Development and validation of a nomogram based on multiparametric magnetic resonance imaging and elastography-derived data for the stratification of patients with prostate cancer
Introduction
Prostate cancer (PCa) is the 2nd most common cancer among men worldwide and has the 5th highest mortality rate (1). In China, due to urbanization and an aging population, PCa has rapidly increasing morbidity, and it has risen to be the 6th most common malignancy among Chinese men (2). Ultrasound (US)-guided systematic prostate biopsy is the most frequently used diagnostic method for PCa with elevated prostate-specific antigen (PSA); however, this method can also result in unnecessary biopsy, overdiagnosis of non-significant PCa, and underdiagnosis of clinically significant PCa (SPCa) (3).
In men with elevated PSA, multiparametric magnetic resonance imaging (MP-MRI) of the prostate combined with systematic biopsy (SBS) and targeted biopsy (TBS) has been shown to improve cancer detection rates of clinically SPCa compared to SBS alone (4). MP-MRI is the most sensitive imaging technique for the detection of PCa, although it has only moderate specificity (5), and still has some limitations. For instance, benign lesions, such as prostatitis, scarring, high-grade prostatic intraepithelial neoplasia, and hyperplasia, mimic the characteristics of PCa and lower the specificity (6). Thus, some new approaches combining MP-MRI with other novel modalities, such as elastography and micro-ultrasound, are needed to more accurately predict PCa (7).
Elastography-based imaging techniques have received substantial attention in recent years for their non-invasive assessment of the mechanical properties of tissues. Such methods have proven to be promising in the non-invasive assessment of liver fibrosis, and new applications in breast, thyroid, prostate, kidney, and lymph node imaging are emerging (8). PCa lesions are often stiffer than the surrounding tissue, and elastography can reflect tissue stiffness. Elastography Q-analysis is a type of software that is preset in the equipment to analyze the mean curve of the elastic changes in the lesions. A previous primary study at our institution showed that the elastographic Q-analysis score (EQS) combined with the Prostate Imaging Reporting and Data System (PI-RADS) score in patients undergoing fusion-TBS (FTBS) could improve PCa diagnosis (9). US/MP-MRI FTBS-based nomograms have been created to predict PCa (10); however, very few nomograms have used a volume-navigation–based prostate EQS combined with PI-RADS, clinical parameters, and FTBS pathological data. This study sought to develop and validate a risk-stratified nomogram combining EQS, MP-MRI, clinical parameters, and FTBS pathological data which can aid clinicians and patients in deciding whether a prostate biopsy is necessary.
Methods
Patients and study design
We retrospectively analyzed 536 patients who underwent volume-navigation transrectal ultrasound (TRUS)-MP-MRI FTBS between April 2017 and January 2020 at Shenzhen People’s Hospital. To be eligible to participate in this study, patients had to meet the following inclusion criteria: (I) underwent 3.0-T MRI of the prostate at our hospital because of suspicious results of a digital rectal examination (DRE), and/or (II) had an elevated PSA level of ≥10 ng/mL or a PSA level of 4–10 µg/L with abnormal free/total (F/T) PSA and PSA density (PSAD). Baseline clinicopathologic data, including age, PSA, free PSA (FPSA), prostate volume (PV), FPSA/PSA (F/T), PASD, PI-RADS, EQS, and FTBS pathology data, were collected. According to the FTBS pathology data, the diagnoses were defined as no cancer, low-risk PCa [Gleason score (GS) ≤3+4], or high-risk PCa (GS ≥4+3) (11-13). The following were excluded: (I) patients who had a previous history of PCa; (II) patients lacking biopsy pathology results; and (III) patients with missing elastographic or clinical parameters. The study was approved by Shenzhen People’s Hospital Ethics Committee and all patients provided informed consent.
