Three-dimensional quantitative assessment of myocardial infarction via multimodality fusion imaging: methodology, validation, and preliminary clinical application
Introduction
Despite advances in myocardial infarction (MI) treatment, the damage of myocardial tissue caused by prolonged ischemia and hypoxia is generally considered irreversible (1). For patients with end-stage heart failure, the only viable option is whole heart transplantation (2). However, due to the shortage of donors’ hearts, this approach is unavailable to most patients. Thus, new techniques and therapies to repair injured myocardium are urgently needed. According to previous studies (3-5), cardiac tissue engineering is a promising alternative treatment for end-stage ischemic heart diseases (6). Three-dimensional (3D) bioprinting is an actively studied cardiac tissue engineering method, which has been shown to have good prospects and has been successfully applied in pre-clinical studies (7,8).
Today, 3D bio-printed cardiac tissue is evolving toward a custom-designed, thick patch with a blood vessel (7). The printed cardiac patch can be perfused and can maintain large-scale tissue construction functionality (9). However, this technique still has some limitations. Delimiting cardiac tissues from cardiac images currently requires a manual and subjective intervention by a clinician experienced in image analysis to ensure printing accuracy. This operator-dependent process inevitably translates into variability. More importantly, the current accuracy of infarct assessment does not yet meet the printing requirements of 3D cardiac patches. The typical approach to assessing infarction is to map the 3D volume data onto a two-dimensional (2D) plane and then generate a bull’s-eye map (10-12). However, such 3D-2D mapping leads to unavoidable distortions that affect the accuracy of quantification (13). Further, as myocardial segments are not defined regarding the ventricular septum and papillary muscles, bull’s-eye mapping does not accurately reflect patient-specific anatomical changes (14).
The 3D assessment of infarction cannot be accomplished using single-modality cardiac imaging. Given that the thickness of the coronary vessel wall is small, high-resolution gated axial computed tomography (CT) slices of 0.5 mm with adaptive statistical iteration reconstruction provide the best data source for 3D printing. Single-photon emission computed tomography (SPECT) enables high-quality examinations of cardiac perfusion and metabolism to be undertaken (10). The combined computed tomography angiography (CTA) and SPECT would facilitate the comprehensive assessment of MI post-MI based on the incremental information provided by multimodality fusion images (11,15). However, the existing CTA-SPECT registration methods use only the geometric features of the left ventricular (LV) myocardium (16-18). Registration based only on LV myocardium is not sufficiently structurally constrained and may lead to undesirable distortions. Thus, manual correction is often required in a post-process.
This paper developed a novel automatic MI assessment using customized 3D CTA-SPECT image fusion and a machine learning tool. This visual quantification method accurately assesses the size, location, and thickness of infarction and represents the infarction combined with the coronary tree in a 3D space. The method proposed in this paper could be used not only to manage patients post-MI but also to provide technical support for the design of patient-specific cardiac patches.
This study sought to: (I) describe the methodology of the 3D fusion quantitative method; (II) describe the visual quantification method of LV infarct size and validate it in animal studies; and (III) test the feasibility of the proposed method in clinical settings.
Methods
Study design
Figure 1 provides a summary of the study design. A total of 30 minipigs were used to develop porcine MI models. Of these 30 minipigs, 20 survived 3 months after operation. Rest electrocardiogram (ECG)-gated SPECT myocardial perfusion imaging and CTA were then performed 3 months post-MI in the 20 surviving minipigs. Infarct size was quantitatively assessed using a novel 3D visual quantification method. The results assessed from the CTA-SPECT were compared with those assessed from histological staining. For the clinical application, 6 patients with MI in the anterior wall and 2 patients with mild coronary stenosis were examined by coronary CTA and SPECT/CT. The LV infarct sizes were evaluated using the proposed 3D CTA-SPECT method and were then compared with results that the widely used commercial software package had calculated, Quantitative Perfusion SPECT (QPS) (Cedars-Sinai Medical Center, Los Angeles, CA).
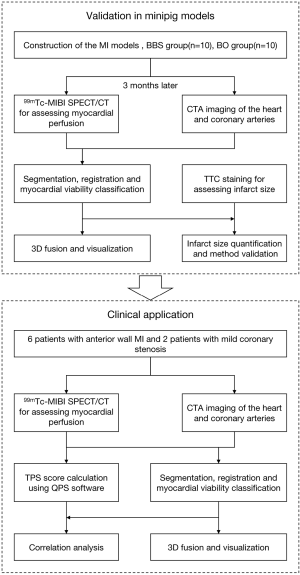
The authors are accountable for all aspects of the work, including ensuring that questions related to the accuracy or integrity of any part of the work were appropriately investigated and resolved. The experiments were approved by the Air Force Medical University Committee and conducted in compliance with the National Institutes of Health Guide for the Care and Use of Laboratory Animals and national or institutional guidelines on the care and use of animals (project license 2015-D01-07).
Anesthesia before and during operation
The minipigs were anesthetized with ketamine hydrochloride (20 mg/kg, Intramuscular), after which intravenous catheters were inserted through the marginal ear veins. Propofol (0.003 mL/kg/min) was continuously infused to maintain anesthesia. ECG monitoring was performed during the image scanning and construction of the MI model. During the MI model construction, animals were intubated and ventilated with a 1:1 mixture of air and oxygen.
