in the largest tumoral area on the axial images, and areas of necrosis and artifact were avoided. The average values of the quantitative parameters (which were measured 3 times, twice by observer 1 and once by observer 2) were used for the subsequent analysis.
The normalized IC (NIC), slope of the spectral Hounsfield unit curve (λHU), and normalized Zeff (NZeff) values were calculated using the following formulas: NIC = IC value of primary lesion/IC value of common carotid artery; λHU = (attenuation value of primary lesion in 40 keV − attenuation value of primary lesion in 110 keV)/70; and NZeff = Zeff value of primary lesion/Zeff value of common carotid artery. IC values were measured in the “VNC” mode at the syngo.via workstation using the enhanced images. The attenuation values of the primary lesions at 40–140 keV VMIs (A40, A50, A60, A70, A80, A90, A100, A110, A120, A130, and A140) were measured. The Zeff values were measured in the “Rho/Zeff” mode at the syngo.via workstation using the nonenhanced phase images. The attenuation values in both the unenhanced phase (An) and the enhanced phase (Ae) PEIs were obtained. Their difference value (ΔA) was calculated as follows: ΔA = Ae − An.
Histopathological analysis
All the surgically resected HNSCC specimens were used for the pathological evaluations. A pathologist with 20 years of experience in head and neck pathology who knew the clinical information of patients but was blinded to the DECT data analyzed these samples using hematoxylin and eosin staining and an immunohistochemistry panel. The pathologically differentiated grades of HNSCC were divided into the following 3 groups according to the eighth edition of American Joint Committee on Cancer Stage (AJCC): well differentiated (grade I), moderately differentiated (grade II), and poorly differentiated (grade III). LVI status and PNI status were classified as either positive or negative. The histopathological results were used as reference standards according to the long-time experience.
Statistical analysis
Statistical analyses were performed using SPSS statistics version 25.0 (IBM Corporation, Armonk, NY, USA). All the variabilities of the quantitative parameters derived from the DECT were exploratory. Intraclass correlation coefficients (ICCs) and Bland-Altman plots were used to assess intra- and interobserver agreement in relation to the quantitative parameters. The normality of the quantitative data distribution was evaluated using the Kolmogorov-Smirnov test. An independent sample t test and a 1-way ANOVA with a Bonferroni post hoc test were used to assess the differences between the quantitative data with normal distributions. The Mann-Whitney U test and Kruskal-Wallis with a Bonferroni post hoc test were used to assess the differences between the quantitative data without normal distributions. The performances of the observers in distinguishing the histopathological features were evaluated by a receiver operating characteristic (ROC) curve analysis. The Youden index was used to determine the optimal threshold. The Delong test was used to compare the differences of the areas under the curves (AUCs). The independent predictive parameters for the histopathologic features were determined by a binary logistic regression analysis. A 2-sided P value <0.05 indicated a statistically significant difference. We performed an a priori power calculation using R 3.6.2 software with the “pwr” package (The R Foundation for Statistical Computing). The minimum sample size required to obtain 90% power in differentiating tumor grade, LVI, and PNI was 20 cases per group.
Results
Patients
Of the 89 patients enrolled in this study, 17 patients were excluded (5 due to poor quality images, 8 due to incomplete clinical or histopathological data, and 4 with maximum axial diameters of the primary tumor <10 mm). Ultimately, 72 patients were enrolled in the study (40 males and 32 females; median age 60 years, interquartile range, 53–70 years; see Figure S1). The clinical characteristics of the enrolled patients are shown in Table 1. No adverse events were observed in this study.
Table 1
Characteristic | Number | % |
---|---|---|
Anatomy | ||
Oral cavity | 33 | 45.8 |
Oropharynx | 6 | 8.3 |
Hypopharynx | 12 | 16.7 |
Larynx | 21 | 29.2 |
T stage | ||
T1 | 7 | 9.7 |
T2 | 23 | 31.9 |
T3 | 18 | 25 |
T4 | 24 | 33.3 |
N stage | ||
N0 | 41 | 56.9 |
N1 | 17 | 23.6 |
N2 | 12 | 16.7 |
N3 | 2 | 2.8 |
M stage | ||
M0 | 69 | 95.8 |
M1 | 3 | 4.2 |
Overall stage | ||
I | 5 | 6.9 |
II | 12 | 16.7 |
III | 20 | 27.8 |
IV | 35 | 48.6 |
Tumor grade | ||
Grade I | 17 | 23.6 |
Grade II | 30 | 41.7 |
Grade III | 25 | 34.7 |
Lymphovascular invasion | ||
Negative | 45 | 62.5 |
Positive | 27 | 37.5 |
Perineural invasion | ||
Negative | 48 | 66.7 |
Positive | 24 | 33.3 |
Intra-and interobserver agreement
The NIC, λHU, NZeff, A40, A50, A60, A70, A80, A90, A100, A110, A120, A130, A140, An, Ae, and ΔA showed good intra- and interobserver agreement (ICC: 0.886–0.997; see Table 2). The Bland-Altman plots of the NIC, λHU, NZeff, and A40 are shown in Figures 1,2.
