Segmentation and characterization of visceral and abdominal subcutaneous adipose tissue on CT with and without contrast medium: influence of 2D- and 3D-segmentation
Introduction
Quantity and density of adipose tissue determined by CT may serve as valuable biomarkers in a variety of settings, e.g., for the assessment of frailty, metabolic characterization, stratification of cardiovascular risk or assessment of risk prior to major surgery or chemotherapy (1-7). Body composition analysis with CT has been performed since the early 1980s and in many instances now replaces previous methods such as nuclear medicine, morphometric techniques or bioelectrical impedance analysis for the purpose of fat quantification for its advantages in precision, accessibility or simply because of the availability of CT scans in patients with serious illnesses (8-13). Kvist et al. introduced thresholds for semi-automatic segmentation of adipose tissue derived from a series of CT slices over the entire human body that are still frequently used today (14).
Abdominal adipose tissue can either be segmented in its entirety or more rapidly and more commonly done in a single slice. Some studies have determined the relationship between volumetric and planimetric measurements to each other, to morphometric measurements and to cardio-metabolic risk factors e.g., of the Framingham Heart Study offspring cohort to determine the most representative single slice for 2D-measurements (11,15). Despite some studies having analyzed the effect of iodinated contrast medium (ICM) on the results of the segmentation of adipose tissue (16-19), most of the work in validating and correlating these results has been performed on non-enhanced scans (NES) (1,9,10,14,15).
Recently, we demonstrated the conversion of volumetrically derived density and quantity of adipose tissue segmented in contrast-enhanced scans (CES) for three commonly acquired contrast phases into values obtained from NES to be feasible with only small error (20). Such conversion can be performed by entering the obtained results of segmentation of CES into simple conversion formulae. Despite being more time-consuming than segmenting a single CT slice, this is important as it potentially enables the comparison of data derived from patients scanned with ICM and/or scanned in a different contrast phase or NES to one another. However, for clinical correlation 2D-segmetnation is still most frequently employed.
The aim of our study was to analyze the influence of contrast medium and contrast phase on the density and quantity of adipose tissue in the visceral and subcutaneous abdominal compartments through three commonly acquired contrast-phases in a single segmented CT slice and to determine whether or not those values obtained from CES may also be converted into those of NES. Furthermore, we wanted to compare the precision of conversion of the values obtained from segmentation of a single slice (2D) to that of the entire abdomen (3D).
Methods
Study design
The study population and design have previously been described (20). Briefly, consecutive CT-studies of the abdomen of 31 patients with an NES and at least one CES, scanned with the same CT-scanner at identical scan parameters, were retrospectively included. No enteral contrast medium was applied. The inclusion criteria were constant scan parameters within each examination, identical reconstruction of images and identical application and amount of ICM. No further selection took place. In sum, 95 scans were taken into account: 31 NES, 23 arterial (ART), 10 portal-venous (PVN) and 31 venous (VEN).
The study was conducted in accordance with the Declaration of Helsinki (as revised in 2013). The study was approved by the institutional ethics committee of Leipzig University (reference number: 337/19-ek) and individual consent for this retrospective analysis was waived.
Baseline characteristics
Patients were 69.3±12.5 years old, weighed 77.5±15.9 kg, had a body mass index (BMI) of 26.9±4.7 kg/m2 and 42% (13/31) were women. Further details and clinical characterization have been described previously (20).
CT acquisition, image reconstruction and analysis
Image acquisition and reconstruction was performed identically to a previous study for 3D-segmentation (20). Care was taken to ensure very similar delays between the scans (ART, PVN, VEN) after intravenous injection of the same amount of ICM. For image reconstruction, slice thickness was 3 mm and a medium smooth filtered back projection kernel (B30f) was used. For planimetric segmentation of adipose tissue the same threshold-based semi-automatic method was employed using specialized open source software (Slicer, v4.10.2, http://www.slicer.org/) (21). The threshold range for segmentation of adipose tissue was set from −190 to −30 HU (14). In contrast to our previous study (20), segmentation was performed in a single slice at L2/3, just above L3 (11,22); again, separately for visceral adipose tissue (VAT) and subcutaneous adipose tissue (SAT) (Figure 1). To ensure consistency of segmentation, five data sets were initially segmented for training purposes multiple times. After reliably identifying the chosen landmarks and obtaining very consistent data, no intra- or inter-observer agreement was taken. Area and density were recorded separately for the two compartments. After training, segmentation of SAT and VAT took less than 2 minutes per slice.