MRI
All patients underwent MRI using a Siemens Magnetom Skyra 3.0-T scanner (SIEMENS, Germany) with a superconductive magnet before receiving a biopsy. The sequences of examination mainly included T2-weighted imaging, diffusion-weighted imaging, and dynamic contrast-enhanced imaging. All MP-MRI scans were performed at our institution according to the PI-RADS version two criteria by two radiologists with more than 5 years’ MRI diagnostic experience (14).
Volume-navigation TRUS-MRI fusion and US elastographic Q-analysis
The LOGIQ E9 color Doppler ultrasound (GE Healthcare, Milwaukee, USA) and IC5-9-D cavity probe were used. Under the volume-navigation TRUS-MRI fusion mode, the lesion was localized using the MP-MRI images, and the size, boundary, shape, and color Doppler ultrasound of the blood flow of the lesion were assessed. Strain elastography was subsequently performed, with the lesion compressed vertically during the examination. Dynamic images were simultaneously recorded and analyzed with the EQS software preset in the instrument.
Prostate biopsy
TRUS-MRI fusion-guided TBS was performed. The TSK 18G automatic biopsy gun (ACECUT, TSK Laboratory, Tochigi, Japan) was employed for the TRUS-guided prostate biopsy. Under the navigation of the MRI-US image fusion, two biopsy cores per patient were sampled from the suspicious lesion with the highest PI-RADS score, and then the traditional 12-core SBS was performed. Finally, each core specimen was stored, labeled, fixed in 10% formaldehyde solution, and sent for pathological examination (Figure 1).
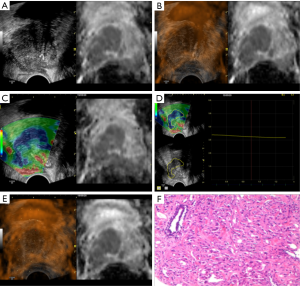
Statistical analysis
Statistical analyses were performed using R version 3.6.2 (http://www.r-project.org/). There were a number of true outliers in the PSA, FPSA, and PSAD parameters, which were converted to the edge value of the corresponding predictor. The outliers were defined according to interquartile range (IQR), and the criterion was that the PSA level exceeded the upper IQR ±1.5 IQR (15).
A model was built using the training cohort and validated in the independent validation cohort. First, the predictive factors of the model with the smallest Akaike information criterion (AIC) value were screened by stepwise regression for any PCa and high-risk PCa; a P value <0.05 was considered to show statistical significance (16). Second, multicollinearity was assessed, and the predictors were further screened to determine the final predictors of the model. Third, receiver operating characteristic (ROC) and calibration curves were derived based on the regression analysis (17). The incremental predictive value of the EQS for the nomogram was evaluated after obtaining the area under the ROC curve (AUC) and the calibration curve, and integrated discrimination improvement (IDI) was calculated (18). Finally, a decision curve analysis (DCA) was conducted to determine the clinical usefulness of the pre-biopsy PCa nomogram by quantifying the net benefits at different threshold probabilities (19).
Results
Clinical characteristics
A total of 375 patients with 375 lesions were divided into the training cohort and the validation cohort at an approximate ratio of 7 to 3. Patients diagnosed from April 2017 to June 2019 formed the training cohort (n=271), and patients diagnosed after July 2019 formed the validation cohort (n=104). The characteristics of patients in the training and validation cohorts are set out in Table 1. In the training and validation cohorts, the morbidity rates of PCa were 64.9% and 78.8%, respectively, and the proportions of high-risk PCa after undergoing a FTBS were 65.3% and 68.2%, respectively. Of the 375 patients enrolled, 5 were diagnosed with PCa after undergoing FTBS but not SBS, including 4 patients who had a GS ≤3+4, and 1 patient who had a GS of 4+4. Twenty-one patients were diagnosed with PCa after undergoing SBS but not FTBS. Twenty of these patients had a GS ≤3+4, and 1 had a GS of 4+4 (Figure 2). The distributions of the PI-RADS score and the PCa GS are set out in Table S1.