Table 2
Parameters | Intraobserver agreement (95% CI) | Interobserver agreement (95% CI) |
---|---|---|
NIC | 0.924 (0.881–0.954) | 0.905 (0.853–0.940) |
λHU (HU/keV) | 0.997 (0.995–0.998) | 0.995 (0.992–0.997) |
NZeff | 0.917 (0.867–0.948) | 0.907 (0.851–0.942) |
A40 (HU) | 0.921 (0.835–0.966) | 0.901 (0.826–0.933) |
A50 (HU) | 0.915 (0.862–0.950) | 0.903 (0.835–0.940) |
A60 (HU) | 0.908 (0.826–0.937) | 0.902 (0.828–0.936) |
A70 (HU) | 0.912 (0.860–0.938) | 0.908 (0.855–0.946) |
A80 (HU) | 0.921 (0.873–0.941) | 0.910 (0.862–0.947) |
A90 (HU) | 0.914 (0.854–0.940) | 0.906 (0.855–0.950) |
A100 (HU) | 0.910 (0.843–0.936) | 0.900 (0.829–0.927) |
A110 (HU) | 0.913 (0.855–0.939) | 0.904 (0.839–0.933) |
A120 (HU) | 0.896 (0.815–0.933) | 0.889 (0.805–0.931) |
A130 (HU) | 0.892 (0.805–0.930) | 0.890 (0.800–0.928) |
A140 (HU) | 0.893 (0.811–0.935) | 0.889 (0.801–0.925) |
An (HU) | 0.950 (0.921–0.968) | 0.909 (0.854–0.943) |
Ae (HU) | 0.899 (0.839–0.937) | 0.890 (0.823–0.931) |
ΔA (HU) | 0.890 (0.821–0.928) | 0.886 (0.801–0.914) |
CT, computed tomography; CI, confidence interval; NIC, normalized iodine concentration; λHU, slope of the spectral Hounsfield unit curve; NZeff, normalized effective atomic number; VMI (+), noise-optimized virtual monoenergetic images; A40–140, attenuation value in enhanced VMIs (+) at 40–140 keV; An, attenuation value in nonenhanced polyenergetic images; Ae, attenuation value in enhanced polyenergetic images.
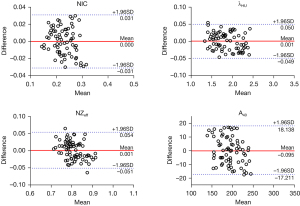
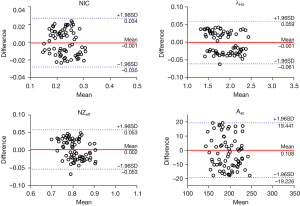
Comparison of DECT morphological features in relation to different histopathological features
The VMIs at 40 keV had the highest overall image quality and demarcation of lesion margins scores, signal-to-noise ratio (SNR), and contrast-to-noise ratio (CNR) (see Tables S1,S2 and Figure S2). Thus, a qualitative DECT analysis of HNSCC was performed on the 40 keV VMIs. None of the morphological features could be used to distinguish the grade III group from the grade I and II groups, the LVI-positive group from the LVI-negative group, and the PNI-positive group from the PNI-negative group (see Table S3).
Comparison of DECT parameters in relation to different histopathologic features
The DECT quantitative parameters and conventional attenuation values are summarized in Tables 3,4 and Figures 3,4. The NIC, λHU, NZeff, and A40 values derived from the DECT in the grade III group were significantly higher than those in the grade I and II groups (all P values <0.05). The NIC, λHU, NZeff, and A40 values in the LVI-positive group were higher than those in the LV-negative group, while the NIC, λHU, NZeff, and A40 values in the PNI--positive group were also higher than those in the PNI-negative group (all P values <0.05). However, A50, A60, A70, A80, A90, A100, A110, A120, A130, A140, An, Ae, and ΔA showed no significant differences between any 2 tumor grade groups, between the LVI-positive group and the LVI-negative group, or between the PNI-positive group and the PNI-negative group.
