A subregion-based positron emission tomography/computed tomography (PET/CT) radiomics model for the classification of non-small cell lung cancer histopathological subtypes
Introduction
Lung cancer is a fatal disease with a high incidence rate in Asia (up to 520,000 cases per year in China), and has the highest mortality among all the cancers with a poor 5-year survival rate (1). According to histological and cytological tumor types, lung cancer can be divided into small cell lung cancer (SCLC, ~15%) and non-small cell lung cancer (NSCLC, ~85%). Adenocarcinoma (ADC) and squamous cell carcinoma (SCC) are the 2 main histopathological subtypes of NSCLC, accounting for approximately 80% of cases (2). Classifying SCC and ADC is clinically important and heavily influences clinical decision-making (3,4). ADC easily metastasizes in the early stage. The most common sites of metastasis are the liver, bone, brain, and adrenal glands. ADC is sensitive to radiotherapy and chemotherapy, but can easily develop resistance to chemotherapy drugs (5). On the other hand, the development of SCC is relatively slow, and metastasis is late. Thus, resection surgery is normally applied rather than chemotherapy and radiotherapy (6). Classifying SCC and ADC helps to determine the optimal clinical treatment and improve the 5-year survival rate and postoperative quality of life of patients.
Biopsy or surgical resection is often used to determine the histopathological subtypes of lung cancer, and is considered to be the gold standard. However, this method is invasive and requires random sampling of tumor fragments (7). In contrast, radiomics analysis (8,9) is considered to be a noninvasive tool for the classification of lung cancer histopathological subtypes through medical image analysis, which offers quantitative tumor heterogeneity information (10,11) by quantitatively describing shapes, gray level histograms, or textures (12,13). Radiomics features often include Haralick features (14), Gabor features (15), histograms of oriented gradients (HOG) (16), and the co-occurrence of local anisotropic gradient orientations (CoLIAGe) (17). Gabor features are extracted from the image transformed by Gabor filters, which are a set of linear filters for edge detection. Thus, these features can capture the textural information in terms of different frequencies and orientations. HOG features, which are initially used for pedestrian detection, acquire information based on the histograms of local gradient cells. CoLIAGe features are the improved version of HOG, capturing anisotropic tensor gradient differences across similar appearing pathologies in medical images.
Furthermore, researchers have shown that radiomics is a noninvasive method for helping to distinguish ADC from SCC. For example, Haga et al. (7) evaluated and demonstrated the potential of radiomics analysis of computed tomography (CT) images to predict the histological subtypes of NSCLC (SCC and ADC), with an area under the curve (AUC) value of 0.7250±0.070. Ferreira Junior et al. (18) obtained an AUC value of 0.81 from their radiomics models for the classification of SCC and ADC in CT images. Wu et al. (19) performed a multicohort radiomics study for differentiating between NSCLC histological subtypes (ADC vs. SCC), with an AUC value of 0.72.
In addition to CT images, positron emission tomography/CT (PET/CT), which is considered to be a powerful and widely used system for malignant tumor imaging, not only offers information on both biological metabolism and accurate anatomical location, but also plays an important role in staging and therapy monitoring in numerous tumors (20). Tsubakimoto et al. (21) developed a binary logistic regression model based on skewness and kurtosis features from CT, as well as the standardized uptake value (SUV)max feature from PET. Their results showed that the PET/CT-based model outperformed the other models that were based on features from a single modality. In addition to NSCLC subtype classification, Dissaux et al. (22) demonstrated that PET/CT-based radiomics has the potential to prognostically predict local recurrence for patients who received radiotherapy.
On top of the aforementioned radiomics studies, which are based on the features extracted from the whole tumor, a novel approach (called subregion-based radiomics) takes intratumoral regional heterogeneity into account (23). This approach is based on the premise that the tumor is heterogeneous and does not share the same heterogeneous pattern across the entire tumor (24). Specifically, within a single tumor, intratumoral phenotypes vary (e.g., necrotic or highly active features), which may reflect different biological processes (24,25). Subregion-based radiomics (26) is an effective solution to address this problem, as it first divides the whole tumor into several subregions and then develops a comprehensive radiomics model according to the features extracted from these subregions. By considering the intratumoral regional heterogeneity, grouping variant heterogeneities across the tumor may improve the performance of the conventional radiomics method. Thus far, researchers have applied subregion-based radiomics to determine the prognosis of breast cancer (27), nasopharyngeal carcinoma (28), and esophageal squamous cell carcinoma (26). However, to date, no studies have explored the classification of lung ADC and SCC according to subregion-based radiomics analysis with PET/CT scans. In addition, the optimized subregion generation method for this classification also needs to be studied.