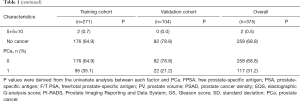
Full table
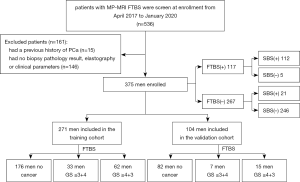
Development of a PCa risk-stratified nomogram
In the univariate analysis, all of the examined risk factors were associated with PCa (Table 1), but multicollinearity existed among PSA, FPSA, and PSAD. The predictive factors of the model with the smallest AIC was screened by stepwise regression, and the multicollinearity was eliminated.
Multivariate analysis showed that PSAD, the EQS, PI-RADS, and PV were independently associated with PCa (Table 2). These independently associated risk factors were used to develop and present a PCa risk-stratified nomogram (Figure 3).
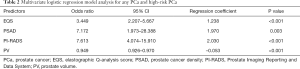
Full table
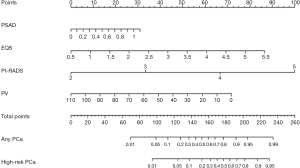
The performance of the PCa nomogram in the training cohort
The calibration curve of the nomogram for the probability of any PCa and high-risk PCa demonstrated good agreement between the predicted and observed results in the training cohort (Figure S1A,B,C,D). The Hosmer-Lemeshow test showed a non-significant difference between any PCa (P=0.1957) and high-risk PCa (P=0.3994), suggesting that the nomogram was well calibrated. In the training cohort, the AUCs of the nomogram for predicting any and high-risk PCa were 0.949 [95% confidence interval (CI), 0.921 to 0.978] and 0.936 (95% CI, 0.906 to 0.965), respectively (Figure 4A,B).
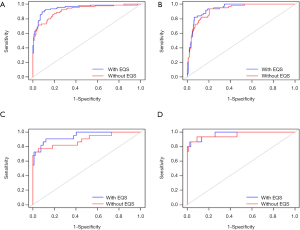
Validation of the PCa nomogram
Using 104 patients diagnosed after July 2019 as the validation cohort, the AUCs of the nomogram for predicting any PCa and high-risk PCa were 0.946 (95% CI, 0.894 to 0.997) and 0.971 (95% CI, 0.9331 to 1), respectively, with both AUCs demonstrating good discrimination (Figure 4C,D). Good calibration was observed for the probability of PCa in the validation cohort (Figure S1E,F,G,H). The Hosmer-Lemeshow test showed a non-significant difference between any PCa (P=0.6115) and high-risk PCa (P=0.6303).
Incremental predictive value of the EQS to the PCa nomogram
To examine whether the EQS was an important factor for the prediction of PCa within the nomogram, we removed the EQS and performed a ROC curve analysis. The drops in the incremental AUCs for any PCa and high-risk PCa were 0.031 and 0.015, respectively, in the training cohort (Figure 4A,B), and 0.054 and 0.010, respectively, in the validation cohort (Figure 4C,D). Not only did we observe a slightly higher AUC for the model incorporating the EQS in both the training and validation cohorts, but the integration of the EQS into the model also significantly improved the predictive performance (training cohort: IDI, 9.89%; 95% CI, 0.0651 to 0.1328, P<0.001; validation cohort: IDI, 14.67%; 95% CI, 0.0482 to 0.2452, P<0.001).
Clinical use
The DCA for the nomogram with and without the EQS is presented in Figure 5. The decision curves showed that if the threshold probability for any PCa was <67%, using the nomogram was more beneficial than biopsy or no biopsy, although beyond this range, the net benefit was comparable, with several overlaps, both with and without the EQS. However, for high-risk PCa, the net benefits of the model with and without the EQS were comparable in the validation cohort.
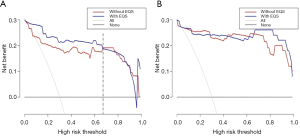
Discussion
We have developed and validated a diagnostic, risk-stratified nomogram for the pre-biopsy of PCa based on MP-MRI, the EQS, PSAD, and PV. Compared with the commonly used PI-RADS, the incorporation of the EQS and clinical risk factors into the nomogram allowed for the individualized pre-biopsy prediction of any PCa and high-risk PCa.