Construction of MI animal model
To construct animal models of different infarct sizes, two methods were used for the left anterior descending (LAD) occlusion, that is, the balloon occlusion (BO) method or the ballon-balloon-sponge (BBS) method. The difference between the two models was the infarct size. The BO method was performed by occluding the middle LAD coronary artery with a temporary balloon for 60 minutes, followed by reperfusion. Conversely, in the BBS embolism group, animal models were constructed using the sequential embolism method as previously reported (19). A sequential embolus gradually and permanently occluded the blood flow distal to the middle segment of LAD. Figure 2 shows a comparison of the two MI models.
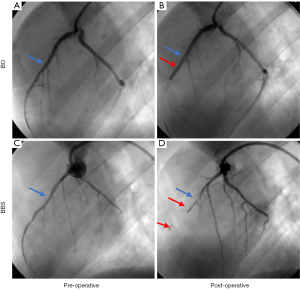
Coronary CTA imaging
The animals were placed in a right lateral recumbent position and underwent a full-body CTA scan on a dual-source 128-slice CT system (Somatom Definition Flash, Siemens Medical Systems, Forchheim, Germany). Nitroglycerin aerosol was sublingually administered to dilate the coronary artery, while heart rate was controlled within 60–80 beats per minute by esmolol hydrochloride injection (0.05–0.15 mg/kg min) to acquire high-quality images. Coronary enhancement was achieved by an intravenous injection of a contrast agent (Iohexol, Italy, 2 mL/kg) followed by a saline bolus flush. The scan was performed with the following parameters: a detector configuration of 128×0.6 mm, beam collimation of 38.4 mm, a rotation time of 300 ms, a tube voltage of 120 kV, an effective tube current 2 of 40–350 mAs, and a pitch of 0.23. A conventional retrospective ECG-gated scan was performed in the spiral mode with a phase window of 20–90% of the R–R interval. The raw CTA were reconstructed with a slice thickness of 0.75 mm (a 0.4-mm increment) and a pixel matrix of 512×512.
99mTc-MIBI SPECT/CT scanning
The SPECT/CT scans were performed using SymbiaT2 (Siemens, Germany). The animals were kept in right lateral recumbency during the entire scanning process. A non-enhanced low-dose CT scan was first performed (110 kV, 40–60 mA); the reconstructed volume had approximately 50 sections of 512×512 pixels with a detective element size of 0.98×0.98 mm2, and a section thickness of 5.00 mm. The myocardial perfusion projections were then acquired 60 minutes after a technetium-99m methoxyisobutylisonitrile (99mTc-MIBI) (0.3 mCi/kg) injection by a dual-headed camera (for which each head required 180 degrees of rotation). The camera’s analyzer was set at 140-kilo electron volts with a 20% window. The reconstructed SPECT volume comprised 64 sections of 64×64 pixels with a detective element size of 6.59×6.59 mm2, and a section thickness of 6.59 mm. The SPECT and CT images were co-registered using the integrated software Syngo MI VA30A (SIEMENS, Germany).
Histological analysis
Ex vivo histological staining with 2-, 3-, 5-triphenyl tetrazolium chloride (TTC) was performed on the last day of the study to quantify the infarct size. Upon completing the final imaging study, the animals were euthanized with intravenous potassium chloride (20 mL, 10%), and their hearts were excised and frozen. Each dissociated heart was sliced perpendicular to the heart’s long axis at a thickness of 0.4–0.5 cm. The slices were placed in 1% fresh TTC solution for 20 minutes at 37±0.2 °C. The histological slices were then placed on a coordinate paper and photographed with a digital camera. The digital images of the TTC stained heart slices were analyzed with ImageJ (US National Institutes of Health, Bethesda, MD, USA). The midline (i.e., the line at the center between the epicardial and endocardial surfaces) was drawn manually on each slice by an expert cardiologist physician. The infarct ratio (IR) was then calculated using the midline length measurement method following the guidelines for experimental models of myocardial ischemia and infarction (20,21). As the LV was dilated after MI, the infarct area wall became thinner, and the viable area became hypertrophic. The area-based method was likely to underestimate the size of the infarct, as there were opposing changes in wall thickness between dead and surviving myocardium. Conversely, the length-based method measured the extent to which the infarct scar radially covered the LV wall independent of any structural changes in the wall and thus provided a more accurate assessment.
Preliminary clinical application
With their agreement, 6 patients with anterior wall MI and 2 patients with mild coronary artery stenosis were recruited from the General Hospital of the Chinese People’s Liberation Army (Beijing). Six patients with anterior MI were studied within 1 year after first MI. Treatments were implemented following the Chinese guidelines for managing acute myocardial infarction (AMI) (22).
CTA was performed using a 128-detector CT system (Somatom Definition Flash, Siemens Medical Systems, Forchheim, Germany). Before the examination, all of the patients were instructed on breath holding to minimize artifacts during the examinations. Coronary enhancement was achieved by the intravenous injection of a contrast agent (Iohexol, Italy, 1 mL/kg) followed by a 25-mL saline bolus flush. The scanning range extended from the mid-level of the left main pulmonary artery to below the diaphragm with a trigger threshold of 100 Hounsfield unit (HU). Prospective CTA was performed with the following parameters: 128×0.6 collimation, 0.3 s gantry rotation time, 80 kV tube voltage, 205 mAs tube current, and the triggering window was set at 0–100% of the R-R interval. Pitch varied between 0.2 and 0.5 depending on heart rate and patient size.