Table 3
Parameters | Tumor grade | F | P value | P# value | P* value | Pǂ value | ||
---|---|---|---|---|---|---|---|---|
Grade I (n=17) | Grade II (n=30) | Grade III (n=25) | ||||||
NIC | 0.19±0.03 | 0.22±0.03 | 0.26±0.03 | 26.351 | <0.001 | 0.001 | <0.001 | <0.001 |
λHU (HU/keV) | 1.64±0.17 | 1.81±0.30 | 2.05± 0.25 | 12.973 | <0.001 | 0.015 | <0.001 | 0.004 |
NZeff | 0.77±0.04 | 0.81±0.04 | 0.84±0.04 | 15.328 | <0.001 | 0.007 | <0.001 | 0.011 |
A40 (HU) | 173.90±19.10 | 192.07±22.43 | 208.41±21.91 | 13.119 | <0.001 | 0.021 | <0.001 | 0.020 |
A50 (HU) | 132.79±28.35 | 136.98±20.47 | 146.31±20.56 | 2.073 | 0.134 | |||
A60 (HU) | 115.90±23.64 | 120.02±17.18 | 124.93±19.95 | 1.089 | 0.342 | |||
A70 (HU) | 85.85±18.25 | 89.26±10.35 | 92.55±17.25 | 1.021 | 0.366 | |||
A80 (HU) | 67.63±17.03 | 71.66±9.07 | 72.25±17.13 | 0.600 | 0.552 | |||
A90 (HU) | 63.16±17.86 | 68.06±9.32 | 67.97±17.47 | 0.706 | 0.497 | |||
A100 (HU) | 61.32±17.95 | 65.99±9.40 | 65.83±17.52 | 0.629 | 0.536 | |||
A110 (HU) | 59.52±17.91 | 63.26±9.38 | 62.11±17.58 | 0.650 | 0.525 | |||
A120 (HU) | 59.33±18.20 | 63.07±9.81 | 62.02±18.21 | 0.668 | 0.513 | |||
A130 (HU) | 58.95±18.15 | 62.86±9.79 | 61.91±17.85 | 0.635 | 0.531 | |||
A140 (HU) | 58.47±18.03 | 62.35±9.92 | 61.12±17.93 | 0.655 | 0.525 | |||
An (HU) | 30.95±8.43 | 30.43±7.28 | 32.61±6.79 | 0.617 | 0.543 | |||
Ae (HU) | 62.12±7.13 | 62.81±8.16 | 63.24±5.73 | 0.123 | 0.884 | |||
ΔA (HU) | 31.17±6.79 | 32.37±6.05 | 30.62±7.61 | 0.474 | 0.624 |
Data are presented as the mean ± standard deviation. A 1-way analysis of variance with a Bonferroni post hoc test. F represents the statistical value. A P value <0.050 is statistically significant; P# (Grade I vs. Grade II), P* (Grade I vs. Grade III) and Pǂ (Grade II vs. Grade III) values <0.017 are statistically significant. CT, computed tomography; NIC, normalized iodine concentration in arterial phase; λHU, slope of the spectral Hounsfield unit curve in arterial phase; NZeff, normalized effective atomic number; VMI (+), noise-optimized virtual monoenergetic images; A40-140, attenuation value in enhanced VMIs (+) at 40–140 keV; An, attenuation value in nonenhanced polyenergetic images; Ae, attenuation value in enhanced polyenergetic images; ΔA = Ae − An.
Table 4
Parameters | Lymphovascular invasion | P value | Perineural invasion | P value | ||
---|---|---|---|---|---|---|
Negative (n=45) | Positive (n=27) | Negative (n=48) | Positive (n=24) | |||
NIC | 0.21±0.03 | 0.25±0.03 | <0.001 | 0.21±0.03 | 0.26±0.03 | <0.001 |
λHU (HU/keV) | 1.76±0.28 | 2.01± 0.27 | 0.001 | 1.80±0.28 | 2.00±0.30 | 0.019 |
NZeff | 0.79±0.04 | 0.83±0.04 | <0.001 | 0.79±0.04 | 0.84±0.04 | <0.001 |
A40 (HU) | 186.56±23.27 | 204.95±23.67 | 0.002 | 187.83±23.13 | 204.71±25.01 | 0.006 |
A50 (HU) | 135.87±22.51 | 144.84±22.89 | 0.111 | 135.82±22.33 | 146.05±23.00 | 0.074 |
A60 (HU) | 118.62±19.16 | 124.32±20.76 | 0.251 | 117.98±19.64 | 126.30±19.24 | 0.094 |
A70 (HU) | 88.08±13.35 | 92.12±17.45 | 0.306 | 87.70±13.84 | 93.39±16.83 | 0.131 |
A80 (HU) | 69.89±12.45 | 72.61±16.83 | 0.470 | 69.44±13.25 | 73.85±15.82 | 0.217 |
A90 (HU) | 65.91±13.09 | 68.46±17.09 | 0.509 | 65.42±13.75 | 69.77±16.23 | 0.238 |
A100 (HU) | 63.91±13.12 | 66.36±17.19 | 0.528 | 63.48±13.78 | 67.54±16.38 | 0.272 |
A110 (HU) | 61.14±13.09 | 64.66±17.20 | 0.516 | 61.71±13.76 | 65.84±16.37 | 0.264 |
A120 (HU) | 60.89±13.42 | 64.21±17.36 | 0.553 | 61.33±13.83 | 65.47±16.98 | 0.281 |
A130 (HU) | 60.43±13.57 | 64.01±17.93 | 0.563 | 61.01±13.91 | 64.01±16.73 | 0.276 |
A140 (HU) | 59.92±13.81 | 63.89±18.23 | 0.567 | 60.89±13.95 | 63.89±16.88 | 0.293 |
An (HU) | 31.03±7.52 | 31.77±7.21 | 0.681 | 30.40±7.48 | 33.13±6.91 | 0.139 |
Ae (HU) | 62.99±7.75 | 62.46±5.86 | 0.759 | 63.22±7.43 | 61.94±6.34 | 0.471 |
ΔA (HU) | 31.46±5.98 | 31.52±7.99 | 0.972 | 31.71±6.42 | 31.04±7.48 | 0.710 |
Data are presented as the mean ± standard deviation. Independent samples t-test. A P value <0.050 is statistically significant. CT, computed tomography; NIC, normalized iodine concentration; λHU, slope of the spectral Hounsfield unit curve; NZeff, normalized effective atomic number; VMI (+), noise-optimized virtual monoenergetic images; A40-140, attenuation value in enhanced VMIs (+) at 40–140 keV; An, attenuation value in nonenhanced polyenergetic images; Ae, attenuation value in enhanced polyenergetic images; ΔA = Ae − An.
