Brain neurometabolites differences in individuals with subjective cognitive decline plus: a quantitative single- and multi-voxel proton magnetic resonance spectroscopy study
Introduction
Subjective cognitive decline (SCD), refers to a self-experienced decline in cognitive capacity without measurable cognitive impairment, is regarded as one of the earliest manifestations of the Alzheimer’s disease (AD) continuum and exhibits a higher risk of developing into AD (1). It is well-known that SCD is pervasive in the elderly over 65 years of age and approximately 50% maintain cognitively stable (2). Since the cognitive function in SCD individuals maintain within normal range, it is hard to capture the subtle cognitive decline that patients may undergo before the onset of mild cognitive impairment (MCI) with the current neuropsychological evaluations. Mitchell et al. reported that SCD individuals were almost twice as likely to develop into MCI compared to normal elderly people (3). Previous studies also showed that patients with SCD present with gray matter atrophy (4,5), white matter alterations (6), brain metabolism decline (7), high β-amyloid accumulation (8), and functional activity disruption (9). These findings revealed that SCD, MCI, and AD appeared to be the same spectrum of disease (10,11). Despite the prediction of dementia by SCD, the proportion of progression of MCI/AD is low on account of its characteristics of heterogeneity. SCD could be characterized as a memory decline and/or multiple cognitive domain impairment. In addition to the pathophysiological factors of AD, SCD could be caused by many other factors (psychological and physiological factors, drug effect, and other neurological or medical diseases), for instance (12). If the SCD criteria are applied to this study, the heterogeneity should be ruled out since AD-related SCD should not be confused by the above-mentioned situations that affect subjective cognitive ability. Compared with SCD, SCD plus could be a higher risk of AD that prior to amnesiac aMCI (aMCI). According to the SCD Initiative, as an enrichment criterion, the SCD plus concept in a population-based SCD increases the likelihood of SCD indicating pathological change due to AD (13). It has a set of specific SCD features permitting addition and subtraction of items on progression of research. The features of SCD plus include subjective decline only confined to the area of memory cognition, complaints in regard to SCD (within the past 5 years), onset of SCD greater than or equal to 60 years, worry associated with SCD, the complainers feeling worse than the same age stages (memory loss), cognitive decline confirmed by an observer, and the existence of the apolipoprotein E 4 (APOE ε4) carriers and other biomarkers evidence for a potentially risk progression to AD. Therefore, with higher sensitivity and specificity for development into aMCI/AD, SCD plus obviously increased the likelihood of preclinical AD in individuals with SCD (14,15).
Proton magnetic resonance spectroscopy (1H-MRS) is a valuable non-invasive tool that can be used for the detection of several neurometabolites, and understanding the underlying pathophysiological processes in brain disorders (16). Many brain metabolites, such as N-acetylaspartate (NAA), choline-containing compounds (Cho), total creatine (tCr), myoinositol (mI), and glutamine and glutamate (Glx) can be investigated. As the most abundant amino acids, NAA is synthesized in the mitochondria and located primarily in neural cells, axons, and dendrites. It usually has been interpreted as a marker of neuronal integrity and function based on the energy metabolism in neuronal mitochondria (17). As a maker of neuroglial cells, mI is mainly located in the glial cells and plays an important role as the brain osmoregulator (18). Cho represents a component of cell membranes, which plays an important role in maintaining their integrity and function. As part of the high-energy phosphate buffering system, tCr (the sum of creatine and phosphocreatine) is considered relatively constant in the neurons and glia of the brain. Hence, its concentration is used as a reference value (19,20). Reduced NAA/Cr may reflect decreased neuronal integrity and raised mI/Cr may reflect glial activation related to neuronal degeneration, while decreased NAA/mI could be more suitable for monitoring AD progression (21,22). They use metabolite ratios rather than absolute concentrations, which has been challenged since tCr could have abnormal levels (23,24) and thus, it is hard to draw definite conclusions on which of the two metabolites in the ratio was responsible for the distinction. There are some reports of tCr values changing across the MCI-AD spectrum (25,26). Thus, using the absolute metabolite concentrations is as an accepted method since the true metabolites’ levels could be determined (27). As an established and practical technology, MRS may supply brain neurometabolic information for the early phase of AD (28,29). In addition, the underlying mechanism with respect to which neurometabolites altered first in SCD plus should be precisely elucidated. Most studies consistently showed that decreased NAA, Glx, NAA/tCr and Glx/tCr, along with increased mI and mI/tCr were observed in different areas of the brain in MCI and/or AD (19,30-32). However, the findings with respect to Cho were less consistent (21,26,30). These results indicated that MCI and/or AD are not only caused by damage to a single brain region, but also result from alterations in several brain regions. Multi-voxel 1H-magnetic resonance spectroscopy (1H-MVS) is also named chemical shift imaging (CSI), which contains two-dimensional, three-dimensional CSI. In our previous studies (30,33-35), we documented absolute quantification in 1H-MVS performed in a series of different diseases, including studies with MCI/aMCI. However, as far as we know, no study has reported the changes in metabolites in patients with SCD plus, especially using a combination of single-voxel and multi-voxel MRS (1H-SVS and 1H-MVS). Therefore, identification of neuroimaging markers of SCD plus is crucial for early detection, early diagnosis, early intervention, and reducing the burden of AD-prone populations.
We hypothesized that 1H-SVS and 1H-MVS could be useful in the diagnosis of SCD plus in clinical practice. Moreover, we speculated that the alterations of metabolites of 1H-SVS and 1H-MVS in vulnerable areas of AD, which may reflect early neurochemical alterations, precede the clinical onset, and predict preclinical AD. In order to enhance our knowledge of the underlying course of AD, promote early diagnosis and screening, provide guidance for clinical treatment, and understand the underlying pathophysiology of individuals at different stages of the disease, we aimed to explore and evaluate the absolute metabolite alterations in patients with SCD plus compared to those in aMCI and elderly controls using 1H-SVS and 1H-MVS by multiple brain region positioning. In addition, we also evaluated the changes in MRS-detected metabolites in correlation with key neuropsychological test scores.
Methods
Human participants and neuropsychological testing
A total of 96 participants from September 2017 to July 2019 were recruited in this study. Participants with SCD plus and aMCI were enrolled from the outpatient department of neurology and age- and sex-matched elderly controls (ECs) were recruited from the medical examination center. Five subjects were excluded due to MR contraindications (pacemaker claustrophobia and intracranial metallic foreign body) and insufficient image quality. The final sample consisted of 33 ECs participants, 27 SCD plus participants, and 31 aMCI participants. The study was conducted in accordance with the Declaration of Helsinki (as revised in 2013) and approved by the Ethics Committee of the second Affiliated Hospital of Shantou University Medical College. Written informed consent was obtained from all participants or his/her legal guardians before enrolment. The study was reviewed and approved by the Institutional Review Board of the Second Affiliated Hospital of Shantou University Medical College (Protocol ID: 2017-10), and all procedures were conducted following the 1964 Helsinki declaration and its later amendments. Each participant provided demographic and clinical data and underwent neuropsychological assessments by two experienced neurologists. All participants underwent a battery of standardized neuropsychological assessments (including cognitive functioning in the area of memory, attention, executive functioning, and language). Psychological tests were carried out the day before MRI acquisition. These psychological tests included the Mini-Mental State Examination Scale (MMSE) (36), the Montreal Cognitive Assessment Scale (MoCA, Beijing version) (37), assessment on the Clinical Dementia Rating Scale (CDR) (38), global deterioration scale (GDS) (39), the Auditory Verbal Learning Test [AVLT, Chinese Huashan version, including three subtests: AVLT-immediate recall scores, AVLT-delayed recall scores, and AVLT-recognition scores] (40), an activities of daily living assessment (ADL) (41), the Hachinski Ischemic Scale (HIS) (42), and the Hamilton Depression Rating Scale (HAMD) (43).
