Ultra-high resolution computed tomography of joints: practical recommendations for acquisition protocol optimization
Introduction
Increasing spatial resolution represents a confirmed trend among various computed tomography (CT) manufacturers, and the bulk of literature supporting the clinical benefit of CT scanners using detector elements of 0.25 mm2 (ultra-high resolution CT, UHRCT) is ever-increasing (1-7). Oostveen et al. have indicated that UHRCT may double the spatial resolution compared with standard resolution multi-detector CT (SRCT) scanners, reaching spatial resolutions as low as 0.12 mm (8,9). Ultra-high resolution is one of the expected parallel benefits of the detector systems used for photon counting CT; however, photon counting scanners’ availability and commercialization are still limited by technical issues (e.g., detector cross-talk and pulse pile-up) and cost (10). UHRCT using conventional detectors, on the other hand, is currently available and is particularly promising for the evaluation of small structures with high intrinsic contrast (11-15). Thus, UHRCT can be potentially useful for musculoskeletal imaging, improving the identification of superficial cartilage lesion on CT arthrography, trabecular fractures and characterization of bone tumors, and soft tissue calcifications (16,17) (Figure 1). Moreover, UHRCT coupled with finite element fractal analysis could improve fracture risk estimation in central and peripheral bone structures (18).
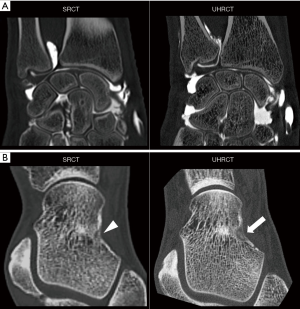
However, in clinical practice, spatial resolution may vary considerably depending on the acquisition protocol, image reconstruction options, and patient body habitus. Part of this problem comes from the fact that a wider range of protocol settings affecting spatial resolution is available with UHRCT scanners (e.g., larger matrix sizes, multiple focal spot sizes, and slice thicknesses as thin as 0.25 mm) and the effect of protocol options in UHRCT might be more pronounced than SRCT. Since UHRCT may require more exposure than SRCT, protocol optimization is paramount to increase spatial resolution while limiting patient exposure (8). Another important issue with UHRCT is the massive amount of data generated, which is sometimes incompatible with current data transfer speeds and storage capabilities, leading to image accessibility issues in clinical practice. Indeed, decreasing slice thickness by half and increasing matrix size from the conventional 512×512 to 2048×2048 leads to a 32-fold increase in the amount of data generated for the same z-axis coverage. Finally, with the decrease in detector element size, factors such as vibrations and patient positioning may have a greater influence on spatial resolution on UHRCT scanners compared to SR ones.
Methods
This study’s objective was to evaluate the influence of various factors on spatial resolution, one of the main factors influencing diagnostic performance in musculoskeletal CT, and provide protocol optimization recommendations in UHRCT. For this purpose, phantom acquisitions and task transfer functions (TTF) (both in-plane and through-plane) were analyzed with different protocol settings to assess the degree of influence of each of these factors on spatial resolution (19).
All acquisitions were performed with a UHRCT scanner (Aquilion Precision, Canon Medical Systems, Otawara, Japan) in helical mode with a z-axis coverage of 40 mm and a one-second tube rotation speed. Variations of the following acquisition parameters were evaluated: field-of-view (FOV), tube voltage, tube current, focal spot size, slice thickness, and reconstruction matrix. Two image reconstruction algorithms were evaluated: hybrid iterative reconstruction (HIR) (AIDR 3D—adaptive iterative reconstruction, Canon Medical Systems) using two different bone kernels (a standard bone kernel - FC30 and a sharp bone kernel—FC80) and model-based iterative reconstruction (MBIR) (FIRST—forward projected model-based iterative reconstruction—bone, Canon Medical Systems). Acquisitions were also performed with the phantom centered and laterally displaced 5 and 8.5 cm. Acquisition dose was kept constant for all acquisitions except for tube output, acquisition matrix, and reconstruction algorithm variations. Table 1 depicts all acquisition protocol variations evaluated.