Therefore, the aim of this study was to develop a subregion-based radiomics model for histopathological classification with PET/CT images. We also evaluated different feature vectors for subregion generation to achieve the best histopathological classification results.
Methods
Data
This study was approved by the PET Center of the First Affiliated Hospital, College of Medicine, Zhejiang University. A total of 250 patients who underwent PET/CT scanning and had primary lung cancers were enrolled in this study (Table 1). All tumors were histopathologically determined to be 1 of the 2 subtypes of NSCLC (using surgical resection specimens), with 103 SCC tumors and 160 ADC tumors. All volumes of interest (VOIs) of lung tumors were semiautomatically delineated by a radiologist with 15 years of experience using ITK-Snap ver. 3.6.0 (29) with the Region Competition Snakes method (30) on CT. 18F-fluorodeoxyglucose (FDG) PET/CT images were scanned using PET/CT Biograph 16 (Siemens Healthineers, Hoffman Estates, IL, USA).
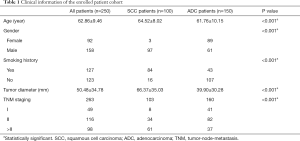
Full table
The PET images were reconstructed using the iterative algorithm with 4 iterations and 8 subsets. Normalization, decay, attenuation, random, and scatter corrections were implemented. A Gaussian filter with a full width at half maximum (FWHM) of 6.0 mm was applied to post processing. The convolutional kernel for CT reconstruction was B31f. The CT tube voltage was 120 kV, and the CT tube current was 207.5±57.6 mA. The CT exposure was 105.1±29.2 mAs. The CT pixel size was 1.00±0.10 mm, while the PET pixel size was 4.06±0.00 mm. The CT slice thickness was 4.02±0.37 mm, while the PET slice thickness was 4.94±0.42 mm. To take advantage of the size information and for uniformity, the pixel spacing and slice thickness of all images were resampled to 1 mm/voxel. All PET images were converted into a standardized uptake value (SUV).
Subregion generation
A subregion-based radiomics analysis considers the intratumoral regional heterogeneity rather than subjecting the entire tumor to a radiomics analysis (28). The entire tumor is divided into several subregions. However, most of the feature vectors for subregion generation include the information on local entropy and pixel/voxel value (26,28). In our method, we evaluated the optimized feature vector for subregion generation based on different metrics, including the 3D feature vector (whose basis is PET value, CT value, and CT local dominant orientation), 4D feature vector (whose basis is PET value, CT value, PET local entropy, and CT local entropy), and 5D feature vector (whose basis is PET value, CT value, PET local entropy, CT local entropy, and CT local dominant orientation). Taking the subregion generation based on the 3D feature vector as an example, there are 3 steps needed to obtain the subregions in our method, which are illustrated in Figure 1:
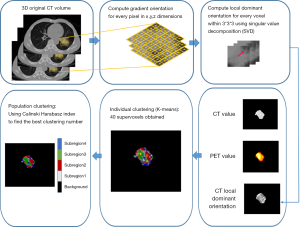
- For every voxel, the CT local dominant orientation within a small neighborhood window of 3×3×3 is computed with the help of the singular value decomposition (SVD) algorithm.
- Next, for each voxel, a feature vector (whose basis is PET value, CT value, and CT local dominant orientation) is obtained and prepared for the subsequent clustering.
- With the help of individual and population clustering, each tumor is divided into several subregions.
Note that step (I) can be replaced by different metric computations to acquire a new feature vector for the clustering in step (III).
Construction of the feature vector
Computation of the local dominant gradient orientations
For every voxel c in the tumor (denoted as c∈C), the gradients along the X, Y and Z directions are computed, and are illustrated as ∂fX(c), ∂fY(c) and ∂fZ(c). For every voxel c∈C, a 3×3×3 neighborhood window W centered around c is selected to compute the local dominant gradient orientations. Thus, for the voxels ck∈W, , the local gradient matrix , is achieved. By taking out the SVD algorithm, the most dominant components in the three directions can be derived as , , . The most dominant orientations in the neighborhood window W are then calculated as .
Computation of the local entropy
For every voxel c denoted as c∈C in the tumor, a 3×3×3 neighborhood window W centered c is chosen to calculate the local entropy H(c) according to Eq. [1]. When calculating the PET local entropy, f(ck) is the PET value of voxel ck, while in the CT local entropy calculation, f(ck)turns to the CT value of voxel ck.