SCD plus participants were enrolled according to the criteria proposed by SCD-I (13). Psychological tests that needed to be met were performance within normal range of the MoCA, MMSE, and ADL score after sex-, age-, and education adjustment, CDR score =0, GDS score =2, HIS score <4, and HAMD score <7. aMCI participants met the following criteria by Petersen et al. (44), including (I) memory decline confirmed by an informant or clinical judgment, (II) objective memory decline that must belong to the type of episodic memory, decided by neurologists’ judgment on the basis of neuropsychological assessments, (III) CDR score =0.5, GDS score =3, HIS score <4, HAMD score <7, and ADL score <22, and (IV) did not meet the criteria by National Institute of Neurological and Communicative Diseases and Stroke-Alzheimer’s Disease and Related Disorders Association. Inclusion criteria of ECs were as follows: no subjective cognitive complaints, no physical/psychiatric/neurological disorders, no abnormalities in routine brain MR imaging (including physiological brain atrophy), and no abnormalities on the neuropsychological measurement. The exclusion criteria were: (I) HIS score >4, (II) HAMD score >24, (III) white matter injury (multiple sclerosis, tuberous sclerosis, encephalitis, cranial arteritis, epilepsy, trauma, and tumor) (IV) dementia (AD, vascular dementia, frontotemporal dementia, Lewy body dementia), (V) Moyamoya disease, Parkinson’s disease, aphasia, hepatic encephalopathy, intracranial hemorrhage, and systemic diseases, and (VI) history of alcohol dependence and other drug abuse, serious medical disease (liver and kidney dysfunction, heart and respiratory failure, chronic electrolyte disturbance, heavy metal poisoning), mental illness (depression, anxiety, bipolar disorder, schizophrenia); (VII) contraindications for MRI examination.
Conventional magnetic resonance imaging (MRI), MRS data acquisition and post-processing for metabolite quantification
Conventional MRI scans were obtained by a standard 8-channel head coil using a 3.0 T GE MRI Systems (Signa HDx Twin speed; GE Medical, Milwaukee, Wisconsin, USA). Host scanning was then changed from the clinical mode to research mode. Under this mode, axial T2 fluid attenuated inversion recovery images were used for performing spectroscopic acquisition. To ensure the same locations for each participant, the volume of interests (VOIs) for the 1H-MRS spectra were assigned to a total of 16 brain regions, as shown in Figure 1A,B and Figure 2 including the bilateral hippocampus (Hip), precuneus (Pr), posterior cingulate cortex (PCC), white matter of occipital lobe (OLWM), dorsal thalamus (DT), lenticular nucleus (LN), caput nuclei caudati (CNC), and white matter of frontal lobe (FLWM) for further metabolite quantification. Since each participant’s head size differs, the brain structure is also slightly different. To minimize the impact of cerebrospinal fluid (CSF) contamination, skull bone and air on the metabolite concentration, the VOI acquisitions were determined based on the anatomical landmarks of each participant. Owing to poor shimming on the basicranial level due to the tissue-air interface near the petrous bone, a fully automated point-resolved spectroscopy (PRESS, Probe-P) pulse sequence was used to obtain single-voxel proton MRS data (1H-SVS, TE/TR =35/1,500 ms, NEX: 4, phase × frequency: 1×1) for the bilateral Hip. The SVS VOIs were average in size 3.00 cm3 [1.0 cm (width) × 2.0 cm (length) × 1.5 cm (thickness)]. Two-dimensional multi-voxel proton MRS with PRESS pulse sequence (FOV: 16.0 cm × 16.0 cm; TE/TR =35/1,500 ms, NEX: 1, phase × frequency: 18×18) was performed at the basal ganglia level to acquire the multi-voxel MRS data. The VOIs of the multi-voxel section was about 8.0 cm (left to right) × 10.0 cm (anterior to posterior) ×1.5 cm (thickness). Thus, every single average VOI was 1.19 cm3 [(FOV: 16.0 cm × 16.0 cm/phase, frequency: 18×18) ×1.5 cm]. The VOI size and signal-to-noise ratio (S/N) in the left and right brain hemispheres of the three groups are shown in Table S1 and Table S2. Automatic prescan procedures such as calibration, shimming, and chemical shift selective water suppression were optimized as a concentration reference for the VOIs. The acquisition time of the 1H-SVS on each side of Hip were 3 minutes 42 seconds and the acquisition time of the 1H-MVS on basal ganglia slice were 8 minutes 12 seconds.
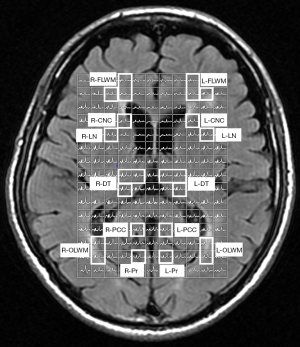
The raw data (P files, Supplementary file 1) were created by the host and were acquired from the dedicated workstation for Windows 4.6 (ADW 4.6, GE Medical, Rue de la Minière, France). All P files were subsequently imported to UNIX system (Red Hat Enterprise of Linux 8.1 version) to process the MR spectroscopic data. The data was voxel-shifted to align the CSI grid using the NAA VOI. As reported in our previous work (30,33-35), the data first underwent Fourier transformation and was subsequently processed using the software Spectroscopy Analysis by General Electric (SAGE version 7.0), which included automatic phase and frequency correction, line broadening, and zero-filling. The data were then entered into the Linear Combination of Model (LCModel version 6.3.1L, Stephen Provencher, Inc., Oakville, ON, Canada) for metabolite quantification. We used a standard detectable phantom concentration (12.5 mmol/L NAA, 3.0 mmol/L choline chloride, 10.0 mmol/L creatine hydrate, 5 mmol/L lactate, plus 0.1% sodium azide, 0.1% magnavis, and 50 mmol/L sodium hydroxide) as an outer reference to calibrate the concentrations of metabolite as well. The absolute concentrations of these metabolites (NAA, tCr, Cho, and mI; mmol/L) were then calculated from the VOI regions (See Supplementary file 2). The MVS of the metabolic profile were post-processed using the ReadyView software in the ADW 4.6 environment. SVS with full width at half maximum (FWHM) ≤7 Hz and MVS with FWHM ≤15 Hz were used for quality control. The quantifications of the metabolite concentrations were assessed using Cramér-Rao lower bounds (CRLBs). Metabolites quantified with CRLBs >50% were classified as detected, and metabolites quantified with standard deviations CRLBs that did not exceed a threshold of 20% were considered for further analysis.
