A prior image constraint robust principal component analysis reconstruction method for sparse segmental multi-energy computed tomography
Introduction
Since Hounsfield proposed the first computerized transverse axial scanning (1), X-ray computed tomography (CT) has been well studied and widely applied in clinical practice. However, the polychromatic X-ray spectra can cause beam hardening and poor tissue contrast in conventional CT imaging. Moreover, different materials with different elemental compositions can be represented by the same CT numbers, which makes it difficult to classify different tissue types. Alvarez and Macovski (2) first proposed two linear combinations of attenuation coefficient to use dual-energy information to allow the differentiation of materials. In the past decades, material decomposition or differentiation techniques by using dual-energy CT (DECT) were well studied. Nowadays, four DECT configurations have been commercially used: dual-energy scans (3), dual-sources technique (4), dual-layer detector technique (5) and rapid kVp switching technique (6,7). The DECT has proven to be a promising application in medical imaging with the ability of material differentiation, chemical composition analysis, automatic subtraction, and pseudo-monochromatic imaging (5,8-10).
Inspired by the success of DECT in the clinic, multi-energy CT, with more than two energy levels, has been put forward to seek deeper insights into a material. Multi-energy computed tomography (MECT), as well as DECT, has a large range of diagnostic applications: automated bone removal in CT angiography, urinary stone characterization, perfused blood volume, virtual non-contrast-enhanced images and so on. These MECT applications show great image quality improvement and clinical advantages over current single-energy CT. Recently, two categories of MECT have been proposed, one is discriminating multi-energy signals based on photon counting detectors (PCD) (11-13), and the other is scanning objects under multiple kVp levels (14-17). Although PCD-based MECT has shown promising applications in clinics (13,18), it is still in the research stage and commercially unavailable because of the limitations in pulse pile-up effect, charge sharing and other hardware problems (5,8,19). Some alternative methods were proposed to implement MECT on a conventional single-source CT machine. Lee et al. (20) proposed a many-view undersampling framework that exploits a multi-slit filter to realize single-scan dual-energy low-dose cone-beam CT imaging. To cope with high noise level due to the beam filtration, they developed a new reconstruction algorithm that exploits the joint sparsity between the low and high-energy CT images. To enable some standard and non-standard configurations of MECT, Chen et al. (21) developed a new adaptive steepest descent non-convex projection-onto-convex-set (ASD-NC-POCS) algorithm, which could solve the non-convex reconstruction problem. Kim et al. (14) proposed a sparse-view kVp-switching based MECT framework with a conventional energy-integrated detector, where tube voltage switches among triple-energy levels rapidly in a gantry rotation. A penalized maximum likelihood method was proposed to reconstruct images using spectral patch-based low-rank penalty. However, this system requires tube voltage switching frequency to be above kHz, which could be extremely difficult to be realized on a conventional CT platform. To reduce this technical difficulty of kVp switching-based MECT, Shen et al. (15) proposed a segmental scanning protocol (SegMECT) with an iterative quotient total variation (Q-TV) based reconstruction method. In their scanning configuration, a circular trajectory is divided into multiple arcs with different X-ray tube voltages, thus its switching frequency is reduced to Hz magnitude. Their iterative reconstruction algorithm was specifically developed to address the severe limited-angle artifacts induced by the segmental scan. However, some residual artifacts and noise remained and the image quality was not satisfactory in some cases.
In this study, we propose a novel sparse segmental MECT (SSMECT) scan scheme, which is a cost-efficient and no-extra dose induced way to realize multi-energy data acquisition on a conventional single-source CT platform. Distinct from previous kVp-switching systems, the X-ray source is controlled to maintain an energy within a segmental arc, and then switch to another voltage alternately in the sparse segmental scan. Thus this scan could reduce the voltage switching frequency to several Hz, which is much easier to be implemented on a single-source CT machine compared with the conventional kVp-switching scan. Nevertheless, the sparse-view and limited-angle artifacts could also be induced by the sparse segmental scan when utilizing conventional reconstruction methods. To specifically address this problem, we then propose a prior image constraint robust principal component analysis (PIC-RPCA) regularization based reconstruction method with prior image constraint. The PIC-RPCA based method regularizes multi-energy images on the low rank component and sparse component, to adaptively preserve the detailed structure information of heterogeneous tissues and improve the smoothness of homogeneity. The prior image is also introduced, which is reconstructed by filtered back-projection (FBP) method with the full-scan data, to fully utilize the structural similarity between different energy channels. A numerical simulation and a real experiment are conducted to demonstrate the feasibility of our scan scheme and the effectiveness of our reconstruction method.
The rest of this paper is organized as follows. Section Methods will present the proposed SSMECT data acquisition and RPCA based reconstruction method. A detailed description of the implementation and the configuration of our method will also be given in this section. Results are shown and evaluated by comparing the proposed method with some state-of-the-art methods. Finally, Section Discussion draws the discussion and conclusions of our study.
