Translating state-of-the-art brain magnetic resonance imaging (MRI) techniques into clinical practice: multimodal MRI differentiates dementia subtypes in a traditional clinical setting
Introduction
Alzheimer’s disease and Parkinson’s disease both yield significant rates of neurocognitive disorders, including in adults younger than 65 years of age (1). Differentiating the neurocognitive disorders that arise from these diseases is imprecise and confounded by overlapping pathological biomarkers (2,3). Developing accurate diagnostic procedures, particularly in early stages of the diseases, is critically important for the early implementation of assistive treatments as well as for the development of novel treatments (4). Therefore, non-invasive neuroimaging methods such as magnetic resonance imaging (MRI) may be beneficial if objective diagnostic information could be attained from differential brain metrics. Statistical inference aided diagnoses using MRI data may use subtle group differences and novel data combinations to render more accurate diagnoses than that which might otherwise be attained using clinical appraisal alone (5).
Advanced MRI techniques are commonly used in research and are gradually entering into clinical practice. Current examples include functional MRI (fMRI), which is used in presurgical language mapping with ever-increasing prevalence over the past 15 years (1,2). More recently, programs for quantitative statistical analysis of structural volumetric data have been FDA-approved and used in clinical practice (e.g., Icometrix, NeuroQuant, NeuroReader). In addition to these, numerous other MR modalities and analysis methods (e.g., statistical comparison) which are commonly used in research could contribute more frequently to clinical patient evaluation.
Previous publications have postulated that well-established neuroanatomical underpinnings of psychiatric and neurologic disorders, such as abnormal neural circuitry, warrant the clinical use of advanced, multimodal MRI (3,4). However, clinical adoption of these and other advanced MR techniques has been slow and limited to niche applications. A recent survey found that while 90% of responding clinicians had access to fMRI acquisition tools (a majority of these had previous experience using fMRI in a research setting), only 40% intended to use fMRI clinically in the next year; most of the clinical utility was noted for presurgical planning (6). Reported barriers to the clinical use of fMRI included reimbursement rates, limited training opportunities, methodological concerns about the intensity of data processing and the consistent reliability of results (6). Traditional clinical MRI involves structural and diffusion-weighted imaging; less commonly, clinical MRI may also include sequences for brain perfusion and metabolite analysis, but even these are generally acquired without standardized statistical analyses.
One of the primary referral requests to adult and geriatric neurology and neuropsychology clinics is to differentiate healthy aging from neurodegenerative disorders. Two of the more common neurodegenerative subtypes are Alzheimer’s disease, characterized primarily by degeneration in medial temporal lobe regions in those with amnestic presentations, and Parkinson’s disease dementia (PDD), characterized primarily by degeneration in basal ganglia and frontal lobe circuits (7). In order to be a helpful diagnostic tool in this setting, multimodal MRI and statistics must be able to reliably differentiate “worried-well” adults who are experiencing healthy aging from patients undergoing a true neurodegenerative process. Furthermore, these tools will ideally be able to differentiate between various types of neurodegenerative processes, preferably earlier in the disease process.
Toward this end, several important studies have investigated such tools for differentiation of neurodegenerative disease (NDD) within large, publicly available datasets (6-11). In smaller participant pools, support vector regressions have been trained to differentiate neurodegenerative conditions from healthy aging using standard clinical structural MRI (12-14); however, this work must be expanded to evaluate for the clinical utility of other nontraditional MRI modalities which currently exist solely in the research space. Our group sought to validate the capabilities of each MRI modality to successfully differentiate patients with NDD, classified based on the clinical gold-standard methods, from healthy individuals. This was conducted using discriminant function analysis-based classification of the data derived from each MR modality. Algorithmic classification attempted to determine which gold-standard diagnostic classification each participant fit into based on data from each MRI modality. Additionally, given that clinical neuroimaging is largely rater-review-based, this statistical classification was compared to classification of each image by trained raters, again comparing their rating to the gold-standard diagnostic classification. This was performed by two trained readers who reviewed and assigned a diagnostic classification to each image (similar to the way traditional neuroradiological reading is conducted). While additional neuroimaging techniques warrant similar investigations, positron emission tomography (PET) and single photon emission computed tomography (SPECT) techniques were not included in this study due to the invasive and radiologic nature of these modalities, their limited clinical availability, and the relative financial burden of these measures. By attempting to validate the clinical utility of each MR modality in a neurologic patient group, we hope to provide some preliminary insight into the potential use of both multimodal MR and advanced statistical analysis for improving diagnostic and prognostic utility.
Methods
Participants
All procedures were carried out in accordance with the Declaration of Helsinki (as revised in 2013) and were reviewed and approved by the Western Institutional Review Board prior to participant enrollment (Pro #20152535). All participants provided written informed consent. Due to in-scanner motion and artifact in the MR data, three patients with Alzheimer’s disease [dementia of the Alzheimer’s type (DAT)] and four patients with PDD were excluded from the analyses. Thereafter, twenty DAT patients (Mage =78.33±5.98 years, 46% female) were identified using gold-standard lumbar puncture amyloid-beta and tau markers (15). Eighteen PDD patients (Mage =76.43±9.33 years, 52% female) were identified using comprehensive neurologic and neuropsychological evaluations, including assessment of motor symptoms, neurocognitive profile and genetic evaluation. All diagnoses of neurocognitive disorders were rendered by consensus of an expert panel consisting of board-certified neurologists, neuropsychologists, radiologists, and psychiatrists. To avoid circularity in the study design, advanced MRI was not used for the purposes of initial screening or diagnosis. All participants were administered the Montreal Cognitive Assessment (MoCA) and the Repeatable Battery Assessment of Neuropsychological Status (RBANS). Twenty-three older adults (Mage =80.1±8.8 years, 48% female) who were considered normal-aging (i.e., without a history remarkable for neurologic, cognitive, or psychiatric disorder, including seizure, head injury with associated loss of consciousness, chemotherapy or radiation treatment, neoplasm, alcohol or substance use disorder, and subjective concern) were also recruited for this study. These normal-aging adults were determined to have MoCA scores ≥28, RBANS domain-specific and global scores in the “average” range or higher. These normal-aging adults were age and sex matched to the two NDD groups. Analysis of variance (ANOVA), corrected for multiple comparison using Bonferroni method, assessed for differences in demographic and neurocognitive factors between the three diagnostic groups. Chi-square analysis was used for categorical data comparison [e.g., clinical dementia rating (CDR) level].
MRI acquisition
MR data was collected using a 1.5T Siemens Espree scanner (Siemens, Germany) at a Los Angeles Clinical MRI Center.