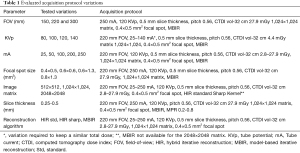
Full table
The CTP528 module of a CATPHAN 600 (The Phantom Laboratory Incorporated) was imaged. This module contains a high-resolution test gauge [from 1 to 21 line pairs per centimeter (lp/cm)] for spatial resolution evaluation. The Catphan phantom’s 404 module was also used to assess TTF on a Teflon insert (e.g., closest to the bone density). This module was scanned perpendicular to the recommended position for evaluating the slice thickness on the “through-plane” spatial resolution, and coronal images with 0.2, 0.5, and 0.8 mm thickness were reconstructed. Images were analyzed with ImageJ 1.48v (National Institute of Health, USA) and ImQuest 7.1, Duke University, Durham, USA). The TTF values at 10% (TTF10%) were calculated in all acquisitions. The TTF10% value amplitude yielded by the variations in each acquisition parameter studied was calculated and compared to each other.
Each variable’s importance on the spatial resolution was first evaluated by a musculoskeletal radiologist with 13 years of clinical experience with CT considering the maximum line pairs per centimeter on the high-resolution test gauge (representing the visual impact of spatial resolution variations). The evaluation was dichotomic (e.g., clearly visible or not). The maximum TTF10% values were then used to stratify parameters further, yielding the same gain in line pairs per centimeter. Based on this analysis, practical recommendations are proposed.
Acquisitions were performed on a cadaveric knee (fresh frozen lower limb specimen) to assess the potential impact of the evaluated protocol changes in clinical practice. This evaluation was done using the same UHRCT scanner but also on a SRCT scanner (Aquilion One Genesis edition, Canon Medical Systems, Otawara, Japan) with the following acquisition protocol: 180 mm FOV, 110 mA, 120 KVp, 0.5 mm slice thickness, 512×512 matrix, 0.9×0.8 mm2 focal spot, MBIR. The specimen was left to thaw for 24hrs on room temperature before imaging.
Since this study was performed on phantom and fully anonymized cadaveric specimens written informed consent and ethical approval were not required, and the terms of the declaration of Helsinki do not apply.
Results
FOV and phantom centering effects
As expected, reducing the FOV led to increased spatial resolution (e.g., linearly decreasing pixel size). There was a 15.1% reduction in the TTF10% values when the FOV was increased from 150 to 300 mm. Visually, the spatial resolution increased from 13 to 16 lp/cm (spatial resolution gain 3 lp/cm).
Lateral displacement had a considerable effect on spatial resolution, with a 30.8% decrease in TTF10% values at maximal lateral displacement (8.5 cm). Visually, this translated into a loss of 4 lp/cm in resolution (from 18 to 14 lp/cm in a centered and laterally displaced phantom, respectively).
Tube output effects
Using the MBIR algorithm, which allegedly offers the best performance at low doses, when the volumic CT dose index estimated with a 32 cm phantom (CTDIvol-32 cm) was increased from 2.8 to 27.9 mGy (25 to 250 mA and 120 KVp), there was 29.6% increase in TTF10% values (TTF10% values were 14.8 and 21 lp/cm, respectively). Visually, the spatial resolution increased from 12 to 17 lp/cm (spatial resolution gain 5 lp/cm) (Figure 2). When a tube voltage variation (80 to 140 KVp) was implemented while keeping the dose level constant (CTDIvol-32 cm of 4.4 mGy), there was no noticeable change in spatial resolution with no variation in the discernable line pairs and a mean TTF10% variation of 0.1%.