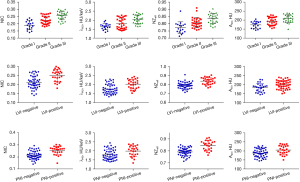
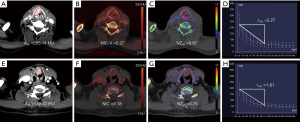
Diagnostic performance of the DECT parameters in predicting histopathological features
The AUC values of NIC were higher than those of λHU, NZeff, and A40 in distinguishing the grade III group from the grade I and II groups, the LVI-positive group from the LVI-negative group, and the PNI-positive group from the PNI-negative group. In the multivariable binary logistic regression analysis, compared to any single parameter, a combination of these 4 quantitative parameters obtained the highest AUC values for predicting tumor grade, LVI, and PNI (all P values <0.05; see Table 5 and Figure 5 and Figure S3).
Table 5
Parameters | Tumor grade (G I, G II vs. G III) | Lymphovascular invasion | Perineural invasion | |||||||||||
---|---|---|---|---|---|---|---|---|---|---|---|---|---|---|
AUC | Cutoff value | Sensitivity | Specificity | AUC | Cutoff value | Sensitivity | Specificity | AUC | Cutoff value | Sensitivity | Specificity | |||
NIC | 0.841 | 0.234 | 80.0% | 76.6% | 0.812 | 0.234 | 77.8% | 77.8% | 0.824 | 0.234 | 83.3% | 77.1% | ||
λHU (HU/keV) | 0.794 | 1.798 | 88.0% | 66.0% | 0.739 | 1.798 | 77.8% | 62.2% | 0.676 | 1.820 | 66.7% | 60.4% | ||
NZeff | 0.793 | 0.806 | 80.0% | 72.3% | 0.770 | 0.807 | 74.1% | 71.1% | 0.794 | 0.814 | 70.8% | 72.9% | ||
A40 (HU) | 0.769 | 195.850 | 72.0% | 70.2% | 0.715 | 190.240 | 77.8% | 57.8% | 0.690 | 195.850 | 62.5% | 64.6% | ||
Combined | 0.969 | −0.140 | 92.0% | 95.7% | 0.944 | −0.625 | 92.6% | 88.9% | 0.931 | −0.695 | 91.7% | 85.4% |
CT, computed tomography; NIC, normalized iodine concentration; λHU, slope of the spectral Hounsfield unit curve; NZeff, normalized effective atomic number; VMI (+), noise-optimized virtual monoenergetic images; A40, attenuation value in enhanced VMI (+) at 40 keV; Combined, combined NIC, λHU, NZeff and A40 by multivariate logistic regression analysis; AUC, area under the curve.
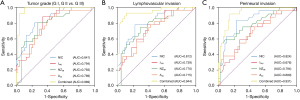
Discussion
Histopathological features, including tumor grade, LVI and PNI, are highly associated with the prognosis of patients with HNSCC (23,24). However, SDCT cannot be used to accurately assess these histopathologic features before biopsy (25,26). DECT is an emerging CT imaging technique that provides multiple quantitative parameters for characterizing the intrinsic characteristics of tumors in the head and neck (27,28). In our study, the quantitative parameters derived from pretreatment DECT successfully predicted tumor grade, LVI, and PNI in patients with HNSCC. Further, combining these quantitative parameters improved the predictive performance of the model.
The histological grade of HNSCC at pretreatment has long been considered to be important prognostic factor (29). Tumors with a high grade are characterized by more tumor angiogenesis and higher cellularity, which indicate a poor prognosis (2). In our study, we found that the NIC values of grade III HNSCC were higher than those of grades I and II HNSCC. These results are in line with research findings on colorectal cancer and lung adenocarcinoma (30,31). It may be that high-grade tumors with abundant tumor neovascularization result in increased tumor blood supply and increased iodine uptake (32). Additionally, immature neovascularization endothelial cells in high-grade tumors may result in more extravascular contrast media leakage (33). Consistent with the findings of a previous study (34), we found that the λHU values of grade III HNSCC were higher than those of grades I and II HNSCC. When the contrast is enhanced, λHU values might indicate the rate of attenuation changes in tumors, and higher λHU values may represent a higher percentage of iodine contrast media in tumors (17). Consequently, high-grade tumors with abundant tumor blood supply and higher iodine uptake will result in higher λHU values (35). The NZeff reflects the atomic number of the composition in the tumor tissue: the denser the tumor tissue, the higher the effective atomic number (19). We found that the NZeff values of grade III HNSCC were higher than those of grades I and II HNSCC. It may be that high-grade HNSCC with abundant and densely packed tumor cells leads to an increase in NZeff values (36).