T1-data was collected as a sagittal magnetization-prepared rapid gradient-echo (MPRAGE) sequence with repetition time (TR) =1,600 ms, echo time (TE) =3 ms, voxel size =1.0 mm × 1.0 mm × 1.0 mm, acquisition dimensions =192×256×256, scan time 270 s. Pulsed arterial spin labelling (ASL) was collected as an echo planar sequence with TR =3,400 ms, TE =16 ms, voxel size =4.0 mm × 4.0 mm × 6.0 mm, acquisition dimensions =64×64×26, with 121 measurements, bolus time =700 ms, inversion time =1,800 ms, scan time =411 s. Resting BOLD data was collected as an echo planar sequence with TR =2,500 ms, TE =50 ms, voxel size =4.0 mm × 4.0 mm × 4.0 mm, acquisition dimensions =64×64×36, 200 measurements, scan time =500 s. Twenty diffusion-weighted volumes (b =1,000 s/mm2) and two non-diffusion-weighted volumes were obtained using a single shot spin-echo echo planar imaging (EPI) sequence with flip angle =90, TR =11,700 ms, TE =127 ms, voxel size =2.0 mm ×2.0 mm × 2.0 mm, acquisition dimensions =150×150×64, b-shells =0, 1,000, scan time 737 s.
MRI analysis
All MRI datasets were quality controlled and visually inspected prior to being preprocessed and analyzed. All imaging data were processed using FMRIB Software Library [FSL, www.fmrib.ox.ac.uk.fsl; (16)].
Structural image processing
T1 images were corrected for field biasing. Then, using FMRIB’s Automated Segmentation Tool (FAST), T1 images were then skull-stripped and linearly registered to standard MNI space (17,18). Each patient’s T1 image was segmented into 100 cortical and 15 subcortical areas using the Harvard-Oxford Cortical and Subcortical structural atlas in conjunction with fslmaths (19). Mean volume was computed for each of these regions for each patient with fslstats (19).
ASL processing
ASL data was processed and analyzed using FSLs Bayesian Inference for ASL MRI [BASIL; (19)]. Briefly, all images were co-registered to the first volume; perfusion difference was then calculated (subtracting tagged/control pairs) and averaged to create a mean perfusion-weighted image. Quantification to cerebral blood flow (CBF) values (milliliters of blood per 100 g of tissue per minute) was implemented using an estimate of the equilibrium magnetization of arterial blood and the mean perfusion values (19). Given the variability of ASL across subjects, CBF data was examined as a ratio to mean grey matter signal.
Diffusion imaging processing
Diffusion weighted imaging (DWI) data was motion and eddy current corrected, skull stripped using BET, and then diffusion tensors were fit to the data using dtifit in FSL. Tract-Based Spatial Statistics (TBSS) was used to generate a white matter (WM) skeleton comprised of WM voxels shared by all participants (20). This WM skeleton was applied to each participant’s individual diffusion tensor imaging (DTI) maps and mean fractional anisotropy (FA), axial (AD), radial (RD) and mean (MD) diffusivity were extracted from various regions of interest (ROIs) based on the intersection between the TBSS skeleton and labels defined in probabilistic anatomical atlas in addition to a global average across the skeleton (20). This atlas included twenty ROIs from which DTI metrics were extracted: anterior thalamic radiation, corticospinal tract, cingulate bundle, WM underlying the hippocampus, inferior longitudinal fasciculus, inferior fronto-occipital fasciculus, superior longitudinal fasciculus, arcuate fasciculus, forceps major, forceps minor and the uncinate fasciculus. DTI metrics were also calculated from the entire WM skeleton.
Magnetic resonance spectroscopy (MRS) processing
MRS data was processed using Osirix (https://www.osirix-viewer.com/osirix/osirix-md/) and Tarquin (http://tarquin.sourceforge.net/) in order to calculate neurotransmitter specific peak heights from which neurotransmitter comparison ratios [e.g., N-acetylaspartate to glutamine (NAA/Gl)] were computed. MRS values were creatine adjusted to improve the likelihood that data was derived from tissue and not cerebrospinal fluid (CSF). For seeds acquired in the frontal lobe and the precuneus, NAA/Gl ratio, glutamate-glutamine (Glx) ratio and choline/creatine (Cho/Cr) ratio were calculated.
Trained rater review
Given that current clinical neuroimaging typically involves subjective review of images by a trained rater, this study included two trained raters who attempted to classify study participants into the correct diagnostic category (e.g., DAT, typically aging) using each of the multimodal imaging modalities (21,22).
Two trained raters (SE Jordan, 40-year experience as a board-certified clinical neurologist; T Kuhn, 10-year experience as a neuroimaging researcher and neuropsychologist) reviewed 6 unblinded participants (3 patients, 3 healthy controls) in order to determine uniform classification criteria to use when reviewing blinded scans to determine whether each scan was that of a control participant or a patient with a neurodegenerative disorder, as previously determined by the gold standard. For structural T1 images, this included visual assessment of structural images for gross and regional atrophy including evaluation of specific subregions including the hippocampus, basal ganglia and gyral anatomy. For ASL, this involved visual assessment of global and regional perfusion (e.g., basal ganglia, frontal, temporal and parietal lobe) with additional evaluation of potential hemispheric asymmetry in perfusion for DTI, this included visual assessment of DWI images as well as statistical review of FA, MD, AD and RD values which were calculated for twenty regions, based on the Johns Hopkins DTI Atlas. For these metrics, participants were compared to see if any fell above the mean ± three standard deviations.
For MRS, the spectroscopy curve was reviewed and ratios were calculated, particularly for N-acetylaspartate to creatine (NAA/Cr) and glutamate/glutamine-to-NAA peak as collected from the hippocampus, precuneus and right frontal lobe. Values were compared to see if any fell above the mean ± three standard deviations. Values were also compared to standard clinical protocols (e.g., 3/1 NAA/Cr ratio).
Each rater classified each image in a discrete fashion (i.e., 0/1) as that of a healthy control or patient with neurodegenerative disorder. This was done in a blinded fashion such that neither rater was aware of the other rater’s classification of the scans. Thereafter, using each rater’s classifications, a third investigator computed sensitivity, specificity, positive and negative predictive value (NPV) and agreement between raters.
Statistical analysis
Discriminant function analyses are a form of statistical analysis which can be used to determine which, if any, variables can be used to discriminate data between two or more groups (22-25). Discriminant function classification analyses determine the probability that an individual fits a certain predetermined group (i.e., normal aging or neurodegenerative condition) by determining the relative distance between each individual’s data and the centroid of the group (i.e., Mahalanobis distance). By conducting these analyses in an iterative fashion, the results can be improved. Therefore, iterative discriminant function analyses were conducted to determine the utility of regional volumetrics, regional FA/MD/AD/RD, regional perfusion, and regional MRS ratios to differentiate (I) healthy participants from neurological patients; (II) healthy participants, Alzheimer’s and Parkinson’s groups. Discriminant ability as well as specificity, sensitivity, Youden’s index, positive and NPV were computed for each modality. Youden’s index is a single metric representing performance of a diagnostic test. It is calculated as:
Youden’s index the height above the change line at the inflection point of a receiver operating curve and defines the probability of an informed decision based on a classifying cut-off point, taking into account a range of predictions. Therefore, it is well suited for classification analyses with empirically-derived cut-off points for group differentiation.