Clustering subregions
Therefore, for each voxel in tumors, a feature vector (whose basis is PET value, CT value, and CT local dominant orientation) is obtained and prepared for the subsequent clustering. Individual clustering is carried out to generate subregions. Each tumor is independently divided into 40 supervoxels based on the K-means clustering algorithm. The squared Euclidean distances between voxel-wise 3D feature vectors (PET value, CT value, and CT local dominant orientation) are considered as the K-means similarity metric. Subsequently, the average feature vector is calculated for each supervoxel. Population-level clustering is performed based on the obtained 40*N supervoxels (where N is the total number of tumors). The similarity metric is the squared Euclidean distances between supervoxel-wise average 3D feature vectors. The consistently labelled supervoxels in each tumor are merged as a subregion. To determine the optimized cluster number (from 2 to 10) and obtain the final subregion results, the Calinski-Harabasz index (31) is used as the criterion, which minimizes intrasubregion variance and maximizes the intersubregion differences in feature vectors.
Feature extraction
In our experiment, 1,543 radiomics-based features (385 features in each subregion and 3 clinical features) were extracted. The bin width of CT and PET were 25 and 0.25, respectively. Among the 385 radiomics features, 191 radiomics features were from CT images and 194 were from PET ones. Among the 191 radiomics features, 107 were extracted using the open source Python toolkit, PyRadiomics 3.0 (32), and included the first-order, shape, gray-level co-occurrence matrix (GLCM) (33), gray-level run-length matrix (GLRLM) (34), gray-level size zone matrix (GLSZM) (35), gray-level dependence matrix (GLDM) (36), and neighboring gray-tone difference matrix (NGTDM) (37). Based on the CoLIAGe, 84 features were extracted (17), capturing anisotropic tensor gradient differences across similar-appearing pathologies in an image. All of the above-mentioned radiomics followed the definition in The Image Biomarker Standardization Initiative (38).
For the PET images, in addition to the 191 quantitative radiomics features extracted, which were the same as those of CT, 3 common PET semiquantitative parameters [SUVmean, SUVmax, and SUVpeak (39)] were also extracted. Finally, 3 clinical features (age, gender, and smoking history) were also taken into consideration. All of the features were normalized to [0, 1].
Feature and classifier selection
Feature selection was applied to select features that were beneficial to the classification of ADC and SCC. First, features whose variance was 0 were abandoned, and redundant features (with a Spearman’s rank coefficient greater than 0.99) were removed. Second, the chi-square test was carried out to eliminate the irrelevant features whose P value was greater than 0.05. The false discovery rate (FDR) of the Benjamini-Hochberg procedure method was utilized to perform multiple testing correction. Next, SVM-recursive feature elimination (SVM-RFE) was performed to select the optimized feature subset to distinguish ADC from SCC. The scoring metric for feature selection was the AUC, and the estimator for the RFE feature selection method was SVM-linear (kernel=‘linear’, gammar=‘scale’, min_features_to_select=3). Scikit-learn 0.23.2 (40) was used for feature selection and model development. The above 3 processes formed our feature selection pipeline.
SVM (37) has been successfully applied in numerous scenarios and offers the following advantages: (I) computational efficiency, (II) good generalization capability; and (III) satisfactory performance for a small training set. When SVM is used for RFE, SVM-RFE can be considered a sequential backward selection algorithm, and it is one of the most widely used feature selection approaches based on the wrapper method (17). SVM-RFE uses model training samples and then sorts the scores of each feature to remove the features of minimum feature score, without which, it has the maximum AUC score. It then retrains the model with the remaining features for the next iteration, and finally, selects the number of features needed. Five-fold cross-validation and stratified sampling were used to improve the generalization ability. The algorithm diagram details are shown in Figure 2. All of the samples were divided into 5 folds. Each fold was used once as the testing set, while the remaining 4 folds were the training set, which were used for feature selection and hyperparameter tuning. At each fold, the radiomics model was developed after the feature selection and hyperparameter tuning using the training set, and then the testing set used the selected features to evaluate the performance of the developed radiomics model. Notably, the testing set did not participate in any process of radiomics model development and was only used for performance evaluation.
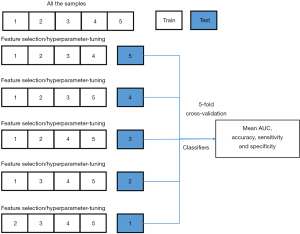
Experimental design
The goal of this study was to develop a subregion-based radiomics model for histopathological classification. The subregion-based radiomics developments followed the same pipeline as in Figure 3: (I) subregion generation based on feature vector clustering; (II) subregion-based radiomics feature extraction; (III) feature normalization and selection; and (IV) subregion-based radiomics model development. To achieve better performance, we set up an experiment for optimizing the feature vector for subregion generations. This study compared 3 kinds of feature vectors: (I) 3D vector (whose basis is PET value, CT value, and CT local dominant orientation), (II) 4D vector (whose basis is PET value, CT value, PET local entropy, and CT local entropy), and (III) 5D vector (whose basis is PET value, CT value, PET local entropy, CT local entropy, and CT local dominant orientation). By comparing their performance, we could obtain the optimized feature vector for subregion generation.