Statistical analyses
Statistical analyses were conducted by the SPSS statistics version 25.0 (SPSS Inc., Chicago, III, USA) and GraphPad Prism (Inc. Prism Version 8.3.0, USA). All comparisons of the means from the demographic data (such as age, sex) were performed using the Mann-Whitney U test. One-way analyses of variance (ANOVA) with Bonferroni’s correction for post hoc (Bonferroni test) comparisons were used to assess neuropsychological tests among the three groups. As for metabolite concentrations, group differences were analyzed among the three groups by multivariate ANOVA using VOIs (×16) and metabolites (×4) as within and between factors. Bonferroni correction was used to perform post-hoc multiple pairwise comparisons among the three groups. Nonparametric tests were used when the data was non-normal distribution. Pearson’s correlation analysis controlling for sex, age, and length of education as covariates was performed to assess the relationship between the concentration of metabolites and the key neuropsychological scores for SCD plus participants. Heat maps were generated for the metabolites of interest by the R software (version 4.0.3) to a get an overview of the differences among the three groups. Furthermore, receiver operating characteristic (ROC) curve analysis was conducted to assess diagnostic accuracy of various neurometabolites. The area under the ROC curve (AUROC) was conducted to determine the predictive accuracy of the image metrics in distinguishing SCD plus from ECs or aMCI participants. In order to better distinguish the different groups and supply useful information to clinicians, combined AUC values were further acquired using binary logistic regression. Random forest (RF) was used to determine whether predictors load for the ROC curves and the model had problem of overfitting. All data are expressed as mean±SD, and the significance threshold was set at P<0.05.
Results
Demographic and key neuropsychological scores results
The demographic data of the subjects and key neuropsychological scores are summarized in Table 1. Only AVLT delayed recall (AVLT-De) scores between the ECs and SCD plus participants were significant. SCD plus participants showed significantly decreased AVLT-De and AVLT-recognition scores compared to aMCI participants. aMCI group showed significantly lower in all subitems of AVLT scores (AVLT-immediate recall scores, AVLT-De scores, and AVLT-recognition scores) than ECs group. In addition, only MoCA scores and MMSE scores were observed lower in aMCI compared with ECs participants. No significant differences were observed in terms of age, sex, and education among the participants.
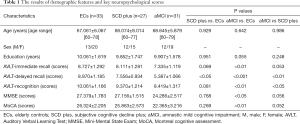
Full table
Differences in 1H-MRS neurometabolites in participants with ECs, SCD plus, and aMCI
The results of VOIs and S/N showed relatively stable performance (Tables S1,S2). Therefore, all measurements were regarded as reliable, and metabolite concentrations values were used for subsequent statistical analyses. NAA, mI, Cho, tCr and NAA/mI ratios were acquired in bilateral regions of the Hip, PCC, Pr, OLWM, FLWM, DT, LN, and CNC in three groups. Tables 2,3 show the differences in brain metabolite values from the left VOIs among the three groups. Tables 4,5 show the differences in brain metabolite levels from the right VOIs among the three groups. Figure S1A,B,C and Figure S2A,B,C show representative single-voxel MRS in left and right Hip, respectively.
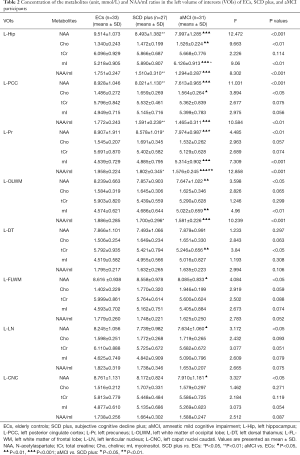
Full table
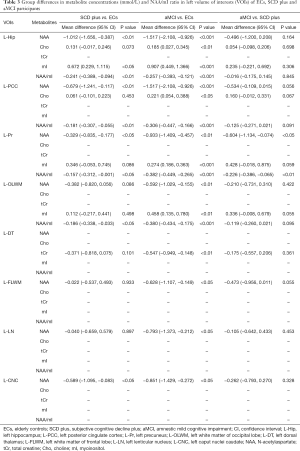
Full table
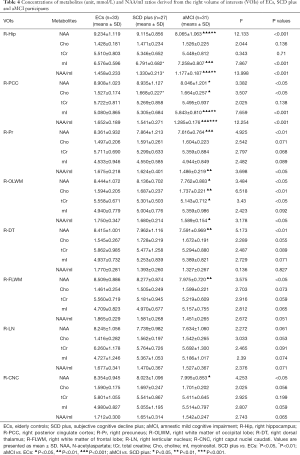
Full table
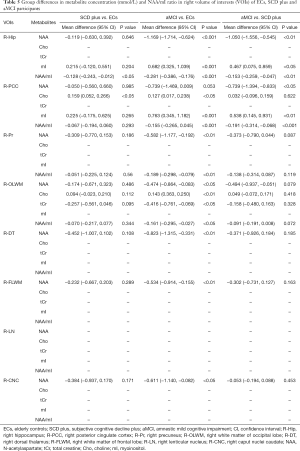
Full table
Compared with ECs group, SCD plus individuals were observed to have significantly reduced NAA levels in the left Hip, left PCC, left Pr and left CNC. mI value was only found elevated in the right Hip. The NAA/mI ratio decreased in the left Hip, left PCC, left Pr, left OLWM, and right Hip. The concentration of Cho was observed to only increase in the right PCC. Figure 3 shows an example images of increased Cho levels in the right PCC in patients with SCD plus, while no significant difference was observed in the left PCC.
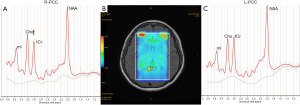
Compared with ECs group, aMCI group showed obvious lower NAA values in the bilateral Hip, bilateral Pr, bilateral OLWM, bilateral CNC, left PCC, left LN, left FLWM, and right DT. Cho levels were observed increased in the bilateral PCC, left Hip, and right OLWM. The mI levels were found increased in the bilateral Hip, left Pr, left OLWM, and right PCC. A decrease in tCr values was observed in the left DT and right OLWM. The metabolite ratios of NAA/mI were observed to decline in the bilateral Hip, bilateral PCC, bilateral Pr, and bilateral OLWM.
Compared with SCD plus group, aMCI group had decreased concentrations of NAA in the left Pr, right Hip, and right PCC. Increased mI concentrations were found in the left Hip and the right PCC. The NAA/mI ratio was decreased in the left Pr, right Hip, and right PCC. No significant metabolic differences in Cho and tCr were observed in any VOIs between the two groups.
Heat maps were constructed to visualize the distinction power of neurometabolites of interest (NAA, mI, and NAA/mI) among the three groups, as shown in Figure 4A,B,C.