The patients were then subjected to SPECT scanning following the scanning above procedure. The SPECT images were transformed into the polar bull’s-eye plots using QPS software (Cedars-Sinai Medical Center, Los Angeles, CA). Total perfusion deficit (TPD) scores were computed using QPS to reflect the overall perfusion defect’s extent and severity. Two experienced nuclear physicians independently analyzed the images.
Quantification
The quantitative assessment based on 3D CTA-SPECT imaging was conducted automatically with customized Matlab scripts. CTA images reconstructed from the 75% R-R interval were manually selected for analysis, which identified the best cardiac phase for coronary artery image reconstruction in most cases. The choice of the image reconstruction window was based on the recommendation of published experts (23). The complete image-processing framework is depicted in Figure 3. The processes of myocardial segmentation, multimodality image registration, myocardial classification, and quantification were automated.
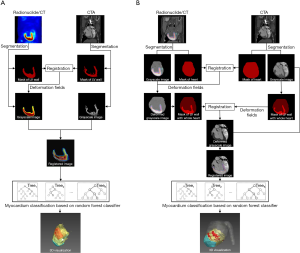
The whole heart and LV myocardium segmentation
The whole heart and LV myocardium were segmented using different strategies. Segmentations of the whole heart in CTA and CT were achieved using the multi-atlas label fusion (MALF) method, which has demonstrated superior performance in the field of automatic image segmentation (24). For the segmentation of LV myocardium in CTA, as the degree of LV dilatation varied with the location and extent of MI (25), the global, regional, and segmental myocardial deformation differed significantly between the participants. As the MALF method can only produce initial masks covering about 50% of the LV myocardium, the region growing method (26) was then employed to expand the masks with 3D active-shape models (27) as the shape constraint. For the SPECT images, the LV myocardium was marked out based on the regional tracer uptake. In this study, 50% of the peak activity was adopted for myocardium segmentation in the SPECT images (28). The whole heart and LV segmentation methods are applied to both the minipig and patient data. Concerning the patient data, the coronary trees in CTA were extracted using the region growing method.
Multimodality image registration
The registration of CTA-SPECT was performed by maximizing the similarity between the floating image and the reference image using the following transformation (T) formula:
where S denotes similarity, R denotes the reference image, and F denotes the floating image. In this paper, the mutual information metric was used for the similarity measurement. A coarse-to-fine alignment was performed to match the SPECT and CTA images. Affine transforms were first adopted to match the whole heart masks from CTs to the whole heart mask in the CTA images. The deformation fields generated in this process were propagated to the SPECT/CT gray-scale images. Next, with the additional constraint of the whole heart, the myocardium masks from the CTA images were aligned with the myocardium masks from deformed SPECT images using B-spline deformation. The deformation fields generated in this process were propagated to the CTA gray-scale images. The registration process was implemented using the Elastix toolbox (29).
The accuracy of the CTA-SPECT registration was evaluated for both of the registration methods with and without the whole heart constraint using the Dice similarity coefficient (30,31) to assess the overlap of the tracer and LV myocardium, and the Hausdorff distance (30,31) to assess the distance of the boundaries after performing the registration. The CTA-SPECT registration method was applied to both the minipig and patient data.
Myocardium classification and quantification
The myocardial voxels in the CTA-SPECT images were categorized into the following three groups: (I) infarct; (II) adjacent non-infarcted; and (III) normal perfusion (32-34). In previous SPECT studies, the myocardium has usually been graded using the threshold criteria (35-37). For example, normal perfusion was defined as a myocardial uptake greater than 70% of the maximum myocardial uptake, infarct as less than 40%, and adjacent non-infarction between 40% and 70% (38). Due to the individual variations, no fixed thresholds exist to classify myocardial voxels in all cases optimally. Thus, in the animal study, TTC was first used as the gold standard to select the optimal infarct threshold (the threshold of normal perfusion was kept as 70%) for each respective subject [threshold =40.5%±2.2%, mean ± standard deviation (SD)]. The thresholding results were further corrected manually and used as the ground-truth segmentation. A machine learning model was then built to automatically classify myocardial voxels based on the images’ local texture features (39-42) instead of any predefined thresholds. Each voxel’s local texture features were extracted from the 3×3×3 neighborhood block, including the gray-level co-occurrence matrix, neighborhood gray-tone difference matrix, and gray-level zone size matrix. The block size was an empirical parameter determined by the experiments in which the block sizes of 3, 5, and 7 were compared. The optimal classification result was achieved with a block size of 3. The random forest classifier (39) was adopted as the classification model. The random forest classifier parameters were specified as follows: the number of trees was 100, the maximum depth of a tree was 10, and the feature dimension of each tree was the square-root of the total feature dimension.
To verify the automatic classification model’s accuracy and validity, a leave-one-out cross-validation was conducted to compare the overlap of the automatic segmentation results with the ground truth. One instance was selected for evaluation of the experimental data for the 20 minipigs, and the remaining 19 instances were used for training. The average Dice similarity coefficient was up to 78.3%.
Concerning the clinical translation of the 3D quantitative analysis technique for myocardial perfusion evaluation, as the TTC staining data were not available, the machine learning model built from the animal data was directly applied to the human CTA-SPECT data to gain a better understanding of the MI situation and then optimize secondary prevention therapies and treatment.
