Identification of histological features of endometrioid adenocarcinoma based on amide proton transfer-weighted imaging and multimodel diffusion-weighted imaging
Introduction
Endometrial carcinoma (EC) is one of the most common malignancies of the female reproductive system (1), and its incidence is increasing rapidly in China (2). The treatment response and prognosis of EC are closely related to histologic subtype and grade, the depth of myometrial invasion, and lymph node metastasis (3,4). Among these features, the histological grade is a particularly strong predictor of lymph node metastasis and overall survival rate (5). The Ki-67 index is a reliable identifier of aggressive tumors, can reflect the extent of proliferative activity (6), and has been used to predict the clinical recurrence and outcomes of EC (7). Approximately 80–90% of EC cases are endometrial adenocarcinoma (EA), with the remaining cases made up of other histologic subtypes, such as clear cell or serous carcinoma (1,2). Therefore, accurate assessment of the histologic grade and Ki-67 index of EA is meaningful for patients.
Magnetic resonance imaging (MRI) has been widely used to evaluate the myometrial invasion, lymph node metastasis, and distant metastasis of EA (4,8). However, conventional MRI sequences reflect only the morphological features of lesions, which makes it difficult to pre-evaluate the histological grade and Ki-67 index of EA. Diffusion-weighted imaging (DWI), a noninvasive technique that is sensitive to water molecular diffusion in biological tissue, has been investigated for use in evaluating the histological characteristics of EA (9,10). The biexponential intravoxel incoherent motion (IVIM) DWI model, first proposed by Le Bihan et al. (11), is an effective MRI sequence for the separation of Brownian motion of water molecules and capillary microcirculation perfusion. The stretched exponential DWI model, introduced by Bennett et al. (12), is used to describe the heterogeneity of intravoxel diffusion rates and the distributed diffusion effect. Several previous studies have suggested that the above 2 models can provide more accurate and richer information on tissue properties than can the monoexponential DWI model (13,14). Amide proton transfer-weighted imaging (APTWI) is an MR molecular imaging technology proposed by Zhou et al. (15,16). Based on a chemical exchange between amide protons and water protons, APTWI can achieve a noninvasive quantitative assessment of mobile protein and peptide concentrations in tissue without the use of contrast agents. Previous studies have shown the utility of APTWI for predicting the histological features of some tumors, including gliomas and lung cancers (17,18). To our knowledge, only a few small-sample studies have reported the comparative application of a monoexponential DWI model and APTWI in the histological grade of EA (19). Moreover, these studies did not fully analyze whether the combination of related parameters could provide a better predictive performance, nor did they discuss the correlation between each parameter and the Ki-67 index.
The purpose of this study was to investigate whether parameters derived from APTWI and multimodel DWI could predict the histological grade of EA and to analyze the correlation between each parameter and the Ki-67 index, with the goal of generating new approaches for assessing EA.
Methods
Participants
The study was conducted in accordance with the Declaration of Helsinki (as revised in 2013). This prospective study complied with ethical committee standards and was approved by the ethics committee of the First Affiliated Hospital of Xinxiang Medical University (No. 2018067). Informed consent was taken from all individual participants. A total of 138 consecutive female patients who had undergone computed tomography (CT) or ultrasound (US) and who were suspected of having EC were included for MRI. The exclusion criteria were as follows: (I) patients who had not completed all imaging sequences due to claustrophobia or other physical symptoms (n=6); (II) patients whose maximum area of the multimodel DWI or APTWI axial plane was less than 50 pixels (392 mm2), considering the effect of noise (n=10); (III) patients whose APTWI or multimodel DWI images were seriously hampered by motion artifacts, ghosting artifacts, etc. (n=8); (IV) patients who had received chemotherapy, radiotherapy, or surgery before scanning (n=7); (V) patients with non-EA histological results (n=9); and 6) patients with unclear histological or immunohistochemical results (n=8). Ultimately, a total of 90 subjects were enrolled in the study (Figure 1).
MRI protocol
A whole-body 3.0-T MRI system (Discovery MR750, GE Healthcare, Chicago, IL, USA) and a 16-channel phased-array body coil were used in this study. All patients were placed in the supine position, feet first, and with a partially full bladder. Hyoscine butylbromide (40 mg, Buscopan; Boehringer, Ingelheim, Germany) was administered intramuscularly before the examination to reduce bowel motion. First, 2-dimensional oblique axial (perpendicular to the long axis of the uterus) conventional MRI was performed, which included T1-weighted imaging (T1WI) and T2-weighted imaging (T2WI). Next, the oblique axial multimodel DWI and APTWI sequences were scanned through all slices on which a tumor appeared to be present as selected by radiologists (DMH, with 20 years of experience) on T1WI and T2WI. The monoexponential model was performed using 2 b values (0 and 1,000 s/mm2). The biexponential and stretched models were performed using 10 b values (0, 25, 50, 75, 100, 150, 200, 400, 800, and 1,000 s/mm2) (11,12,20). APTWI was conducted using 4 saturation pulses (Tsat) with a Fermi pulse, a duration of 0.5 s, and a saturation power level of 2.0 µT (19,21,22). A total of 52 frequencies were used for the APTWI and z-spectrum scans for signal normalization, including 49 offsets ranging from –600 to +600 Hz with an interval of 25 Hz and a frequency of 5,000 Hz. The water saturation shift reference (WASSR) was applied for B0 correction, which uses a densely sampled frequency list around 0 ppm, covering –250 to 250 Hz with an interval of 25 Hz. The saturation power and length were constrained to minimize the spillover effects. The power and duration of saturation pulse were 0.5 µT and 0.5 s, respectively. Finally, the contrast-enhanced sequence with intravenous injection (0.1 mL/kg, 2.0 mL/s) of gadopentetate dimeglumine (Gd-DTPA, Bayer Pharmaceutical, Berlin, Germany) using an automatic injector. The protocol details are provided in Table 1.