Methods
Data acquisition
For SSMECT data acquisition, the X-ray source is controlled to maintain an energy within a segmental arc, and then switch to another kVp level alternately with segmental arc angle θ equal to
where N is the total number of energy channels and Ω is a positive integer. For the multi-energy subsets, each subset has 360/N projections in total with Ω number of θ-angle-covered projection data. Thus, we only need to switch tube voltage a few times to complete data acquisition. We refer to this scheme as sparse segmental scan, which can greatly decrease voltage switching frequency and easy-to-implement on a conventional CT machine without introducing extra dose. Figure 1 shows an example of a tri-energy SSMECT data acquisition when Ω=3, θ=2π/9,. There are three multi-energy subsets, each subset has 120 projections in total with three 2π/9-covered projection data.
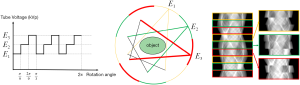
Especially, when N=3, if Ω=120 and θ=π/180, this scan mode can be seen as sparse MECT scan (14) with three energy channels, each channel has 120 projections in total with one hundred twenty π/180-covered projection data; if Ω=1 and θ=2π/3, this scan mode can be seen as SegMECT scan (15), each subset has 120 projections in total with one 2π/3-covered projection data.
Reconstruction method
The sparse segmental scan leads to sparse-view and limited-angle issues, which poses great challenges to the reconstruction. Sparse-view and limited-angle artifacts can be severe when using conventional methods to reconstruct images from these multiple limited-angle covered projections. Many studies have been published to deal with sparse-view and limited-angle problems (14-16,22-24). In this work, we proposed a prior image constraint RPCA method to reconstruct SSMECT images from these sparse-view and limited-angle projections.
PIC-RPCA reconstruction
For SSMECT system, multi-energy CT images X can be written mathematically as with totally N spectra, and . The acquired multi-energy projections can be expressed as and . A multi-energy CT system can be generally defined as
where is the system matrix of n-th spectrum. The multi-energy CT reconstruction is to recover X from Y. Note that this equation takes an approximation that neglecting the measurement errors in Y.
In a typical CT data acquisition, the system matrix A is often overdetermined and the projection Y is always contaminated by noise. In addition, our proposed sparse segmental scan poses a challenge on reconstruction with sparse-view and limited-angle problem. To solve the ill-conditioned problem, a RPCA regularization was utilized to hold image structural information and plugged into multi-energy CT reconstruction, which suggests that the representation of these images can be further sparsified by considering the rank-and-sparsity decomposition (25-27). A multi-energy image X can be decomposed to a background component XL and variation componentXS. The decomposition is performed by solving the following minimization problem:
where is a nuclear norm that is calculated by the sum of singular values of the input matrix. is the l1-norm as the sum of absolute values of the entries, with the trade-off parameter λ. T represents a sparsifying transform, such as total variation (TV) (28,29), or tight framework (TF) (30,31). Here we used TV as the sparsifying basis.
Based on the traditional RPCA regularization, we then proposed a reconstruction method with a PIC-RPCA regularization, which introduces a prior image reconstructed from all the spectral projections, to fully utilize the structural similarity among the whole energy channels. The overall objective function of our proposed method is as follows:
with PIC-RPCA regularization:
where A is a diagonal block matrix that contains all the system matrix An of different spectral channels. The low rank component XL contains the principal stationary structure and the sparse component XS=X−XL contains the spectral differences. XP is the prior image set reconstructed by conventional FBP method from a weighted combination of the whole spectral projections, which will be explained in detail in the following section. This regularization term consists of two sub-terms: the first term of Eq. [5] is a conventional prior image constraint compressed sensing (PICCS) regularization (32) and the last two terms represent a regular RPCA regularization. λP, λL, λS are three model parameters that balance the data fidelity and regularization. λP is the weight of PICCS regularization, which fully introduced the prior information in this method. Parameter modulates the dependence on prior image in the PIC constraint. λL and λS balanced the spectral differences and the structural details in the reconstruction image.
To solve this constrained optimization problem of Eq. [4], the ASD-POCS framework (29) is adapted to alternatively minimize the terms of data fidelity and regularization. First, we utilized the conjugate gradient least square (CGLS) method (33) to solve the data fidelity problem. Second, we used a gradient descent method (29) to solve the TV based optimization problem iteratively as
where i is the iteration number, β is the step size. x is a discrete two-dimensional image, which is one slice of the three-dimensional image Xn. is the TV of x. This TV norm can be defined as
where s and t are the row and column locations of x. Hence, the derivative of are
where and ρ is a small positive value to keep the denominator not equal to zero. As XL can be decomposed by singular value decomposition XL=UƩV*, the minimization of the nuclear norm, can be explicitly calculated by the singular value thresholding (SVT) method as
where is a diagonal matrix containing singular values a of XL, and is the shrinkage operator with a threshold parameter γ. The singular values would become sparser and this low rank component gradually approaches to the optimal solution in an iterative fashion. The sparse component Xs can be regularized by minimizing the where , using a gradient descent method (29). Then the new X is updated by enforcing non-negative constraint to make it physically meaningful.