Once the normal and infarct area was determined, the rest of the myocardial region was defined as the adjacent non-infarcted area. The IR derived from the 3D fusion image was then determined using the following midline length measurement method (20):
where MI denotes the midlines of the infarct area, m the number of infarct sections or slices, M the midlines of the myocardium per section or slice, n the number of sections or slices, and |∙| the number of pixels that comprise the midlines. The midline of each slice was extracted automatically using a curve skeletonization algorithm (43). The IRs were calculated for both the animal and patient data.
Cardiac patch design for patients
Based on the above methods, a cardiac patch with an infarct-related coronary artery was designed using the following steps. First, the main coronary branches and LV myocardium in CTA images were segmented, and the LV myocardium in the SPECT image was also segmented. Next, the myocardium areas in the CTA and SPECT images were registered, and the infarct myocardium area was identified using the myocardium classification method. Finally, a cardiac patch was obtained by merging the infarct myocardium area and the corresponding coronary main branches. The data file was then exported to a 3D medical image-processing software (3D Slicer, www.slicer.org) program and converted to stereolithography (STL) format for 3D printing.
Statistical analysis
Descriptive statistics were calculated using SPSS version 26.0.0 (SPSS, Chicago, IL). Data were reported as mean ± SD, median, interquartile range (IQR), and percentages. A univariate linear regression analysis was conducted to evaluate the relationship between the infarct size assessed using the 3D fusion learning model and TTC pathology, and Bland-Altman analysis was conducted to determine agreement. Spearman’s correlation was used to analyze the correlation between the infarct size assessed with the 3D CTA-SPECT method and TPD score derived from QPS. A P<0.05 was considered statistically significant.
Results
Completion of the experiment
As stated above, in total, 20 minipigs with MI underwent the imaging procedure. SPECT and CTA imaging were completed for both animals and patients. Restenosis and new coronary atherosclerosis were not found in the CTA images of the MI patients. Two people whose coronary angiography showed mild coronary stenosis 2 years ago underwent CTA and SPECT scans, and the results still showed mild stenosis and normal perfusion. The previous invasive coronary angiographie (ICA) images of all patients were successfully retrieved; thus, 3 sets of image data were available for each enrolled patient.
Multimodality image registration
The automatic registration of the CTA and the SPECT/CT was successfully performed for all experimental subjects. The Dice similarity coefficients were 0.95±0.01 and 0.91±0.01, while the Hausdorff distances were 4.26±1.21 and 9.48±1.61 mm for registration with and without the constraint of the whole heart, respectively. A higher Dice similarity was obtained by registration with the whole heart’s constraint, indicating a better overlap of the radiotracer signal with the myocardium region by using the whole heart constraint method instead of the LV myocardium-alone method. Similarly, the whole-heart constrained approach yielded lower Hausdorff distances. Compared to the LV myocardium-alone method, the deviation between the LV myocardial contours in SPECT/CT and CTA was reduced using the whole-heart constraint registration method. The evaluations of the two registration methods have also been represented in a bar graph (see Figure 4A). Registration with the whole heart’s constraint was superior to the LV myocardium-alone method (see Figure 4B).
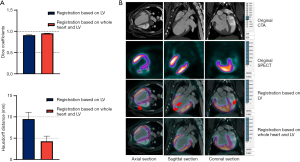
Infarct size analysis of 3D LV myocardium
The function of the LV myocardium was successfully analyzed in all animal experimental subjects. Figure 5A shows four examples of CTA-SPECT fusion images. Concerning the minipigs in the BBS group (Subject 1), the defect appeared in the apical or anterior wall (IR =30.85%). Concerning the minipigs in the BO group (Subject 2), the defect only appeared in the anterior wall segments of the LV (IR =13.12%). The minipigs with larger infarctions tended to have larger dilated cardiac chambers.
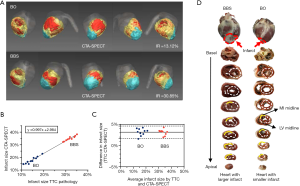
For all of the 20 minipigs, the IR with CTA-SPECT images was 27.6% (IQR, 17.1–34.7%), and the IR with TTC images was 24.1% (IQR, 14.7–32.5%). The linear regression analysis yielded an R-squared value of 0.99 (P<0.01). The regression curve slope was 0.995 (95% confidence interval) (see Figure 5B). Bland-Altman analyses demonstrated good consistency in assessing infarct size between CTA-SPECT and TTC (see Figure 5C). In addition, the infarct positions shown in the CTA-SPECT images were also consistent with those shown in the TTC (see Figure 5D).
In the animal experiments, we also analyzed CTA images reconstructed in systole using the proposed method. There was a significant difference between the IRs as assessed by the proposed method and the TTC. The average absolute error was 8.42%, and the absolute minimum error was up to 6.41%. The large error was attributed to the hearts of the minipigs being kept in the diastolic phase when they were euthanized. Thus, it should be noted that the cardiac phases of the pathological sections and non-invasive images should be consistent when building the machine learning model.