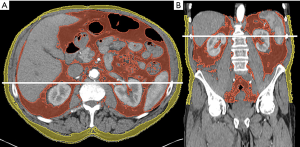
Total adipose tissue (TAT) was calculated as follows:
Statistical analysis
While categorical variables are given as count and percentage, continuous variables are presented as mean and standard deviation when symmetrically distributed or as median and interquartile range for skewed distributions.
Intra-patient differences between CES and NES measurements (defined as CES minus NES) as well as between 2D- and 3D-segmentation with respect to changes in density (defined as 3D minus 2D) were tested using a paired t-test. For relative differences of NES and CES measurements (i.e., ratio CES/NES), we applied a one-sample t-test to the log-transformed ratios. For comparison of changes in quantity of adipose tissue between 2D- and 3D-segmentation, a Wilcoxon signed-rank test was used. All tests were performed at a significance level of 5%. In addition, 95% confidence intervals were calculated for the estimated sample means.
We performed linear regression analyses to assess the relationship between NES and CES measurements. For validation of the regression models, each sample was randomly divided into training and test data at a proportion of 75:25% (ART: 17:6 patients; PVN: 7:3; VEN: 23:8). Linear regression models were derived using only the training data and subsequently validated using the test data. For this purpose, root-mean-square error (RMSE) was calculated for both data sets and compared.
For the comparison of 2D- and 3D-segmentation, we calculated the normalized root-mean-square error (NRMSE) to account for potentially different units of measurement (i.e., dm3vs. dm2). In contrast to RMSE, training and test data were merged for the calculation of NRMSE. The normalization of RMSE was performed using the standard deviation. Interdimensional NRMSE differences were calculated as 3D minus 2D.
All statistical analyses were performed using R (v4.0.3, R Foundation for Statistical Computing, Vienna, Austria).
Results
2D-segmentation
After the application of ICM, mean density between NES and CES increased significantly (P<0.05) in all compartments (TAT, VAT and SAT) and contrast phases (ART, PVN and VEN) with the exception of SAT in ART (+0.9±2.2 HU, P=0.07). The changes in density were more pronounced in the visceral compartment (VAT > TAT > SAT) and overall, they were more marked in VEN and PVN compared to ART (VEN ≈ PVN > ART). Further detail regarding the density of adipose tissue in NES and CES segmented in 2D is given in Table 1.
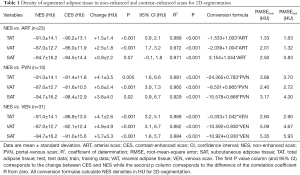
Full table
Area of segmented adipose tissue decreased after the application of ICM in all contrast phases and compartments with the exception of SAT in ART [+0.85% (3.66%), P=0.64], but only reached significance in VAT and TAT in both ART and VEN, and TAT in PVN (P<0.05). Similarly to density, the changes in area were more pronounced in later CES (VEN > PVN > ART). Further detail regarding the area of adipose tissue segmented in NES and CES is listed in Table 2.
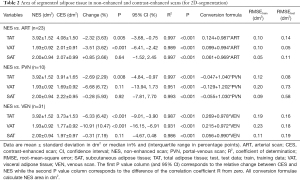
Full table
Overall, the linear regression models constructed for NES and CES for density and area showed good fit to the respective data points (Tables 1,2, Figures 2,3). However, the goodness of fit and therefore the precision of the conversion of these models was best for ART and increased further for area in ART when considering VAT and SAT separately, shown by smaller RMSE (Table 1, Figure 2). RMSE of density in PVN is smallest for VAT, and in VEN for TAT. RMSE of area in PVN and VEN does not seem to favor any compartment.