Additionally, a stepwise linear regression can be incorporated into the analysis to remove any variables entered into the model which do not meaningfully contribute to the model’s ability to discriminate data into groups. In this way, the stepwise regression serves a feature reducing function (22). Thus, for both two- and three-group analyses within each modality, we re-ran separate discriminant function analyses incorporating a secondary hierarchical regression feature reduction technique to determine the fewest number of regional metrics necessary to successfully delineate group membership (25). This regression is incorporated directly into the classification analysis and as such the same cross-validation applies to both discriminant function analyses, with and without the feature-reducing regression.
All discriminant function analyses were evaluated for collinearity and over-fitting. A leave-one-out cross validation step was included in each analysis to account for overfitting. This method involved cross-validation using a number of folds equivalent to the data set sample size wherein the discriminant function was applied to each participant data as a single-item test set predicting each participant’s diagnostic classification based on a model trained using the remaining data as the training set. Variables included as predictors in the final models of both the full and feature-reduced classification analyses were required to pass tolerance and variance inflation factor (VIF) thresholds.
Sensitivity, specificity, Youden’s index, positive and negative predictive power and interrater agreement were computed based on the results of the trained rater identification of the patients.
For classification analyses, iterative discriminant function analyses were conducted to determine the utility of regional volumetrics, regional FA/MD/AD/RD, regional perfusion, and MRS ratios to differentiate (I) healthy participants from neurological patients; (II) healthy participants, Alzheimer’s and Parkinson’s groups. For the three-group analysis, a one-to-one strategy was implemented. Discriminant ability as well as specificity, sensitivity, Youden’s index, positive and NPV were computed for each modality. These same analyses were also conducted using whole brain metrics from each imaging modality, allowing for comparison of the utility of whole brain versus regional metrics for diagnostic accuracy.
Results
Participants
The participants with neurodegenerative conditions did not differ on general cognitive ability (RBANS Total, P>0.05; DAT RBANS: 83.67±16.82; PDD RBANS: 90.8±21.04). Neurodegenerative diagnostic groups also did not differ on dementia severity as determined by the CDR scale (P>0.05). The PDD group was comprised of 44% mild cognitive impairment (CDR =0.5), 44% mild dementia (CDR =1) and 11% moderate (CDR =2). Similarly, the DAT group was comprised of 46% mild cognitive impairment (CDR =0.5), 39% mild dementia (CDR =1), and 16% moderate-to-severe dementia (8% CDR =2, 8% CDR =3).
Inter-rater classification
All 68 participants (DAT =23, PDD =22, controls =23) completed the protocol, although seven scans were excluded due to artifact or excessive motion. Trained raters classified each image as that of a healthy control or patient with neurodegenerative disorder (combined rater results, Table 1; Figure 1). ASL and MRS demonstrated excellent sensitivity, specificity, positive predictive value (PPV) and NPV compared to gold-standard diagnosis. Please see Table 1 for a review of the inter-rater classification metrics for each modality. T1-based structural images displayed the highest interrater reliability (i.e., agreement). Please see Table S1 for review of quantitative DTI and MRS values used for analyses.
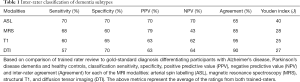
Full table
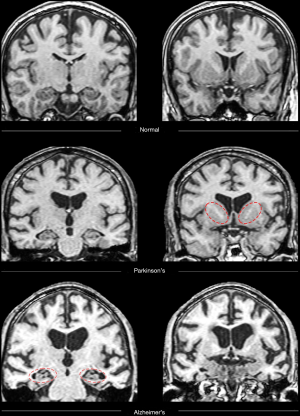
Classification analysis
Iterative discriminant function analyses were conducted to attempt to accurately differentiate participants into disease categories (A: healthy vs. neurodegenerative disorder; B: healthy vs. DAT vs. PDD). Cross-validation was successfully conducted for all models. Overall model accuracy, sensitivity, specificity, Youden’s index, positive and negative predictive power were computed based on the results of the discriminant function classification of the patients in four schemes: healthy vs. neurodegenerative disorder using all available data (Table 2), healthy vs. DAT vs. PDD using all available data (Table 3), healthy vs. neurodegenerative disorder using data reduction (Table 4), and healthy vs. DAT vs. PDD using data reduction (Table 5).
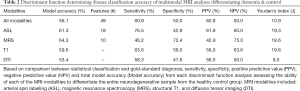
Full table
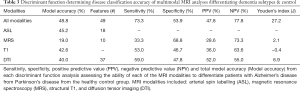
Full table
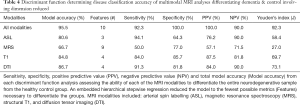
Full table
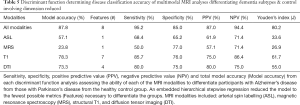
Full table
Discriminant function classification using feature reduction successfully differentiated diagnostic groups with excellent accuracy using T1, DTI, and ASL independently, as well as when all modalities were combined into a single model. Using the full model with all available data, neither diagnostic classification model was able to accurately differentiate healthy from neurodegenerative disorder patients using MRS values or MRS ratios, though specificity was substantially higher than sensitivity. Feature reduction did not successfully improve either MRS model. Additionally, when all metrics were available to the model, model accuracy and predictive power were substantially worse than when feature selection was used, despite using tolerance thresholds to correct for collinearity. Feature reduction resulted in the following regions driving separation of neurodegenerative conditions from controls: volume of the left frontal pole, right posterior superior temporal gyrus, left posterior cingulate gyrus, perfusion of the left hippocampus and left occipital lobe; FA of the forceps major and bilateral anterior thalamic radiation.
Discriminant function classification successfully differentiated neurodegenerative disorder from control groups following feature reduction. Using the following ten metrics, excellent separation (95.5% accuracy, 92.3% sensitivity; 100.0% specificity) was achieved: volume of the left frontal pole, left occipital pole, right posterior superior temporal gyrus, left posterior cingulate gyrus, right planum temporale; perfusion of the left hippocampus and left occipital lobe; FA of the forceps major and bilateral anterior thalamic radiation. No MRS metrics were included in the final model. Interestingly, the multimodality model without feature reduction yielded relatively poor classification accuracy.
Structural image classification
Using structural T1 data following dimension reduction, neurologic and control participants could be differentiated with 84.8% accuracy using four regional volumes: right posterior supramarginal gyrus, right fusiform gyrus, left middle frontal gyrus and right occipital pole. Also using T1, DAT, PDD and control participants were grouped with 78.3% accuracy using 7 regional volumes: right posterior supramarginal gyrus, right pallidum, right occipital pole, right anterior cingulate gyrus, right posterior inferior temporal gyrus, left superior lateral occipital gyrus and left postcentral gyrus.
Diffusion image classification
Using DTI data following feature reduction regressions, neurologic and control participants could be differentiated with 86.7% accuracy using four regional DTI metrics: FA of the forceps major, MD of the right superior longitudinal fasciculus, RD of the right inferior longitudinal fasciculus and uncinate fasciculus. Further, DAT, PDD and control participants were grouped with 73.3% accuracy using four regional DTI metrics: FA of the forceps major and right uncinate fasciculus, AD of the left cingulate bundle and RD of the WM underlying the left hippocampus. When including all 75 DTI variables in the model, 38 failed the tolerance threshold test, resulting in a final model including 37 metrics which yielded poor accuracy, sensitivity and specificity.