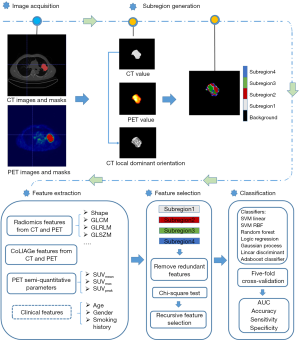
Additionally, the conventional models using the same radiomics pipeline without subregion generation were compared with the subregion-based radiomics models. In order to select the optimized model, we also compared the performance of different classifiers in this task. A total of seven classifiers were compared in terms of sensitivity, specificity, accuracy, and AUC score, including the SVM linear, SVM-RBF, random forest, logistic regression, Gaussian process classifier, linear discriminant analysis, and the AdaBoost classifier. The hyperparameter-tuning ranges of the 7 classifiers are shown in Table S1 of the Supplementary Material.
For further analysis, we used a comprehensive score to rank the features at 5 folds (as shown in Figure 4). The comprehensive score was equal to the occurrence of the feature among the 5 selected feature subsets. Through comprehensive scoring, the ranking of the selected features among the 5 folds was achieved, with the highest comprehensive score denoting the top rank.
Results
Subregion generation
According to the algorithm for the subregion generation mentioned above, the Calinski-Harabasz index was calculated as a metric to select the optimized clustering number from 2 to 10, as shown in Figure 5. We used 4 as our optimized clustering number because it corresponds to the highest Calinski-Harabasz index for the 3D feature vector. Thus, a total of 4 subregions were divided for each tumor in this study. Subregions 1 to 4 were marked by white, red, green, and blue colors, respectively. The subregions generated by 3D, 4D, and 5D feature vectors of 2 discrete slices are shown in Figure 6. The subregion numbers for the 3D, 4D, and 5D feature vectors were 4, 2, and 4, respectively.
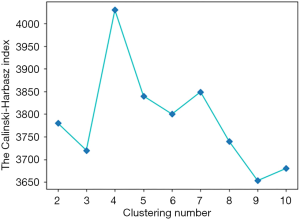
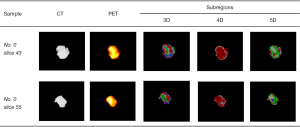
Feature selection
In the case of the subregion-based models based on the 3D or 5D feature vectors, 4 subregions were obtained, while 2 subregions were obtained based on the 4D feature vectors. Radiomics features were extracted and then passed through the feature selection process (as shown in Figure 3). As the selected features at each fold were slightly different, we employed the comprehensive score to evaluate all selected features. The result of comprehensive scoring is shown in Table 2. A total of 6 features were ranked. It should be noted that the interquartile range feature from subregion 2 in CT and the gender feature from clinical reports were selected among 5 folds.
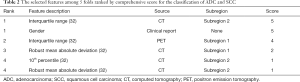
Full table
Performance evaluation of the subregion-based model
We compared the performances of the SVM with linear kernel, SVM with RBF kernel, random forest, logistic regression, Gaussian process, linear discriminant analysis, and AdaBoost methods based on the different feature vectors, which are summarized in Table 3. The subregion-based SVM-RBF with the 3D feature vector showed the best classification performance with a 5-fold cross-validation accuracy of 0.8623 and an AUC of 0.9155 among all of the results. The optimized hyperparameters are listed in Table S2 of the supplemental material. The receiver operating characteristic (ROC) curves of the conventional SVM-RBF model and those of the subregion-based SVM-RBF with the 3D feature vector are shown in Figure 7.
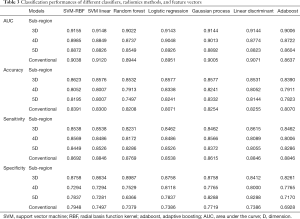
Full table
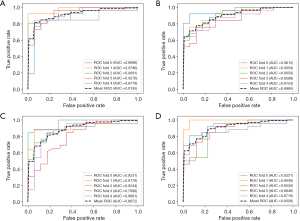
To evaluate the performance improvement, the statistical paired t-test was applied to calculate the significant difference between 7 AUCs of the conventional method and those of the subregion-based method based on the 3D feature vector. The paired t-test result showed that our proposed subregion-based radiomics model with the 3D feature vector was significantly better than the conventional radiomics model in terms of specificity, accuracy, and AUC (P=4.56e-6, P=5.12e-5, and P=4.82e-2, respectively). However, the sensitivity was significantly worse than the conventional model (P=1.71e-3).