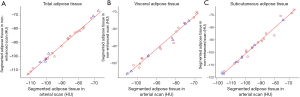
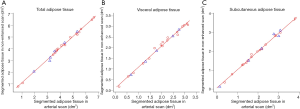
Results of linear regression are displayed as conversion formulae (Tables 1,2), which can be used to obtain values for NES by simply entering the results of segmentation of CES.
Differences between 2D- and 3D-segmentation
Differences in the change of the density of adipose tissue segmented in 2D and 3D were small (<2 HU) and, overall, slightly lower for 3D-segmentation. The changes were significantly different for TAT in ART (–0.6±1.3 HU, P=0.03), VAT in PVN (–1.2±1.5 HU, P=0.03) and TAT in VEN (–1.1±1.8 HU, P=0.002). No further significant differences in the changes of density were observed between modes of segmentation (Table 3).
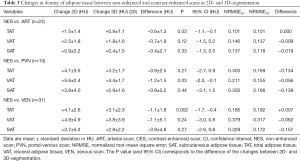
Full table
Regression analyses of the relationship of density in NES and CES showed relatively small differences between 2D- and 3D-segmentation with equivalent goodness of fit in ART in all compartments with almost identical NRMSE. In PVN NRMSE for TAT, VAT and SAT is approximately half for 3D-segmentation. Except for TAT in ART and VEN, NRMSE for density is consistently smaller for 3D-segmentation. For further detail see Table 3.
Differences in the change of the quantity (area and volume) of adipose tissue segmented in 2D and 3D were small (<5%) and most pronounced in PVN. However, no significant differences were observed (Table 4).
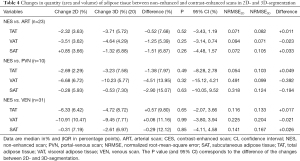
Full table
Regression analyses of the relation of quantity in NES and CES between 2D- and 3D-segmentation showed mixed results for different compartments. NRMSE for quantity is consistently smaller with 3D-segmentation for VAT. Except for SAT in PVN, NRMSE for quantity is consistently smaller with 2D-segmentation for TAT and SAT (Table 4).
Discussion
The application of contrast medium changes the density and quantity of segmented adipose tissue, a biomarker potentially obtained from any CT scan. The extent of this change is influenced by the time elapsed after injection, differs between abdominal compartments and varies between 2D- and 3D-segmentation. This may introduce bias when comparing results to studies performed with different techniques in scan and/or evaluation, which may well be adjusted for by means of conversion formulae (Tables 1,2).
Intravenously applied ICM increases the density and decreases the area of abdominal adipose tissue segmented in a single slice. Evidently, ICM increases the density of adipose tissue leading to both increased density of voxels within the given threshold range and thus to the misclassification of voxels that otherwise would have been classified as adipose tissue. Presumably, this is mediated through a mix of direct and partial volume effect. However, as adipose tissue is generally poorly vascularized, partial volume effect is likely to dominate, particularly in ART. Furthermore, the arterial vascular cross-section is relatively constant between individuals and is much less influenced by acute or even chronic pathological processes compared to the venous vasculature. This likely explains the more precise conversion of results from ART in to that of NES with universally smaller RMSE and NRMSE compared to PVN and VEN (Tables 1,2,3,4).