Preliminary clinical application
Table 1 shows the patient characteristics. The automatic registration of the CTA and the SPECT/CT images were successfully performed in all clinical cases. The IRs of all 8 clinical cases were quantified using the proposed 3D fusion method. The correlations between the IRs with the TPD scores were analyzed using Spearman’s rank test. The results revealed a rank correlation of 0.976 with a P value <0.01 [IR: 11.0% (IQR, 0.4–17.8%) vs. TPD score: 21.0% (IQR, 2.3–36.0%)], suggesting a significant correlation between the IR and TPD score. Notably, the median of the TPD score (21.0%) was greater than that of the IR (11.0%). This is because the TPD score reflects not the size of the infarct but the TPD. However, the perfusion deficient myocardium is the infarcted myocardium and includes the hypoperfused but viable myocardium (44).
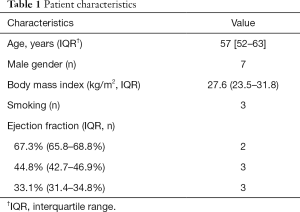
Full table
Patient-specific cardiac patches can now be designed based on analysis results. Figure 6 shows two representative examples of clinical cases, including ICA, SPECT, and the 3D volume rendering of CTA-SPECT fusion images. Concerning the patient with mild coronary stenosis (IR =0), ICA showed no obvious stenosis, and the SPECT showed no defect. We also found that the coronary arterial tree was comparatively intact, and the 3D fusion images showed that the LV myocardium was similar to a truncated prolate spheroid. The patient with AMI, who underwent emergency percutaneous coronary intervention, did not report any discomfort at his 1-year follow-up. However, MI can still be seen from the images intuitively and accurately. The 3D fusion images demonstrate a large infarction (the blue area) involving the interventricular septum, anterior, lateral, and apical wall of the LV. It is also easy to discern that both the LV and the whole heart were noticeably dilated and spherized. For the 3D analysis results of all patients included in the study, please refer to the Supplementary file (Appendix 1). Based on the CTA-SPECT quantitative analysis, a personalized 3D model of a cardiac patch with LAD was generated (see Figure 6).
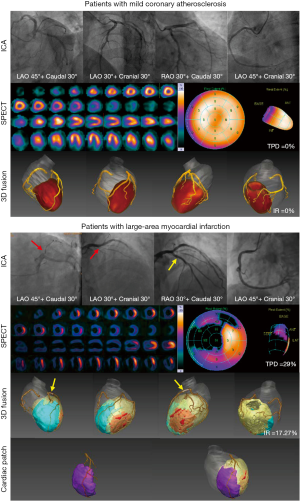
Discussion
In this study, a preliminary method was developed to assess the size of MI using clinically available imaging modalities. To the best of our knowledge, this is the first study to provide a visual quantitative analysis for the assessment of MI using a custom multimodal image fusion and machine learning approach.
The CTA-SPECT imaging and 3D fusion quantitative analysis method has been established in pre-clinical large animal imaging studies. The imaging techniques used in large animal studies are currently clinically available. As animal experiments’ imaging equipment and imaging procedures are identical to those used in clinical practice, it will be easy to transfer all the modality imaging approaches to human studies. To ensure the accurate assessment of infarcts, we used a machine learning approach to optimize the perfusion analysis. Based on the animal experiment data, a methodological assessment was conducted. The registration technique and quantification method used in the methodological assessment was related to how the data is generated but not to the object. Based on current data, we found that applying the methodological assessment of human data is reproducible. The quantitative analysis method proposed in this paper can also be applied to CTA-PET imaging. For more details on CTA-PET fusion please refer to the Supplementary file (Appendix 1).
The IR assessed by the proposed method correlated well with the histological analysis; however, it had a systematic overestimation (y-intercept 2.984; see Figure 5B). We attributed the systematic error to the low spatial resolution of SPECT/CT. The ground truth for training the machine learning model was obtained by adjusting the individual thresholds to make the IRs derived from the CTA-SPECT images as close to that in the corresponding TTC data as possible. Our experiment observed that the optimal thresholds mostly produced larger IRs, which led to the systematic overestimation of the trained classification model.
The results of 3D fusion analysis showed both the infarct, the areas of functional preservation, and the coronary tree, thus reflecting the relationship between the damaged lesion and the coronary segment. Such representations may help in the evaluation of patients with old myocardial infarct (OMI). 3D fusion analyses can determine the exact location and extent of an infarct in advance to guide operations (45), or provide technical support for the design of patient-specific cardiac patches. As the manufacturing of cardiac patches, which involves tissue engineering and biomaterials, is a complex, interdisciplinary study that requires the cooperation of researchers from different disciplines, the practical application of patching technology needs to be studied further.
Limitations
This study had several limitations. First, the sample size was not large, and only patients with anterior wall MI were involved in the preliminary clinical application. Clinical applications of the 3D fusion method require validation in large prospective cohort studies. We intend to increase the sample size and include infarction of other myocardial walls in our follow-up research. The perfusion SPECT examined in this paper was all performed using 99mTc rest protocols. The perfusion defects at rest represent scar and the hypoperfused but viable myocardium (46). For the assessment of myocardial ischemia, MPI should be performed using stress protocols (47). The ischemia caused by stenosis may not be reflected by our 3D fusion method. In this paper, the IR was calculated using the midline length-based method. In our future work, the area-based method will be considered as an option for the quantitative analysis of infarcts. Finally, this study quantified the IR without reference to the LV chamber size and wall thickness. In our follow-up study, we will further develop and validate indexes to evaluate the LV remodeling.