Furthermore, the optimized basis of the feature vector was evaluated. The subregion-based models were obtained by clustering based on the feature vector. Although the most commonly used vector was the 4D feature vector (whose basis is PET value, CT value, PET local entropy, and CT local entropy), we proposed 2 novel feature vectors. They were the 3D feature vector (whose basis is PET value, CT value, and CT local dominant orientation) and the 5D feature vector (whose basis is PET value, CT value, PET local entropy, CT local entropy, and CT local dominant orientation). The method based on the 3D feature vector was also significantly better than those based on the 4D feature vector (P=3.71e-5, P=1.41e-5, and P=1.62e-3) or 5D feature vector (P=2.23e-3, P=7.17e-4, and P=1.82e-4) in terms of specificity, accuracy and AUC, respectively (Figure 7).
Discussion
In this study, we developed and evaluated subregion-based PET/CT radiomics models to classify NSCLC histopathological subtypes. We compared the performances of subregion-based radiomics based on different feature vectors for subregion generation. The results revealed that the subregion-based radiomics models generated from our proposed 3D feature vector had the best performance in the classification of ADC and SCC as compared to the models based on 4D or 5D feature vectors. The paired t-tests indicated that the 3D feature vector had a better capability to depict intratumoral regional heterogeneity of NSCLCs in PET/CT images.
Furthermore, we also compared the subregion-based models based on the 3D feature vector with conventional models. The result showed that our subregion-based radiomics models had advantages and great potential to distinguish SCC from ADC compared with the conventional radiomics models. This will improve the early diagnosis of NSCLC using intratumoral regional heterogeneity. The subregion-based models generated from the 3D feature vector surpassed the conventional models in terms of specificity, accuracy, and AUC. This implies that for lung tumors that grow with variant radiomics textural heterogeneities and that can be divided into several subregions in terms of heterogeneity, subregion-based radiomics can be applied to better improve the performance of the predictive model. This result supports the statement of Gatenby et al. (24), who argued that the whole tumor can be considered as multiple regional coalitions of ecological communities.
However, the sensitivity of the subregion-based radiomics model was significantly worse than the conventional model. Notably, the sensitivity in our study can be considered as the true ADC rate, since we assigned ADC a positive label in classification. On the other hand, the specificity in our study can be considered as the true SCC rate. It is also noteworthy that in the imbalance data set, the proportion of ADC was around 50% more than that of SCC. This indicates that the model would have better accuracy if it tended to classify the sample as ADC. In contrast, the subregion-based models based on the 3D feature vector showed better accuracy, as more SCC samples were classified correctly. This is because the size of the tumor in SCC is significantly larger than that in ADC (as shown in Table 1). Dercle et al. showed that the radiomics feature extracted from a smaller region of interest (ROI) area is more unstable (41). Moreover, a larger volume of interest (VOI) can provide more comprehensive information to cluster subregions. Thus, the subregion-based radiomics method can significantly improve the classification performance of histopathological subtypes of NSCLC as compared to the conventional method.
The results of subregion generation for 3 tumors (no. 0 and no. 23 were the SCC cases, while no. 41 was the ADC case) based on the 3D feature vector are shown in Figure 8, and for each sample, 2 discrete slices are listed. A total of 6 optimized features were ranked by comprehensive score and are shown in Table 2. There were 4 CT radiomics features, a PET radiomics feature, and a clinical feature. These provide information for the classification from anatomical, metabolic, and clinical perspectives. The two first-rank features were the interquartile range feature from subregion 2 of CT and the gender feature from clinical reports. The inclusion of the gender feature was expected since some studies have reported that gender is strongly correlated with the histopathological subtypes of NSCLC (42,43). The interquartile range feature from subregion 2 of CT measures the range from the 25th to the 75th percentile of the gray level in subregion 2 of the CT image. From Figure 8, we can see that subregion 2 of CT is mostly the inner peritumorual region, but does not include the rim region. This first-rank feature implies that the gray level distributions in this subregion are quite different between ADC and SCC, as the phenotypes of NSCLC subtypes vary.
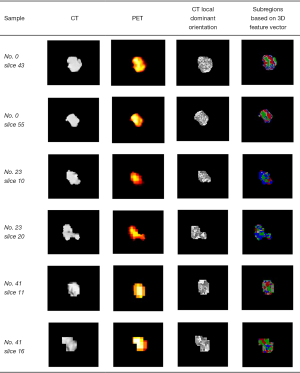
The second-rank feature is the interquartile range feature from subregion 1 of PET. Subregion 1 spreads widely and fractionally all over the tumor. This feature tells us that the PET SUV uptake in this subregion has a differential ability for classifying ADC vs. SCC, and this phenomenon may also due to the different phenotypes of NSCLC subtypes. The robust mean absolute deviation feature appears on the third and fourth rank simultaneously, but the third-rank feature is from subregion 2 of CT and the fourth-rank feature is from subregion 1 of CT. This fact demonstrates the effectiveness of subregion-based radiomics. Although the extracted features are the same, the regions to extract are different, which leads to the same feature embodying different textural/metabolic information. The other fourth-rank feature is the 10th percentile feature from subregion 2 of CT. This feature also implies that the distribution of gray level between ADC and SCC in CT is significantly different, especially the left tail part of the distribution.