Similar to our previous study that had segmented the adipose tissue of the entire abdomen, the effect of ICM on the results of segmentation was influenced by the time elapsed after injection of ICM and was thus most pronounced in PVN and VEN and least pronounced in ART (20). Similarly, changes were different between the abdominal compartments, with the largest changes observed in VAT and the smallest changes in SAT. The observed changes in area (ART median: −2.32%, mean: −2.74%; PVN median: −2.69%, mean: −1.92%) and density (Table 1) between NES and CES of VAT are comparable to the observed changes in a different patient cohort (reported mean of change in area for ART and PVN between −2% to −3%) (18) and the same patient cohort but volumetrically derived results (Tables 3,4) (20). The results of another study assessing changes of segmented adipose tissue after application of ICM in perfusion CT are very similar regarding the change in density (reported mean ART: +2 HU; PVN: +3 HU) but seem somewhat high for the reported change in adipose tissue index (reported mean for ART: −6.5%; and PVN: −6.4%) (16). A very large study performed on healthy potential kidney donors found the area of adipose tissue to decrease by as much as −25.4% for VAT and −9.4% for SAT in VEN (19). However, this study employed a relatively large dose of ICM (150 mL) and long delay of over 5 minutes after injecting part of that ICM, likely explaining the much larger changes. The only other publication on this matter neither kept the amount of ICM constant between patients nor did it dose it according to lean body mass but rather dosed it according to patients’ body mass index (17). This study observed a positive correlation between the changes of segmented adipose tissue and patients’ BMI, likely constituting confounding as ICM was dosed according to patients’ BMI.
While the direction of the change in segmented adipose tissue shows strong parallels in regard to density and quantity between contrast phases and abdominal compartments to the study that had segmented the entire abdominal adipose tissue of the same patients, the extent of the change of density partially differs significantly (Table 3). These differences may be attributed to differing structure of adipose tissue within a given compartment. Similarly to the unequal impact of ICM on adipose tissue between compartments with differing embryological origin (23,24), differing metabolic and endocrine function (11,25) and ultimately differing structure, the adipose tissue within a given compartment is not homogeneous. This is particularly evident within the abdominal cavity with VAT in the upper abdomen mostly surrounding the parenchymal abdominal organs and in the lower abdomen being contained predominantly within mesentery, the greater omentum and around the large and small bowel. Consequently, the effect of ICM on segmented adipose tissue varies depending not only on the anatomical compartment, but also on the particular level examined.
In spite of the inhomogeneity within the adipose tissue of the abdomen, the relation of values obtained in NES and CES from just a single slice is strong and allows for a robust conversion of values obtained from CES into those of NES with only a small error (Figures 2,3, Tables 1,2). Despite the differences in the extent of change between 2D- and 3D-segmentation, the respective relationship of NES and CES is very strong and shows virtually no difference between the two modes of segmentation for density in ART (Table 3). While for density in later contrast phases, segmentation in 3D seems to be somewhat advantageous, the relationship for quantity is more complex and partly even more tight-fitting for 2D and hence precise when used for conversion (Table 4).
One could argue that the observed changes in the density and quantity of segmented adipose tissue after the application of ICM were not clinically relevant at all for stratifying cardio-vascular risk or risk in general. This argument might become particularly true when focusing on ART as frequently acquired in cardio-vascular radiology, considering the relatively small changes compared to those in VEN or PVN. However, changes of as little as +6 HU of SAT density or −13% of VAT area have been shown to negatively influence the survival of elderly patients with severe aortic stenosis after transcatheter aortic valve implantation (1). It is likely that even more subtle differences in the density and quantity of adipose tissue may be indicative of increased risk or a pathological state in other patient populations. Therefore, the possibility of converting adipose tissue metrics from one contrast phase into another by means of simple conversion formulae may be of great value. Thus, said metrics can be compared to those of previous studies with only small error.
Considering the rather small difference in error between 2D- and 3D-segmentation in the conversion of density and quantity from CES into that of NES, 2D-segmentation seems to be the reasonable approach in most instances for several reasons. First, 2D-segmentation is more frequently applied in body composition analysis today and therefore offers a greater opportunity for the comparison of data to that of other cohorts. Furthermore, although the direct measurement of abdominal adipose tissue is more precise for obvious reasons, its additional value compared to the precise exemplary measurement of a single slice is not well characterized and may therefore not justify the additional effort required when performed semi-automatically. However, with the advent of new fully automated options for tissue segmentation including machine learning, volumetric measurements or the combined volumetric and planimetric measurement may soon become a reasonable choice (26-28).