In a sparse segmental scan, a full circular scan consists of multiple limited-angle covered projection sets from different energy spectra. A natural idea to deal with sparse-view and limited-angle problems is to combine all the segmental data together, which could contain all the structural information even though it varies in intensity. A prior image could be obtained by combining all the normalized projections to mitigate the influence of intensity variation. Hence, we combine all the segmental data into a full 2π-coverage projection, and then get normalized projection YP through normalizing all the projections value into [0 1].
where , which is a normalization operator using the reciprocal of l1-norm of the projection in n-th spectra. Then the prior image XP can be reconstructed through a conventional FBP method. Although the FBP method is not comparable to regularized reconstruction for obtaining the prior image, it is a feasible way to reconstruct the prior image in this study when considering the reconstruction efficiency and applicability.
The purpose of this PIC-RPCA method is to use the structural similarity among multi-energy images to remove most of the noise and improve its smoothness, and then to regularize the low-rank component and the sparse component of the images to preserve the detailed structure information.
Detailed implementation
Figure 2 summarizes the pseudo-code of this proposed PIC-RPCA method. For the simulation and experimental studies, initialization of X, XS are set to be zero matrix. The parameter α in PIC constraint is selected to be 0.8 by comparing reconstruction performance in terms of quantitative metrics mentioned below. The step size β1 and β2 are chosen to be 0.2, which kept the same as those used in the conventional ASD-POCS algorithm (29). The shrinkage threshold γ is 10−5, which is empirically chosen and the same as the previous study by Otazo et al. (24). The stopping criterion in the main iteration reaches the max iteration number or the relative image difference between two adjacent iterations , where ε is a very small value and set to be 10−5 in our study. The iteration number of the inner loop is determined by trial-and-error. We tried different iteration numbers ranging from 10 to 500 and found that 50 iterations produced the best performance. Then we empirically chose the iteration number to be 50 in our experiments.
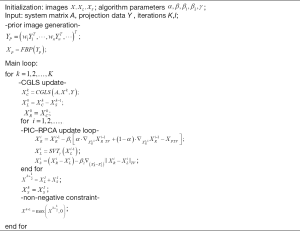
The proposed method consists of three main steps: (I) combine all the segmental data together into a full 2π-coverage projection, then get YP through Eq. [10] and utilize FBP method to reconstruct prior images XP; (II) perform an iteration with the sparse segmental projections and prior images. This iteration contains three sub-steps: first, reconstruct with the incomplete projections by the CGLS method; second, take the intermediate image and prior image into PIC-RPCA regularization to fully utilize the structural similarity of multi-energy images and prior images; third, use non-negative constraint to make the reconstructed attenuation coefficient physically meaningful; (III) loop until the iteration meets the stopping criteria. During the iteration loop, the previous iteration result is utilized as the initial value of the current step.
Evaluation
To evaluate the performance of the proposed SSMECT scan with the PIC-RPCA reconstruction method, we conducted a numerical simulation and a real experiment on a tri-energy SSMECT system.
Scan configuration
The simulation study was conducted with a numerical NCAT phantom and projections were generated using Siddon’s ray-tracing algorithm (34) (Figure 3A). The real experiment was performed with a Catphan 600 phantom (Figure 3B) on a Varian TrueBeam linear accelerator on-board imaging cone-beam CT (Varian Medical System, Palo Alto, CA). We narrowed the source blade to greatly reduce scattering signal to mimic a CT scan. The energy channels were empirically chosen to scan under 80, 100 and 120 kVp spectra (shown in Figure 3C). We modulated the tube current and exposure time for 80, 100 and 120 kVp to 1.4, 0.8 and 0.5 mAs respectively. The source to rotation center is 100 cm and to detector is 150 cm. The detector has 384 pixels. The resolution of NCAT and the real phantom image is 512×512 and their physical sizes are 25.6×25.6 cm2.
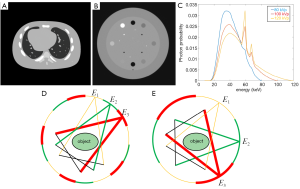
For the full-scan MECT, three full 360° scan datasets were collected, each dataset consists of 360 projections acquired with 1° angular increment. As for the SegMECT, one segmental dataset of an energy channel consists of 120 projections acquired with 120° angular increment. For the sparse segmental scan, tube voltage was held unchanged in a segmental arc and switched alternately among 80, 100 and 120 kVp in a full-scan. In our study, we chose θ equal to 2π/15 (shown in Figure 3D) and π/3 (shown in Figure 3E) respectively, to better implement our data acquisition and image reconstruction in the simulation and real case experiments. There are three subsets in total, when θ equal to 2π/15, each subset has 120 projections with five 2π/15-covered projection data; when θ equal to π/3, each subset has 120 projections with two π/3-covered projection data. Hence, we collected these datasets in the above sequence from the full-scan datasets.