Conclusions
The proposed 3D fusion visual, quantitative method provides a reliable and intuitive evaluation for heart anatomical perfusion and infarction. This novel multimodality fusion visualization technique will provide whole heart quantification for infarction assessment, especially in the pre-operation evaluation and post-diagnosis management of patients.
Acknowledgments
Funding: This work was supported by the National Key Research and Development Program of China (2016YFC1300300, 2016YFA0100903), the National Natural Science Foundation of China (61976167, 91939303, 81820108019), and the Science and Technology Projects of Xi’an, China (201809170CX11JC12).
Footnote
Conflicts of Interest: All authors have completed the ICMJE uniform disclosure form (available at http://dx.doi.org/10.21037/qims-20-702). ZX, BT, FC, and JL have a patent (CN109498046A) pending. The other authors have no conflicts of interest to declare.
Ethical Statement: The experiments were approved by the Air Force Medical University Committee and conducted in compliance with the National Institutes of Health Guide for the Care and Use of Laboratory Animals and national or institutional guidelines on the care and use of animals (project license 2015-D01-07).
Open Access Statement: This is an Open Access article distributed in accordance with the Creative Commons Attribution-NonCommercial-NoDerivs 4.0 International License (CC BY-NC-ND 4.0), which permits the non-commercial replication and distribution of the article with the strict proviso that no changes or edits are made and the original work is properly cited (including links to both the formal publication through the relevant DOI and the license). See: https://creativecommons.org/licenses/by-nc-nd/4.0/.
References
- Vogel B, Claessen BE, Arnold SV, Chan D, Cohen DJ, Giannitsis E, Gibson CM, Goto S, Katus HA, Kerneis M, Kimura T, Kunadian V, Pinto DS, Shiomi H, Spertus JA, Steg PG, Mehran R. ST-segment elevation myocardial infarction. Nat Rev Dis Primers 2019;5:39. [Crossref] [PubMed]
- Stehlik J, Kobashigawa J, Hunt SA, Reichenspurner H, Kirklin JK. Honoring 50 Years of Clinical Heart Transplantation in Circulation: In-Depth State-of-the-Art Review. Circulation 2018;137:71-87. [Crossref] [PubMed]
- Shadrin IY, Allen BW, Qian Y, Jackman CP, Carlson AL, Juhas ME, Bursac N. Cardiopatch platform enables maturation and scale-up of human pluripotent stem cell-derived engineered heart tissues. Nat Commun 2017;8:1825. [Crossref] [PubMed]
- Huang K, Ozpinar EW, Su T, Tang J, Shen D, Qiao L, Hu S, Li Z, Liang H, Mathews K, Scharf V, Freytes DO, Cheng K. An off-the-shelf artificial cardiac patch improves cardiac repair after myocardial infarction in rats and pigs. Sci Transl Med 2020;12:eaat9683 [Crossref] [PubMed]
- Yeung E, Fukunishi T, Bai Y, Bedja D, Pitaktong I, Mattson G, Jeyaram A, Lui C, Ong CS, Inoue T, Matsushita H, Abdollahi S, Jay SM, Hibino N. Cardiac regeneration using human-induced pluripotent stem cell-derived biomaterial-free 3D-bioprinted cardiac patch in vivo. J Tissue Eng Regen Med 2019;13:2031-9. [Crossref] [PubMed]
- Ogle BM, Bursac N, Domian I, Huang NF, Menasche P, Murry CE, Pruitt B, Radisic M, Wu JC, Wu SM, Zhang J, Zimmermann WH, Vunjak-Novakovic G. Distilling complexity to advance cardiac tissue engineering. Sci Transl Med 2016;8:342ps13 [Crossref] [PubMed]
- Noor N, Shapira A, Edri R, Gal I, Wertheim L, Dvir T. 3D Printing of Personalized Thick and Perfusable Cardiac Patches and Hearts. Adv Sci (Weinh) 2019;6:1900344 [Crossref] [PubMed]
- Wnorowski A, Wu JC. 3-Dimensionally Printed, Native-Like Scaffolds for Myocardial Tissue Engineering. Circ Res 2017;120:1224-6. [Crossref] [PubMed]
- Murphy SV, De Coppi P, Atala A. Opportunities and challenges of translational 3D bioprinting. Nat Biomed Eng 2020;4:370-80. [Crossref] [PubMed]
- Higuchi T, Nekolla SG, Jankaukas A, Weber AW, Huisman MC, Reder S, Ziegler SI, Schwaiger M, Bengel FM. Characterization of normal and infarcted rat myocardium using a combination of small-animal PET and clinical MRI. J Nucl Med 2007;48:288-94. [PubMed]
- Lautamäki R, Schuleri KH, Sasano T, Javadi MS, Youssef A, Merrill J, Nekolla SG, Abraham MR, Lardo AC, Bengel FM. Integration of infarct size, tissue perfusion, and metabolism by hybrid cardiac positron emission tomography/computed tomography: evaluation in a porcine model of myocardial infarction. Circulation 2009;2:299-305. [PubMed]