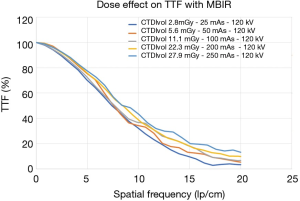
Matrix effects
The matrix effect with a constant FOV on the spatial resolution was dependent on the dose, and the greatest benefit of increasing the matrix size was seen in higher dose levels (Figure 3A). With a CTDIvol-32 cm of 22.3 mGy (120 KVp and 200 mA), increasing the matrix from 512×512 to 2048×2048 led to a 28.2% increase in TTF10% values (from 13.6 to 17.5 lp/cm, respectively), which corresponded to a visual increase of 8 lp/cm (from 13 to 21 lp/cm). The same matrix size increase with a CTDIvol-32 cm of 2.8 mGy (120 KVp and 25 mA) led to a 6% increase in TTF10% values with no difference in the number of line pairs per centimeter (10 lp/cm in both 512×512 and 2048×2048 acquisitions). The spatial resolution gain was slightly lower when the matrix size changed from 1,024×1,024 to 2048×2048 (7.9% increase in TTF10% values) compared to the 512×512 to 1,024×1,024 change (10.6% increase in TTF10% values) with a CTDIvol-32 cm of 27.9 mGy (Figure 3B).
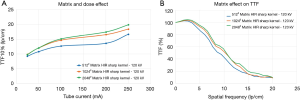
Figure 4 demonstrates the potential influence of tube output and matrix settings on the image aspect of bone in a cadaveric knee.
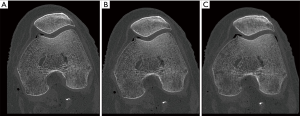
Reconstruction algorithm effects
Overall, the reconstruction algorithm that yielded the best spatial resolution was MBIR, followed by HIR with the sharp bone kernel and, finally, HIR with the standard bone kernel. The reconstruction algorithm’s effect on TTF10% values was also dose-dependent, but as opposed to the matrix effect, these benefits were most important at low doses (Figure 5). With a CTDIvol-32 cm of 2.8 mGy (120 KVp and 25 mA), changing the reconstruction algorithm from HIR with a standard bone kernel to MBIR led to a 93.3% increase in TTF10% values (from 7.6 to 14.8 lp/cm, respectively). At the highest dose level (CTDIvol-32 cm 27.9 mGy, 120 KVp, 250 mA), changing the reconstruction algorithm from HIR with a standard bone kernel to MBIR evaluated led to a lower TTF10% increase of 55.3%. However, regardless of the dose, there was a visual gain in spatial resolution of 4 lp/cm (from 14 to 18 lp/cm at the highest dose setting and from 9 to 13 lp/cm at the lowest; Figure 6). Changing from a standard to a sharp bone kernel using HIR also increased TTF10% values from 26.8% to 36.2% (from 2.3 to 4.9 lp/cm), depending on the dose. Changing HIR with a sharp bone kernel to MBIR also led to an increase in TTF10% values varying from 14% to 48.4% depending on the dose.
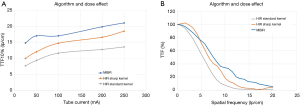
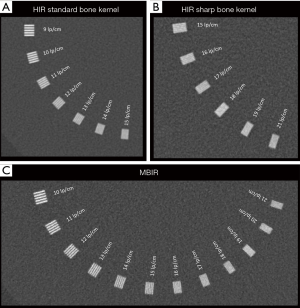
Figure 7 demonstrates the reconstruction algorithm’s potential influence on the image aspect of bone in a cadaveric knee.
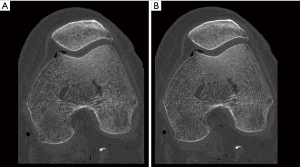
Slice thickness effect
Decreasing the acquisition slice thickness (collimation) from 0.5 to 0.25 mm, improved the “through-plane” spatial resolution. The maximal spatial resolution gain was seen with the thickest reconstruction slice thickness (0.8 mm) with a visual increase of 3 lp/cm (from 17 to 20 lp/cm), and an improvement of 18.1% TTF10% values was noted (Figure 8). With the thinnest reconstruction slice thickness (0.2 mm), the gain in visually detected line pairs was only 1 lp/cm. Varying the acquisition slice thickness had no impact on the “in-plane” spatial resolution (no change in the number of discernable line pairs and no significative variation on TTF10%).