Quantitative evaluation
The following three figures of merit are utilized to evaluate image quality of different reconstruction methods. The contrast-to-noise ratio (CNR) is defined as,
S and BG are the two image windows of a specific area and background respectively. μ is the average values and σ2 is the standard deviation. The mean square error (MSE) is defined as,
xr is the reconstructed image, xa is the phantom image used as ground-truth, M is the total number of image pixels. The structural similarity (SSIM) is defined as (35):
where x1, x2 are two images for comparison. c1, c2 are two variables to stabilize the division with weak denominator, which are empirically chosen to be 0.01 and 0.03 respectively.
Line profiles and HU linearity analysis (36) are also assessed on Catphan phantom for quantitative evaluation.
Methods for comparison
We first compare our PIC-RPCA method with the PICCS method:
and RPCA method, respectively:
Then we also evaluate the performance of three different scan protocols: a full MECT scan (5) as reference, and a SegMECT scan (15) for comparison with our SSMECT scan.
Results
Segmental arc range
For the choice of segmental arc range, a natural idea is to avoid projection overlap of each energy channel. Note that different energy numbers will have different optimal ranges to avoid the overlap. The segmental arc angle would be better chosen to be subject to the following equations:
where Ω1,Ω2 are positive integers. For example, if N=3, to achieve low kVp frequency in Hz level and avoid projection overlap, θ is better equal to 2π/15, 2π/9 or 2π/3. We performed a simulation study on NCAT phantom to test our point. We chose N=3and 360 projections in total. The segmental arc angles were selected to be: π/18, 2π/15, π/6, 2π/9, π/3, 2π/3. When θ equal to π/18, π/6 or π/3, they are not satisfied Eq. [17] and [18] and [18] and exist overlap. θ should equal to 2π/15, 2π/9 or 2π/3to satisfy Eq. [17] and [18].
We first performed a simulation study to test our SSMECT scan and the corresponding reconstruction method. The projections of numerical NCAT phantom were generated by using the ray-tracing method. The forward operator is the same as that used in the reconstruction. In the simulation study, we considered an ideal measurement that didn’t add noise into the primary data, and only focused on dealing with the limited-angle and sparse-view artifacts. Image reconstruction was performed by the following five strategies: FBP with full scan data, prior image constrained compressed sensing (PICCS) reconstruction with SegMECT data, and PICCS, RPCA and PIC-RPCA regularizations with the SSMECT data for comparison.
For the PICCS method, visual inspection can consistently find that reconstructed images suffered from severe distortion while θ not satisfied Eq. [17] and [18], but the images showed better suppression on noise and artifacts when θ satisfied Eq. [17] and [18], as shown in Figure 4. For the RPCA method, we can find that the results suffer from less steak artifact but noisy problem still remain regardless of the choice of θ, as shown in Figure 5. For the PIC-RPCA method, visual inspection of Figure 6 can find the reconstructed images always showed good performance on noisy and artifact suppression, which may not be affected by the segmental arc range. The quantitative performance of average MSE and SSIM are shown in Figure 7. These figures of merits also demonstrate the consistent findings with the visual inspection. The proposed PIC-RPCA method showed robust reconstruction performance and was less sensitive to the choice of segmental arc range than the other compared methods.
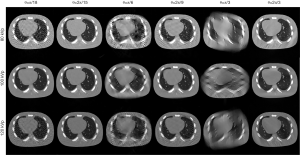
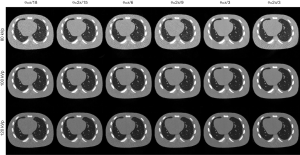
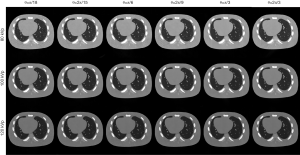
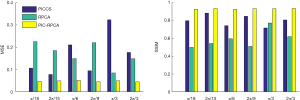
Simulation case
The setup of the simulation case has been reported in the above section. The zoom-in images of the rectangle region in Figure 8 are shown in Figure 9, to better demonstrate the detail of reconstructed images. For SSMECT scan, visual inspection can consistently find that PICCS method induces streak artifacts, and the RPCA method suffers from severe noise problem. Meanwhile, Our PIC-RPCA method shows a better streak-artifacts suppression and edge restoration than the other comparison methods. Compared with the SegMECT method, our SSMECT scan with PIC-RPCA method also shows a better suppression on noise and artifacts.
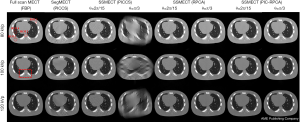
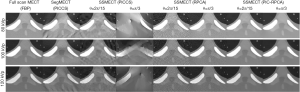
The CNR results are shown in Figure 10 and quantitative evaluation of the SSIM and MSE are shown in Table 1. These figures of merits demonstrate that the proposed SSMECT method could generate multi-energy images with satisfactory quality. Specifically, PIC-RPCA regularization can increase SSIM from ~0.85 to 0.98, while decreasing MSE by about 56% and increasing CNR by about 5 times compared with RPCA regularizations.