Limitations
This study was retrospectively conducted and only comprises a moderate number of patients. As the indication for examination varied, not all patients included had received all contrast phases with PVN being less frequently included than other contrast phases. The precise characterization of the influence of ICM on PVN and delineation from VEN is therefore somewhat limited. Furthermore, differences in adipose tissue segmented with 2D and 3D were small and only reached statistical significance for density of TAT in ART and VEN, but not for quantity, likely attributable to the moderate sample size. Furthermore, the sample size was relatively small. However, as mainly intra-individual differences were assessed at a single point in time, while all other parameters of the scan were kept constant, the sample size was sufficient for calculating and validating conversion formulae (Tables 1,2) and for comparing the modes of segmentation adequately (Tables 3,4).
No selection or exclusion of potentially interfering medical conditions possibly changing the interaction of adipose tissue with ICM took place. Even though mainly intra-individual differences were observed and the direction and magnitude of change after the application of ICM were relatively constant between individuals and within the given mode of segmentation, the extent of change may still have been influenced by these conditions. Therefore, caution is advised when applying our findings to other patient cohorts or healthy volunteers, particularly in regard to later contrast phases.
Conclusions
Iodinated contrast medium changes the density and area of adipose tissue segmented in a single slice. These changes vary similarly between abdominal compartments (visceral > total > subcutaneous abdominal adipose tissue) and contrast phases (venous ≈ portal-venous > arterial) compared to those observed with the segmentation of the entire abdomen but differ somewhat in extent between 2D- and 3D-segmentation.
As the changes in density and quantity induced by contrast medium are fairly characteristic for a given contrast phase, compartment and mode of segmentation, the density and quantity of adipose tissue may be accurately calculated from the segmentation of contrast enhanced scans by means of dedicated conversion formulae with similar precision for 2D- and 3D-segmentation.
Acknowledgments
The authors thank Werner Daßler for his kind support in language editing. We acknowledge the position of Robin F. Gohmann to have been equally funded by Leipzig University and Leipzig Heart Center. We acknowledge support from Leipzig University for Open Access Publishing.
Funding: None.
Footnote
Conflicts of Interest: All authors have completed the ICMJE uniform disclosure form (available at https://dx.doi.org/10.21037/qims-21-178). The authors have no conflicts of interest to declare.
Ethical Statement: The authors are accountable for all aspects of the work in ensuring that questions related to the accuracy or integrity of any part of the work are appropriately investigated and resolved. The study was conducted in accordance with the Declaration of Helsinki (as revised in 2013). The study was approved by the institutional ethics committee of Leipzig University (reference number: 337/19-ek) and individual consent for this retrospective analysis was waived.
Open Access Statement: This is an Open Access article distributed in accordance with the Creative Commons Attribution-NonCommercial-NoDerivs 4.0 International License (CC BY-NC-ND 4.0), which permits the non-commercial replication and distribution of the article with the strict proviso that no changes or edits are made and the original work is properly cited (including links to both the formal publication through the relevant DOI and the license). See: https://creativecommons.org/licenses/by-nc-nd/4.0/.