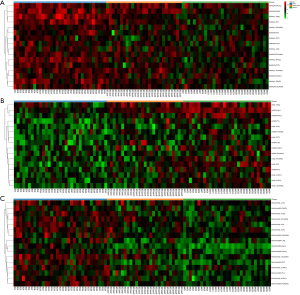
Predictive accuracy using combinations of metabolites differences and correlation analyses for data from patients with SCD plus
The NAA/mI ratio mentioned in previous literature was considered a screening marker for predicting AD using neuroimaging (45-47). Our results showed that the differences in brain neurometabolites in patients with SCD plus and aMCI are multi-regional, especially in areas of atrophy associated with AD-sensitive brain located on the hippocampus, posterior cingulate, precuneus, with a left-sided predominance (48). In addition to the differences between groups with statistically significant (P<0.05), P values of AUC were also set as less than 0.05 in differentiating SCD plus from ECs or aMCI groups. Only when the thresholds passed, the corresponding metabolites were selected for ROC analysis. Our results showed that the P values of the AUC values on NAA [left Hip, P<0.01; left PCC, P<0.01; left Pr, P<0.05; left CNC, P<0.05;] and mI value (right Hip, P<0.05) were statistically significant in distinguishing SCD plus from ECs. Although the P value of Cho level in the right PCC showed statistical differences between SCD plus and ECs groups (P<0.05), the P value of the AUC is 0.053 (P>0.05); hence, this index was omitted. As to other indices, the above conditions were all met between the SCD plus and ECs/aMCI; hence, the ROC analysis could be performed. Thus, we sought to investigate whether the differentiation SCD plus from ECs or aMCI groups may be estimated more accurately using the concentration of metabolites of NAA combined with mI or the NAA/mI ratio. Supplementary file 3 showed the basic process flow of the RF algorithm. Figure S3A,B and Figure S3C,D showed the AUC area and importance measurements from the RF based on the testing dataset in discriminating participants with SCD plus from ECs using NAA and mI values and NAA/mI ratio, respectively. Figure S4A,B and Figure S4C,D showed the AUC area and importance measurements from the RF based on the testing dataset in discriminating SCD plus from aMCI using NAA and mI values and NAA/mI ratio, respectively. The results of testing dataset showed that the AUC values for final models were not overfitting. Therefore, the AUC values based on significant group differences could be used for subsequent statistical analysis. The combined AUC levels in distinguishing SCD plus from ECs participants acquired by NAA values in the left Hip, left Pr, left PCC, left CNC and the mI value in the right Hip increased to 0.895 [Figure 5A, 95% confidence interval (CI): 0.817–0.973], whereas the combined AUC levels in differentiating SCD plus from ECs participants acquired by the NAA/mI ratios in the left Hip, left Pr, left PCC, left OLWM and the right Hip added to 0.860 (Figure 5B, 95% CI: 0.759–0.960). Likewise, the combined AUC levels in differentiating participants with SCD plus from aMCI acquired by the NAA levels in the left Pr, right Hip, right PCC, together with mI values in the left Hip and right PCC added up to 0.892 (Figure 6A, 95% CI: 0.811–0.973). The combined AUC values in distinguishing SCD plus from aMCI participants acquired by the NAA/mI ratios in the left Pr, right Hip and right PCC added up to 0.836 (Figure 6B, 95% CI: 0.735–0.938). These results revealed that the AUC values obtained by combining the levels of NAA with mI made more significant contributions to the classification than those calculated using the NAA/mI ratios. Besides, correlation tests between the key neuropsychological scores and 1H-MRS metabolites were conducted for SCD plus group. Positive correlations were found between AVLT-De scores and NAA levels in the left Hip (Figure 7A, r=0.386, P=0.047) and in the left PCC (Figure 7B, r=0.395, P=0.042). While AVLT-De scores were only positively associated with NAA/mI ratio in the left Hip (Figure 7C, r=0.416, P=0.031) of SCD plus group. There were no significant correlations (P>0.05) between AVLT-De scores and the rest of MRS metrics in SCD plus participants.
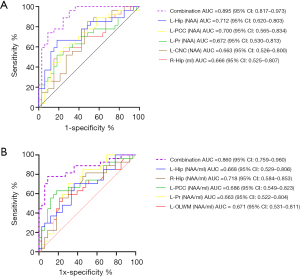
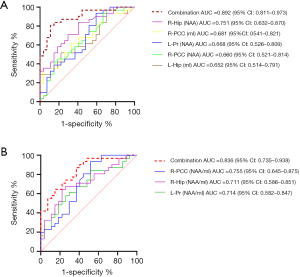
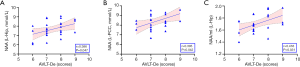
Discussion
In our study, 1H-SVS and 1H-MVS were employed to generate and output plenty of rich metabolite data by combined with many different brain regional distributions’ characteristics of MRS. This allowed us to explore the changes in metabolic distribution in different brain areas and acquire more accurate bioinformation for discrimination and diagnosis using this practical technique. Firstly, our main results suggested that patients with SCD plus reflect brain neurometabolic changes in many brain areas, especially in the Hip and PCC. Patients with aMCI revealed more obvious difference compared to individuals with SCD plus and ECs in many brain areas. These findings also suggested that SCD plus participants could share a similar tendency of brain neurometabolic differences with individuals with aMCI. Compared with ECs, Cho was observed to only increase in the right PCC in SCD plus, while tCr values were observed to decrease in the left DT and right OLWM in aMCI. Secondly, NAA concentrations may be used as one of the earliest potential neuroimaging markers at this stage, while NAA/mI ratio could be more suitable for monitoring AD progression. Moreover, the AVLT-De scores were positively associated with the NAA levels in the left Hip and left PCC in SCD plus group, whereas only the NAA/mI ratio was positively associated with the AVLT-De scores in the left Hip.
In our previous studies and other studies, patients with aMCI/MCI had been reported to have different metabolite distribution and aberrant metabolite patterns in multiple regions of the brain (30,34,49). In this study, we observed a different metabolite distribution, such as in the Hip, Pr, PCC, OLWM, DT, LN, CNC, and FLWM in aMCI participants, reflecting metabolic differences in these regions. Our results are consistent with previous research and extend the reports on the metabolic differences to include regions other than PCC and Hip in the likelihood of AD. However, our main purpose was to explore the differences in the levels of several brain metabolites in patients with SCD plus. Additionally, we also compared the advantages of absolute quantification of metabolites (NAA and mI) and metabolite ratios (NAA/mI) in distinguishing SCD plus group from aMCI or ECs individuals.
NAA is abundant in the human brain and metabolized in the mitochondria of neurons from aspartic acid and acetyl-coenzyme A. NAA is considered a part of the neuronal mitochondrial energetic metabolism through Kreb’s cycle. The Kreb’s cycle guarantees an additional supply of energy by the oxidation of glutathione via aspartate amino transferase that leads to produced alpha-ketoglutarate and NAA (50). The energy metabolism decline, which is known to take place early in the process of AD may also depress the NAA concentration (51). Therefore, NAA decline is closely related to the ATP synthesis in mitochondrial dysfunction. Since SCD plus may lead to a higher risk of a very early stage of AD, minor alterations in the NAA levels detected could be the early response to brain energy depletion. Detection of the alterations in NAA seem to be an effective way in evaluation of individuals at high-risk for dementia since it might reflect an early neuroimaging marker of energy metabolism injury in the brain, a factor referred to the pathological mechanism of AD (52,53).