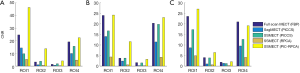
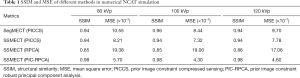
Full table
Real case
We then test our method with a real Catphan phantom experiment on a single-source platform. As shown in Figure 11, the sparse-view and limited-angle data of SSMECT scan can result in severe blurry artifacts if conventional methods were used, especially on the edge of the reconstructed images. In contrast, our proposed PIC-RPCA method suppresses sparse-view and limited-angle artifacts with good edge recovery. The zoom-in images show the detail of reconstructed images in Figure 12. For the PICCS method with SegMECT data, there are some blurry artifacts and edge distortion that degrade image quality. For the PICCS and RPCA methods with SSMECT data, their reconstruction results were affected by some residual noise and artifacts. Instead, the PIC-RPCA method could suppress most noise and streak artifacts, while preserving the edge of these contrast rods well.
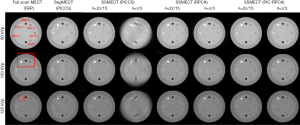
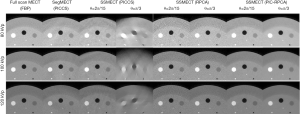
Quantitative evaluation in Figure 14 and Table 2 also validates that our method can achieve better reconstruction accuracy than the other comparison methods. Specifically, PIC-RPCA regularization can increase SSIM from ~0.64 to 0.90, while decreasing MSE by 46.07% and increasing CNR by about 3 times compared with RPCA regularizations. The results of θ=2π/15 were used to assess the HU fidelity. The line profiles in Figure 13 show that the reference FBP images are still contaminated with severe noise while the PIC-RPCA reduces the noise on the homogeneous region and preserves higher HU value fidelity than other competitive methods. HU linearity analysis in Figure 15 demonstrates that these iterative regularization approaches could maintain the linearity of the reference data as assessed by the R2 value.
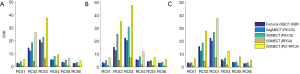
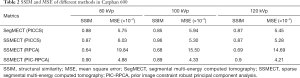
Full table
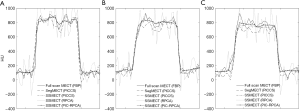
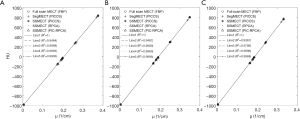
Discussion
In this work, we proposed a novel SSMECT scan scheme to realize a multi-energy scan on a conventional CT platform with low tube voltage switching frequency. A PIC-RPCA reconstruction method was specifically developed to deal with the SSMECT induced sparse-view and limited-angle problem. Both numerical and experimental experiments were conducted to validate our SSMECT scan with the PIC-RPCA reconstruction method. Results showed that our proposed method could realize MECT with high-quality images on a single-source CT platform.
The main advantage of SSMECT is that, by controlling the X-ray source to maintain an energy within a segmental arc, the kVp switching frequency can be down to Hz level and this can greatly reduce technical difficulties and costs without introducing extra dose in practice. To facilitate rapid multi-energy data acquisition and imaging, under-sampling strategies are commonly used (37). A many-view under-sampling framework (20) was proposed to achieve low dose single-scan dual-energy imaging on a cone beam CT platform with a multi-slit filter and an iterative image reconstruction algorithm was also proposed to reduce noisy artifacts due to the beam filtration. Without introducing any hardware, a sparse-view kVp-switching based tri-energy CT system (14) was developed, where the source energies are rapidly switched during gantry rotation. The switching frequency of such a scan scheme could be high to above kHz and is extremely difficult to realize on a common single-source platform. A SegMECT scan scheme (15) was developed to divide a circular trajectory into multiple arcs with different tube voltages, thus its switching frequency is reduced to Hz magnitude. Our proposed SSMECT scan slightly increases switching frequency in Hz level, but still be available on a traditional single-source CT platform. Note that the sparse-view kVp-switching and SegMECT are two special cases of this scan scheme, as discussed in the Method section. The generalization of this SSMECT scan would make it suitable for various scenarios in clinical practice. As our SSMECT scan narrows the range of limited-angle arc, it could greatly alleviate noise and artifacts in the reconstructed images. For the reconstruction method, the proposed PIC-RPCA method utilized global spectral information of the prior image and structural similarity among different energies to solve the sparse-view and limited-angle problem induced by the SSMECT scan.
In a practical scan, tube voltage will have a transition region from high to low (or low to high) voltage (as shown in Figure 16), as discussed in the previous study by Shen et al. (15). Because of these transition regions, the summation of the segmental arc coverage for E1…EN will be less than 2π. A compensation for this sum could be applied,
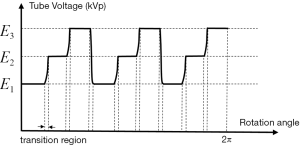
Here, are projections from transition regions. are weights applied to the projection from transition regions. By applying to adjust the prior image, the contribution from transition regions is incorporated. The only difference of this segmental arc range would be slightly smaller compared with that of the ideal case. In the paper, we studied two scan schemes with the segmental arc angle θ=2π/15 and θ=π/3. As different values of θ yield different reconstruction results, the optimal scan strategy needs further investigation for different tasks. As we stated in the Method, our experiment was conducted on a linear accelerator on-board imaging cone-beam CT rather than a commercial CT machine. This is mainly due to the fact that this cone-beam CT is easily controlled to realize our proposed scheme, while this cannot be done currently on a CT scanner without the help from manufacturers. Although it is beyond the scope of this study, we would like to realize our proposed technique on the diagnostic CT scanner and do a series of real patient studies by cooperation with the manufacturers.