ASL classification
Using ASL data following dimension reduction, healthy controls and patients with neurodegenerative disorder could be classified with 80.6% accuracy using right temporal and right parietal lobe perfusion. Dementia subtypes could be differentiated from healthy participants with 57.1% accuracy using right temporal perfusion data.
MRS classification
Using MRS data following dimension reduction, healthy controls and patients with neurodegenerative disorder were classified with 66.7% accuracy. MRS was substantially more specific (77.0%) than sensitive (50.0%). Dementia subtypes were differentiated from controls with poor accuracy (19.0%), sensitivity (33.3%) and moderate specificity (68.8%).
Whole brain metric classification
FA from WM across the entire cerebrum was 71.1% successful in differentiating the neurodegenerative group from the healthy aging group and 73.3% successful in differentiating different neurodegenerative subtypes from each other and healthy aging. MD, AD and RD were excluded from the model due to multicollinearity. Whole brain (left and right hemisphere) volume data was between 58.1–72.1% successful at differentiating neurodegenerative and healthy aging groups, with the higher classification accuracy achieved with the inclusion of brainstem volume. Whole brain ASL was 47.6% accurate in differentiating the neurodegenerative group from the healthy aging group and 45.2% successful in differentiating different neurodegenerative subtypes from each other and healthy aging. Whole brain metrics from MRS could not be calculated due to the inherent single voxel nature of that data collection modality.
Differentiating DAT from PDD
We further investigated the utility of multimodal MRI to differentiate specific neurodegenerative conditions from each other. Given the improved accuracy including the feature reduction step in the model, we report only models using this tool here (Table 6). When using all available multimodal MRI data with feature reduction, DAT and PDD were successfully differentiated (95.0% accuracy) using the volume of the left angular gyrus, left precentral gyrus, right middle temporo-occipital gyrus, right planum temporale, right posterior supramarginal gyrus, and precuneus, as well as perfusion of the bilateral hippocampus and right frontal pole. Structural volumetry of the following regions was very successful at differentiating neurodegenerative subgroups (91.7% accuracy): left angular gyrus, pars orbitalis, pars triangularis, inferior temporal gyrus and occipital pole, as well as right precentral gyrus, middle temporal gyrus, anterior and posterior parahippocampal gyrus, fusiform gyrus and cuneus. FA of the left arcuate fasciculus and right uncinate fasciculus differentiated DAT from PDD with 75.0% accuracy. ASL and MRS poorly differentiated neurodegenerative groups (Figure 2).
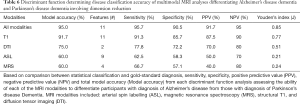
Full table
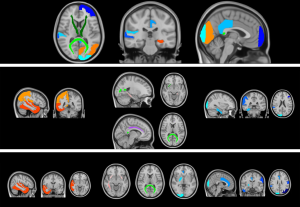
Discussion
This study sought to validate the clinical utility of multimodal MRI techniques in the assessment of neurodegenerative disorders. Discriminant function analyses were implemented to classify patients into diagnostic categories using each individual MRI modality. Using structural T1, DTI, and ASL images (each independently and with feature reduction) the algorithm was able to successfully differentiate healthy from neurodegenerative groups. Following a secondary feature reduction step, the algorithm remained able to successfully classify patients as either healthy or with a neurodegenerative disorder using structural T1 or DTI data from select brain region. This was achieved using only a small number of regions: volume of four T1 structural regions and, separately, diffusivity metrics of four DTI regions were used to classify patients successfully. Similarly, using ASL-based perfusion values for two regions, the algorithm remained able to accurately classify patients with a high degree of accuracy. Finally, an overall model including all modalities with feature reduction was able to successfully differentiate diagnostic groups using ten features. Interestingly, the multimodality model without feature reduction yielded relatively poor classification accuracy, potentially due to multicollinearity which was not sufficiently accounted for by the embedded tolerance threshold.
The same classification schedule was again implemented in order to differentiate healthy participants from patients with different neurodegenerative subtypes (DAT, PDD). Following feature reduction, all modalities remained substantively able to differentiate groups with relatively few anatomical regions. While these dimension reduction findings are important, particularly given the recent machine learning advances related to recovering data lost during MR acquisition, it is important not to over-interpret the clinical significance of the specific features themselves (i.e., regional metrics). An inherent limitation of feature selection algorithms involves the limited generalizability of those features selected by the model. Nevertheless, these regional metrics were substantially better classifiers than whole brain metrics. It is important to note that the use of an atlas (e.g., JHU atlas from which DTI metrics were derived) was based on a previous group procedure and included younger adults. Future clinical applications of this technique may want to consider hand drawn, atlas and single-subject automatic segmentation as it relates to optimizing classification accuracy. Additionally, all of these feature-reduced algorithms yielded substantially more accurate diagnoses than the trained rater qualitative review. Therefore, there appears to be clinically meaningful information in quantitative assessment of multimodal MRI derived from specific brain regions. As such, regional analyses may provide more sound diagnostic information than more global metrics. This is an important area for further investigation, particularly in larger data sets (e.g., ADNI) which may allow for ranking regional metrics in terms of incremental validity as well as potentially yield regional metrics that could be generalized to the evaluation of single, clinical patients.
Taken together, these findings provide preliminary evidence for the clinical utility of quantitative T1-based structural volumetrics, DTI and ASL. Recently, quantitative volumetric analyses have begun to be used in clinical practice (e.g., Icometrix, NeuroQuant, NeuroReader). However, to the best of our knowledge, quantitative analysis of these other multimodal imaging modalities are not yet in widespread clinical use. In order to more fully integrate these tools within the clinical space, it is important to reflect on the time and computing requirements for the techniques discussed in this study. Computing time for preprocessing, quality assuring and statistically analyzing each MRI modality ranged from 30 minutes (MRS) to 4 hours (diffusion data). This is a relatively robust processing pipeline that would need to be optimized to both finish faster and be more automated in order to allow for streamlined clinical use. While further validation in larger samples as well as among other diagnostic groups (e.g., psychiatric, traumatic brain injury) are needed, these results confirm that future work appears warranted to help further to translate state-of-the-art multimodal brain MRI techniques to clinical practice.
The brain regions which were found to be the most significant predictors of group classification appear to relate directly to systems implicated in neurodegenerative conditions. The hippocampus and entorhinal cortex are the hallmark regions associated with the initial neurodegeneration that in classical Alzheimer’s disease. Volumetric, functional connectivity and perfusion changes in the hippocampus has been shown as both biomarkers for Alzheimer’s disease progression as well as markers for the episodic memory deficits seen in these conditions (26). Additionally, patients with Alzheimer’s disease have been known to have reduced blood flow in the left occipital lobe (27). Other studies have shown that increased connectivity in the occipital pole is implicated with better cognition (28). Beyond Alzheimer’s disease, voxel-based morphometry has shown a volume loss in left occipital pole and right inferior temporal cortex in Huntington’s disease (29). The frontal pole is commonly affected across multiple neurodegenerative conditions from Alzheimer’s disease to PDD to frontotemporal lobar degeneration. Interestingly, the frontal pole has been implicated in the regulation of emotional behaviors (30) which is frequently evidenced in patients with NDD, with patients with NDD having poor emotional regulation and control (31). ASL has been shown to differentiate between Alzheimer’s disease and PDD (28). In particular, differences were seen in the medial temporal lobe, with Alzheimer’s disease having less relative perfusion than PDD, and in right frontal cortex, with PDD having less relative perfusion than AD. A separate study of volumetrics demonstrated that AD patients have the most severe atrophy of medial temporal lobe, followed by Parkinson’s and then healthy controls (32-34). Other work has shown that PDD have greater atrophy in the amygdala and hippocampus than nondemented Parkinson’s disease patients (35,36).