In this study, elevated mI was only found in the left Hip in participants with SCD plus compared to the ECs. The mI concentration changes were observed in two brain regions (right Hip and right PCC) between SCD plus and aMCI participants. Further, our study showed that increased mI values were extended to three regions (bilateral Hip and right PCC) in participants with aMCI compared to those in ECs. Increased mI may indicate glial activation or proliferation. In the neuropathological process of AD, gliosis was associated with neuronal loss, which may result in disruption of the osmotic balance (18). mI might accumulate to regulate the osmotic balance to maintain cell volume homeostasis in the neuroglial cells (54). We speculate that the combined effect of gliosis and osmotic stress may be responsible for the elevated mI levels observed in the left Hip region. A study reported that the mI values were already increased during the preclinical stages of AD, especially in APOE ε4 carriers, indicating that mI levels may reveal brain region consequences of APOE ε4 before detectable amyloid-related pathology (55). Compared with the concentration of NAA, the brain regions involved with changes in mI are few in patients with SCD plus. A recent longitudinal study (18) and a seven-year follow-up study (47) have shown that mI and NAA/mI ratio may be useful in predicting AD progression. In this study, we found mI values gradually increased and the number of brain regions with elevated mI levels increased from ECs to aMCI. Our results indicated that alterations of NAA were detected prior to those in mI. One study has shown that decreased NAA and increased mI represents lack of correlation and reflected different pathologic AD processes (46). Our study also showed that NAA and mI levels may represent different processes underlying different stages of AD. Hence, NAA could be served as an early diagnosis marker, while mI could be better suited for monitoring AD progression. However, long-term follow-up studies are also needed to determine whether NAA/mI is suitable for monitoring AD progression.
As precursors of the neurotransmitter acetylcholine, elevated Cho values may be associated with the increase of cell membrane phospholipid turnover (56). In this study, increased Cho value was only observed in the right PCC in SCD plus compared with ECs individuals. The PCC is a limbic cortical region that experiences neuronal density loss and mitochondrial energy metabolism decline as well as cognitive dysfunction in AD (57). Therefore, the increased Cho value in the PCC of patients with SCD plus may reflect a compensatory mechanism of decreased choline-acetyltransferase activity necessitated by increased cholinergic input, and increased neuronal membrane turnover activity in the bilateral Hip and right PCC in the aMCI stage due to further neuronal degeneration. This is consistent with our previous observations (30,34).
In previous studies, tCr concentrations have been considered to be stable and commonly used as an internal reference for normalization of other metabolites. Our results showed that the tCr concentrations had a decreasing trend from ECs to patients with aMCI. The noteworthy result of our study was that we found a significant reduction in tCr values in the left DT and right OLWM in aMCI participants. Some reports have also found that the tCr concentrations may vary in different pathological conditions (23,35). The reduction in tCr levels represent an insufficient energy supply that may result from a defect in oxidative metabolism in impaired nerve cell (58). Together, the observations from previous studies and those from this study provided evidence that SCD plus and aMCI participants showed ultrastructural damage in the neuronal mitochondria. These results confirmed that the absolute concentration might be better than the relative ratios estimated using tCr as a reference.
There were five brain regions with altered metabolites, including four regions with decreased NAA (left Hip, PCC, Pr, and CNC) and one region with increased mI (left Hip). Similarly, the differences in NAA/mI ratios were also found in five brain regions (left Hip, left PCC, left Pr, left OLWM, and right Hip). Both these evaluations revealed four brain areas in the left hemisphere that contained vulnerable regions involved in memory function and language skills (59-62). The different concentration of NAA between ECs and SCD plus in the left cerebral hemisphere indicated that atrophy in SCD plus could have already occurred in AD-sensitive brain areas such as left Hip (48). The combined AUC values in distinguishing SCD plus from ECs participants acquired by the concentration of NAA combined with mI was 0.895, which was higher than the combined AUC values of 0.860 acquired by the NAA/mI ratios. Compared to patients with aMCI, the concentration changes of NAA were found in the left Pr, right Hip, right PCC, and mI levels in the right Hip and right PCC in SCD plus participants. Significantly different NAA/mI ratios were observed in three brain regions (left Pr, right Hip, and right PCC) in SCD plus participants. Likewise, the combined AUC levels in distinguishing SCD plus from aMCI participants acquired by NAA combined with mI levels was 0.892, which was also higher than the combined AUC values of 0.836 acquired by NAA/mI ratios. Our results further demonstrated that the absolute concentration may be superior to the metabolite ratio, which is consistent with previous 1H-MRS studies. The possible reason is that the absolute concentration could offer more accurate information than the metabolite ratio reflecting brain metabolite changes.
In addition, we observed AVLT-De scores were significantly associated with the NAA values in the left Hip and left PCC in SCD plus group. Only the AVLT-De scores were positively correlated with NAA/mI ratio in the left Hip. We speculated that the main reason for the effect on the two brain regions could have been the abnormal changes of NAA, while mI may have had a synergistic effect. Our correlation results were also in favor of the idea that the NAA change might be the best predictor of cognitive impairment scores, especially in the extremely early phase of AD.
This study has some limitations. Firstly, as a cross-sectional study, the samples of patients with SCD plus are small. A study with a larger sample size with a multicenter and longitudinal design is acquired to seek the early neuroimaging markers for AD (63,64). Secondly, in order to fully elucidate the AD continuous spectrum, AD individuals need to be included in the subsequent research. Thirdly, according to the features of SCD plus, the inclusion criteria for patients with SCD plus usually met more than three (13). Although the NAA and mI levels are emerging as the most useful MRS markers in AD since this measure could be strongly associated with the existence of potential amyloidosis and neurodegeneration, our study lacked the completeness of testing the status of ApoE ε4 (65), tau protein, or β-amyloid. Incorporating the examinations of these risk factors may be crucial for SCD plus detection. Moreover, although 1H-MVS could obtain a wider range of multiple single voxel spectra, reduce the partial volume effect, and reflect the distribution of metabolite concentrations throughout multi-dimensional data acquisition, certain limitations of 1H-MVS, such as long acquisition time, poor quality of shimming, inadequate water peak suppression, and susceptibility alterations related to air and bone, make it hard to acquire high-quality spectra. Besides, the study only used MRS technique for evaluation. Multi-modal MR imaging methods would yield a comprehensive understanding to elucidate the pathophysiologic mechanisms of participants with SCD plus by structural and functional MRI (66-68). Finally, we did not register the MRS data to utilize tissue segmentation results from a high-resolution anatomical image to calculate the white matter, gray matter, or CSF fraction within the VOIs; therefore, a variance source should be taken into account for correcting the metabolite concentrations more accurately.
Conclusions
In conclusion, our findings indicated that quantitative 1H-SVS and 1H-MVS could offer useful information to detect the feature of the alterative brain neurometabolite levels in patients with SCD plus. The use of absolute concentration rather than ratios might provide more valuable information regarding alternative metabolites associated with AD. NAA may be one of the earliest potential neuroimaging markers at this very early stage. Changes in brain metabolite concentrations in the PCC and Hip appeared to represent a noninvasive, effective, and potentially useful neuroimaging marker of the preclinical stage of AD.
Acknowledgments
Funding: This study was supported by the National Natural Science Foundation of China (Grant Number: 81671660), Natural Science Foundation of Guangdong Province (Grant Number: 2018A030310154), Medical Science Research Foundation of Guangdong Province (Grant Number: A2017532), Science and Technology Project of Shenzhen (Grant Number: JCYJ20170307144338570), Clinical Research Startup Program of Southern Medical University by High-level University Construction Funding of Guangdong Provincial Department of Education (Grant Number: LC2016PY061) and Shenzhen Hospital of Southern Medical University, Research Promotion Funds for the Key Discipline Construction Program (Grant Number: ZDXKKYTS010 & ZDXKKYTS011).
Footnote
Conflicts of Interest: All authors have completed the ICMJE uniform disclosure form (available at http://dx.doi.org/10.21037/qims-20-1254). The authors have no conflicts of interest to declare.