For the proposed PIC-RPCA reconstruction method, we implemented and adapted an adaptive-steepest-descent projection-onto-convex-sets (ASD-POCS) framework that has been developed by Sidky and Pan (29). This framework is proven to be effective and robust for CT image reconstruction purposes (15,21,22). We do not exclude the possibilities to investigate more advanced algorithms that can reconstruct images more accurately. Especially, some deep-learning based approaches (36,38,39) have shown their superiority for such sparse and/or limited view CT reconstruction. One of our future work is to explore some more advanced reconstruction algorithms on our proposed sparse segmental multi-energy acquisition scheme. Proper selection of regularization parameters is a crucial task to achieve superior image quality for all iterative reconstruction algorithms. Four regularization parameters are involved in the objective function of the proposed PIC-RPCA method. In our work, a trial-and-error strategy was utilized to select optimal parameters. However, in real clinical practice, all the parameters should be predetermined or adaptively adjusted on-the-fly. Especially for prior-image-based reconstruction, one major challenge with PIBR methods is how to optimally determine the strength of prior image information that would be introduced into the reconstructed images (40). Several strategies have been investigated for optimal parameter selection based on the data property or specific clinical task (41-43). A deep insight into adaptive parameter selection for our approach would be a topic for future work. In this study, some commonly used metrics were chosen for evaluation such as CNR, SSIM, line profiles and HU linearity. The quantitative metrics MTF and NPS were not included in this study but would be evaluated in further investigation. These figures of metrics can provide some quantitative measures, but they may not predict performance of human observers who interpret CT images. To overcome the limitation of these metrics, some task-based evaluation methods have been reported and remain an active area in the medical imaging field (44-46). As this paper reports an initial study that mainly focus on developing a novel MECT acquisition and reconstruction method, we would like to apply a task-based evaluation to validate the effectiveness and applicability for clinical practice.
This study mainly focuses on the novel MECT scan scheme design and its corresponding reconstruction method development. As material decomposition technique is one main advantage of MECT over conventional single-energy CT (5,8), our future work will incorporate a material decomposition method into our SSMECT system. Another potential improvement of our reconstruction method is the computation time, which is an important factor of SSMECT to be applied in clinical use. In our study, the reconstruction time with 360 projections to reconstruct an image matrix is about 250 s in Matlab R2016a on a regular desktop computer with an Intel Core i7-7700 CPU. Recently, graphics processing units (GPUs) based parallel computing techniques have been successfully implemented in the reconstruction process (33,47,48). The reconstruction time of our method could be greatly shortened by this technique in the future work.
Acknowledgments
We thank Dr. Armin Samimi for editing this manuscript.
Funding: This work is supported in part by Guangdong Basic and Applied Basic Research Foundation (2020A1515110352), National key R&D Program of China (2016YFA0202003), National Natural Science Foundation of China (61971463), Ministry of Science and Technology Planning Project of Guangdong (2015B020233005 and 2015B020233002), and Guangzhou Science and technology plan project (202002030385).
Footnote
Conflicts of Interest: All authors have completed the ICMJE uniform disclosure form (available at http://dx.doi.org/10.21037/qims-20-844). The authors have no conflicts of interest to declare.
Ethical Statement: The authors are accountable for all aspects of the work in ensuring that questions related to the accuracy or integrity of any part of the work are appropriately investigated and resolved. Our ethical approval is obtained for future clinical development in our institution. However, ONLY phantoms, i.e., numerical NCAT phantom in the simulation and Catphan phantom in the experimental studies, and NO human or animal data were used in this paper.
Open Access Statement: This is an Open Access article distributed in accordance with the Creative Commons Attribution-NonCommercial-NoDerivs 4.0 International License (CC BY-NC-ND 4.0), which permits the non-commercial replication and distribution of the article with the strict proviso that no changes or edits are made and the original work is properly cited (including links to both the formal publication through the relevant DOI and the license). See: https://creativecommons.org/licenses/by-nc-nd/4.0/.