The caudal part of left posterior cingulate cortex is approximately BA 31. BA 31 has been known to have grey matter volume reductions early in the progression of Alzheimer’s disease (33). These effects have also been seen in patients with Parkinson’s disease and essential tremor (34). This region could perhaps play a role in the cognitive dysfunction often seen in these patient populations. Further, changes in FA of forceps major and anterior thalamic radiation have been identified in many emotional disorders (37). Thus, these changes in FA which are seen in neurodegenerative disorder patients, could be biomarkers for the emotional dysregulation that these patients often present with. Similarly, MRI studies of schizophrenia have shown bilateral decrease in grey matter volume in the posterior superior temporal gyrus (38). This could be a factor in thought disorders seen in late stage NDD patients.
Interestingly, although the least predictive classifier, MRS was substantially more specific than sensitive. This may be related to the common finding that MRS changes are very subtle in neurodegenerative conditions which limits the ability of MRS data to differentiate between diagnostic conditions (39). This may be due to the specifications of MRS employed in the present study. For example, this study used single-voxel MRS and it may be the case that multi-voxel MRS provides more meaningful clinical data for neurodegenerative patient populations. It may also be the case that other MRS metabolites not included in this study would have been more sensitive to neurodegenerative processes, such as myoinositol (MI). It is also possible that the MRS data was collected sub-optimally. For example, hippocampal MRS data was collected which is an area that is susceptible to atrophy in degenerative conditions and is therefore plagued with partial volume effects related to higher CSF content in the sample volume. However, creatine does not exist in CSF, so the creatine corrected ratios which were reviewed in this study ought to have avoided this limitation. Nevertheless, future studies may want to investigate methods for optimizing the clinical utility of MRS for patients with neurodegenerative disorder. Regardless, MRS remains a meaningful tool for grading cerebral tumors and may well be a meaningful diagnostic tool in other clinical populations such as psychiatric disorders (40,41).
Other techniques reported in the literature for diagnosing neurodegenerative conditions include other imaging modalities, clinical biomarkers (e.g., blood, plasma, lumbar puncture analysis) and neuropsychological evaluation. A metanalysis of SPECT imaging of different neurodegenerative disorder subtypes was found to have pooled sensitivities of 65.7% and specificities of 79.1% (42). Presynaptic dopaminergic imaging using 123I-FP-CIT (DATSCAN) was found in a recent metanalysis to have 86.5% sensitive and 93.6% specific in diagnosis patients with Lewy body dementia (43). Similarly, patients with mild cognitive impairment of the Lewy body type were differentiated from healthy aging participants using the DATSCAN with 54.2% sensitivity and 89.0% specificity (44). It is important to note that both of these DATSCAN studies were conducted using visual inspection of the DATSCAN imaging and in patients with Lewy body disease. The quantitative analysis of DATSCAN or other PET scans has yet to be investigated in neurodegenerative samples. A metanalysis of traditional and quantitative electroencephalogram (qEEG) found that, despite the previously reported high diagnostic accuracy of EEG, there is not currently sufficient evidence for the meaningful clinical utility of EEG/qEEG for the diagnosis of patients with mild cognitive impairment or dementia (45). CSF biomarkers of neurodegenerative conditions, such as amyloid beta and phosphorylated tau, have been shown to have robust sensitivity and specificity (70–84%) for differentiating Alzheimer’s disease from healthy aging, particularly when considered together (46). In this same study, sensitivity and specificity of these CSF biomarkers were both lower when attempting to differentiate participants with mild cognitive impairment from healthy aging. Similarly, phosphorylated tau derived from blood samples was shown to correlate well with Alzheimer’s progression as well as successfully differentiate Alzheimer’s disease from cognitively healthy older adults as well as other neurodegenerative conditions. Plasma phosphorylated tau has been shown to have similar classification accuracy and to correlate well with Tau PET imaging. Estimates of diagnostic accuracy for neuropsychological assessment of neurodegenerative conditions vary widely based largely on the type of neuropsychological data investigated. A recent metanalysis assessed the diagnostic accuracy of neuropsychological test batteries for the diagnosis of DAT and amnestic mild cognitive impairment. This analysis yielded sensitivity and specificity of 87–89% and 88–89% when classifying participants with DAT and 72–75% and 81%, respectively, when classifying participants with amnestic mild cognitive impairment (47). Not surprisingly, studies investigating the use of single neuropsychological tests yield lower diagnostic accuracy findings. For example, studies of the clock drawing test reported overall diagnostic accuracies ranging from 59% to 85.0% when attempting to differentiate DAT from healthy aging (48,49). Caregiver questionnaires have been devised for screening for frontotemporal dementia with sensitivity of 79% and specificity of 90% (49). However, the main takeaway noted in this paper was the importance of informant-based data gathering in the clinical assessment of neurodegenerative disorders. Conversely, diagnostic accuracy of instrumental activities of daily living questionnaires have been shown to be of minimal clinical significance (50).
Considering this literature on diagnostic tools for neurodegenerative conditions, the diagnostic accuracy of the statistical analysis of multimodal MRI presented in the present study appear to match or supersede the findings of other diagnostic tools. While PET-based imaging yielded similar diagnostic accuracy, PET is invasive and requires the injection of a radioactive ligand, and therefore has a higher risk profile than MRI without contrast (51). Similarly, CSF-based biomarkers appear to provide meaningful clinical data though their ability to differentiate neurodegenerative disorder (52) subtypes is somewhat unclear and again the extraction of these biomarkers requires an invasive lumbar puncture. SPECT imaging and EEG/qEEG appear to be unreliable for differential diagnosis of neurodegenerative conditions (42-45). Neuropsychological assessment is certainly a robust diagnostic tool, particularly when complete neuropsychological batteries are used (47). Given that multimodal MRI, or any other neuroimaging metric to our knowledge, is not currently able to differentiate participants with and without cognitive deficit, neuropsychological assessment provides clinically meaningful data that cannot currently be captured by a brain scan. As such, quantitative analysis of multimodal MRI may be a meaningful clinical adjunct to neuropsychological assessment.