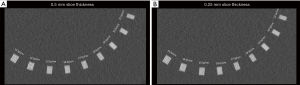
Focal spot effect
Changing the focal spot size from 0.4×0.5 to 0.8×1.3 mm2 had a modest influence on spatial resolution with no perceptible effect on the number of line pairs per centimeter and a 24% TTF10% variation. Among the focal spot sizes evaluated, the most important spatial resolution gain was noted when the focal spot changed from 0.6×0.6 to 0.6×1.3 mm2 (19.6%).
Based on these data, the order of importance in terms of spatial resolution gain of the parameters evaluated and acquisition protocol recommendations are presented in Table 2.
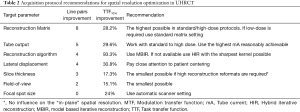
Full table
Discussion
The factor that most intensely influenced spatial resolution was the matrix size (additional visualization of up to 8 lp/cm). This effect, however, was dose-dependent and was practically nullified at the lowest dose level (CTDIvol-32 cm of 2.8 mGy). Indeed, the second most important factor affecting spatial resolution was the tube output (29.6% TTF10% gain and 5 additional lp/cm visualized), followed by the reconstruction algorithm choice (up to 93.3% TTF10% gain and 4 additional lp/cm visualized). Even though physically, the delivered dose is not directly liked to spatial resolution, we hypothesize the dose effect on spatial resolution might be linked to image noise level and reconstruction algorithm performance. This hypothesis may also explain the dose dependency of the matrix effect. A concomitant increase matrix size and tube output reduction may increase image noise to a level that cannot be compensated by the iterative reconstruction algorithm leading to a spatial resolution loss. Although various studies previously reported the spatial resolution benefits of MBIR algorithms, the weight of the reconstruction algorithm choice compared to other factors affecting spatial resolution was not thoroughly reported (20). The data presented also confirms that an increase in the delivered dose is currently required to achieve the maximal spatial resolution potential of UHRCT. Part of this dose increase requirement might likely be reduced or even suppressed by further improvements in iterative reconstruction algorithms and detector technology such as deep learning-based iterative reconstruction (3,21,22). Meanwhile, the AHARA (as high as reasonably achievable) principle should be advocated to optimize spatial resolution with UHRCT being well suited for evaluating peripheral joints, which have low radiosensitivity (23).
Patient centering, often neglected in clinical practice, was the fourth most important factor affecting spatial resolution. This effect is likely related to the fact that butterfly filter geometry is better suited for evaluating round or elliptical objects at the center of the gantry, leading to an increase in image noise at the gantry periphery (24,25). Additionally, it is likely that with a constant number of projections, lateral off-centering reduces the angular sampling, further increasing image noise. Finally, the importance of patient centering in optimizing patient dose and image quality has received recent attention in the literature and underscores the benefit of scanner models offering lateral table displacement possibilities and automatic patient positioning systems (24-26). Moreover, the dose-effect on spatial resolution was greater than that of the focal spot size. Thus, using the automatically selected focal spot size selected by the scanner software (e.g., the smallest possible based on the used tube potential, current, scan mode [helical versus sequential], and Z-axis coverage) is likely the best option (as opposed to manually selecting small focal spots and reducing gantry rotation speeds to allow higher dose delivery) (27).
Although the reduced detector element sizes in UHRCT produce a considerable increase in spatial resolution than standard CT scanners, reducing the slice thickness from 0.5 to 0.25 mm had no noticeable impact on the “in-plane” spatial resolution (8). The benefit of reducing the acquisition slice thicknesses to 0.25 mm was only seen on reformats (“through-plane” spatial resolution) and was maximal with higher reconstruction slice thicknesses (0.8 mm). Given the 2-fold increase in data volume associated with reducing the slice thickness down to 0.25 mm, studies with large Z-axis coverage requirements may generate massive amounts of data (particularly if large matrix sizes are used). Given current CT scanner-PACS (picture archiving and communication system) data transfer and storage capabilities, 0.25 mm thickness slices are more suitable for centered acquisitions when high resolution multiplanar reformats are required (e.g., fine trabecular bone and microvasculature analysis).