Table 1
Parameters | T1WI | T2WI | Multimodel-DWI | APTWI | Contrast-enhancedI |
---|---|---|---|---|---|
Orientation/sequence | 2D – Axial – FSE | 2D – Axial – FSE | 2D – Axial – SS – EPI | 2D – Axial – EPI | 3D – Axial – LAVA |
TE/TR (ms) | 8/605 | 109/5,455 | 68.7/2,000 | 12/3,000 | 2.1/4.2 |
Field of view (cm2) | 36×36 | 36×36 | 36×36 | 36×36 | 36×36 |
Matrix | 320×224 | 320×224 | 128×128 | 128×128 | 320×320 |
Bandwidth (kHz) | 62.50 | 83.33 | 250 | 250 | 83.33 |
Slice number | 20 | 20 | Based on lesion size | 1 | 80 |
Thickness (mm) | 5 | 5 | 5 | 5 | 1 |
Number of excitations | 1 | 1 | 1, 1, 1, 1, 1, 1, 2, 4, 4, 6 | 1 | 0.7 |
Fat suppression | – | STIR | STIR | STIR | FLEX |
b-values (s/mm2) | – | – | 0, 25, 50, 75, 100, 150, 200, 400, 800, 1,000 | – | – |
Respiratory compensation | No | No | No | No | Breath holding |
Time | 1 min 57 s | 1 min 33 s | 2 min 24 s | 2 min 36 s | 0:09 (each phase) |
T1WI, T1-weighted imaging; T2WI, T2-weighted imaging; Multimodel-DWI, multimodel diffusion-weighted imaging; APTWI, amide proton transfer–weighted imaging; FSE, fast spin echo; TE/TR, echo time/repetition time; SS-EPI, single-shot echo planar imaging; LAVA, liver acquisition with volume Assessment; STIR, short-inversion time recovery; FLEX, flexible.
Postprocessing and analysis
All MR images were transformed to the Advantage Workstation (version 4.6, GE Healthcare) and independently analyzed by 2 radiologists (NM and FF F, with 6 and 20 years of experience in interpreting MR images of EC, respectively) who were blinded to the clinical and histological data as well as each other’s results. The multimodel DWI and APTWI protocols were postprocessed using Functool’s MADC software and APT software, respectively. The monoexponential model parameters were obtained from the following equation (11):
where b is the diffusion sensitizing factor, and S0 and Sb represent the signal intensity (SI) under different b values (0 s/mm2 or other values), respectively. The biexponential model parameters were calculated using the following equation (11):
where D (×10–3 mm2/s) indicates the pure diffusion coefficient, D* (×10–3 mm2/s) indicates the pseudo diffusion coefficient, and f (%) indicates the perfusion fraction. The stretched exponential model parameters were calculated using the following equation (12):
where DDC (×10–3 mm2/s) represents the mean intravoxel diffusion rate, and α (arbitrary units, ranging from 0 to 1) indicates the intravoxel water molecular diffusion heterogeneity. The APTWI parameter was calculated using the equation (15,16):
where Ssat and S0 indicate the SIs obtained with and without selective saturation, respectively, and the magnetization transfer ratio asymmetry [(MTRasym (3.5 ppm)] represents the magnetization transfer ratio asymmetry at 3.5 ppm downfield from the water signal. The interest regions (ROIs) were plotted as follows: First, the T1WI, T2WI, and contrast-enhanced images were used as a reference to delineate the solid component of the tumor on the DWI images. The pseudo–color maps of various parameters were then merged with the same layer of DWI images to outline the ROIs, which we aimed to contain as much solid area of the tumor as possible while avoiding necrosis, hemorrhage, cystic degeneration, and blood vessels. Third, lesion information (e.g., parameter values, size, etc.) for each slice was automatically computed using the transferred ROIs, and the average value of the related parameter on all slices was the final value of each lesion parameter.
Histopathologic analysis
All lesion specimens were obtained by biopsy or surgery within 1 week of the MRI scan. A pathologist with 8 years of experience analyzed all surgically resected specimens of each patient. The histological grade was determined by hematoxylin and eosin (HE) staining. The specimens were classified into low- (grade 1 and 2) and high-grade (grade 3) groups based on the International Federation of Gynecology and Obstetrics (FIGO) grading system (23). For the Ki-67 index, a murine Ki-67 monoclonal antibody (M3G4; Celnovte, Rockville, MD, USA) was used to stain tissue fragments, and then the positive cells were counted at 400× magnification in 10 hotspots (areas with more positive cells).