Based on data availability and clinical evidence, we identified age, FPSA, PSA, FT, PV, PSAD, the EQS, and PI-RADS as being predictors of PCa. However, other studies have found that some of these factors may not be useful in nomograms for the prediction of PCa. Nam et al., for instance, found that PSA had little weight for predicting the risk of PCa in their nomogram (20). Moreover, other studies have shown that PSA lacks accuracy for PCa diagnosis, which may be attributable to the influence of PV (21,22). Conversely, using PSAD can significantly improve the ability to predict clinically SPCa (23), which the results of our study confirm. A previous study also showed that the mortality rate of PCa with a GS 4+3 was three times higher than that with a GS 3+4 in prostatectomy specimens (24). According to the significant difference in prognosis, we distinguished between a GS ≤3+4 and a GS ≥4+3 to achieve a more accurate risk stratification of GS (11-13). Thus, the diagnoses in our study were defined as no cancer, low-risk PCa (GS ≤3+4), and high-risk PCa (GS ≥4+3). As DRE screening has been shown to significantly elevate the risk of overdiagnosis and overtreatment (20,25), DRE was not included in the nomogram.
Other research has found evidence of the high diagnostic accuracy of MP-MRI (26-28). A prostate biopsy under US/MP-MRI fusion can greatly improve the detection rate of clinically SPCa and contribute to a multivariate PCa prediction model, but no one has combined the EQS with MRI and clinical factors to build a PCa prediction model before, despite MRI contributing significantly to existing models (10). Chatterjee et al. (29) noted that prostate bleeding, inflammation, focal prostate atrophy, and benign prostatic hyperplasia nodules were prone to giving false-positive results in MP-MRI. Also, Sheridan et al. (30) found that in patients with PI-RADS 5, the biopsy results were inconsistent with the MRI results 28% of the time, mainly due to proliferative nodules (39%) and inflammatory changes (28%). The present study showed that most PI-RADS four patients showed no evidence of PCa (58.4% and 61.8% in the training and verification cohorts, respectively). The application of the EQS to suspicious lesions on MP-MRI could combine the advantages of the two imaging modalities and significantly improve the predictive performance of the model. With the addition of the EQS, we achieved satisfactory discrimination (training cohort: AUC, 0.949 for any PCa and 0.939 for high-risk PCa; validation cohort: AUC, 0.946 for any PCa and 0.971 for high-risk PCa). The integrated discrimination ability of the model was also significantly improved with the addition of the EQS (training cohort: IDI, 9.89%; 95% CI, 0.0651 to 0.1328, P<0.001; validation cohort: IDI, 14.67%; 95% CI, 0.0482 to 0.2452, P<0.001).
This study used elastography to improve the diagnostic accuracy. Specifically, we evaluated whether the addition of the EQS could significantly improve the net benefit for patients. We conducted a DCA and found that if the threshold probability of the for any PCa was <67%, using the nomogram carried more benefits than a biopsy or no biopsy. Outside this range, the net benefit was comparable, with several overlaps, with and without the EQS. However, for high-risk PCa, the net benefits with and without the EQS were comparable in the validation cohort, indicating that elastography has greater clinical application value for low-risk than high-risk PCa.
The decision to perform a biopsy can be complex, and predictive nomograms for PCa with varying degrees of accuracy, feasibility, and usefulness have been developed to assist clinicians in deciding whether a prostate biopsy is necessary. Thus, naïve patients with benign lesions can avoid unnecessary biopsies, and complications, such as infection and bleeding, caused by prostate biopsy can be reduced (31,32).