Using higher matrix sizes with the appropriate dose setting led to a considerable increased spatial resolution. However, in clinical practice, the benefit of larger matrix sizes is highly dependent on the acquisition FOV and zooming effect. The benefit of higher matrices basically translates to having the capacity to zoom in on an image without losing detail. This is particularly useful in acquisitions with a larger FOV, allowing a detailed analysis of smaller structures. However, Zooming is of less importance for centered acquisitions with small FOV (e.g., visual information is already accessible directly). Moreover, reducing the FOV led to a higher increase in spatial resolution (line pairs per centimeter) than increasing the acquisition matrix, with no change in the image size. Thus, the clinical context and the anatomic region evaluated should also be considered for UHRCT protocol optimization.
Some limitations of this study should be acknowledged. Although the most clinically important and user-accessible factors influencing spatial resolution were assessed, various additional factors that affect spatial resolution on CT were not evaluated (e.g., acquisition mode—helical versus sequential, vertical off-centering, tube rotation speed, etc.). Other factors influencing image quality, such as low-contrast detectability, noise, texture, which are of secondary importance for analyzing structures with high intrinsic contrast, were not evaluated in this study. A contrast dependence of MBIR on spatial resolution has also been reported and was also not assessed (28). Deep learning-based image reconstruction algorithms could reduce the dose requirements for optimal spatial resolution; however, such algorithms were not evaluated in this study. The clinical impact of UHRCT was also not assessed in this study. Given the size of the used phantom, the presented results are better suited for peripheral joints. Further studies are necessary to evaluate the influence of the evaluated factors on central joints. Finally, as optimal spatial resolution still requires moderate to high dose levels, further studies are necessary to assess these dose requirements’ risk/benefit in specific clinical scenarios.
Conclusions
Hence, the presented results led to evidence-based recommendations that can help optimize the spatial resolution of UHRCT for the evaluation of joints. Although UHRCT scanners have a great potential to improve spatial resolution, factors such as matrix size, dose, reconstruction algorithm, and patient centering are paramount and likely interrelated. Neglecting protocol optimization may considerably limit the spatial resolution gain produced by these scanner models.
Acknowledgments
I would like to thank the CT technician team of our department and particularly Mr. Benzid Nouredine for their support in the data acquisition process of this work.
Funding: None.
Footnote
Conflicts of Interest: All authors have completed the ICMJE uniform disclosure form (available at http://dx.doi.org/10.21037/qims-21-217). Two authors involved in this work (P.A.G.T and A.B.) participate in a non-remunerated research contract with Canon Medical Systems, manufacturer of the CT scanner used in this study. The other authors have no conflicts of interest to disclose.
Ethical Statement: The authors are accountable for all aspects of the work in ensuring that questions related to the accuracy or integrity of any part of the work are appropriately investigated and resolved. Since this study was performed on phantom and fully anonymized cadaveric specimens written informed consent and ethical approval were not required, and the terms of the declaration of Helsinki do not apply.
Open Access Statement: This is an Open Access article distributed in accordance with the Creative Commons Attribution-NonCommercial-NoDerivs 4.0 International License (CC BY-NC-ND 4.0), which permits the non-commercial replication and distribution of the article with the strict proviso that no changes or edits are made and the original work is properly cited (including links to both the formal publication through the relevant DOI and the license). See: https://creativecommons.org/licenses/by-nc-nd/4.0/.