Statistical analysis
Statistical analyses were performed using MedCalc (Version 15.0; MedCalc Software, Ostend, Belgium) and SPSS (Version 23.0; IBM Corp., Armonk, NY, USA). The intraclass correlation coefficient (ICC) was used to evaluate agreement between 2 readers (r≥0.75, excellent; 0.60≤r<0.75, good; 0.40≤r<0.60, fair; and r<0.40, poor) (19). The Shapiro-Wilk test was applied to evaluate whether the data of each group were normally distributed. For normally distributed data, we used the independent samples t test for comparisons, and for nonnormally distributed data, we used the Mann-Whitney U test for comparisons. Analysis of variance (ANOVA) was used to compare the differences in each parameter among the 3 grades. A receiver operating characteristic (ROC) curve was drawn to describe the diagnostic efficacy of each parameter, and the DeLong test was used to determine whether the area under the ROC curve (AUC) of each parameter was different. Logistic regression analysis was used to identify independent factors that could distinguish high- from low-grade EA. Spearman rank and Pearson correlations were applied to depict the correlation of each parameter with histological grade and the Ki-67 index, respectively (r≥0.75, good; 0.50≤r<0.75, moderate; 0.25≤r<0.50, mild; and r<0.25, little or none) (24). A P value<0.05 was considered statistically significant.
Results
Patients characteristics
The clinical-pathological characteristics of each patient are shown in Table 2.
Table 2
Variable | Data |
---|---|
Age (mean ± SD) (years) | 57.46±7.35 |
Maximum diameter (mean ± SD) (mm) | 51.19±13.45 |
FIGO stage, n (%) | |
IA | 31 (34.44) |
IB | 19 (21.11) |
II | 14 (15.56) |
IIIA | 6 (6.67) |
IIIB | 6 (6.67) |
IIIC1 | 3 (3.33) |
IIIC2 | 3 (3.33) |
IVA | 3 (3.33) |
IVB | 5 (5.56) |
Histologic grade, n (%) | |
Grade 1 | 40 (44.44) |
Grade 2 | 26 (28.89) |
Grade 3 | 24 (26.67) |
Ki-67 index, n (%) | |
≤10% (−) | 7 (7.78) |
11%–25% (+) | 19 (21.11) |
26–50% (+ +) | 36 (40.00) |
≥51% (+ + +) | 28 (31.11) |
FIGO, Federation International of Gynecology and Obstetrics.
Consistency test
The data measured by the 2 readers showed good consistency. The ICC was 0.868 for apparent diffusion coefficient (ADC), 0.854 for D, 0.762 for D*, 0.837 for f, 0.834 for DDC, 0.809 for α, and 0.772 for MTRasym (3.5 ppm). The mean results of the 2 readers for each parameter were used for quantitative statistical analyses.
Parameter comparison
MTRasym (3.5 ppm), f, and α were higher, while ADC, D, and DDC were lower in the high-grade group than in the low-grade group (P<0.001, P<0.001, P<0.001, P=0.001, P<0.001, and P=0.004, respectively; Figures 2,3). The difference in D* between the 2 groups was not significant (P=0.479). Detailed comparisons of the different parameters between the 3 EA grades are shown in Table 3.
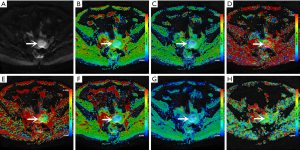
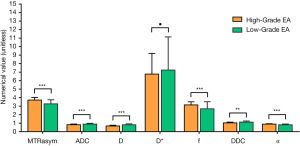
Table 3
Parameters | MTRasym (3.5 ppm) (%) | ADC (×10−3 mm2/s) | D (×10−3 mm2/s) | D* (×10−3 mm2/s) | f (%) | DDC (×10−3 mm2/s) | α |
---|---|---|---|---|---|---|---|
G1 (n=40) | 3.13±0.47 | 0.92±0.09 | 0.83±0.09 | 73.89±43.81 | 2.61±0.86 | 1.17±0.10 | 0.77±0.07 |
G2 (n=26) | 3.50±0.41 | 0.86±0.12 | 0.77±0.13 | 70.15±30.87 | 2.83±0.79 | 1.06±0.15 | 0.88±0.06 |
G3 (n=24) | 3.72±0.31 | 0.82±0.08 | 0.67±0.09 | 67.69±24.39 | 3.15±0.36 | 1.04±0.09 | 0.89±0.05 |
P value | <0.001a | <0.001d | <0.001a | 0.770b | 0.002b | <0.001a | <0.001b |
P value (G1 vs. G2) | 0.001c | 0.131d | 0.043c | 0.968d | 0.638d | <0.001c | 0.056d |
P value (G2 vs. G3) | 0.069c | 0.297d | 0.001c | 0.985d | 0.186d | 0.526c | 0.105d |
P value (G3 vs. G1) | <0.001c | <0.001d | <0.001c | 0.849d | 0.003d | <0.001c | <0.001d |
High-grade (G3) | 3.72±0.31 | 0.82±0.08 | 0.67±0.09 | 67.69±24.39 | 3.15±0.36 | 1.04±0.09 | 0.89±0.05 |
Low-grade (G1 + G2) | 3.27±0.48 | 0.89±0.10 | 0.81±0.11 | 72.42±39.00 | 2.69±0.83 | 1.13±0.13 | 0.81±0.09 |
t/z value | −4.190 | 3.345 | 5.378 | 0.683 | −3.622 | 2.994 | −4.001 |
P value | <0.001e | 0.001e | <0.001e | 0.497e | <0.001e | 0.004e | <0.001f |
a, Comparison by ANOVA test; b, comparison by Welch test; c, comparison by L-S-D test; d, comparison by Dunnett T3 test; e, comparison by independent t test; f, comparison by Mann–Whitney U test. MTRasym (3.5 ppm), magnetization transfer ratio asymmetry; ADC, apparent diffusion coefficient; D: diffusion coefficient; D*, pseudo-diffusion coefficient; f, perfusion fraction; DDC, distributed diffusion coefficient; α, water molecular diffusion heterogeneity index; G 1, 2, and 3 = grade 1, 2, and 3. The bold typeface indicates statistically significant.