LVI has been defined as cancer cells in peritumoral lymphatic vessels or small nonmuscularized blood vessels or both (37). It is associated with biologically aggressive diseases and the systemic dissemination of cancer cells (38). Previous studies have shown that pretreatment CT provides a noninvasive method for predicting LVI in gastric cancer and rectal cancer (26,39). A recent study showed that radiomic analyses based on pretreatment CT images could successfully predict LVI in patients with HNSCC (40). Based on the above studies, we used DECT to predict LVI in HNSCC and found that the NIC, λHU, and NZeff values of the LVI-positive group were higher than those of the LVI-negative group. There could be a number of reasons for these results. First, vascular invasion in LVI-positive tumors increases the extravascular leakage of contrast agents and leads to higher NIC and λHU values. Second, LVI demonstrates a positive correlation with tumor size and dissemination (41). Thus, tumor cells with relatively active proliferation in LVI-positive patients may increase the cell density of tumoral tissues, leading to an increase in Zeff values (4).
PNI is defined as the movement of cancer cells into the neural space, usually into small nerves (42). PNI has been shown to be associated with poor survival outcomes in patients with HNSCC and to indicate radical surgery and adjuvant radiochemotherapy (5). A previous study showed that heterogeneity features in primary tumors (as observed in pretreatment CT scans) can be used to predict PNI in patients with HNSCC (39). Another recent study showed that quantitative DECT could predict PNI in perihilar cholangiocarcinoma (43). On the basis of these studies, we applied quantitative parameters derived from DECT, which characterize the heterogeneity of tumor angiogenesis and cell proliferation, to predict PNI in patients with HNSCC. We found that the NIC values of the PNI-positive group were higher than those of the PNI-negative group. It may be that the increase of axon guidance molecules, including netrins, semaphorins, and ephrins, in PNI-positive patients promote the adhesion of tumor cells to peripheral nerves and tumor angiogenesis (44). An increase in tumor angiogenesis results in higher iodine uptake, which in turn increases NIC values. The NZeff values of the PNI-positive group were higher than those of the PNI-negative group. The tumor cells in the PNI-positive HNSCC have been shown to upregulate the genes that increase cell proliferation (45). Thus, increased cell proliferation may increase the density of tumoral tissue and result in higher NZeff values.
In our study, quantitative DECT parameters, including NIC, λHU, NZeff, and A40, successfully predicted the histopathological features, and a combination of these parameters improved the predictive performance of the model. However, all the attenuation values (i.e., An, Ae, and ΔA) in PEI failed to predict these histological features. This may be because attenuation values in PEI are affected by a variety of variables, such as convolution kernels, reconstruction, artifacts, beam hardening, scanner linearity, and patient geometric features (46). Additionally, ΔA represents the attenuation value difference between the unenhanced and enhanced phases. A split-level image of these 2 phases due to a swallowing motion will result in inaccurate ΔA. IC directly captures the distribution of iodine contrast agent in tumor tissues. NIC is a standardized IC, which reduces individual differences in perfusion and body weight and accurately reflects the blood perfusion status of tumors (47). This may explain why NIC can predict the pathological characteristics of HNSCC, but ΔA cannot.
HNSCCs are highly heterogeneous malignant tumors. Regardless of the pathological grade, the LVI status, and the PNI status, the CT features of HNSCCs may show heterogeneous density, ill-defined margins, the infiltration of adjacent structures, and high, medium, or low enhancement. Thus, the qualitative morphological analysis used in our work did not accurately characterize the histopathological heterogeneity of HNSCCs. Consistent with a recent study on nasopharyngeal malignancies (23), the morphological features failed to predict the histopathological characteristics in this study.
Our study had several limitations. First, while we found significant correlations between the DECT parameters and the histopathological features known to be related to poor clinical outcomes, we did not find that the DECT parameters translated directly into clinical outcome parameters. Second, our study was a single-center study with no external validation. Third, the HNSCC cases were selected from a heterogeneous group with malignancies in multiple head and neck areas from the oral cavity to the larynx. A study with a large sample size needs to be conducted to explore the ability of dual-energy CT to predict the histopathological features of tongue, oropharyngeal, hypopharyngeal, and laryngeal cancers. Fourth, the quantitative parameters were only obtained from axial slices of tumors but not from whole lesions. Finally, we did not compare the value of DECT in predicting the histopathological features of mucosal lesions and mass-like lesions due to the small sample size. However, we intend to analyze such lesions in a future prospective study with a large sample size.
In conclusion, quantitative parameters derived from pretreatment DECT, including NIC, λHU NZeff, and A40, may serve as imaging markers for predicting the histopathologic characteristics of HNSCC, and a combination of all 3 quantitative parameters can improve the predictive performance of the model.
Acknowledgments
Funding: This study received funding from the National Natural Science Foundation of China (No. 82071883), the Combination Projects of Medicine and Engineering of the Fundamental Research Funds for the Central Universities in 2019 (project No. 2019CDYGYB008), the Chongqing Key Medical Research Project of a Combination of Science and Medicine (No. 2019ZDXM007), the 2019 SKY Imaging Research Fund of the Chinese International Medical Foundation (Project No. Z-2014-07-1912-10), the Chongqing Medical Research project of a Combination of Science and Medicine (No. 2021MSXM077), and the Chongqing Medical Research Project of a Combination of Science and Medicine (No. 2021MSXM035).
Footnote
Reporting Checklist: The authors have completed the STARD reporting checklist. Available at https://dx.doi.org/10.21037/qims-21-650
Conflicts of Interest: All authors have completed the ICMJE uniform disclosure form (available at https://dx.doi.org/10.21037/qims-21-650). The authors have no conflicts of interest to declare.