Ethical Statement: The authors are accountable for all aspects of the work in ensuring that questions related to the accuracy or integrity of any part of the work are appropriately investigated and resolved. The study was conducted in accordance with the Declaration of Helsinki (as revised in 2013) and approved by the Ethics Committee of the second Affiliated Hospital of Shantou University Medical College. Written informed consent was obtained from all participants or his/her legal guardians.
Open Access Statement: This is an Open Access article distributed in accordance with the Creative Commons Attribution-NonCommercial-NoDerivs 4.0 International License (CC BY-NC-ND 4.0), which permits the non-commercial replication and distribution of the article with the strict proviso that no changes or edits are made and the original work is properly cited (including links to both the formal publication through the relevant DOI and the license). See: https://creativecommons.org/licenses/by-nc-nd/4.0/.
References
- Tandetnik C, Farrell MT, Cary MS, Cines S, Emrani S, Karlawish J, Cosentino S. Ascertaining Subjective Cognitive Decline: A Comparison of Approaches and Evidence for Using an Age-Anchored Reference Group. J Alzheimers Dis 2015;48:S43-55. [Crossref] [PubMed]
- Jonker C, Geerlings MI, Schmand B. Are memory complaints predictive for dementia? A review of clinical and population-based studies. Int J Geriatr Psychiatry 2000;15:983-91. [Crossref] [PubMed]
- Mitchell AJ, Beaumont H, Ferguson D, Yadegarfar M, Stubbs B. Risk of dementia and mild cognitive impairment in older people with subjective memory complaints: meta-analysis. Acta Psychiatr Scand 2014;130:439-51. [Crossref] [PubMed]
- Peter J, Scheef L, Abdulkadir A, Boecker H, Heneka M, Wagner M, Koppara A, Klöppel S, Jessen FAlzheimer’s Disease Neuroimaging Initiative. Gray matter atrophy pattern in elderly with subjective memory impairment. Alzheimers Dement 2014;10:99-108. [Crossref] [PubMed]
- Saykin AJ, Wishart HA, Rabin LA, Santulli RB, Flashman LA, West JD, McHugh TL, Mamourian AC. Older adults with cognitive complaints show brain atrophy similar to that of amnestic MCI. Neurology 2006;67:834-42. [Crossref] [PubMed]
- Wen Q, Mustafi SM, Li J, Risacher SL, Tallman E, Brown SA, West JD, Harezlak J, Farlow MR, Unverzagt FW, Gao S, Apostolova LG, Saykin AJ, Wu YC. White matter alterations in early-stage Alzheimer’s disease: A tract-specific study. Alzheimers Dement (Amst) 2019;11:576-87. [Crossref] [PubMed]
- Jeong HS, Park JS, Song IU, Chung YA, Rhie SJ. Changes in cognitive function and brain glucose metabolism in elderly women with subjective memory impairment: a 24-month prospective pilot study. Acta Neurol Scand 2017;135:108-14. [Crossref] [PubMed]
- Snitz BE, Weissfeld LA, Lopez OL, Kuller LH, Saxton J, Singhabahu DM, Klunk WE, Mathis CA, Price JC, Ives DG, Cohen AD, McDade E, Dekosky ST. Cognitive trajectories associated with β-amyloid deposition in the oldest-old without dementia. Neurology 2013;80:1378-84. [Crossref] [PubMed]
- Shu N, Wang X, Bi Q, Zhao T, Han Y. Disrupted Topologic Efficiency of White Matter Structural Connectome in Individuals with Subjective Cognitive Decline. Radiology 2018;286:229-38. [Crossref] [PubMed]
- Reisberg B, Shao Y, Moosavi M, Kenowsky S, Vedvyas A, Marsh K, Bao J, Buj M, Torossian C, Kluger A, Vedvyas G, Oo T, Malik F, Arain F, Masurkar AV, Wisniewski T. Psychometric Cognitive Decline Precedes the Advent of Subjective Cognitive Decline in the Evolution of Alzheimer’s Disease. Dement Geriatr Cogn Disord 2020;49:16-21. [Crossref] [PubMed]
- Yan T, Wang W, Yang L, Chen K, Chen R, Han Y. Rich club disturbances of the human connectome from subjective cognitive decline to Alzheimer’s disease. Theranostics 2018;8:3237-55. [Crossref] [PubMed]
- Perrotin A, La Joie R, de La Sayette V, Barré L, Mézenge F, Mutlu J, Guilloteau D, Egret S, Eustache F, Chételat G. Subjective cognitive decline in cognitively normal elders from the community or from a memory clinic: Differential affective and imaging correlates. Alzheimers Dement 2017;13:550-60. [Crossref] [PubMed]
- Jessen F, Amariglio RE, van Boxtel M, Breteler M, Ceccaldi M, Chételat GSubjective Cognitive Decline Initiative (SCD-I) Working Group, et al. A conceptual framework for research on subjective cognitive decline in preclinical Alzheimer’s disease. Alzheimers Dement 2014;10:844-52. [Crossref] [PubMed]
- Hao L, Sun Y, Li Y, Wang J, Wang Z, Zhang Z, Wei Z, Gao G, Jia J, Xing Y, Han Y. Demographic characteristics and europsychological assessments of subjective cognitive decline (SCD) (plus). Ann Clin Transl Neurol 2020;7:1002-12. [Crossref] [PubMed]
- Sánchez-Benavides G, Grau-Rivera O, Suárez-Calvet M, Minguillon C, Cacciaglia R, Gramunt N, Falcon C. ALFA Study, Gispert JD, Molinuevo JL. Brain and cognitive correlates of subjective cognitive decline-plus features in a population-based cohort. Alzheimers Res Ther 2018;10:123. [Crossref] [PubMed]
- Fayed N, Modrego PJ, Salinas GR, Gazulla J. Magnetic resonance imaging based clinical research in Alzheimer’s disease. J Alzheimers Dis 2012;31:S5-S18. [Crossref] [PubMed]
- Moffett JR, Ross B, Arun P, Madhavarao CN, Namboodiri AM. N-Acetylaspartate in the CNS: from neurodiagnostics to neurobiology. Prog Neurobiol 2007;81:89-131. [Crossref] [PubMed]
- Voevodskaya O, Poulakis K, Sundgren P, van Westen D, Palmqvist S, Wahlund LO, Stomrud E, Hansson O, Westman E. Swedish BioFINDER Study Group. Brain myoinositol as a potential marker of amyloid-related pathology: A longitudinal study. Neurology 2019;92:e395-e405. [Crossref] [PubMed]
- Catani M, Cherubini A, Howard R, Tarducci R, Pelliccioli GP, Piccirilli M, Gobbi G, Senin U, Mecocci P. (1)H-MR spectroscopy differentiates mild cognitive impairment from normal brain aging. Neuroreport 2001;12:2315-7. [Crossref] [PubMed]
- Waldman AD, Rai GS. The relationship between cognitive impairment and in vivo metabolite ratios in patients with clinical Alzheimer’s disease and vascular dementia: a proton magnetic resonance spectroscopy study. Neuroradiology 2003;45:507-12. [Crossref] [PubMed]