This study has some limitations which should be noted. Generalizability to other neurologic or psychiatric patient populations is limited due both to the relatively small sample size and the specific neurodegenerative diagnoses included in this study. In this vein, the interrater statistics for differentiating patients with neurodegenerative disorder from healthy participants was not sufficient to warrant further classification into neurodegenerative disorder subtypes. Further, given the cross-sectional nature of this study, we are unable to make inferences regarding the ability of these imaging modalities to predict such clinically meaningful metrics as diagnostic progression, prognosis or response to treatment. Similarly, there is no evidence to suggest that the multimodal MRI assessment presented herein would allow for differentiation between patients with diagnosis of Parkinson’s disease with dementia and those with Parkinson’s disease without dementia. The ability to predict cognitive performance from multimodal MRI is an interesting avenue for future research (53-58). Additionally, the ability for these tools to differentiate neurologic and psychiatric conditions should be further evaluated in future studies. Further, it is important to note that qualitative, visual inspection of DTI is not traditionally used in the clinical setting at all. However, this style of inspection as used in the present study sought to provide a trained rater analogue to the statistical classification procedures. Although qualitative inspection of these modalities was able to somewhat differentiate groups, the comparison of quantitative classification to visual investigation, both compared to gold-standard diagnostic procedures, in the present study suggests that the addition of quantitative assessment may provide additional, meaningful clinical data. Although FA values accounted for the majority of the predictive value of DTI metrics, MD and RD values were also important for group classification. As such, there appears to be clinically useful information in these additional DTI metrics, although FA alone also appears to be an important metric in its own right (59). Finally, as with all MR imaging analyses, the results of analyses from each of these MR modalities should be interpreted with caution, as MR data is an indirect assessment of the underlying physiological process it purports to measure (e.g., DTI and WM health); therefore, direct information about changes in the brain cannot always be derived from MR data. Comparison of MR data with other clinical labs (e.g., blood panels, genetic testing) is critically important; it is always necessary for clinicians to consider the data from any neuroimaging study in the context of each patient’s unique history and clinical presentation. Clinical usage of multimodal MR with statistical comparisons and mapping clinically available should allow the clinician to incorporate that data into their overall clinical interpretation, similar to the results of genetic analyses or psychodiagnostic tests. Currently, there are limited software packages available that automate and streamline the preprocessing and analytic pipelines required to conduct such analyses in a clinical context. As it stands, a cumbersome amount of in-house programming is required to develop the analytic pipeline, followed by approximately 90–120 minutes of human time per multimodal patient data set. To make these multimodality MR tools clinically useful, these hurdles must be overcome.
Conclusions
The current study provides a unique contribution to the existing literature on the potential clinical viability of multimodal MR imaging. As this study found that structural T1 assessment of regional volume, diffusion weighted assessment of regional WM, and ASL assessment of regional perfusion all were able to successfully differentiate different neurodegenerative disorder subtypes from healthy aging, it is likely that these techniques can be implemented in routine clinical neurologic practice in a way that meaningfully supplements the clinician’s existing patient data. Additionally, as these MR modalities were more precisely able to accurately differentiate diagnostic groups when used with advanced statistical analytic tools, it is likely that the advent of streamlined statistical software for cleaning and clarifying this data will improve the clinical utility of multimodal MR. Indeed, as such software packages achieve regulatory approval and minimize the work needed to set up and run such analyses, these tools will overcome several of the hurdles identified by clinicians as barriers to clinical use of advanced multimodal MR. Given the success of these tools to differentiate neurodegenerative disorder subtypes from worried-well, healthy aging patients, such evidence-based, statistically-sound, multimodal MR tools may have a profound impact on the fields of neurology and psychiatry. Taken together, these findings suggest the need for ongoing investigation of multimodal MR sequences and statistical programs as valid tools that can and should be translated into routine clinical care.
Acknowledgments
Funding: None.
Footnote
Conflicts of Interest: All authors have completed the ICMJE uniform disclosure form (available at http://dx.doi.org/10.21037/qims-20-1355). Author SEJ, MD is the owner of Synaptec Network, an MRI software analytics company. Authors SB and TK, PhD. are both part-time employees of Synaptec Network. The other authors have no conflicts of interest to declare.
Ethical Statement: The authors are accountable for all aspects of the work in ensuring that questions related to the accuracy or integrity of any part of the work are appropriately investigated and resolved. All procedures were carried out in accordance with the Declaration of Helsinki (as revised in 2013) and were reviewed and approved by the Western Institutional Review Board prior to participant enrollment (Pro #20152535). All participants provided written informed consent.
Open Access Statement: This is an Open Access article distributed in accordance with the Creative Commons Attribution-NonCommercial-NoDerivs 4.0 International License (CC BY-NC-ND 4.0), which permits the non-commercial replication and distribution of the article with the strict proviso that no changes or edits are made and the original work is properly cited (including links to both the formal publication through the relevant DOI and the license). See: https://creativecommons.org/licenses/by-nc-nd/4.0/.
References
- Alzheimer's Association. 2018 Alzheimer's disease facts and figures. Alzheimers Dement 2018;14:367-429. [Crossref]
- Karantzoulis S, Galvin JE. Distinguishing Alzheimer’s disease from other major forms of dementia. Expert Rev Neurother 2011;11:1579-91. [Crossref] [PubMed]
- Ryan J, Fransquet P, Wrigglesworth J, Lacaze P. Phenotypic heterogeneity in dementia: a challenge for epidemiology and biomarker studies. Front Public Health 2018;6:181. [Crossref] [PubMed]
- Rasmussen J, Langerman H. Alzheimer's Disease - Why We Need Early Diagnosis. Degener Neurol Neuromuscul Dis 2019;9:123-30. [Crossref] [PubMed]
- Pellegrini E, Ballerini L, Hernandez MDCV, Chappell FM, González-Castro V, Anblagan D, Danso S, Muñoz-Maniega S, Job D, Pernet C, Mair G, MacGillivray TJ, Trucco E, Wardlaw JM. Machine learning of neuroimaging for assisted diagnosis of cognitive impairment and dementia: A systematic review. Alzheimers Dement (Amst) 2018;10:519-35. [Crossref] [PubMed]
- Stippich C. editor. Clinical functional MRI: presurgical functional neuroimaging. Springer Berlin, 2015.