References
- Foldyna B, Troschel FM, Addison D, Fintelmann FJ, Elmariah S, Furman D, Eslami P, Ghoshhajra B, Lu MT, Murthy VL, Hoffmann U, Shah R. Computed tomography-based fat and muscle characteristics are associated with mortality after transcatheter aortic valve replacement. J Cardiovasc Comput Tomogr 2018;12:223-8. [Crossref] [PubMed]
- Kalafateli M, Mantzoukis K, Choi Yau Y, Mohammad AO, Arora S, Rodrigues S, de Vos M, Papadimitriou K, Thorburn D, O'Beirne J, Patch D, Pinzani M, Morgan MY, Agarwal B, Yu D, Burroughs AK, Tsochatzis EA. Malnutrition and sarcopenia predict post-liver transplantation outcomes independently of the Model for End-stage Liver Disease score. J Cachexia Sarcopenia Muscle 2017;8:113-21. [Crossref] [PubMed]
- Popinat G, Cousse S, Goldfarb L, Becker S, Gardin I, Salaün M, Thureau S, Vera P, Guisier F, Decazes P. Sub-cutaneous Fat Mass measured on multislice computed tomography of pretreatment PET/CT is a prognostic factor of stage IV non-small cell lung cancer treated by nivolumab. Oncoimmunology 2019;8:e1580128 [Crossref] [PubMed]
- Prado CM, Lieffers JR, McCargar LJ, Reiman T, Sawyer MB, Martin L, Baracos VE. Prevalence and clinical implications of sarcopenic obesity in patients with solid tumours of the respiratory and gastrointestinal tracts: a population-based study. Lancet Oncol 2008;9:629-35. [Crossref] [PubMed]
- Shuster A, Patlas M, Pinthus JH, Mourtzakis M. The clinical importance of visceral adiposity: a critical review of methods for visceral adipose tissue analysis. Br J Radiol 2012;85:1-10. [Crossref] [PubMed]
- Doyle SL, Bennett AM, Donohoe CL, Mongan AM, Howard JM, Lithander FE, Pidgeon GP, Reynolds JV, Lysaght J. Establishing computed tomography-defined visceral fat area thresholds for use in obesity-related cancer research. Nutr Res 2013;33:171-9. [Crossref] [PubMed]
- Reijrink M, de Boer SA, Spoor DS, Lefrandt JD, Lambers Heerspink HJ, Boellaard R, Greuter MJ, Borra RJH, Hillebrands JL, Slart RHJA, Mulder DJ. Visceral adipose tissue volume is associated with premature atherosclerosis in early type 2 diabetes mellitus independent of traditional risk factors. Atherosclerosis 2019;290:87-93. [Crossref] [PubMed]
- Borkan GA, Gerzof SG, Robbins AH, Hults DE, Silbert CK, Silbert JE. Assessment of abdominal fat content by computed tomography. Am J Clin Nutr 1982;36:172-7. [Crossref] [PubMed]
- Mitsiopoulos N, Baumgartner RN, Heymsfield SB, Lyons W, Gallagher D, Ross R. Cadaver validation of skeletal muscle measurement by magnetic resonance imaging and computerized tomography. J Appl Physiol 1998;85:115-22. [Crossref] [PubMed]
- Sjöström L, Kvist H, Cederblad A, Tylén U. Determination of total adipose tissue and body fat in women by computed tomography, 40K, and tritium. Am J Physiol 1986;250:E736-45. [PubMed]
- Maurovich-Horvat P, Massaro J, Fox CS, Moselewski F, O'Donnell CJ, Hoffmann U. Comparison of anthropometric, area- and volume-based assessment of abdominal subcutaneous and visceral adipose tissue volumes using multi-detector computed tomography. Int J Obes (Lond) 2007;31:500-6. [Crossref] [PubMed]
- Mourtzakis M, Prado CMM, Lieffers JR, Reiman T, McCargar LJ, Baracos VE. A practical and precise approach to quantification of body composition in cancer patients using computed tomography images acquired during routine care. Appl Physiol Nutr Metab 2008;33:997-1006. [Crossref] [PubMed]
- Pickhardt PJ, Graffy PM, Zea R, Lee SJ, Liu J, Sandfort V, Summers RM. Automated CT biomarkers for opportunistic prediction of future cardiovascular events and mortality in an asymptomatic screening population: a retrospective cohort study. Lancet Digit Health 2020;2:e192-200. [Crossref] [PubMed]