References
- Wildberger JE, Prokop M. Hounsfield’s Legacy. Invest Radiol 2020;55:556-8. [Crossref] [PubMed]
- Alkadhi H, Euler A. The Future of Computed Tomography: Personalized, Functional, and Precise. Invest Radiol 2020;55:545-55. [Crossref] [PubMed]
- Lell MM, Kachelrieß M. Recent and Upcoming Technological Developments in Computed Tomography: High Speed, Low Dose, Deep Learning, Multienergy. Invest Radiol 2020;55:8-19. [Crossref] [PubMed]
- Stok KS, Burghardt AJ, Boutroy S, Peters MPH, Manske SL, Stadelmann V, Vilayphiou N, Van den Bergh JP, Geusens P, Li X, Marotte H, Van Rietbergen B, Boyd S, Barnabe C. SPECTRA Collaboration. Consensus approach for 3D joint space width of metacarpophalangeal joints of rheumatoid arthritis patients using high-resolution peripheral quantitative computed tomography. Quant Imaging Med Surg 2020;10:314-25. [Crossref] [PubMed]
- Zhu Y, Hou D, Lan M, Sun X, Ma X. A comparison of ultra-high-resolution CT target scan versus conventional CT target reconstruction in the evaluation of ground-glass-nodule-like lung adenocarcinoma. Quant Imaging Med Surg 2019;9:1087-94. [Crossref] [PubMed]
- Si-Mohamed S, Boccalini S, Rodesch PA, Dessouky R, Lahoud E, Broussaud T, Sigovan M, Gamondes D, Coulon P, Yagil Y, Boussel L, Douek P. Feasibility of lung imaging with a large field-of-view spectral photon-counting CT system. Diagn Interv Imaging 2021;102:305-12. [Crossref] [PubMed]
- Blum A, Gillet R, Rauch A, Urbaneja A, Biouichi H, Dodin G, Germain E, Lombard C, Jacquet P, Louis M, Simon L, Gondim Teixeira P. 3D reconstructions, 4D imaging and postprocessing with CT in musculoskeletal disorders: Past, present and future. Diagn Interv Imaging 2020;101:693-705. [Crossref] [PubMed]
- Oostveen LJ, Boedeker KL, Brink M, Prokop M, de Lange F, Sechopoulos I. Physical evaluation of an ultra-high-resolution CT scanner. Eur Radiol 2020;30:2552-60. Erratum in: Eur Radiol 2020;30:4709-10. [Crossref] [PubMed]
- Kakinuma R, Moriyama N, Muramatsu Y, Gomi S, Suzuki M, Nagasawa H, Kusumoto M, Aso T, Muramatsu Y, Tsuchida T, Tsuta K, Maeshima AM, Tochigi N, Watanabe S, Sugihara N, Tsukagoshi S, Saito Y, Kazama M, Ashizawa K, Awai K, Honda O, Ishikawa H, Koizumi N, Komoto D, Moriya H, Oda S, Oshiro Y, Yanagawa M, Tomiyama N, Asamura H. Ultra-High-Resolution Computed Tomography of the Lung: Image Quality of a Prototype Scanner. PLoS One 2015;10:e0137165 [Crossref] [PubMed]
- Willemink MJ, Persson M, Pourmorteza A, Pelc NJ, Fleischmann D. Photon-counting CT: Technical Principles and Clinical Prospects. Radiology 2018;289:293-312. [Crossref] [PubMed]
- Rajendran K, Murthy NS, Frick MA, Tao S, Unger MD, LaVallee KT, Larson NB, Leng S, Maus T, McCollough C. Quantitative Knee Arthrography in a Large Animal Model of Osteoarthritis Using Photon-Counting Detector CT. Invest Radiol 2020;55:349-56. [Crossref] [PubMed]
- Yanagawa M, Hata A, Honda O, Kikuchi N, Miyata T, Uranishi A, Tsukagoshi S, Tomiyama N. Subjective and objective comparisons of image quality between ultra-high-resolution CT and conventional area detector CT in phantoms and cadaveric human lungs. Eur Radiol 2018;28:5060-8. [Crossref] [PubMed]
- Klepper R, Johner C, Hesch RD. ROFO Fortschr Geb Rontgenstr Nuklearmed 1993;159:337-42. [A structural parameter for characterizing early trabecular changes in the spine in osteoporosis]. [Crossref] [PubMed]