- Dagher A, Zeighami Y, Collins DL. Brain networks as targets of neurodegeneration in PD and AD. Alzheimers Dement 2016;12:328-9. [Crossref]
- Połczyńska M, Japardi K, Curtiss S, Moody T, Benjamin C, Cho A, Vigil C, Kuhn T, Jones M, Bookheimer S. Improving language mapping in clinical fMRI through assessment of grammar. Neuroimage Clin 2017;15:415-27. [Crossref] [PubMed]
- Ressler KJ, Mayberg HS. Targeting abnormal neural circuits in mood and anxiety disorders: from the laboratory to the clinic. Nat Neurosci 2007;10:1116. [Crossref] [PubMed]
- Ganesan K, Ursekar M. Clinical utility of BOLD fMRI in preoperative work-up of epilepsy. Indian J Radiol Imaging 2014;24:22-36. [Crossref] [PubMed]
- Lee MH, Smyser CD, Shimony JS. Resting-state fMRI: a review of methods and clinical applications. AJNR Am J Neuroradiol 2013;34:1866-72. [Crossref] [PubMed]
- Raamana PR, Rosen H, Miller B, Weiner MW, Wang L, Beg MF. Three-class differential diagnosis among Alzheimer disease, frontotemporal dementia, and controls. Front Neurol 2014;5:71. [Crossref] [PubMed]
- Möller C, Pijnenburg YA, van der Flier WM, Versteeg A, Tijms B, de Munck JC, Hafkemeijer A, Rombouts SA, van der Grond J, van Swieten J, Dopper E, Scheltens P, Barkhof F, Vrenken H, Wink AM. Alzheimer Disease and Behavioral Variant Frontotemporal Dementia: Automatic Classification Based on Cortical Atrophy for Single-Subject Diagnosis. Radiology 2016;279:838-48. [Crossref] [PubMed]
- Bron EE, Smitsc M, van der Flier WM, Vrenken H, Barkhof F, Scheltensd P, et al. Standardized evaluation of algorithms for computer-aided diagnosis of dementia based on structural MRI: The CADDementia challenge. NeuroImage 2015;111:562-79. [Crossref] [PubMed]
- Blennow K, Hampel H. CSF markers for incipient Alzheimer's disease. Lancet Neurol 2003;2:605-13. [Crossref] [PubMed]
- Woolrich MW, Jbabdi S, Patenaude B, Chappell M, Makni S, Behrens T, Beckmann C, Jenkinson M, Smith SM. Bayesian analysis of neuroimaging data in FSL. Neuroimage 2009;45:S173-86. [Crossref] [PubMed]
- Smith SM. Fast robust automated brain extraction. Hum Brain Mapp 2002;17:143-55. [Crossref] [PubMed]
- Manera AL, Dadar M, Fonov V, Collins DL. CerebrA, registration and manual label correction of Mindboggle-101 atlas for MNI-ICBM152 template. Sci Data 2020;7:237. [Crossref] [PubMed]
- Chappell MA, Groves AR, Whitcher B, Woolrich MW. Variational Bayesian inference for a non-linear forward model. IEEE Trans Sign Proc 2009;57:223-36. [Crossref]
- Mori S, Oishi K, Jiang H, Jiang L, Li X, Akhter K, et al. Stereotaxic white matter atlas based on diffusion tensor imaging in an ICBM template. Neuroimage 2008;40:570-82. [Crossref] [PubMed]
- Tong T, Ledig C, Guerrero R, Schuh A, Koikkalainen J, Tolonen A, Rhodius H, Barkhof F, Tijms B, Lemstra AW, Soininen H, Remes AM, Waldemar G, Hasselbalch S, Mecocci P, Baroni M, Lötjönen J, Flier WV, Rueckert D. Five-class differential diagnostics of neurodegenerative diseases using random undersampling boosting. Neuroimage Clin 2017;15:613-24. [Crossref] [PubMed]
- Brunzell H, Eriksson J. Feature reduction for classification of multidimensional data. Pattern Recognit 2000;33:1741-8. [Crossref]
- Tharwat A, Gaber T, Ibrahim A, Hassanien AE. Linear discriminant analysis: A detailed tutorial. AI Communications 2017;30:169-90. [Crossref]
- Poulsen J, French A. Discriminant function analysis. San Francisco State University: San Francisco, CA; 2008 [cited 2021 Apr 14]. Available online: http://userwww.sfsu.edu/efc/classes/biol710/discrim/discrim.pdf
- Duda RO, Fossum H. Pattern classification by iteratively determined linear and piecewise linear discriminant functions. IEEE Transactions on Electronic Computers 1966;EC-15:220-32. [Crossref]
- Junqué C, Ramírez-Ruiz B, Tolosa E, Summerfield C, Martí MJ, Pastor P, Gómez-Ansón B, Mercader JM. Amygdalar and hippocampal MRI volumetric reductions in Parkinson's disease with dementia. Mov Disord 2005;20:540-4. [Crossref] [PubMed]
- Ding B, Ling HW, Zhang Y, Huang J, Zhang H, Wang T, Yan FH. Pattern of cerebral hyperperfusion in Alzheimer's disease and amnestic mild cognitive impairment using voxel-based analysis of 3D arterial spin-labeling imaging: initial experience. Clin Interv Aging 2014;9:493-500. [Crossref] [PubMed]
- Le Heron CJ, Wright SL, Melzer TR, Myall DJ, MacAskill MR, Livingston L, Keenan RJ, Watts R, Dalrymple-Alford JC, Anderson TJ. Comparing cerebral perfusion in Alzheimer's disease and Parkinson's disease dementia: an ASL-MRI study. J Cereb Blood Flow Metab 2014;34:964-70. [Crossref] [PubMed]
- Coppen EM, Grond JV, Hafkemeijer A, Barkey Wolf JJH, Roos RAC. Structural and functional changes of the visual cortex in early Huntington's disease. Hum Brain Mapp 2018;39:4776-86. [Crossref] [PubMed]
- Bramson B, Folloni D, Verhagen L, Hartogsveld B, Mars RB, Toni I, Roelofs K. Human Lateral Frontal Pole Contributes to Control over Emotional Approach-Avoidance Actions. J Neurosci 2020;40:2925-34. [Crossref] [PubMed]
- Levenson RW, Sturm VE, Haase CM. Emotional and behavioral symptoms in neurodegenerative disease: a model for studying the neural bases of psychopathology. Annu Rev Clin Psychol 2014;10:581-606. [Crossref] [PubMed]
- Risacher SL, Saykin AJ. Neuroimaging biomarkers of neurodegenerative diseases and dementia. Semin Neurol 2013;33:386-416. [Crossref] [PubMed]
- Baron JC, Chételat G, Desgranges B, Perchey G, Landeau B, de la Sayette V, Eustache F. In vivo mapping of gray matter loss with voxel-based morphometry in mild Alzheimer's disease. Neuroimage 2001;14:298-309. [Crossref] [PubMed]
- Han Q, Hou Y, Shang H. A voxel-wise meta-analysis of gray matter abnormalities in essential tremor. Front Neurol 2018;9:495. [Crossref] [PubMed]
- Rane S, Koh N, Boord P, Madhyastha T, Askren MK, Jayadev S, Cholerton B, Larson E, Grabowski TJ. Quantitative cerebrovascular pathology in a community-based cohort of older adults. Neurobiol Aging 2018;65:77-85. [Crossref] [PubMed]
- Joki H, Higashiyama Y, Nakae Y, Kugimoto C, Doi H, Kimura K, Kishida H, Ueda N, Nakano T, Takahashi T, Koyano S, Takeuchi H, Tanaka F. White matter hyperintensities on MRI in dementia with Lewy bodies, Parkinson's disease with dementia, and Alzheimer's disease. J Neurol Sci 2018;385:99-104. [Crossref] [PubMed]