- Kvist H, Sjöström L, Tylén U. Adipose tissue volume determinations in women by computed tomography: technical considerations. Int J Obes 1986;10:53-67. [PubMed]
- Irlbeck T, Massaro JM, Bamberg F, O'Donnell CJ, Hoffmann U, Fox CS. Association between single-slice measurements of visceral and abdominal subcutaneous adipose tissue with volumetric measurements: the Framingham Heart Study. Int J Obes (Lond) 2010;34:781-7. [Crossref] [PubMed]
- Morsbach F, Zhang YH, Martin L, Lindqvist C, Brismar T. Body composition evaluation with computed tomography: Contrast media and slice thickness cause methodological errors. Nutrition 2019;59:50-5. [Crossref] [PubMed]
- Vehmas T, Kairemo KJ, Taavitsainen MJ. Measuring visceral adipose tissue content from contrast enhanced computed tomography. Int J Obes Relat Metab Disord 1996;20:570-3. [PubMed]
- Rollins KE, Javanmard-Emamghissi H, Awwad A, Macdonald IA, Fearon KCH, Lobo DN. Body composition measurement using computed tomography: Does the phase of the scan matter? Nutrition 2017;41:37-44. [Crossref] [PubMed]
- Perez AA, Pickhardt PJ, Elton DC, Sandfort V, Summers RM. Fully automated CT imaging biomarkers of bone, muscle, and fat: correcting for the effect of intravenous contrast. Abdom Radiol (NY) 2021;46:1229-35. [Crossref] [PubMed]
- Gohmann RF, Gottschling S, Seitz P, Temiz B, Krieghoff C, Lücke C, Horn M, Gutberlet M. 3D-segmentation and characterization of visceral and abdominal subcutaneous adipose tissue on CT: influence of contrast medium and contrast phase. Quant Imaging Med Surg 2021;11:697-705. [Crossref] [PubMed]
- Fedorov A, Beichel R, Kalpathy-Cramer J, Finet J, Fillion-Robin JC, Pujol S, Bauer C, Jennings D, Fennessy F, Sonka M, Buatti J, Aylward S, Miller JV, Pieper S, Kikinis R. 3D Slicer as an image computing platform for the Quantitative Imaging Network. Magn Reson Imaging 2012;30:1323-41. [Crossref] [PubMed]
- Faron A, Luetkens JA, Schmeel FC, Kuetting DLR, Thomas D, Sprinkart AM. Quantification of fat and skeletal muscle tissue at abdominal computed tomography: associations between single-slice measurements and total compartment volumes. Abdom Radiol (NY) 2019;44:1907-16. [Crossref] [PubMed]
- Chau YY, Bandiera R, Serrels A, Martínez-Estrada OM, Qing W, Lee M, Slight J, Thornburn A, Berry R, McHaffie S, Stimson RH, Walker BR, Chapuli RM, Schedl A, Hastie N. Visceral and subcutaneous fat have different origins and evidence supports a mesothelial source. Nat Cell Biol 2014;16:367-75. [Crossref] [PubMed]
- Hwang I, Kim JB. Two Faces of White Adipose Tissue with Heterogeneous Adipogenic Progenitors. Diabetes Metab J 2019;43:752-62. [Crossref] [PubMed]
- Misra M, Bredella MA, Tsai P, Mendes N, Miller KK, Klibanski A. Lower growth hormone and higher cortisol are associated with greater visceral adiposity, intramyocellular lipids, and insulin resistance in overweight girls. Am J Physiol Endocrinol Metab 2008;295:E385-92. [Crossref] [PubMed]
- Hemke R, Buckless CG, Tsao A, Wang B, Torriani M. Deep learning for automated segmentation of pelvic muscles, fat, and bone from CT studies for body composition assessment. Skeletal Radiol 2020;49:387-95. [Crossref] [PubMed]
- Koitka S, Kroll L, Malamutmann E, Oezcelik A, Nensa F. Fully automated body composition analysis in routine CT imaging using 3D semantic segmentation convolutional neural networks. Eur Radiol 2021;31:1795-804. [Crossref] [PubMed]
- Lee SJ, Liu J, Yao J, Kanarek A, Summers RM, Pickhardt PJ. Fully automated segmentation and quantification of visceral and subcutaneous fat at abdominal CT: application to a longitudinal adult screening cohort. Br J Radiol 2018;91:20170968 [Crossref] [PubMed]