- Feng Y, Bogaert J, Oyen R, Ni Y. An overview on development and application of an experimental platform for quantitative cardiac imaging research in rabbit models of myocardial infarction. Quant Imaging Med Surg 2014;4:358-75. [PubMed]
- Smith MF, Dilsizian V. Display of 3D Multimodality Cardiac Images With 2D Polar Maps: Simplicity Can Be a Virtue. JACC Cardiovasc Imaging 2016;9:712-4. [Crossref] [PubMed]
- . Jung J, Kim YH, Kim N, Yang DH. Patient-specific 17-segment myocardial modeling on a bull's eye map. J Appl Clin Med Phys 2016;17:453-65. [Crossref] [PubMed]
- Kyung S, Benjamin MM, Rabbat M. Exercise electrocardiography and computed tomography coronary angiography: use of combined functional and anatomical testing in stable angina pectoris. Quantitative Imaging in Medicine and Surgery 2020;10:2218-22. [Crossref] [PubMed]
- Slomka PJ, Cheng VY, Dey D, Woo J, Ramesh A, Van Kriekinge S, Suzuki Y, Elad Y, Karlsberg R, Berman DS, Germano G. Quantitative analysis of myocardial perfusion SPECT anatomically guided by coregistered 64-slice coronary CT angiography. J Nucl Med 2009;50:1621-30. [Crossref] [PubMed]
- Dongdong S, Jing W, Yue T, Kazim N, Haichang W, Chengxiang L, Xiaowei M, Yabing W, Dongjuan W, Chunhong LJT. Multimodality imaging evaluation of functional and clinical benefits of percutaneous coronary intervention in patients with chronic total occlusion lesion. Theranostics 2012;2:788-800. [Crossref] [PubMed]
- Pazhenkottil AP, Benz DC, Grani C, Madsen MA, Mikulicic F, von Felten E, Fuchs TA, Moch BH, Stehli J, Luscher TF, Gaemperli O, Buechel RR, Kaufmann PA. Hybrid SPECT Perfusion Imaging and Coronary CT Angiography: Long-term Prognostic Value for Cardiovascular Outcomes. Radiology 2018;288:694-702. [Crossref] [PubMed]
- Tao B, Gao H, Zheng M, Luo Z, Liu L, Bai W, Wang J, Liu D, Ma S, Luo Z, Gao L, Wang Y, Cao F. Preclinical modeling and multimodality imaging of chronic myocardial infarction in minipigs induced by novel interventional embolization technique. EJNMMI Res 2016;6:59. [Crossref] [PubMed]
- Takagawa J, Zhang Y, Wong ML, Sievers RE, Kapasi NK, Wang Y, Yeghiazarians Y, Lee RJ, Grossman W, Springer ML. Myocardial infarct size measurement in the mouse chronic infarction model: comparison of area- and length-based approaches. J Appl Physiol (1985) 2007;102:2104-11. [PubMed]
- Lindsey ML, Bolli R, Canty JM Jr, Du XJ, Frangogiannis NG, Frantz S, Gourdie RG, Holmes JW, Jones SP, Kloner RA, Lefer DJ, Liao R, Murphy E, Ping P, Przyklenk K, Recchia FA, Schwartz Longacre L, Ripplinger CM, Van Eyk JE, Heusch G. Guidelines for experimental models of myocardial ischemia and infarction. Am J Physiol Heart Circ Physiol 2018;314:H812-H838. [Crossref] [PubMed]
- China Society of Cardiology of Chinese Medical Association EBoCJoC, Editorial Board of Chinese Journal of Circulation. Guideline on the diagnosis and therapy of ST-segment elevation myocardial infarction. Chin J Circ 2015;16:407-22.
- Abbara S, Blanke P, Maroules CD, Cheezum M, Choi AD, Han BK, Marwan M, Naoum C, Norgaard BL, Rubinshtein R, Schoenhagen P, Villines T, Leipsic J. SCCT guidelines for the performance and acquisition of coronary computed tomographic angiography: A report of the society of Cardiovascular Computed Tomography Guidelines Committee: Endorsed by the North American Society for Cardiovascular Imaging (NASCI). J Cardiovasc Comput Tomogr 2016;10:435-49. [Crossref] [PubMed]
- Zhenzhen X, Tao B, Li Y, Zhang J, Qu X, Cao F, Liang J. 3D Fusion Framework for Infarction and Angiogenesis Analysis in a Myocardial Infarct Minipig Model. Mol Imaging 2017;16:1536012117708735 [Crossref] [PubMed]
- O’Regan DP, Shi W, Ariff B, Baksi AJ, Durighel G, Rueckert D, Cook SA. Remodeling after acute myocardial infarction: mapping ventricular dilatation using three dimensional CMR image registration. J Cardiovasc Magn Reson 2012;14:41. [Crossref] [PubMed]
- Wang XH, Liu B, Song ZQ. 3-Dimensional Brain MRI Segmentation Based on Multi-Layer Background Subtraction and Seed Region Growing Algorithm. Applied Mechanics & Materials 2014;536-537:218-21. [Crossref]
- Esfandiarkhani M, Foruzan AH. A generalized active shape model for segmentation of liver in low-contrast CT volumes. Comput Biol Med 2017;82:59-70. [Crossref] [PubMed]
- Shirasaki H, Nakano A, Uzui H, Yonekura Y, Okazawa H, Ueda T, Lee JD. Comparative assessment of 18F-fluorodeoxyglucose PET and 99mTc-tetrofosmin SPECT for the prediction of functional recovery in patients with reperfused acute myocardial infarction. Eur J Nucl Med Mol Imaging 2006;33:879-86. [Crossref] [PubMed]