- Wang H, Tan L, Wang HF, Liu Y, Yin RH, Wang WY, Chang XL, Jiang T, Yu JT. Magnetic Resonance Spectroscopy in Alzheimer's Disease: Systematic Review and Meta-Analysis. J Alzheimers Dis 2015;46:1049-70. [Crossref] [PubMed]
- Kantarci K, Weigand SD, Przybelski SA, Shiung MM, Whitwell JL, Negash S, Knopman DS, Boeve BF, O'Brien PC, Petersen RC, Jack CR Jr. Risk of dementia in MCI: combined effect of cerebrovascular disease, volumetric MRI, and 1H MRS. Neurology 2009;72:1519-25. [Crossref] [PubMed]
- Gasparovic C, Prestopnik J, Thompson J, Taheri S, Huisa B, Schrader R, Adair JC, Rosenberg GA. 1H-MR spectroscopy metabolite levels correlate with executive function in vascular cognitive impairment. J Neurol Neurosurg Psychiatry 2013;84:715-21. [Crossref] [PubMed]
- Xu S, Zhu W, Wan Y, Wang J, Chen X, Pi L, Lobo MK, Ren B, Ying Z, Morris M, Cao Q. Decreased Taurine and Creatine in the Thalamus May Relate to Behavioral Impairments in Ethanol-Fed Mice: A Pilot Study of Proton Magnetic Resonance Spectroscopy. Mol Imaging 2018;17:1536012117749051 [Crossref] [PubMed]
- Jessen F, Gür O, Block W, Ende G, Frölich L, Hammen T, Wiltfang J, Kucinski T, Jahn H, Heun R, Maier W, Kölsch H, Kornhuber J, Träber F. A multicenter (1)H-MRS study of the medial temporal lobe in AD and MCI. Neurology 2009;72:1735-40. [Crossref] [PubMed]
- Franczak M, Prost RW, Antuono PG, Mark LP, Jones JL, Ulmer JL. Proton magnetic resonance spectroscopy of the hippocampus in patients with mild cognitive impairment: a pilot study. J Comput Assist Tomogr 2007;31:666-70. [Crossref] [PubMed]
- Chawla S, Wang S, Moore P, Woo JH, Elman L, McCluskey LF, Melhem ER, Grossman M, Poptani H. Quantitative proton magnetic resonance spectroscopy detects abnormalities in dorsolateral prefrontal cortex and motor cortex of patients with frontotemporal lobar degeneration. J Neurol 2010;257:114-21. [Crossref] [PubMed]
- Modrego PJ, Fayed N, Pina MA. Conversion from mild cognitive impairment to probable Alzheimer's disease predicted by brain magnetic resonance spectroscopy. Am J Psychiatry 2005;162:667-75. [Crossref] [PubMed]
- Modrego PJ, Fayed N, Sarasa M. Magnetic resonance spectroscopy in the prediction of early conversion from amnestic mild cognitive impairment to dementia: a prospective cohort study. BMJ Open 2011;1:e000007 [Crossref] [PubMed]
- Yang ZX, Huo SS, Cheng XF, Xu ZF, Cao Z, Zeng JX, Xiao YY, You KZ, Chen W, Liu YY, Wu RH. Quantitative multivoxel proton MR spectroscopy study of brain metabolites in patients with amnestic mild cognitive impairment: a pilot study. Neuroradiology 2012;54:451-8. [Crossref] [PubMed]
- Zimny A, Szewczyk P, Trypka E, Wojtynska R, Noga L, Leszek J, Sasiadek M. Multimodal imaging in diagnosis of Alzheimer’s disease and amnestic mild cognitive impairment: value of magnetic resonance spectroscopy, perfusion, and diffusion tensor imaging of the posterior cingulate region. J Alzheimers Dis 2011;27:591-601. [Crossref] [PubMed]
- Tumati S, Martens S, Aleman A. Magnetic resonance spectroscopy in mild cognitive impairment: systematic review and meta-analysis. Neurosci Biobehav Rev 2013;37:2571-86. [Crossref] [PubMed]
- Li H, Xu H, Zhang Y, Guan J, Zhang J, Xu C, Shen Z, Xiao B, Liang C, Chen K, Zhang J, Wu R. Differential neurometabolite alterations in brains of medication-free individuals with bipolar disorder and those with unipolar depression: a two-dimensional proton magnetic resonance spectroscopy study. Bipolar Disord 2016;18:583-90. [Crossref] [PubMed]
- Liu YY, Yang ZX, Shen ZW, Xiao YY, Cheng XF, Chen W, Chen YW, Wu RH. Magnetic Resonance Spectroscopy Study of Amnestic Mild Cognitive Impairment and Vascular Cognitive Impairment With No Dementia. Am J Alzheimers Dis Other Demen 2014;29:474-81. [Crossref] [PubMed]
- Zhang Z, Wang Y, Shen Z, Yang Z, Li L, Chen D, Yan G, Cheng X, Shen Y, Tang X, Hu W, Wu R. The Neurochemical and Microstructural Changes in the Brain of Systemic Lupus Erythematosus Patients: A Multimodal MRI Study. Sci Rep 2016;6:19026. [Crossref] [PubMed]
- Folstein MF, Folstein SE, McHugh PR. "Mini-mental state". A practical method for grading the cognitive state of patients for the clinician. J Psychiatr Res 1975;12:189-98. [Crossref] [PubMed]
- Lu J, Li D, Li F, Zhou A, Wang F, Zuo X, Jia XF, Song H, Jia J. Montreal cognitive assessment in detecting cognitive impairment in Chinese elderly individuals: a population-based study. J Geriatr Psychiatry Neurol 2011;24:184-90. [Crossref] [PubMed]
- Morris JC. The Clinical Dementia Rating (CDR): current version and scoring rules. Neurology 1993;43:2412-4. [Crossref] [PubMed]
- Reisberg B, Ferris SH, de Leon MJ, Crook T. The Global Deterioration Scale for assessment of primary degenerative dementia. Am J Psychiatry 1982;139:1136-9. [Crossref] [PubMed]
- Xu Y, Chen K, Zhao Q, Li F, Guo Q. Short-term delayed recall of auditory verbal learning test provides equivalent value to long-term delayed recall in predicting MCI clinical outcomes: A longitudinal follow-up study. Appl Neuropsychol Adult 2020;27:73-81. [Crossref] [PubMed]
- Barberger-Gateau P, Commenges D, Gagnon M, Letenneur L, Sauvel C, Dartigues JF. Instrumental activities of daily living as a screening tool for cognitive impairment and dementia in elderly community dwellers. J Am Geriatr Soc 1992;40:1129-34. [Crossref] [PubMed]
- Larson DB, Lyons JS, Bareta JC, Burns BJ, Blazer DG, Goldstrom ID. The construct validity of the ischemic score of Hachinski for the detection of dementias. J Neuropsychiatry Clin Neurosci 1989;1:181-7. [Crossref] [PubMed]
- Worboys M. The Hamilton Rating Scale for Depression: The making of a “gold standard” and the unmaking of a chronic illness, 1960-1980. Chronic Illn 2013;9:202-19. [Crossref] [PubMed]
- Petersen RC, Doody R, Kurz A, Mohs RC, Morris JC, Rabins PV, Ritchie K, Rossor M, Thal L, Winblad B. Current concepts in mild cognitive impairment. Arch Neurol 2001;58:1985-92. [PubMed]