Regression analyses
Age, FIGO stage, tumor size, and APTWI and multimodel DW–derived parameters were all included. Although univariate analysis showed that FIGO stage, MTRasym (3.5 ppm), ADC, D, F, DDC, and α were all favorable for EA histological grade (P<0.001, P<0.001, P=0.003, P<0.001, P=0.017, P=0.008, and P<0.001, respectively), multivariate analysis revealed that only MTRasym (3.5 ppm) and D were independent predictors for the histological grade of EA (P=0.022 and P=0.012, respectively; Table 4).
Table 4
Parameters | Univariate analyses | Multivariate analyses | |||
---|---|---|---|---|---|
OR (95% CI) | P value | OR (95% CI) | P value | ||
Age (year) | 1.181* (0.736–1.896) | 0.491 | – | – | |
Tumor size (mm) | 1.521* (0.949–2.436) | 0.081 | 1.553* (0.763–3.161) | 0.255 | |
FIGO stage | 1.560 (1.247–1.950) | <0.001 | 1.113 (0.825–1.502) | 0.482 | |
MTRasym (3.5 ppm) (%) | 3.083* (1.663–5.716) | <0.001 | 2.509* (1.052v5.985) | 0.038# | |
ADC (×10−3 mm2/s) | 0.394* (0.214–0.725) | 0.003 | 1.258* (0.451–3.513) | 0.661 | |
D (×10−3 mm2/s) | 0.178* (0.078–0.406) | <0.001 | 0.152* (0.034–0.677) | 0.013# | |
D* (×10−3 mm2/s) | 0.872* (0.538–1.412) | 0.576 | – | – | |
F (%) | 1.985* (1.130–3.485) | 0.017 | 1.789* (0.783–4.087) | 0.168 | |
DDC (×10−3 mm2/s) | 0.481* (0.281–0.823) | 0.008 | 1.884* (0.688–4.871) | 0.284 | |
α | 3.631* (1.775–7.428) | <0.001 | 1.830* (0.754–5.241) | 0.226 |
*, OR for per 1 standard deviation. #, statistically significant. All factors with P<0.1 in univariate analyses were included in multivariate regression analyses. MTRasym (3.5 ppm), magnetization transfer ratio asymmetry; ADC, apparent diffusion coefficient; D, diffusion coefficient; D*, pseudo-diffusion coefficient; f, perfusion fraction; DDC, distributed diffusion coefficient; α, water molecular diffusion heterogeneity index; OR, odds ratio; CI, confidence interval.
Diagnostic performance
For the diagnosis of high- and low-grade EA, comparison of the combination of MTRasym (3.5 ppm) and D and each parameter revealed the following: AUC MTRasym (3.5 ppm) + D > AUC D > AUC MTRasym (3.5 ppm) > AUC α > AUC DDC > AUC ADC > AUC f. Among these, the differences between AUC MTRasym (3.5 ppm) + D and AUC MTRasym (3.5 ppm), AUC α, AUC DDC, AUC ADC, and AUC f were significant (Z=2.512, Z=2.433, Z=3.052, Z= 3.818, and Z=3.229, respectively; P=0.012, P=0.015, P=0.002, P=0.001, and P=0.001, respectively); meanwhile, the difference between AUC MTRasym (3.5 ppm) + D and AUC D was not significant (Z=1.753; P=0.079). With respect to the comparison between various parameters, the only significant difference was between AUC D and AUC ADC (Z=2.499; P=0.012; Table 5, Figure 4).