- Nilsson M, Sundh D, Mellström D, Lorentzon M. Current Physical Activity Is Independently Associated With Cortical Bone Size and Bone Strength in Elderly Swedish Women. J Bone Miner Res 2017;32:473-85. [Crossref] [PubMed]
- Meijer FJA, Schuijf JD, de Vries J, Boogaarts HD, van der Woude WJ, Prokop M. Ultra-high-resolution subtraction CT angiography in the follow-up of treated intracranial aneurysms. Insights Imaging 2019;10:2. [Crossref] [PubMed]
- Yaniv G, Shabshin N, Sharon M, Liberman B, Garniack A, Rimon U, Eshed I. Osteoid osteoma--the CT vessel sign. Skeletal Radiol 2011;40:1311-4. [Crossref] [PubMed]
- Buijze GA, Bachoura A, Mahmood B, Wolfe SW, Osterman AL, Jupiter JB. Reevaluation of the Scaphoid Fracture: What Is the Current Best Evidence? Instr Course Lect 2020;69:317-30. [PubMed]
- Campbell GM, Glüer C-C. Skeletal assessment with finite element analysis: relevance, pitfalls and interpretation. Curr Opin Rheumatol 2017;29:402-9. [Crossref] [PubMed]
- Samei E, Bakalyar D, Boedeker KL, Brady S, Fan J, Leng S, Myers KJ, Popescu L, Ramirez Giraldo JC, Ranallo F, Solomon J, Vaishnav J, Wang J. Performance evaluation of computed tomography systems: Summary of AAPM Task Group 233. Med Phys 2019;46:e735-56. [Crossref] [PubMed]
- Laurent G, Villani N, Hossu G, Rauch A, Noël A, Blum A, Gondim Teixeira PA. Full model-based iterative reconstruction (MBIR) in abdominal CT increases objective image quality, but decreases subjective acceptance. Eur Radiol 2019;29:4016-25. [Crossref] [PubMed]
- Matsukiyo R, Ohno Y, Matsuyama T, Nagata H, Kimata H, Ito Y, Ogawa Y, Murayama K, Kato R, Toyama H. Deep learning-based and hybrid-type iterative reconstructions for CT: comparison of capability for quantitative and qualitative image quality improvements and small vessel evaluation at dynamic CE-abdominal CT with ultra-high and standard resolutions. Jpn J Radiol 2021;39:186-197. [Crossref] [PubMed]
- Park C, Choo KS, Jung Y, Jeong HS, Hwang JY, Yun MS. CT iterative vs deep learning reconstruction: comparison of noise and sharpness. Eur Radiol 2021;31:3156-64. [Crossref] [PubMed]
- Thrall JH. Radiation exposure in CT scanning and risk: where are we? Radiology 2012;264:325-8. [Crossref] [PubMed]
- Shaffiq Said Rahmat SM, Abdul Karim MK, Che Isa IN, Abd Rahman MA, Noor NM, Hoong NK. Effect of miscentering and low-dose protocols on contrast resolution in computed tomography head examination. Comput Biol Med 2020;123:103840 [Crossref] [PubMed]
- Euler A, Saltybaeva N, Alkadhi H. How patient off-centering impacts organ dose and image noise in pediatric head and thoracoabdominal CT. Eur Radiol 2019;29:6790-3. [Crossref] [PubMed]
- Saltybaeva N, Schmidt B, Wimmer A, Flohr T, Alkadhi H. Precise and Automatic Patient Positioning in Computed Tomography: Avatar Modeling of the Patient Surface Using a 3-Dimensional Camera. Invest Radiol 2018;53:641-6. [Crossref] [PubMed]
- Wang J, Fleischmann D. Improving Spatial Resolution at CT: Development, Benefits, and Pitfalls. Radiology 2018;289:261-2. [Crossref] [PubMed]
- Richard S, Husarik DB, Yadava G, Murphy SN, Samei E. Towards task-based assessment of CT performance: system and object MTF across different reconstruction algorithms. Med Phys 2012;39:4115-22. [Crossref] [PubMed]