- Mitolo M, Stanzani-Maserati M, Capellari S, Testa C, Rucci P, Poda R, Oppi F, Gallassi R, Sambati L, Rizzo G, Parchi P, Evangelisti S, Talozzi L, Tonon C, Lodi R, Liguori R. Predicting conversion from mild cognitive impairment to Alzheimer’s disease using brain 1H-MRS and volumetric changes: A two-year retrospective follow-up study. Neuroimage Clin 2019;23:101843 [Crossref] [PubMed]
- Zhu X, Schuff N, Kornak J, Soher B, Yaffe K, Kramer JH, Ezekiel F, Miller BL, Jagust WJ, Weiner MW. Effects of Alzheimer disease on fronto-parietal brain N-acetyl aspartate and myo-inositol using magnetic resonance spectroscopic imaging. Alzheimer Dis Assoc Disord 2006;20:77-85. [Crossref] [PubMed]
- Waragai M, Moriya M, Nojo T. Decreased N-Acetyl Aspartate/Myo-Inositol Ratio in the Posterior Cingulate Cortex Shown by Magnetic Resonance Spectroscopy May Be One of the Risk Markers of Preclinical Alzheimer’s Disease: A 7-Year Follow-Up Study. J Alzheimers Dis 2017;60:1411-27. [Crossref] [PubMed]
- Perrotin A, de Flores R, Lamberton F, Poisnel G, La Joie R, de la Sayette V, Mézenge F, Tomadesso C, Landeau B, Desgranges B, Chételat G. Hippocampal Subfield Volumetry and 3D Surface Mapping in Subjective Cognitive Decline. J Alzheimers Dis 2015;48:S141-50. [Crossref] [PubMed]
- Watanabe T, Shiino A, Akiguchi I. Absolute quantification in proton magnetic resonance spectroscopy is useful to differentiate amnesic mild cognitive impairment from Alzheimer’s disease and healthy aging. Dement Geriatr Cogn Disord 2010;30:71-7. [Crossref] [PubMed]
- Heales SJ, Davies SE, Bates TE, Clark JB. Depletion of brain glutathione is accompanied by impaired mitochondrial function and decreased N-acetyl aspartate concentration. Neurochem Res 1995;20:31-8. [Crossref] [PubMed]
- Liang S, Huang J, Liu W, Jin H, Li L, Zhang X, Nie B, Lin R, Tao J, Zhao S, Shan B, Chen L. Magnetic resonance spectroscopy analysis of neurochemical changes in the atrophic hippocampus of APP/PS1 transgenic mice. Behav Brain Res 2017;335:26-31. [Crossref] [PubMed]
- Glodzik L, Sollberger M, Gass A, Gokhale A, Rusinek H, Babb JS, Hirsch JG, Amann M, Monsch AU, Gonen O. Global N-acetylaspartate in normal subjects, mild cognitive impairment and Alzheimer’s disease patients. J Alzheimers Dis 2015;43:939-47. [Crossref] [PubMed]
- Madhavarao CN, Chinopoulos C, Chandrasekaran K, Namboodiri MA. Characterization of the N-acetylaspartate biosynthetic enzyme from rat brain. J Neurochem 2003;86:824-35. [Crossref] [PubMed]
- Schofield E, Kersaitis C, Shepherd CE, Kril JJ, Halliday GM. Severity of gliosis in Pick’s disease and frontotemporal lobar degeneration: tau-positive glia differentiate these disorders. Brain 2003;126:827-40. [Crossref] [PubMed]
- Voevodskaya O, Sundgren PC, Strandberg O, Zetterberg H, Minthon L, Blennow K, Wahlund LO, Westman E, Hansson O. Swedish BioFINDER study group. Myo-inositol changes precede amyloid pathology and relate to APOE genotype in Alzheimer disease. Neurology 2016;86:1754-61. [Crossref] [PubMed]
- Majós C, Alonso J, Aguilera C, Serrallonga M, Acebes JJ, Arús C, Gili J. Adult primitive neuroectodermal tumor: proton MR spectroscopic findings with possible application for differential diagnosis. Radiology 2002;225:556-66. [Crossref] [PubMed]
- Huang C, Wahlund LO, Svensson L, Winblad B, Julin P. Cingulate cortex hypoperfusion predicts Alzheimer's disease in mild cognitive impairment. BMC Neurol 2002;2:9. [Crossref] [PubMed]
- Watanabe T, Shiino A, Akiguchi I. Absolute quantification in proton magnetic resonance spectroscopy is superior to relative ratio to discriminate Alzheimer's disease from Binswanger's disease. Dement Geriatr Cogn Disord 2008;26:89-100. [Crossref] [PubMed]
- Corballis MC. Evolution of cerebral asymmetry. Prog Brain Res 2019;250:153-78. [Crossref] [PubMed]
- Keller SS, Roberts N, Baker G, Sluming V, Cezayirli E, Mayes A, Eldridge P, Marson AG, Wieshmann UC. A voxel-based asymmetry study of the relationship between hemispheric asymmetry and language dominance in Wada tested patients. Hum Brain Mapp 2018;39:3032-45. [Crossref] [PubMed]
- Watanabe T, Shiino A, Akiguchi I. Hippocampal metabolites and memory performances in patients with amnestic mild cognitive impairment and Alzheimer's disease. Neurobiol Learn Mem 2012;97:289-93. [Crossref] [PubMed]
- Zhou IY, Ding AY, Li Q, McAlonan GM, Wu EX. Magnetic resonance spectroscopy reveals N-acetylaspartate reduction in hippocampus and cingulate cortex after fear conditioning. Psychiatry Res 2012;204:178-83. [Crossref] [PubMed]
- Jessen F, Spottke A, Boecker H, Brosseron F, Buerger K, Catak C, et al. Design and first baseline data of the DZNE multicenter observational study on predementia Alzheimer's disease (DELCODE). Alzheimers Res Ther 2018;10:15. [Crossref] [PubMed]
- Modrego PJ, Fayed N. Longitudinal magnetic resonance spectroscopy as marker of cognitive deterioration in mild cognitive impairment. Am J Alzheimers Dis Other Demen 2011;26:631-6. [Crossref] [PubMed]
- Zhang C, Kong M, Wei H, Zhang H, Ma G, Ba MAlzheimer’s Disease Neuroimaging Initiative. The effect of ApoE ε 4 on clinical and structural MRI markers in prodromal Alzheimer's disease. Quant Imaging Med Surg 2020;10:464-74. [Crossref] [PubMed]
- Costigan AG, Umla-Runge K, Evans CJ, Hodgetts CJ, Lawrence AD, Graham KS. Neurochemical correlates of scene processing in the precuneus/posterior cingulate cortex: A multimodal fMRI and 1H-MRS study. Hum Brain Mapp 2019;40:2884-98. [Crossref] [PubMed]
- Wei H, Kong M, Zhang C, Guan L, Ba M. for Alzheimer’s Disease Neuroimaging Initiative*. The structural MRI markers and cognitive decline in prodromal Alzheimer’s disease: a 2-year longitudinal study. Quant Imaging Med Surg 2018;8:1004-19. [Crossref] [PubMed]
- Yang Z, Rong Y, Cao Z, Wu Y, Zhao X, Xie Q, Luo M, Liu Y. Microstructural and Cerebral Blood Flow Abnormalities in Subjective Cognitive Decline Plus: Diffusional Kurtosis Imaging and Three-Dimensional Arterial Spin Labeling Study. Front Aging Neurosci 2021;13:625843 [Crossref] [PubMed]