References
- Hounsfield GN. Computerized transverse axial scanning (tomography). 1. Description of system. Br J Radiol 1973;46:1016. [Crossref] [PubMed]
- Alvarez RE, Macovski A. Energy-selective reconstructions in X-ray computerised tomography. Phys Med Biol 1976;21:733-44. [Crossref] [PubMed]
- Kelcz F, Joseph PM, Hilal SK. Noise considerations in dual energy CT scanning. Med Phys 1979;6:418. [Crossref] [PubMed]
- Petersilka M, Krauss B, Stierstorfer K, Flohr TG. Technical principles of dual source CT. Eur J Radiol 2008;68:362-8. [Crossref] [PubMed]
- McCollough CH, Leng S, Yu L, Fletcher JG. Dual- and Multi-Energy CT: Principles, Technical Approaches, and Clinical Applications. Radiology 2015;276:637-53. [Crossref] [PubMed]
- Szczykutowicz TP, Chen GH. Dual energy CT using slow kVp switching acquisition and prior image constrained compressed sensing. Phys Med Biol 2010;55:6411-29. [Crossref] [PubMed]
- Goodsitt MM, Christodoulou EG, Larson SC. Accuracies of the synthesized monochromatic CT numbers and effective atomic numbers obtained with a rapid kVp switching dual energy CT scanner. Med Phys 2011;38:2222-32. [Crossref] [PubMed]
- Taguchi K, Iwanczyk JS. Vision 20/20: Single photon counting x-ray detectors in medical imaging. Med Phys 2013;40:100901 [Crossref] [PubMed]
- Shen C, Li B, Lou Y, Yang M, Zhou L, Jia X. Multi-energy Element-resolved Cone Beam CT (MEER-CBCT) Realized on a Conventional CBCT Platform. Med Phys 2018;45:4461-70. [Crossref] [PubMed]
- Li B, Lee HC, Duan X, Shen C, Zhou L, Jia X, Yang M. Comprehensive analysis of proton range uncertainties related to stopping-power-ratio estimation using dual-energy CT imaging. Phys Med Biol 2017;62:7056-74. [Crossref] [PubMed]
- Yu Z, Leng S, Jorgensen S, Li Z, Gutjahr R, Chen B, Halaweish A, Kappler S, Yu L, Ritman E. Evaluation of conventional imaging performance in a research whole-body CT system with a photon-counting detector array. Phys Med Biol 2016;61:1572. [Crossref] [PubMed]
- Fredenberg E, Hemmendorff M, Cederström B, Aslund M, Danielsson M. Contrast-enhanced spectral mammography with a photon-counting detector. Med Phys 2010;37:2017-29. [Crossref] [PubMed]
- Badea CT, Clark DP, Barber WC, Holbrook M. editors. Development of a spectral photon-counting micro-CT system with a translate-rotate geometry. Physics of Medical Imaging; 2018.
- Kim K, Ye JC, Worstell W, Ouyang J, Rakvongthai Y, El FG, Li Q. Sparse-view spectral CT reconstruction using spectral patch-based low-rank penalty. IEEE Trans Med Imaging 2015;34:748. [Crossref] [PubMed]
- Shen L, Xing Y. Multienergy CT acquisition and reconstruction with a stepped tube potential scan. Med Phys 2015;42:282-96. [Crossref] [PubMed]
- Zhang H, Xing Y. editors. Limited-angle multi-energy CT using joint clustering prior and sparsity regularization. SPIE Medical Imaging; 2016.
- Li B, Shen C, Chi Y, Yang M, Lou Y, Zhou L, Jia X. Multienergy Cone-Beam Computed Tomography Reconstruction with a Spatial Spectral Nonlocal Means Algorithm. SIAM J Imaging Sci 2018;11:1205-29. [Crossref] [PubMed]
- Ronaldson JP, Zainon R, Scott NJ, Gieseg SP, Butler AP, Butler PH, Anderson NG. Toward quantifying the composition of soft tissues by spectral CT with Medipix3. Med Phys 2012;39:6847. [Crossref] [PubMed]
- Ponchut C. Correction of the charge sharing in photon-counting pixel detector data. Nuclear Instruments & Methods in Physics Research 2008;591:311-3. [Crossref]
- Lee D, Lee J, Kim H, Lee T, Soh J, Park M, Kim C, Lee YJ, Cho S. A Feasibility Study of Low-Dose Single-Scan Dual-Energy Cone-Beam CT in Many-View Under-Sampling Framework. IEEE Trans Med Imaging 2017;36:2578-87. [Crossref] [PubMed]
- Chen B, Zhang Z, Sidky EY, Xia D, Pan X. Image reconstruction and scan configurations enabled by optimization-based algorithms in multispectral CT. Phys Med Biol 2017;62:8763. [Crossref] [PubMed]
- Sidky EY, Kao CM, Pan X. Accurate image reconstruction from few-views and limited-angle data in divergent-beam CT. J Xray Sci Technol 2009;14:119-39.
- Gao H, Yu H, Osher S, Wang G. Multi-energy CT based on a prior rank, intensity and sparsity model (PRISM). Inverse Probl 2011;27:115012 [Crossref] [PubMed]
- Otazo R, Candès E, Sodickson DK. Low-rank and Sparse Matrix Decomposition for Accelerated Dynamic MRI with Separation of Background and Dynamic Components. Magnetic Resonance in Medicine 2015;73:1125-36. [Crossref] [PubMed]
- Candes EJ, Li X, Ma Y, Wright J. Robust principal component analysis? Journal of the ACM 2009;58:11.