Our study has several limitations. First, it was conducted at a single center with strict screening criteria and a relatively small sample size. Second, we used biopsy pathologic results as the gold standard, rather than gross specimens after prostatectomy. Third, as the MRI interpretation and EQS measurement under multimodality imaging fusion must be performed by experienced doctors, the question of whether this nomogram can be used in other hospitals remains to be determined. Finally, the AUCs in our study were slightly higher than those in other reports. This may be explained by the cohort being ethnically homogeneous (almost all of the patients were Chinese). However, in our training cohort, patients with GS ≥4+3 accounted for 65.8% of patients with PCa, which precludes inferences being made about the effects of race.
In conclusion, we developed a risk-stratified nomogram combining elastography-derived data with MP-MRI and clinical parameters that can improve the prediction of PCa, especially low-risk PCa. The nomogram may assist urologists in decision-making regarding the necessity of prostate biopsy and help to avoid unnecessary biopsies as much as possible.
Acknowledgments
Funding: This project was supported by Guangdong Medical Science and Technology Research Fund Project (No. B2019112).
Footnote
Conflicts of Interest: All authors have completed the ICMJE uniform disclosure form (available at http://dx.doi.org/10.21037/qims-20-978). The authors have no conflicts of interest to declare.
Ethical Statement: The study was approved by Shenzhen People’s Hospital Ethics Committee. Patients’ written informed consent was obtained for the study.
Open Access Statement: This is an Open Access article distributed in accordance with the Creative Commons Attribution-NonCommercial-NoDerivs 4.0 International License (CC BY-NC-ND 4.0), which permits the non-commercial replication and distribution of the article with the strict proviso that no changes or edits are made and the original work is properly cited (including links to both the formal publication through the relevant DOI and the license). See: https://creativecommons.org/licenses/by-nc-nd/4.0/.
References
- Bray F, Ferlay J, Soerjomataram I, Siegel RL, Torre LA, Jemal A. Global cancer statistics 2018: GLOBOCAN estimates of incidence and mortality worldwide for 36 cancers in 185 countries. CA Cancer J Clin 2018;68:394-424. [Crossref] [PubMed]
- Chen W, Zheng R, Zhang S, Zeng H, Xia C, Zuo T, Yang Z, Zou X, He J. Cancer incidence and mortality in China, 2013. Cancer Lett 2017;401:63-71. [Crossref] [PubMed]
- Ahmed HU, El-Shater Bosaily A, Brown LC, Gabe R, Kaplan R, Parmar MK, Collaco-Moraes Y, Ward K, Hindley RG, Freeman A, Kirkham AP, Oldroyd R, Parker C, Emberton M. Diagnostic accuracy of multi-parametric MRI and TRUS biopsy in prostate cancer (PROMIS): a paired validating confirmatory study. Lancet 2017;389:815-22. [Crossref] [PubMed]
- Meng X, Rosenkrantz AB, Mendhiratta N, Fenstermaker M, Huang R, Wysock JS, Bjurlin MA, Marshall S, Deng F-M, Zhou M, Melamed J, Huang WC, Lepor H, Taneja SS. Relationship between prebiopsy multiparametric magnetic resonance imaging (MRI), biopsy indication, and MRI-ultrasound fusion-targeted prostate biopsy outcomes. Eur Urol 2016;69:512-7. [Crossref] [PubMed]