Ethical Statement: The authors are accountable for all aspects of the work in ensuring that questions related to the accuracy or integrity of any part of the work are appropriately investigated and resolved. The study was conducted in accordance with the Declaration of Helsinki (as revised in 2013). The study was approved by the ethics committee of the Chongqing University Cancer Hospital, and written informed consent was waived.
Open Access Statement: This is an Open Access article distributed in accordance with the Creative Commons Attribution-NonCommercial-NoDerivs 4.0 International License (CC BY-NC-ND 4.0), which permits the non-commercial replication and distribution of the article with the strict proviso that no changes or edits are made and the original work is properly cited (including links to both the formal publication through the relevant DOI and the license). See: https://creativecommons.org/licenses/by-nc-nd/4.0/.
References
- Bray F, Ferlay J, Soerjomataram I, Siegel RL, Torre LA, Jemal A. Global cancer statistics 2018: GLOBOCAN estimates of incidence and mortality worldwide for 36 cancers in 185 countries. CA Cancer J Clin 2018;68:394-424. [Crossref] [PubMed]
- Wolf GT, Winter W, Bellile E, Nguyen A, Donnelly CR, McHugh JB, Thomas D, Amlani L, Rozek L, Lei YLHead and Neck SPORE Program. Histologic pattern of invasion and epithelial-mesenchymal phenotype predict prognosis in squamous carcinoma of the head and neck. Oral Oncol 2018;87:29-35. [Crossref] [PubMed]
- Taghavi N, Yazdi I. Prognostic factors of survival rate in oral squamous cell carcinoma: clinical, histologic, genetic and molecular concepts. Arch Iran Med 2015;18:314-9. [PubMed]
- Saito Y, Omura G, Yasuhara K, Rikitake R, Akashi K, Fukuoka O, Yoshida M, Ando M, Asakage T, Yamasoba T. Prognostic value of lymphovascular invasion of the primary tumor in hypopharyngeal carcinoma after total laryngopharyngectomy. Head Neck 2017;39:1535-43. [Crossref] [PubMed]
- Jardim JF, Francisco AL, Gondak R, Damascena A, Kowalski LP. Prognostic impact of perineural invasion and lymphovascular invasion in advanced stage oral squamous cell carcinoma. Int J Oral Maxillofac Surg 2015;44:23-8. [Crossref] [PubMed]
- Park J, Megow A, Swalling A, Hodge JC, Foreman A, Boase S, Valentine R, Krishnan S, Ooi EH. Prognosis of oral squamous cell carcinoma with perineural invasion: A comparative study of classification types. Clin Otolaryngol 2020;45:99-105. [Crossref] [PubMed]
- Zhu J, Zhou R, Wang Y, Yu M. Perineural invasion as a prognostic factor in head and neck squamous cell carcinoma: a systematic review and meta-analysis. Acta Otolaryngol 2019;139:1038-43. [Crossref] [PubMed]
- Hsu PK, Chien LI, Wang LC, Chou TYTaipei Veterans General Hospital Esophageal Cancer Panel. Lymphovascular invasion and extracapsular invasion are risk factors for distant recurrence after preoperative chemoradiotherapy and oesophagectomy in patients with oesophageal squamous cell carcinoma. Eur J Cardiothorac Surg 2017;51:1188-94. [Crossref] [PubMed]
- Tang JC, Kim SL, Stratman EJ. Measuring the Depth of Perineural Invasion in Cutaneous Squamous Cell Carcinoma: Implications on Biopsy Technique. Dermatol Surg 2018;44:1170-3. [Crossref] [PubMed]
- Matoba M, Tsuji H, Shimode Y, Nagata H, Tonami H. Diagnostic Performance of Adaptive 4D Volume Perfusion CT for Detecting Metastatic Cervical Lymph Nodes in Head and Neck Squamous Cell Carcinoma. AJR Am J Roentgenol 2018;211:1106-11. [Crossref] [PubMed]
- Cho JK, Ow TJ, Lee AY, Smith RV, Schlecht NF, Schiff BA, Tassler AB, Lin J, Moadel RM, Valdivia A, Abraham T, Gulko E, Neimark M, Ustun B, Bello JA, Shifteh K. Preoperative 18F-FDG-PET/CT vs Contrast-Enhanced CT to Identify Regional Nodal Metastasis among Patients with Head and Neck Squamous Cell Carcinoma. Otolaryngol Head Neck Surg 2017;157:439-47. [Crossref] [PubMed]
- Park JT, Roh JL, Kim JS, Lee JH, Cho KJ, Choi SH, Nam SY, Kim SY. (18)F FDG PET/CT versus CT/MR Imaging and the Prognostic Value of Contralateral Neck Metastases in Patients with Head and Neck Squamous Cell Carcinoma. Radiology 2016;279:481-91. [Crossref] [PubMed]
- McCollough CH, Leng S, Yu L, Fletcher JG. Dual- and Multi-Energy CT: Principles, Technical Approaches, and Clinical Applications. Radiology 2015;276:637-53. [Crossref] [PubMed]