- Jenkins LM, Barba A, Campbell M, Lamar M, Shankman SA, Leow AD, Ajilore O, Langenecker SA. Shared white matter alterations across emotional disorders: A voxel-based meta-analysis of fractional anisotropy. Neuroimage Clin 2016;12:1022-34. [Crossref] [PubMed]
- Holinger DP, Shenton ME, Wible CG, Donnino R, Kikinis R, Jolesz FA, McCarley RW. Superior temporal gyrus volume abnormalities and thought disorder in left-handed schizophrenic men. Am J Psychiatry 1999;156:1730-5. [PubMed]
- Martin WR. MR spectroscopy in neurodegenerative disease. Mol Imaging Biol 2007;9:196-203. [Crossref] [PubMed]
- Shiroishi MS, Panigrahy A, Moore KR, Nelson MD Jr, Gilles FH, Gonzalez-Gomez I, Blüml S. Combined MRI and MRS improves pre-therapeutic diagnoses of pediatric brain tumors over MRI alone. Neuroradiology 2015;57:951-6. [Crossref] [PubMed]
- Schür RR, Draisma LW, Wijnen JP, Boks MP, Koevoets MG, Joëls M, Klomp DW, Kahn RS, Vinkers CH. Brain GABA levels across psychiatric disorders: A systematic literature review and meta-analysis of (1) H-MRS studies. Hum Brain Mapp 2016;37:3337-52. [Crossref] [PubMed]
- Dougall NJ, Bruggink S, Ebmeier KP. Systematic review of the diagnostic accuracy of 99mTc-HMPAO-SPECT in dementia. 2004. In: Database of Abstracts of Reviews of Effects (DARE): Quality-assessed Reviews [Internet]. York (UK): Centre for Reviews and Dissemination (UK); 1995. Available online: https://www.ncbi.nlm.nih.gov/books/NBK70560/
- Thomas AJ, Donaghy P, Roberts G, Colloby SJ, Barnett NA, Petrides G, Lloyd J, Olsen K, Taylor JP, McKeith I, O'Brien JT. Diagnostic accuracy of dopaminergic imaging in prodromal dementia with Lewy bodies. Psychol Med 2019;49:396-402. [Crossref] [PubMed]
- Papathanasiou ND, Boutsiadis A, Dickson J, Bomanji JB. Diagnostic accuracy of 123I-FP-CIT (DaTSCAN) in dementia with Lewy bodies: a meta-analysis of published studies. Parkinsonism Relat Disord 2012;18:225-9. [Crossref] [PubMed]
- Jelic V, Kowalski J. Evidence-based evaluation of diagnostic accuracy of resting EEG in dementia and mild cognitive impairment. Clin EEG Neurosci 2009;40:129-42. [Crossref] [PubMed]
- Forlenza OV, Radanovic M, Talib LL, Aprahamian I, Diniz BS, Zetterberg H, Gattaz WF. Cerebrospinal fluid biomarkers in Alzheimer's disease: Diagnostic accuracy and prediction of dementia. Alzheimers Dement (Amst) 2015;1:455-63. [Crossref] [PubMed]
- Weissberger GH, Strong JV, Stefanidis KB, Summers MJ, Bondi MW, Stricker NH. Diagnostic Accuracy of Memory Measures in Alzheimer's Dementia and Mild Cognitive Impairment: a Systematic Review and Meta-Analysis. Neuropsychol Rev 2017;27:354-88. [Crossref] [PubMed]
- Scanlan JM, Brush M, Quijano C, Borson S. Comparing clock tests for dementia screening: naïve judgments vs formal systems--what is optimal? Int J Geriatr Psychiatry 2002;17:14-21. [Crossref] [PubMed]
- Pijnenburg YA, Mulder JL, van Swieten JC, Uitdehaag BM, Stevens M, Scheltens P, Jonker C. Diagnostic accuracy of consensus diagnostic criteria for frontotemporal dementia in a memory clinic population. Dement Geriatr Cogn Disord 2008;25:157-64. [Crossref] [PubMed]
- Hancock P, Larner AJ. The diagnosis of dementia: diagnostic accuracy of an instrument measuring activities of daily living in a clinic-based population. Dement Geriatr Cogn Disord 2007;23:133-9. [Crossref] [PubMed]
- Tosun D, Schuff N, Rabinovici GD, Ayakta N, Miller BL, Jagust W, Kramer J, Weiner MM, Rosen HJ. Diagnostic utility of ASL-MRI and FDG-PET in the behavioral variant of FTD and AD. Ann Clin Transl Neurol 2016;3:740-51. [Crossref] [PubMed]
- Caminiti SP, Ballarini T, Sala A, Cerami C, Presotto L, Santangelo R, Fallanca F, Vanoli EG, Gianolli L, Iannaccone S, Magnani G, Perani D. BIOMARKAPD Project. FDG-PET and CSF biomarker accuracy in prediction of conversion to different dementias in a large multicentre MCI cohort. Neuroimage Clin 2018;18:167-77. [Crossref] [PubMed]
- Fisher CK, Smith AM, Walsh JR. Coalition Against Major Diseases; Abbott, Alliance for Aging Research, Alzheimer’s Association, Alzheimer’s Foundation of America, AstraZeneca Pharmaceuticals LP, Bristol-Myers Squibb Company, Critical Path Institute, CHDI Foundation, Inc., Eli Lilly and Company, F. Hoffmann-La Roche Ltd, Forest Research Institute, Genentech, Inc., GlaxoSmithKline, Johnson & Johnson, National Health Council, Novartis Pharmaceuticals Corporation, Parkinson’s Action Network, Parkinson’s Disease Foundation, Pfizer, Inc., sanofi-aventis. Collaborating Organizations: Clinical Data Interchange Standards Consortium (CDISC), Ephibian, Metrum Institute. Machine learning for comprehensive forecasting of Alzheimer's Disease progression. Sci Rep 2019;9:13622. [Crossref] [PubMed]
- Bishop CM. Pattern recognition and machine learning. Springer, 2006.
- Smith SM, Jenkinson M, Woolrich MW, Beckmann CF, Behrens TEJ, Johansen-Berg H, Bannister PR, De Luca M, Drobnjak I, Flitney DE, Niazy R, Saunders J, Vickers J, Zhang Y, De Stefano N, Brady JM, Matthews PM. Advances in functional and structural MR image analysis and implementation as FSL. Neuroimage 2004;23:S208-19. [Crossref] [PubMed]
- Jenkinson M, Bannister P, Brady M, Smith S. Improved optimization for the robust and accurate linear registration and motion correction of brain images. Neuroimage 2002;17:825-41. [Crossref] [PubMed]
- Desikan RS, Ségonne F, Fischl B, Quinn BT, Dickerson BC, Blacker D, Buckner RL, Dale AM, Maguire RP, Hyman BT, Albert MS, Killiany RJ. An automated labeling system for subdividing the human cerebral cortex on MRI scans into gyral based regions of interest. Neuroimage 2006;31:968-80. [Crossref] [PubMed]
- Chappell MA, MacIntosh BJ, Donahue MJ, Jezzard P, Woolrich MW. Partial volume correction of multiple inversion time arterial spin labeling MRI data. Magn Reson Med 2011;65:1173-83. [Crossref] [PubMed]
- Hua K, Zhang J, Wakana S, Jiang H, Li X, Reich DS, Calabresi PA, Pekar JJ, van Zijl PC, Mori S. Tract probability maps in stereotaxic spaces: analyses of white matter anatomy and tract-specific quantification. Neuroimage 2008;39:336-47. [Crossref] [PubMed]