For clinical application, we anticipate that the classification performance will be as good as histopathological examination, and should be available for other NSCLC subtypes, such as adenosquamous cell carcinoma (44) and large cell carcinoma. To achieve this, our future work will include more data with comprehensive histopathological subtypes. Methods to better depict intratumoral regional heterogeneity by using radiologic descriptors and building a map between the radiomics heterogeneity and the variance of clinical outcomes and molecular properties remain challenging and need to be further studied. The data used in this study were from only 1 hospital, and thus, the performance might be undermined in other hospitals due to multicenter effects, such as the differences in the scanner, scan time, dose, and reconstruction algorithm. Future work will also include data from other centers for external validation of our results.
Conclusions
Our proposed subregion-based radiomics model can successfully classify ADC and SCC using PET/CT images, which could be a promising tool to assist radiologists or medical physicists in NSCLC diagnosis. The subregion-based method demonstrates that NSCLC depicts intratumoral regional heterogeneity in both CT and PET images. Defining the intratumoral regional heterogeneity by PET/CT images results in improved diagnostic performance. The subregion-based models developed by our proposed 3D feature vector (whose basis is PET value, CT value, and CT local dominant orientation) were significantly better than those developed by 4D and 5D vectors, and thus, the 3D vector can better characterize NSCLC intratumoral regional heterogeneity.
Acknowledgments
Funding: This work was supported by the National Natural Science Foundation of China (no. 62001425) and the Key Research and Development Program of Zhejiang Province (no. 2021C03029).
Footnote
Conflicts of Interest: All authors have completed the ICMJE uniform disclosure form (available at http://dx.doi.org/10.21037/qims-20-1182). Dr. Shen reports that she has a patent pending for a diagnostic device for lung squamous cell carcinoma and adenocarcinoma based on PET/CT subregion-based radiomics features. The other authors have no conflicts of interest to declare.
Ethical Statement: This study was approved by the PET Center of the First Affiliated Hospital, College of Medicine, Zhejiang University, and the necessity to obtain informed consent was waived as the data were analyzed retrospectively and anonymously.
Open Access Statement: This is an Open Access article distributed in accordance with the Creative Commons Attribution-NonCommercial-NoDerivs 4.0 International License (CC BY-NC-ND 4.0), which permits the non-commercial replication and distribution of the article with the strict proviso that no changes or edits are made and the original work is properly cited (including links to both the formal publication through the relevant DOI and the license). See: https://creativecommons.org/licenses/by-nc-nd/4.0/.
References
- Xue C, Hu Z, Jiang W, Zhao Y, Xu F, Huang Y, Zhao H, Wu J, Zhang Y, Zhao L, Zhang J, Chen L, Zhang L. National survey of the medical treatment status for non-small cell lung cancer (NSCLC) in China. Lung Cancer 2012;77:371-5. [Crossref] [PubMed]
- Brainard J, Farver C. The diagnosis of non-small cell lung cancer in the molecular era. Mod Pathol 2019;32:16-26. [Crossref] [PubMed]
- Faruki H, Mayhew G, Serody JS, Hayes DN, Perou CM, Laigoldman M. Lung Adenocarcinoma and Squamous Cell Carcinoma Gene Expression Subtypes Demonstrate Significant Differences in Tumor Immune Landscape. J Thorac Oncol 2017;12:943-53. [Crossref] [PubMed]
- Yue JY, Chen J, Zhou FM, Hu Y, Li MX, Wu QW, Han DM. CT-pathologic correlation in lung adenocarcinoma and squamous cell carcinoma. Medicine (Baltimore) 2018;97:e13362 [Crossref] [PubMed]
- Edwards AT. Tumours of the lung. Br J Surg 2010;26:166-92. [Crossref]
- Ganeshan B, Panayiotou E, Burnand K, Dizdarevic S, Miles KA. Tumour heterogeneity in non-small cell lung carcinoma assessed by CT texture analysis: a potential marker of survival. Eur Radiol 2012;22:796-802. [Crossref] [PubMed]
- Haga A, Takahashi W, Aoki S, Nawa K, Yamashita H, Abe O, Nakagawa K. Classification of early stage non-small cell lung cancers on computed tomographic images into histological types using radiomic features: interobserver delineation variability analysis. Radiol Phys Technol 2018;11:27-35. [Crossref] [PubMed]
- Gillies RJ, Kinahan PE, Hricak H. Radiomics: Images Are More than Pictures, They Are Data. Radiology 2016;278:563-77. [Crossref] [PubMed]