Table 5
Parameters | AUC (95%CI) | P value | Cutoff | Sensitivity | Specificity |
---|---|---|---|---|---|
MTRasym (3.5 ppm) (%) | 0.782 (0.683–0.862) | <0.001 | 3.340 | 91.67% (83/88) | 59.09% (83/88) |
ADC (×10−3 mm2/s) | 0.722 (0.617–0.811) | <0.001 | 0.895 | 87.50% (83/88) | 48.48% (57/88) |
D (×10−3 mm2/s) | 0.833 (0.740–0.903) | <0.001 | 0.666 | 66.67% (57/88) | 93.94% (74/88) |
D* (×10−3 mm2/s) | 0.510 (0.402–0.617) | 0.875 | – | – | – |
f (%) | 0.707 (0.602–0.821) | <0.001 | 2.760 | 87.50% (83/88) | 54.55% (57/88) |
DDC (×10−3 mm2/s) | 0.735 (0.632–0.823) | <0.001 | 1.037 | 62.50% (18/20) | 80.30% (17/20) |
α | 0.777 (0.677–0.858) | <0.001 | 0.855 | 83.33% (18/20) | 68.18% (18/20) |
MTRasym (3.5 ppm) + D | 0.892 (0.809–0.948) | <0.001 | – | 95.83% (18/20) | 66.67% (18/20) |
MTRasym (3.5 ppm), magnetization transfer ratio asymmetry; ADC, apparent diffusion coefficient; D, diffusion coefficient; D*, pseudo-diffusion coefficient; f, perfusion fraction; DDC, distributed diffusion coefficient; α, water molecular diffusion heterogeneity index; AUC, area under the receiver operating characteristic curve. The AUC comparison between the prediction model and different parameters were as follows: MTRasym (3.5ppm), Z=2.512, P=0.012; ADC, Z=3.818, P=0.001; D, Z=1.753, P=0.079; f, Z=3.229, P=0.001; DDC, Z=3.052, P=0.002; α, Z=2.433, P=0.015.
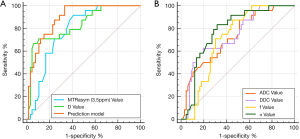
Correlation analysis
MTRasym (3.5 ppm) and α were moderately and weakly positively correlated, respectively, with histological grade and the Ki-67 index (r=0.528, r=0.514, r=0.395, and r=0.367). D was moderately negatively correlated with histological grade and the Ki-67 index (r=–0.540 and r=–0.529). DDC was weakly and moderately negatively correlated with histological grade and the Ki-67 index, respectively (r=–0.473 and r=–0.515). ADC was weakly negatively correlated with histological grade and the Ki-67 index (r=–0.417 and r=–0.427). f was weakly positively correlated with histological grade and the Ki-67 index (r=0.294 and r=0.355; Figure 5).
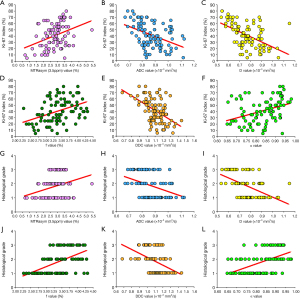
Discussion
Our analyses revealed that high-grade EA was associated with high MTRasym (3.5 ppm), and low-grade EA was associated with low MTRasym (3.5 ppm). These findings are consistent with the results of a study by Takayama et al. (19). The role of APTWI in tumors is mainly related to the contents of mobile proteins and peptides in the tissue (15,16). Previous studies have found that changes in cellular density, nuclear atypia, microvessel density (MVD), pH, and microscopic necrosis can increase the contents of mobile proteins and peptides in tissues (17,25,26). With respect to EA, high-grade tumors usually have a higher cellular density, more significant nuclear atypia, greater MVD, more significant pH changes, and more microscopic necrosis compared with low-grade tumors (26-28). Therefore, high-grade tumors have higher MTRasym (3.5 ppm). Echo-planar imaging (EPI)-based APTWI was used in the present study, and although it has lower signal-to-noise ratio (SNR) and is more sensitive to the susceptibility effect than turbo spin-echo (TSE)-based APTWI, its scanning time is quicker, and multiple saturation spectra with varied frequency offsets may be obtained to enhance quantitative accuracy at the same time (29). A previous study has reported used TSE-based APTWI coupled with acceleration schemes (30), and we will endeavor to undertake an evaluation of its clinical application in the future.
ADC, D, and DDC can be employed to reflect the degree of restriction of water molecules and are affected mainly by cellularity (11,12). In this study, due to high cellularity, the above parameters of high-grade EA were all lower than those of low-grade EA, consistent with most previous studies (31,32). In addition, our results showed that D had greater diagnostic properties than did DDC and ADC for differentiating high-grade EA from low-grade EA. Theoretically, DDC, weighted by the volume fraction of water molecules in each part of the continuous distribution of ADCs, can be considered a composite of individual ADCs (12). Therefore, the measurements of ADC and DDC would be influenced by microcirculation and cellularity in a diametrically opposite direction (13). High-grade EA possesses both high perfusion (high vascularity) and low diffusivity (high cellularity). D, by eliminating the influence of microcirculation, precisely predicted cellularity and reduced bias, which provided better diagnostic performance.
The f value, as the SI ratio of blood capillaries and tumor tissue, is considered proportional to MVD (11). High-grade EA has higher vascularity than does low-grade EA, so its f value increased. However, this finding was not consistent with the results described by Zhang et al. (31). We speculated that this difference might have been related to the choice of b values employed by Zhang et al., which reached 2,000 s/mm2 (31). An IVIM study on prostate cancer showed that when the maximal b value exceeded 750 s/mm2, the f value of tumor tissues significantly decreased and was indistinguishable from that of normal tissue (33).
D* is believed to be mainly proportional to the capillary segment length and average blood velocity (11). In this study, the results showed that the difference in D* between high- and low-grade EA was not significant. We believe that this finding may be due to the opposing influence of the capillary segment length and average blood velocity. Although there were more capillary segments in high-grade EA than in low-grade EA, most of them had a sluggish flow and more proliferating cellularity, which resulted in a similar D* value between high- and low-grade EA. Due to poor measurement reproducibility, low SNR, and tumor heterogeneity, a consistent conclusion among the many current studies related to D* has not been reached (31,33,34). Therefore, the reliability of D* may need to be further studied.