- Chandrasekaran V, Sanghavi S, Parrilo PA, Willsky AS. Rank-Sparsity Incoherence for Matrix Decomposition. SIAM J Optim 2009;21:572-96. [Crossref]
- Gao H, Cai JF, Shen Z, Zhao H. Robust principal component analysis-based four-dimensional computed tomography. Phys Med Biol 2011;56:3181-98. [Crossref] [PubMed]
- Rudin LI, Osher S, Fatemi E. Nonlinear total variation based noise removal algorithms. Physica D 1992;60:259-68. [Crossref]
- Sidky EY, Pan X. Image reconstruction in circular cone-beam computed tomography by constrained, total-variation minimization. Phys Med Biol 2008;53:4777. [Crossref] [PubMed]
- Ron A, Shen Z. Affine Systems inL2(Rd): The Analysis of the Analysis Operator. Journal of Functional Analysis 1997;148:408-47. [Crossref]
- Daubechies I, Han B, Ron A, Shen Z. Framelets: MRA-based constructions of wavelet frames. Applied & Computational Harmonic Analysis 2003;14:1-46. [Crossref]
- Chen GH, Tang J, Leng S. Prior image constrained compressed sensing (PICCS): a method to accurately reconstruct dynamic CT images from highly undersampled projection data sets. Med Phys 2008;35:660-3. [Crossref] [PubMed]
- Jia X, Dong B, Lou Y, Steve BJ. GPU-based iterative cone-beam CT reconstruction using tight frame regularization. Phys Med Biol 2011;56:3787. [Crossref] [PubMed]
- Siddon RL. Fast calculation of the exact radiological path for a three-dimensional CT array. Med Phys 1985;12:252. [Crossref] [PubMed]
- Wang Z, Bovik AC, Sheikh HR, Simoncelli EP. Image quality assessment: from error visibility to structural similarity. IEEE Trans Image Process 2004;13:600-12. [Crossref] [PubMed]
- Podgorsak AR, Shiraz Bhurwani MM, Ionita CN. CT artifact correction for sparse and truncated projection data using generative adversarial networks. Med Phys 2021;48:615-26. [Crossref] [PubMed]
- Shen C, Lou Y, Chen L, Zeng T, Ng MK, Zhu L, Jia X. Comparison of three undersampling approaches in computed tomography reconstruction. Quant Imaging Med Surg 2019;9:1229-41. [Crossref] [PubMed]
- Ghani MU, Karl WC, editors. Deep Learning-Based Sinogram Completion for Low-Dose CT. 2018 IEEE 13th Image, Video, and Multidimensional Signal Processing Workshop (IVMSP); 2018.
- Yoo S, Yang X, Wolfman M, Gursoy D, Katsaggelos AK, editors. Sinogram Image Completion for Limited Angle Tomography With Generative Adversarial Networks. 2019 IEEE International Conference on Image Processing (ICIP); 2019.
- Zhang H, Gang GJ, Dang H, Stayman JW. Regularization Analysis and Design for Prior-Image-Based X-Ray CT Reconstruction. IEEE Trans Med Imaging 2018;37:2675-86. [Crossref] [PubMed]
- Feng J, Qin C, Jia K, Han D, Liu K, Zhu S, Yang X, Tian J. An adaptive regularization parameter choice strategy for multispectral bioluminescence tomography. Med Phys 2011;38:5933-44. [Crossref] [PubMed]
- Wang J, Guan H, Solberg T. Inverse determination of the penalty parameter in penalized weighted least-squares algorithm for noise reduction of low-dose CBCT. Med Phys 2011;38:4066-72. [Crossref] [PubMed]
- Shen C, Gonzalez Y, Chen L, Jiang SB, Jia X. Intelligent Parameter Tuning in Optimization-Based Iterative CT Reconstruction via Deep Reinforcement Learning. IEEE Trans Med Imaging 2018;37:1430-9. [Crossref] [PubMed]
- Richard S, Husarik DB, Yadava G, Murphy SN, Samei E. Towards task-based assessment of CT performance: System and object MTF across different reconstruction algorithms. Med Phys 2012;39:4115-22. [Crossref] [PubMed]
- Yu L, Leng S, Chen L, Kofler JM, Carter RE, McCollough CH. Prediction of human observer performance in a 2-alternative forced choice low-contrast detection task using channelized Hotelling observer: Impact of radiation dose and reconstruction algorithms. Med Phys 2013;40:041908 [Crossref] [PubMed]
- Xu J, Fuld MK, Fung GSK, Tsui BMW. Task-based image quality evaluation of iterative reconstruction methods for low dose CT using computer simulations. Phys Med Biol 2015;60:2881-901. [Crossref] [PubMed]
- Després P, Jia X. A review of GPU-based medical image reconstruction. Phys Med 2017;42:76-92. [Crossref] [PubMed]
- Flores LA, Vidal V, Mayo P, Rodenas F, Verdú G. Parallel CT image reconstruction based on GPUs. Radiat Phys Chem Oxf Engl 2014;95:247-50. [Crossref]