- Klein S, Staring M, Murphy K, Viergever MA, Pluim JP. elastix: a toolbox for intensity-based medical image registration. IEEE Trans Med Imaging 2010;29:196-205. [Crossref] [PubMed]
- Mansilla L, Milone DH, Ferrante E. Learning deformable registration of medical images with anatomical constraints. Neural Netw 2020;124:269-79. [Crossref] [PubMed]
- Taha AA, Hanbury A. Metrics for evaluating 3D medical image segmentation: analysis, selection, and tool. BMC Med Imaging 2015;15:29. [Crossref] [PubMed]
- Akins EW, Hill JA, Sievers KW, Conti CR. Assessment of left ventricular wall thickness in healed myocardial infarction by magnetic resonance imaging. Am J Cardiol 1987;59:24-8. [Crossref] [PubMed]
- Kramer CM, Rogers WJ, Park CS, Seibel PS, Shaffer A, Theobald TM, Reichek N, Onodera T, Gerdes AM. Regional myocyte hypertrophy parallels regional myocardial dysfunction during post-infarct remodeling. J Mol Cell Cardiol 1998;30:1773-8. [Crossref] [PubMed]
- Bär C, Bernardes de Jesus B, Serrano R, Tejera A, Ayuso E, Jimenez V, Formentini I, Bobadilla M, Mizrahi J, de Martino A, Gomez G, Pisano D, Mulero F, Wollert KC, Bosch F, Blasco MA. Telomerase expression confers cardioprotection in the adult mouse heart after acute myocardial infarction. Nat Commun 2014;5:5863. [Crossref] [PubMed]
- Bonow RO. Identification of Viable Myocardium. Circulation 1996;94:2674-80. [Crossref] [PubMed]
- Srinivasan G, Kitsiou AN, Bacharach SL, Bartlett ML, Miller-Davis C, Dilsizian V. [18F]fluorodeoxyglucose single photon emission computed tomography: can it replace PET and thallium SPECT for the assessment of myocardial viability? Circulation 1998;97:843-50. [Crossref] [PubMed]
- Stirrup J, Maenhout A, Wechalekar K, Anagnostopoulos C. Radionuclide imaging in ischaemic heart failure. Br Med Bull 2009;92:43-59. [Crossref] [PubMed]
- Wawrzyniak AJ, Dilsizian V, Krantz DS, Harris KM, Smith MF, Shankovich A, Whittaker KS, Rodriguez GA, Gottdiener J, Li S, Kop W, Gottlieb SS. High Concordance Between Mental Stress-Induced and Adenosine-Induced Myocardial Ischemia Assessed Using SPECT in Heart Failure Patients: Hemodynamic and Biomarker Correlates. J Nucl Med 2015;56:1527-33. [Crossref] [PubMed]
- Cao H, Bernard S, Sabourin R, Heutte L. Random forest dissimilarity based multi-view learning for Radiomics application. Pattern Recognition 2019;88:185-97. [Crossref]
- Wan T, Cao J, Chen J, Qin Z. Automated grading of breast cancer histopathology using cascaded ensemble with combination of multi-level image features. Neurocomputing 2017;229:34-44. [Crossref]
- Chen CH, Chang CK, Tu CY, Liao WC, Wu BR, Chou KT, Chiou YR, Yang SN, Zhang G, Huang TC. Radiomic features analysis in computed tomography images of lung nodule classification. PLoS One 2018;13:e0192002 [Crossref] [PubMed]
- Qian Z, Li Y, Wang Y, Li L, Li R, Wang K, Li S, Tang K, Zhang C, Fan X, Chen B, Li W. Differentiation of glioblastoma from solitary brain metastases using radiomic machine-learning classifiers. Cancer Lett 2019;451:128-35. [Crossref] [PubMed]
- Jin D, Iyer KS, Chen C, Hoffman EA, Saha PK. A Robust and Efficient Curve Skeletonization Algorithm for Tree-Like Objects Using Minimum Cost Paths. Pattern Recognit Lett 2016;76:32-40. [Crossref] [PubMed]
- Partington SL, Kwong RY, Dorbala S. Multimodality imaging in the assessment of myocardial viability. Heart Fail Rev 2011;16:381-95. [Crossref] [PubMed]
- Sun Z. 3D printed coronary models offer new opportunities for developing optimal coronary CT angiography protocols in imaging coronary stents. Quant Imaging Med Surg 2019;9:1350-5. [Crossref] [PubMed]
- Klocke FJ, Baird MG, Lorell BH, Bateman TM, Messer JV, Berman DS, et al. ACC/AHA/ASNC guidelines for the clinical use of cardiac radionuclide imaging--executive summary: a report of the American College of Cardiology/American Heart Association Task Force on Practice Guidelines (ACC/AHA/ASNC Committee to Revise the 1995 Guidelines for the Clinical Use of Cardiac Radionuclide Imaging). J Am Coll Cardiol 2003;42:1318-33. [Crossref] [PubMed]
- Pursnani A, Lee AM, Mayrhofer T, Ahmed W, Uthamalingam S, Ferencik M, Puchner SB, Bamberg F, Schlett CL, Udelson J, Hoffmann U, Ghoshhajra BB. Early resting myocardial computed tomography perfusion for the detection of acute coronary syndrome in patients with coronary artery disease. Circ Cardiovasc Imaging 2015;8:e002404 [Crossref] [PubMed]