- Mileto A, Marin D, Alfaro-Cordoba M, Ramirez-Giraldo JC, Eusemann CD, Scribano E, Blandino A, Mazziotti S, Ascenti G. Iodine quantification to distinguish clear cell from papillary renal cell carcinoma at dual-energy multidetector CT: a multireader diagnostic performance study. Radiology 2014;273:813-20. [Crossref] [PubMed]
- Liu X, Ouyang D, Li H, Zhang R, Lv Y, Yang A, Xie C. Papillary thyroid cancer: dual-energy spectral CT quantitative parameters for preoperative diagnosis of metastasis to the cervical lymph nodes. Radiology 2015;275:167-76. [Crossref] [PubMed]
- Sun XS, Liang YJ, Li XY, Liu SL, Chen QY, Tang LQ, Mai HQ. Palliative chemotherapy with or without anti-EGFR therapy for de novo metastatic nasopharyngeal carcinoma: a propensity score-matching study. Drug Des Devel Ther 2019;13:3207-16. [Crossref] [PubMed]
- Wu J, Lv Y, Wang N, Zhao Y, Zhang P, Liu Y, Chen A, Li J, Li X, Guo Y, Wu T, Liu A. The value of single-source dual-energy CT imaging for discriminating microsatellite instability from microsatellite stability human colorectal cancer. Eur Radiol 2019;29:3782-90. [Crossref] [PubMed]
- Wang X, Liu D, Zeng X, Jiang S, Li L, Yu T, Zhang J. Dual-energy CT quantitative parameters for the differentiation of benign from malignant lesions and the prediction of histopathological and molecular subtypes in breast cancer. Quant Imaging Med Surg 2021;11:1946-57. [Crossref] [PubMed]
- Lam S, Gupta R, Kelly H, Curtin HD, Forghani R. Multiparametric Evaluation of Head and Neck Squamous Cell Carcinoma Using a Single-Source Dual-Energy CT with Fast kVp Switching: State of the Art. Cancers (Basel) 2015;7:2201-16. [Crossref] [PubMed]
- Forghani R, Kelly HR, Curtin HD. Applications of Dual-Energy Computed Tomography for the Evaluation of Head and Neck Squamous Cell Carcinoma. Neuroimaging Clin N Am 2017;27:445-59. [Crossref] [PubMed]
- May MS, Bruegel J, Brand M, Wiesmueller M, Krauss B, Allmendinger T, Uder M, Wuest W. Computed Tomography of the Head and Neck Region for Tumor Staging-Comparison of Dual-Source, Dual-Energy and Low-Kilovolt, Single-Energy Acquisitions. Invest Radiol 2017;52:522-8. [Crossref] [PubMed]
- Bahig H, Lapointe A, Bedwani S, de Guise J, Lambert L, Filion E, Roberge D, Létourneau-Guillon L, Blais D, Ng SP, Nguyen-Tan PF. Dual-energy computed tomography for prediction of loco-regional recurrence after radiotherapy in larynx and hypopharynx squamous cell carcinoma. Eur J Radiol 2019;110:1-6. [Crossref] [PubMed]
- Shen H, Yuan X, Liu D, Huang Y, Wang Y, Jiang S, Zhang J. Multiparametric dual-energy CT for distinguishing nasopharyngeal carcinoma from nasopharyngeal lymphoma. Eur J Radiol 2021;136:109532. [Crossref] [PubMed]
- Sinha P, Mehrad M, Chernock RD, Lewis JS Jr, El-Mofty SK, Wu N, Nussenbaum B, Haughey BH. Histologic and systemic prognosticators for local control and survival in margin-negative transoral laser microsurgery treated oral cavity squamous cell carcinoma. Head Neck 2015;37:52-63. [Crossref] [PubMed]
- Tsuchiya N, Doai M, Usuda K, Uramoto H, Tonami H. Non-small cell lung cancer: Whole-lesion histogram analysis of the apparent diffusion coefficient for assessment of tumor grade, lymphovascular invasion and pleural invasion. PLoS One 2017;12:e0172433. [Crossref] [PubMed]
- Ma Z, Liang C, Huang Y, He L, Liang C, Chen X, Huang X, Xiong Y, Liu Z. Can lymphovascular invasion be predicted by preoperative multiphasic dynamic CT in patients with advanced gastric cancer? Eur Radiol 2017;27:3383-91. [Crossref] [PubMed]
- Seidler M, Forghani B, Reinhold C, Pérez-Lara A, Romero-Sanchez G, Muthukrishnan N, Wichmann JL, Melki G, Yu E, Forghani R, Dual-Energy CT. Texture Analysis With Machine Learning for the Evaluation and Characterization of Cervical Lymphadenopathy. Comput Struct Biotechnol J 2019;17:1009-15. [Crossref] [PubMed]
- Forghani R, Chatterjee A, Reinhold C, Pérez-Lara A, Romero-Sanchez G, Ueno Y, Bayat M, Alexander JWM, Kadi L, Chankowsky J, Seuntjens J, Forghani B. Head and neck squamous cell carcinoma: prediction of cervical lymph node metastasis by dual-energy CT texture analysis with machine learning. Eur Radiol 2019;29:6172-81. [Crossref] [PubMed]