The parameter α, ranging from 0 to 1, describes intravoxel water diffusion heterogeneity. In general, tissue with higher cellular and glandular pleomorphism will have a higher level of intravoxel diffusion heterogeneity, resulting in a lower α value (12,35). Our results revealed that α was significantly lower in high-grade EA than in low-grade EA. One possible reason for this finding is that high-grade EA exhibits more intravoxel diffusion heterogeneity than does low-grade EA because it possesses more histologic heterogeneity, including a higher level of tortuous vascular hyperplasia, heterogeneous cellularity, and intravoxel microscopic necrotic foci.
The present study also showed that D and MTRasym (3.5 ppm) were independent predictors of high- and low-grade EA, and the combination of the 2 parameters had significantly better diagnostic efficacy than did each independent parameter. These results suggested that the true diffusion of water molecules and the mobile protein and peptide contents might have played a dominant role in the prediction of high- and low-grade EA. The combination of a high MTRasym (3.5 ppm) and low D in EA tissue may be an effective imaging marker for predicting histological grade.
As the most commonly used parameter in the clinical evaluation of tumor cell proliferation, the Ki-67 index provides important information on endometrial malignant transformation, cell differentiation, and morphological features of aggressiveness (7). In this study, except for D*, all other parameters showed different degrees of correlation with the Ki-67 index. One possible explanation for this finding is that a high Ki-67 index correlates with increased cell proliferation, which tends to correlate with higher cellular density, more vascular hyperplasia, and more histologic heterogeneity (36-38).
Our study had several limitations. First, this was a single-center study with a relatively small sample size and few EC subtypes, which might have led to selection bias. Second, to fully reflect the characteristics of tumors, multiple APTWI scans must be performed, which significantly increases the scanning time and risk of various artifacts. Third, both APTWI and multimodel DWI, based on EPI acquisition, have poor SNR and low spatial resolution, and we did not perform motion correction, which makes it difficult to evaluate small EA lesions. In the future, we will endeavor to overcome these shortcomings by using a multicenter prospective cohort and external validation to ensure that this method can be used in clinical practice.
In conclusion, we found that both multimodel DWI and APTWI could be used to estimate the histological grade and Ki-67 index of EA, and the combination of a high MTRasym (3.5 ppm) and low D may be an effective imaging marker for predicting the grade of EA.
Acknowledgments
Funding: This study received funding from the National Key R&D Program of China (No. 2017YFE0103600), the Henan Medical Science and Technology Research Program (No. 2018020357 and 2018020367), the National Natural Science Foundation of China (No. 81720108021 and 31470047), and Zhongyuan Thousand Talents Plan Project – Basic Research Leader Talent (No. ZYQR201810117), Zhengzhou Collaborative Innovation Major Project (No. 20XTZX05015).
Footnote
Conflicts of Interest: All authors have completed the ICMJE uniform disclosure form (available at https://dx.doi.org/10.21037/qims-21-189). KYW, an MR collaborating scientist from GE Healthcare provided technical support under the GE collaboration regulations and had no financial or other conflicts for this study. The other authors have no conflicts of interest to declare.
Ethical Statement: The authors are accountable for all aspects of the work in ensuring that questions related to the accuracy or integrity of any part of the work are appropriately investigated and resolved. The study was conducted in accordance with the Declaration of Helsinki (as revised in 2013). This prospective study complied with ethical committee standards and was approved by the ethics committee of the First Affiliated Hospital of Xinxiang Medical University (No. 2018067). Informed consent was taken from all individual participants.
Open Access Statement: This is an Open Access article distributed in accordance with the Creative Commons Attribution-NonCommercial-NoDerivs 4.0 International License (CC BY-NC-ND 4.0), which permits the non-commercial replication and distribution of the article with the strict proviso that no changes or edits are made and the original work is properly cited (including links to both the formal publication through the relevant DOI and the license). See: https://creativecommons.org/licenses/by-nc-nd/4.0/.