- Mortezavi A, Märzendorfer O, Donati OF, Rizzi G, Rupp NJ, Wettstein MS, Gross O, Sulser T, Hermanns T, Eberli D. Diagnostic accuracy of multiparametric magnetic resonance imaging and fusion guided targeted biopsy evaluated by transperineal template saturation prostate biopsy for the detection and characterization of prostate cancer. J Urol 2018;200:309-18. [Crossref] [PubMed]
- Epstein JI, Allsbrook WC, Amin MB, Egevad LL. Update on the Gleason grading system for prostate cancer: results of an international consensus conference of urologic pathologists. Adv Anat Pathol 2006;13:57-9. [Crossref] [PubMed]
- Rodriguez Socarras ME, Gomez Rivas J, Cuadros Rivera V, Reinoso Elbers J, Llanes Gonzalez L, Michel Mercado I, Fernandez Del Alamo J, Juarez Del Dago P, Sancha FG. Prostate mapping for cancer diagnosis: the Madrid protocol. Transperineal prostate biopsies using multiparametric magnetic resonance imaging fusion and micro-ultrasound guided biopsies. J Urol 2020;204:726-33. [Crossref] [PubMed]
- Sigrist RMS, Liau J, Kaffas AE, Chammas MC, Willmann JK. Ultrasound elastography: review of techniques and clinical applications. Theranostics 2017;7:1303-29. [Crossref] [PubMed]
- Ding Z, Ye X, Zhang L, Sun Y, Ni Z, Liu H, Xu J, Dong F. Evaluation of the performance of the ultrasound (US) elastographic Q-analysis score combined with the Prostate Imaging Reporting and Data System for malignancy risk stratification in prostate nodules based on transrectal US-magnetic resonance imaging fusion imaging. J Ultrasound Med 2019;38:2991-8. [Crossref] [PubMed]
- Radtke JP, Wiesenfarth M, Kesch C, Freitag MT, Alt CD, Celik K, Distler F, Roth W, Wieczorek K, Stock C, Duensing S, Roethke MC, Teber D, Schlemmer HP, Hohenfellner M, Bonekamp D, Hadaschik BA. Combined clinical parameters and multiparametric magnetic resonance imaging for advanced risk modeling of prostate cancer-patient-tailored risk stratification can reduce unnecessary biopsies. Eur Urol 2017;72:888-96. [Crossref] [PubMed]
- Zhu X, Gou X, Zhou M. Nomograms predict survival advantages of Gleason score 3+4 over 4+3 for prostate cancer: a SEER-based study. Front Oncol 2019;9:646. [Crossref] [PubMed]
- Lawson P, Sholl AB, Brown JQ, Fasy BT, Wenk C. Persistent homology for the quantitative evaluation of architectural features in prostate cancer histology. Sci Rep 2019;9:1139. [Crossref] [PubMed]
- Epstein JI, Zelefsky MJ, Sjoberg DD, Nelson JB, Egevad L, Magi-Galluzzi C, Vickers AJ, Parwani AV, Reuter VE, Fine SW, Eastham JA, Wiklund P, Han M, Reddy CA, Ciezki JP, Nyberg T, Klein EA. A contemporary prostate cancer grading system: a validated alternative to the Gleason score. Eur Urol 2016;69:428-35. [Crossref] [PubMed]
- Furlan A, Borhani AA, Westphalen AC. Multiparametric MR imaging of the prostate: interpretation including Prostate Imaging Reporting and Data System version 2. Urol Clin North Am 2018;45:439-54. [Crossref] [PubMed]
- Yule GU. An introduction to the theory of statistics. 5th ed. London: Charles Griffin, 1919.