- Mao L, Chen H, Liang M, Li K, Gao J, Qin P, Ding X, Li X, Liu X. Quantitative radiomic model for predicting malignancy of small solid pulmonary nodules detected by low-dose CT screening. Quant Imaging Med Surg 2019;9:263-72. [Crossref] [PubMed]
- Kumar V, Gu Y, Basu S, Berglund A, Eschrich SA, Schabath MB, Forster KM, Aerts HJWL, Dekker A, Fenstermacher D. Radiomics: the process and the challenges. Magnetic Resonance Imaging 2012;30:1234-48. [Crossref] [PubMed]
- Liu C, Ma C, Duan J, Qiu Q, Guo Y, Zhang Z, Yin Y. Using CT texture analysis to differentiate between peripheral lung cancer and pulmonary inflammatory pseudotumor. BMC Medical Imaging 2020;20:75. [Crossref] [PubMed]
- Lambin P, Riosvelazquez E, Leijenaar RTH, Carvalho S, Van Stiphout RGPM, Granton PV, Zegers CML, Gillies RJ, Boellard R, Dekker A. Radiomics: Extracting more information from medical images using advanced feature analysis. Eur J Cancer 2012;48:441-6. [Crossref] [PubMed]
- Liu Y, Shi H, Huang S, Chen X, Zhou H, Chang H, Xia Y, Wang G, Yang X. Early prediction of acute xerostomia during radiation therapy for nasopharyngeal cancer based on delta radiomics from CT images. Quant Imaging Med Surg 2019;9:1288-302. [Crossref] [PubMed]
- Haralick RM. Textural features for image classification. IEEE Transaction on Systems, Man, and Cybernetics 1973;6:610-21. [Crossref]
- Jain AK, Farrokhnia F. Unsupervised texture segmentation using Gabor filters. Pattern Recognition 1991;24:1167-86. [Crossref]
- Dalal N, Triggs B, editors. Histograms of oriented gradients for human detection. computer vision and pattern recognition; 2005.
- Prasanna P, Tiwari P, Madabhushi A. Co-occurrence of Local Anisotropic Gradient Orientations (CoLlAGe): A new radiomics descriptor. Sci Rep 2016;6:37241. [Crossref] [PubMed]
- Ferreira JR Junior, Koenigkamsantos M, Cipriano FEG, Fabro AT, De Azevedomarques PM. Radiomics-based features for pattern recognition of lung cancer histopathology and metastases. Computer Methods and Programs in Biomedicine 2018;159:23-30. [Crossref] [PubMed]
- Wu W, Parmar C, Grossmann P, Quackenbush J, Lambin P, Bussink J, Mak RH, Aerts HJWL. Exploratory Study to Identify Radiomics Classifiers for Lung Cancer Histology. Front Oncol 2016;6:71. [Crossref] [PubMed]
- Lin J, Xie G, Liao G, Wang B, Yuan Y. Prognostic value of 18 F-FDG-PET/CT in patients with nasopharyngeal carcinoma: a systematic review and meta-analysis. Oncotarget 2017;8:33884-96. [Crossref] [PubMed]
- Tsubakimoto M, Yamashiro T, Tamashiro Y, Murayama S, Quantitative CT. Density Histogram Values and Standardized Uptake Values of FDG-PET/CT with Respiratory Gating Can Distinguish Solid Adenocarcinomas from Squamous Cell Carcinomas of the Lung. Eur J Radiol 2018;100:108-15. [Crossref] [PubMed]
- Dissaux G, Visvikis D, Da-ano R, Pradier O, Chajon E, Barillot I, Duvergé L, Masson I, Abgral R, Santiago Ribeiro M-J, Devillers A, Pallardy A, Fleury V, Mahé M-A, De Crevoisier R, Hatt M, Schick U. Pretreatment <sup>18</sup>F-FDG PET/CT Radiomics Predict Local Recurrence in Patients Treated with Stereotactic Body Radiotherapy for Early-Stage Non–Small Cell Lung Cancer: A Multicentric Study. J Nucl Med 2020;61:814-20. [Crossref] [PubMed]
- Swanton C. Intratumor Heterogeneity: Evolution through Space and Time. Cancer Res 2012;72:4875-82. [Crossref] [PubMed]
- Gatenby RA, Grove O, Gillies RJ. Quantitative Imaging in Cancer Evolution and Ecology. Radiology 2013;269:8-15. [Crossref] [PubMed]
- O’Connor JPB, Rose CJ, Waterton JC, Carano RAD, Parker GJM, Jackson A. Imaging Intratumor Heterogeneity: Role in Therapy Response, Resistance, and Clinical Outcome. Clin Cancer Res 2015;21:249-57. [Crossref] [PubMed]
- Xie C, Yang P, Zhang X, Xu L, Wang X, Li X, Zhang L, Xie R, Yang L, Jing Z. Sub-region based radiomics analysis for survival prediction in oesophageal tumours treated by definitive concurrent chemoradiotherapy. EBioMedicine 2019;44:289. [Crossref] [PubMed]
- Fan M, Cheng H, Zhang P, Gao X, Zhang J, Shao G, Li L. DCE‐MRI texture analysis with tumor subregion partitioning for predicting Ki‐67 status of estrogen receptor‐positive breast cancers. J Magn Reson Imaging 2018;48:237-47. [Crossref] [PubMed]
- Xu H, Lv W, Feng H, Du D, Yuan Q, Wang Q, Dai Z, Yang W, Feng Q, Ma J, Lu L. Subregional Radiomics Analysis of PET/CT Imaging with Intratumor Partitioning: Application to Prognosis for Nasopharyngeal Carcinoma. Mol Imaging Biol 2020;22:1414-26. [Crossref] [PubMed]
- Yushkevich PA, Gao Y, Gerig G, editors. ITK-SNAP: An interactive tool for semi-automatic segmentation of multi-modality biomedical images. Engineering in Medicine & Biology Society; 2016.