References
- Siegel RL, Miller KD, Jemal A. Cancer statistics, 2019. CA Cancer J Clin 2019;69:7-34. [Crossref] [PubMed]
- Chen W, Zheng R, Baade PD, Zhang S, Zeng H, Bray F, Jemal A, Yu XQ, He J. Cancer statistics in China, 2015. CA Cancer J Clin 2016;66:115-32. [Crossref] [PubMed]
- Becker JH, Ezendam NP, Boll D, van der Aa M, Pijnenborg JM. Effects of surgical volumes on the survival of endometrial carcinoma. Gynecol Oncol 2015;139:306-11. [Crossref] [PubMed]
- Nougaret S, Horta M, Sala E, Lakhman Y, Thomassin-Naggara I, Kido A, Masselli G, Bharwani N, Sadowski E, Ertmer A, Otero-Garcia M, Kubik-Huch RA, Cunha TM, Rockall A, Forstner R. Endometrial Cancer MRI staging: Updated Guidelines of the European Society of Urogenital Radiology. Eur Radiol 2019;29:792-805. [Crossref] [PubMed]
- Arora V, Quinn MA. Endometrial cancer. Best Pract Res Clin Obstet Gynaecol 2012;26:311-24. [Crossref] [PubMed]
- Sobecki M, Mrouj K, Colinge J, Gerbe F, Jay P, Krasinska L, Dulic V, Fisher D. Cell-Cycle Regulation Accounts for Variability in Ki-67 Expression Levels. Cancer Res 2017;77:2722-34. [Crossref] [PubMed]
- Kitson S, Sivalingam VN, Bolton J, McVey R, Nickkho-Amiry M, Powell ME, Leary A, Nijman HW, Nout RA, Bosse T, Renehan AG, Kitchener HC, Edmondson RJ, Crosbie EJ. Ki-67 in endometrial cancer: scoring optimization and prognostic relevance for window studies. Mod Pathol 2017;30:459-68. [Crossref] [PubMed]
- Ahmed M, Al-Khafaji JF, Class CA, Wei W, Ramalingam P, Wakkaa H, Soliman PT, Frumovitz M, Iyer RB, Bhosale PR. Can MRI help assess aggressiveness of endometrial cancer? Clin Radiol 2018;73:833.e11-8. [Crossref] [PubMed]
- Nougaret S, Reinhold C, Alsharif SS, Addley H, Arceneau J, Molinari N, Guiu B, Sala E. Endometrial Cancer: Combined MR Volumetry and Diffusion-weighted Imaging for Assessment of Myometrial and Lymphovascular Invasion and Tumor Grade. Radiology 2015;276:797-808. [Crossref] [PubMed]
- Savaridas SL, Brook J, Codde JP, Bulsara M, Wylie E. The effect of individual radiographers on rates of attendance to breast screening: a 7-year retrospective study. Clin Radiol 2018;73:413.e7-413.e13. [Crossref] [PubMed]
- Le Bihan D, Breton E, Lallemand D, Aubin ML, Vignaud J, Laval-Jeantet M. Separation of diffusion and perfusion in intravoxel incoherent motion MR imaging. Radiology 1988;168:497-505. [Crossref] [PubMed]
- Bennett KM, Schmainda KM, Bennett RT, Rowe DB, Lu H, Hyde JS. Characterization of continuously distributed cortical water diffusion rates with a stretched-exponential model. Magn Reson Med 2003;50:727-34. [Crossref] [PubMed]
- Bai Y, Lin Y, Tian J, Shi D, Cheng J, Haacke EM, Hong X, Ma B, Zhou J, Wang M. Grading of Gliomas by Using Monoexponential, Biexponential, and Stretched Exponential Diffusion-weighted MR Imaging and Diffusion Kurtosis MR Imaging. Radiology 2016;278:496-504. [Crossref] [PubMed]
- Iima M, Kataoka M, Kanao S, Onishi N, Kawai M, Ohashi A, Sakaguchi R, Toi M, Togashi K. Intravoxel Incoherent Motion and Quantitative Non-Gaussian Diffusion MR Imaging: Evaluation of the Diagnostic and Prognostic Value of Several Markers of Malignant and Benign Breast Lesions. Radiology 2018;287:432-41. [Crossref] [PubMed]
- Zhou J, Payen JF, Wilson DA, Traystman RJ, van Zijl PC. Using the amide proton signals of intracellular proteins and peptides to detect pH effects in MRI. Nat Med 2003;9:1085-90. [Crossref] [PubMed]
- Zhou J, Lal B, Wilson DA, Laterra J, van Zijl PC. Amide proton transfer (APT) contrast for imaging of brain tumors. Magn Reson Med 2003;50:1120-6. [Crossref] [PubMed]
- Togao O, Yoshiura T, Keupp J, Hiwatashi A, Yamashita K, Kikuchi K, Suzuki Y, Suzuki SO, Iwaki T, Hata N, Mizoguchi M, Yoshimoto K, Sagiyama K, Takahashi M, Honda H. Amide proton transfer imaging of adult diffuse gliomas: correlation with histopathological grades. Neuro Oncol 2014;16:441-8. [Crossref] [PubMed]
- Ohno Y, Yui M, Koyama H, Yoshikawa T, Seki S, Ueno Y, Miyazaki M, Ouyang C, Sugimura K. Chemical Exchange Saturation Transfer MR Imaging: Preliminary Results for Differentiation of Malignant and Benign Thoracic Lesions. Radiology 2016;279:578-89. [Crossref] [PubMed]
- Takayama Y, Nishie A, Togao O, Asayama Y, Ishigami K, Ushijima Y, Okamoto D, Fujita N, Sonoda K, Hida T, Ohishi Y, Keupp J, Honda H. Amide Proton Transfer MR Imaging of Endometrioid Endometrial Adenocarcinoma: Association with Histologic Grade. Radiology 2018;286:909-17. [Crossref] [PubMed]
- Iima M, Le Bihan D. Clinical Intravoxel Incoherent Motion and Diffusion MR Imaging: Past, Present, and Future. Radiology 2016;278:13-32. [Crossref] [PubMed]