- Collins GS, Reitsma JB, Altman DG, Moons KGM. Transparent reporting of a multivariable prediction model for individual prognosis or diagnosis (TRIPOD): the TRIPOD statement. BMJ 2015;350:g7594. [Crossref] [PubMed]
- Kramer AA, Zimmerman JE. Assessing the calibration of mortality benchmarks in critical care: the Hosmer-Lemeshow test revisited. Crit Care Med 2007;35:2052-6. [Crossref] [PubMed]
- Huang YQ, Liang CH, He L, Tian J, Liang CS, Chen X, Ma ZL, Liu ZY. Development and Validation of a Radiomics Nomogram for Preoperative Prediction of Lymph Node Metastasis in Colorectal Cancer. J Clin Oncol 2016;34:2157-64. [Crossref] [PubMed]
- Fitzgerald M, Saville BR, Lewis RJ. Decision curve analysis. JAMA 2015;313:409-10. [Crossref] [PubMed]
- Nam RK, Toi A, Klotz LH, Trachtenberg J, Jewett MAS, Appu S, Loblaw DA, Sugar L, Narod SA, Kattan MW. Assessing individual risk for prostate cancer. J Clin Oncol 2007;25:3582-8. [Crossref] [PubMed]
- Stamey TA, Yang N, Hay AR, McNeal JE, Freiha FS, Redwine E. Prostate-specific antigen as a serum marker for adenocarcinoma of the prostate. N Engl J Med 1987;317:909-16. [Crossref] [PubMed]
- Stamey TA, Caldwell M, McNeal JE, Nolley R, Hemenez M, Downs J. The prostate specific antigen era in the United States is over for prostate cancer: what happened in the last 20 years? J Urol 2004;172:1297-301. [Crossref] [PubMed]
- Bhat NR, Vetter JM, Andriole GL, Shetty AS, Ippolito JE, Kim EH. Magnetic resonance imaging-defined prostate-specific antigen density significantly improves the risk prediction for clinically significant prostate cancer on biopsy. Urology 2019;126:152-7. [Crossref] [PubMed]
- Stark JR, Perner S, Stampfer MJ, Sinnott JA, Finn S, Eisenstein AS, Ma J, Fiorentino M, Kurth T, Loda M, Giovannucci EL, Rubin MA, Mucci LA. Gleason score and lethal prostate cancer: does 3 + 4 = 4 + 3? J Clin Oncol 2009;27:3459-64. [Crossref] [PubMed]
- Soares SCM, de Camargo Cancela M, Migowski A, de Souza DLB. Digital rectal examination and its associated factors in the early detection of prostate cancer: a cross-sectional population-based study. BMC Public Health 2019;19:1573. [Crossref] [PubMed]
- Fütterer JJ, Briganti A, De Visschere P, Emberton M, Giannarini G, Kirkham A, Taneja SS, Thoeny H, Villeirs G, Villers A. Can clinically significant prostate cancer be detected with multiparametric magnetic resonance imaging? A systematic review of the literature. Eur Urol 2015;68:1045-53. [Crossref] [PubMed]
- Hamoen EHJ, de Rooij M, Witjes JA, Barentsz JO, Rovers MM. Use of the Prostate Imaging Reporting and Data System (PI-RADS) for prostate cancer detection with multiparametric magnetic resonance imaging: a diagnostic meta-analysis. Eur Urol 2015;67:1112-21. [Crossref] [PubMed]
- Winkel DJ, Breit HC, Shi B, Boll DT, Seifert HH, Wetterauer C. Predicting clinically significant prostate cancer from quantitative image features including compressed sensing radial MRI of prostate perfusion using machine learning: comparison with PI-RADS v2 assessment scores. Quant Imaging Med Surg 2020;10:808-23. [Crossref] [PubMed]
- Chatterjee A, Thomas S, Oto A. Prostate MR: pitfalls and benign lesions. Abdom Radiol (NY) 2020;45:2154-64. [Crossref] [PubMed]
- Sheridan AD, Nath SK, Aneja S, Syed JS, Pahade J, Mathur M, Sprenkle P, Weinreb JC, Spektor M. MRI-ultrasound fusion targeted biopsy of Prostate Imaging Reporting and Data System version 2 category 5 lesions found false-positive at multiparametric prostate MRI. AJR Am J Roentgenol 2018;210:W218-25 [Crossref] [PubMed]
- Shoag JE, Gaffney C, Pantuck M, Sun T, Gorin M, Schaeffer E, Sedrakyan A, Vickers A, Hu J. Risk factors for infection after prostate biopsy in the United States. Urology 2020;138:113-8. [Crossref] [PubMed]
- Gallina A, Suardi N, Montorsi F, Capitanio U, Jeldres C, Saad F, Graefen M, Shariat SF, Widmer H, Arjane P, Péloquin F, Perrotte P, Karakiewicz PI. Mortality at 120 days after prostatic biopsy: a population-based study of 22,175 men. Int J Cancer 2008;123:647-52. [Crossref] [PubMed]