- Liu X, Tuncali K, Wells WM, Morrison PR, Zientara GP, editors. Fully automatic 3D segmentation of iceball for image-guided cryoablation. International Conference of the IEEE Engineering in Medicine & Biology Society; 2012.
- Liu Y, Li Z, Xiong H, Gao X, Wu J, Wu S. Understanding and Enhancement of Internal Clustering Validation Measures. IEEE Trans Cybern 2013;43:982-94. [Crossref] [PubMed]
- van Griethuysen JJM, Fedorov A, Parmar C, Hosny A, Aucoin N, Narayan V, Beets-Tan RGH, Fillion-Robin JC, Pieper S, Aerts HJWL. Computational Radiomics System to Decode the Radiographic Phenotype. Cancer Res 2017;77:e104-e107. [Crossref] [PubMed]
- Haralick RM, editor. Textural features for image classification. IEEE Transaction on Systems, Man, and Cybernetics. systems, man and cybernetics; 1973.
- Galloway M. Texture analysis using gray level run lengths. Computer Graphics & Image Processing 1975;4:172-9. [Crossref]
- Chu A, Sehgal CM, Greenleaf JF. Use of gray value distribution of run lengths for texture analysis. Pattern Recognition Letters 1990;11:415-9. [Crossref]
- Dasarathy BV, Holder EB. Image characterizations based on joint gray level—run length distributions. Pattern Recognition Letters 1991;12:497-502. [Crossref]
- Thibault G, Fertil B, Navarro C, L., Pereira S, Cau P, Lévy N, Sequeira J, Mari J-L, editors. Texture Indexes and Gray Level Size Zone Matrix Application to Cell Nuclei Classification. 10th International Conference on Pattern Recognition and Information Processing; 2009; Minsk, Belarus.
- Zwanenburg A, Vallières M, Abdalah MA, Aerts HJWL, Lck S. The Image Biomarker Standardization Initiative: Standardized Quantitative Radiomics for High-Throughput Image-based Phenotyping. Radiology 2020;295:328-38. [Crossref] [PubMed]
- Tahari AK, Alluri K, Quon H, Koch W, Wahl RL, Subramaniam RM. FDG PET/CT imaging of Oropharyngeal SCC: Characteristics of HPV positive and negative tumors. Clin Nucl Med 2014;39:225. [Crossref] [PubMed]
- Swami A, Jain R. Scikit-learn: Machine Learning in Python. Journal of Machine Learning Research 2013;12:2825-30.
- Dercle L, Ammari S, Bateson M, Blanc P, Eva D. Limits of radiomic-based entropy as a surrogate of tumor heterogeneity: ROI-area, acquisition protocol and tissue site exert substantial influence. Sci Rep 2017;7:7952. [Crossref] [PubMed]
- Afrose R, Akram M, Karimi AM, Siddiqui SA. Correlation of age and gender with different histological subtypes of primary lung cancer. Medical Journal of Dr DY Patil University 2015;8:447. [Crossref]
- Paggi MG, Vona R, Abbruzzese C, Malorni W. Gender-related disparities in non-small cell lung cancer. Cancer Lett 2010;298:1-8. [Crossref] [PubMed]
- Hou S, Zhou S, Qin Z, Yang L, Han X, Yao S, Ji H. Evidence, Mechanism, and Clinical Relevance of the Transdifferentiation from Lung Adenocarcinoma to Squamous Cell Carcinoma. Am J Pathol 2017;187:954-62. [Crossref] [PubMed]