- Jia G, Abaza R, Williams JD, Zynger DL, Zhou J, Shah ZK, Patel M, Sammet S, Wei L, Bahnson RR, Knopp MV. Amide proton transfer MR imaging of prostate cancer: a preliminary study. J Magn Reson Imaging 2011;33:647-54. [Crossref] [PubMed]
- Liu R, Zhang H, Niu W, Lai C, Ding Q, Chen W, Liang S, Zhou J, Wu D, Zhang Y. Improved chemical exchange saturation transfer imaging with real-time frequency drift correction. Magn Reson Med 2019;81:2915-23. [Crossref] [PubMed]
- Lo TK, So CH, Yeung SW, Fung M, Lui KY, Pan NY. Comparison of selective and non-selective internal iliac artery embolization for abnormal placentation with major postpartum hemorrhage. Int J Gynaecol Obstet 2017;136:103-4. [Crossref] [PubMed]
- Chu CJ, Chan A, Song D, Staley KJ, Stufflebeam SM, Kramer MA. A semi-automated method for rapid detection of ripple events on interictal voltage discharges in the scalp electroencephalogram. J Neurosci Methods 2017;277:46-55. [Crossref] [PubMed]
- Zheng S, van der Bom IM, Zu Z, Lin G, Zhao Y, Gounis MJ. Chemical exchange saturation transfer effect in blood. Magn Reson Med 2014;71:1082-92. [Crossref] [PubMed]
- Ray KJ, Simard MA, Larkin JR, Coates J, Kinchesh P, Smart SC, Higgins GS, Chappell MA, Sibson NR. Tumor pH and Protein Concentration Contribute to the Signal of Amide Proton Transfer Magnetic Resonance Imaging. Cancer Res 2019;79:1343-52. [Crossref] [PubMed]
- Kapucuoglu N, Bulbul D, Tulunay G, Temel MA. Reproducibility of grading systems for endometrial endometrioid carcinoma and their relation with pathologic prognostic parameters. Int J Gynecol Cancer 2008;18:790-6. [Crossref] [PubMed]
- Fukunaga T, Fujii S, Inoue C, Kato A, Chikumi J, Kaminou T, Ogawa T. Accuracy of semiquantitative dynamic contrast-enhanced MRI for differentiating type II from type I endometrial carcinoma. J Magn Reson Imaging 2015;41:1662-8. [Crossref] [PubMed]
- Zhao X, Wen Z, Zhang G, Huang F, Lu S, Wang X, Hu S, Chen M, Zhou J. Three-dimensional turbo-spin-echo amide proton transfer MR imaging at 3-Tesla and its application to high-grade human brain tumors. Mol Imaging Biol 2013;15:114-22. [Crossref] [PubMed]
- Heo HY, Zhang Y, Lee DH, Jiang S, Zhao X, Zhou J. Accelerating chemical exchange saturation transfer (CEST) MRI by combining compressed sensing and sensitivity encoding techniques. Magn Reson Med 2017;77:779-86. [Crossref] [PubMed]
- Zhang Q, Ouyang H, Ye F, Chen S, Xie L, Zhao X, Yu X. Multiple mathematical models of diffusion-weighted imaging for endometrial cancer characterization: Correlation with prognosis-related risk factors. Eur J Radiol 2020;130:109102. [Crossref] [PubMed]
- Yan B, Zhao T, Liang X, Niu C, Ding C. Can the apparent diffusion coefficient differentiate the grade of endometrioid adenocarcinoma and the histological subtype of endometrial cancer? Acta Radiol 2018;59:363-70. [Crossref] [PubMed]
- Pang Y, Turkbey B, Bernardo M, Kruecker J, Kadoury S, Merino MJ, Wood BJ, Pinto PA, Choyke PL. Intravoxel incoherent motion MR imaging for prostate cancer: an evaluation of perfusion fraction and diffusion coefficient derived from different b-value combinations. Magn Reson Med 2013;69:553-62. [Crossref] [PubMed]
- Liu C, Wang K, Chan Q, Liu Z, Zhang J, He H, Zhang S, Liang C. Intravoxel incoherent motion MR imaging for breast lesions: comparison and correlation with pharmacokinetic evaluation from dynamic contrast-enhanced MR imaging. Eur Radiol 2016;26:3888-98. [Crossref] [PubMed]
- Seo N, Chung YE, Park YN, Kim E, Hwang J, Kim MJ. Liver fibrosis: stretched exponential model outperforms mono-exponential and bi-exponential models of diffusion-weighted MRI. Eur Radiol 2018;28:2812-22. [Crossref] [PubMed]
- Xu M, Tang Q, Li M, Liu Y, Li F. An analysis of Ki-67 expression in stage 1 invasive ductal breast carcinoma using apparent diffusion coefficient histograms. Quant Imaging Med Surg 2021;11:1518-31. [Crossref] [PubMed]
- Wu W, Jiang G, Xu Z, Wang R, Pan A, Gao M, Yu T, Huang L, Quan Q, Li J. Three-dimensional pulsed continuous arterial spin labeling and intravoxel incoherent motion imaging of nasopharyngeal carcinoma: correlations with Ki-67 proliferation status. Quant Imaging Med Surg 2021;11:1394-405. [Crossref] [PubMed]
- Zhang J, Chen X, Chen D, Wang Z, Li S, Zhu W. Grading and proliferation assessment of diffuse astrocytic tumors with monoexponential, biexponential, and stretched-exponential diffusion-weighted imaging and diffusion kurtosis imaging. Eur J Radiol 2018;109:188-95. [Crossref] [PubMed]