- Ren J, Qi M, Yuan Y, Tao X. Radiomics of apparent diffusion coefficient maps to predict histologic grade in squamous cell carcinoma of the oral tongue and floor of mouth: a preliminary study. Acta Radiol 2021;62:453-61. [Crossref] [PubMed]
- Jiang H, Li X. Correlation of dual-source computed tomography/dual-energy imaging with pathological grading of lung adenocarcinoma and its clinical value. Pak J Med Sci 2017;33:1429-33. [Crossref] [PubMed]
- Gong HX, Zhang KB, Wu LM, Baigorri BF, Yin Y, Geng XC, Xu JR, Zhu J. Dual Energy Spectral CT Imaging for Colorectal Cancer Grading: A Preliminary Study. PLoS One 2016;11:e0147756. [Crossref] [PubMed]
- Kaichi Y, Tatsugami F, Nakamura Y, Baba Y, Iida M, Higaki T, Kiguchi M, Tsushima S, Yamasaki F, Amatya VJ, Takeshima Y, Kurisu K, Awai K. Improved differentiation between high- and low-grade gliomas by combining dual-energy CT analysis and perfusion CT. Medicine (Baltimore) 2018;97:e11670. [Crossref] [PubMed]
- Woo S, Lee JM, Yoon JH, Joo I, Han JK, Choi BI. Intravoxel incoherent motion diffusion-weighted MR imaging of hepatocellular carcinoma: correlation with enhancement degree and histologic grade. Radiology 2014;270:758-67. [Crossref] [PubMed]
- Zhang C, Wang N, Su X, Li K, Yu D, Ouyang A. FORCE dual-energy CT in pathological grading of clear cell renal cell carcinoma. Oncol Lett 2019;18:6405-12. [Crossref] [PubMed]
- Chang N, Cui L, Luo Y, Chang Z, Yu B, Liu Z. Development and multicenter validation of a CT-based radiomics signature for discriminating histological grades of pancreatic ductal adenocarcinoma. Quant Imaging Med Surg 2020;10:692-702. [Crossref] [PubMed]
- Wu W, Ye J, Wang Q, Luo J, Xu S. CT-Based Radiomics Signature for the Preoperative Discrimination Between Head and Neck Squamous Cell Carcinoma Grades. Front Oncol 2019;9:821. [Crossref] [PubMed]
- Nougaret S, Reinhold C, Alsharif SS, Addley H, Arceneau J, Molinari N, Guiu B, Sala E. Endometrial Cancer: Combined MR Volumetry and Diffusion-weighted Imaging for Assessment of Myometrial and Lymphovascular Invasion and Tumor Grade. Radiology 2015;276:797-808. [Crossref] [PubMed]
- Martins-Andrade B, Dos Santos Costa SF, Sant'ana MSP, Altemani A, Vargas PA, Fregnani ER, Abreu LG, Batista AC, Fonseca FP. Prognostic importance of the lymphovascular invasion in head and neck adenoid cystic carcinoma: A systematic review and meta-analysis. Oral Oncol 2019;93:52-8. [Crossref] [PubMed]
- Wu CC, Lee RC, Chang CY. Prediction of lymphovascular invasion in rectal cancer by preoperative CT. AJR Am J Roentgenol 2013;201:985-92. [Crossref] [PubMed]
- Mukherjee P, Cintra M, Huang C, Zhou M, Zhu S, Colevas AD, Fischbein N, Gevaert O. CT-based Radiomic Signatures for Predicting Histopathologic Features in Head and Neck Squamous Cell Carcinoma. Radiol Imaging Cancer 2020;2:e190039. [Crossref] [PubMed]
- Fives C, Feeley L, O'Leary G, Sheahan P. Importance of lymphovascular invasion and invasive front on survival in floor of mouth cancer. Head Neck 2016;38:E1528-34. [Crossref] [PubMed]
- Binmadi NO, Basile JR. Perineural invasion in oral squamous cell carcinoma: a discussion of significance and review of the literature. Oral Oncol 2011;47:1005-10. [Crossref] [PubMed]
- Tanaka H, Igami T, Shimoyama Y, Ebata T, Yokoyama Y, Mori K, Nagino M. New method for the assessment of perineural invasion from perihilar cholangiocarcinoma. Surg Today 2021;51:136-43. [Crossref] [PubMed]
- Saidak Z, Lailler C, Clatot F, Galmiche A. Perineural invasion in head and neck squamous cell carcinoma: background, mechanisms, and prognostic implications. Curr Opin Otolaryngol Head Neck Surg 2020;28:90-5. [Crossref] [PubMed]
- Badger D, Aygun N. Imaging of Perineural Spread in Head and Neck Cancer. Radiol Clin North Am 2017;55:139-49. [Crossref] [PubMed]
- Birnbaum BA, Hindman N, Lee J, Babb JS. Multi-detector row CT attenuation measurements: assessment of intra- and interscanner variability with an anthropomorphic body CT phantom. Radiology 2007;242:109-19. [Crossref] [PubMed]
- Zopfs D, Reimer RP, Sonnabend K, Rinneburger M, Hentschke CM, Persigehl T, Lennartz S, Große Hokamp N. Intraindividual Consistency of Iodine Concentration in Dual-Energy Computed Tomography of the Chest and Abdomen. Invest Radiol 2021;56:181-7. [Crossref] [PubMed]