Improving the image quality of pediatric chest CT angiography with low radiation dose and contrast volume using deep learning image reconstruction
Introduction
Chest CT angiography (CTA) is a common clinical examination technique for identifying thoracic vessel abnormalities and has been widely used in children. It can quickly and noninvasively identify the main vascular malformation (1-3) and pulmonary malformation (4-6), especially in children experiencing refractory wheezing (7), hemoptysis (8), or repeated pneumonia (9). However, children are radiation-sensitive, and CT scans should be performed following the as low as reasonably achievable (ALARA) principle (10). In recent years, the radiation dose and contrast medium (CM) dose for CTA in children have decreased significantly with the application of low tube voltage and iterative reconstruction (IR) algorithms (11), and the combination of low tube voltage and IR algorithms such as the adaptive statistical iterative reconstruction-V (ASIR-V) is routinely used in our hospital. The latest research has shown that the use of low tube voltage, especially 70 kVp in chest CTA, can significantly improve image contrast (12-14). Simultaneously, the use of IR algorithms has been shown to reduce image noise (15-17) effectively, and the combination of these 2 methods can significantly reduce doses and maintain image quality. The exposure greatly influences image noise, and the effectiveness of IR algorithms in reducing image noise depends on their modeling capability.
Almost all currently used IR algorithms are constrained by their modeling limitation, and the non-linear and non-stationary properties of IR algorithms make the spatial frequency distribution dependent on radiation dose and the object contrast (18). Most IR algorithms are designed to have different weights, with higher weights providing more image noise reduction. However, constrained by the modeling complexity, there is a need to balance spatial frequency distribution and image noise. When high weights are used in IR algorithms, the noise power spectrum (NPS) and image texture are often changed, which gives “blotchy” and “plastic-looking” visuals (19) that differ from the look and feel of the traditional filtered back-projection (FBP) images (20). Higher weights in IR algorithms also make the images too smooth, resulting in loss of spatial resolution. These will affect the efficiency of noise reduction in IR algorithms and limit their use in clinical applications.
Recently, a deep learning image reconstruction (DLIR) algorithm was developed based on artificial intelligence and deep neural networks to overcome the usual tradeoffs among image noise, image appearance, and spatial image resolution in the conventional IR algorithms, as demonstrated in the phantom study by Greffier et al. (20). Other studies have demonstrated that using DLIR can further reduce image noise while avoiding blurring artifacts with IR algorithms. These studies focused on imaging coronary arteries, lower extremities, and the brain (21-23). As far as we know, no previous studies have applied DLIR to low radiation dose and contrast medium dose protocols in pediatric chest CTA. Thus, this study aimed to compare the quantitative and qualitative image quality of pediatric chest CTA images obtained with 70 kVp and DLIR to explore whether DLIR can further improve image quality and reduce radiation dose compared with the state-of-the-art ASIR-V algorithm.
Methods
General information
This was a retrospective study approved by our hospital’s ethics committee (Ethics reference number: 2020-Z-161), and informed consent from patients was waived. Chest CTA examinations were acquired continuously over a month from September 16, 2019, to October 20, 2019. A total of 33 children were included, including 14 boys and 19 girls, with an average age of 5.85±4.16 years (range, 4 months – 13 years). There were 17 cases with pneumonia, 8 cases with neoplasms, 5 cases with congenital lung malformations, and 3 cases with Takayasu’s arteritis. The average volume CT dose index (CTDIvol), dose length product (DLP), and contrast volume were 1.37±0.29 mGy, 35.43±10.59 mGy·cm, and 25.43±13.32 mL, respectively.
CT scan and image reconstruction
All examinations were performed on a 256-row multi-detector CT scanner (Revolution CT, GE Healthcare, USA) using a low tube voltage of 70 kVp, helical pitch value of 1.375:1 with 40 mm detector width, and 0.5 s rotation speed. The automatic tube current modulation (ATCM) technique was used with tube current in the range of 50–500 mA to achieve age-dependent noise indices between 11 and 15: 11 for 0–1 year (mAs range: 169.5–231.5 mAs), 13 for 1–7 years (mAs range: 102.0–212.5), and 15 for 7 years and older (mAs range: 53.0–201.5). All scans were performed when children were in a quiet state. For children who could not cooperate, 10% chloral hydrate at a dose of 0.4 ml/kg based on body weight was given orally, and scans did not start until patients fell asleep.
For the contrast-enhanced CT protocol, a peripheral venous cannula was pre-placed in the superficial vein of the dorsum of the hand. An iodinated contrast agent (320 mgI/mL iodixanol; GE Healthcare, USA) was administered using a single-head power injector. The contrast medium dose was calculated according to the bodyweight of each child: 1.4 mL/kg for children weighing 3–5 kg, 1.2 mL/kg for 5–10 kg, 1.1 mL/kg for 10–20 kg, 1.0 mL/kg for 20–30 kg, and 0.9 mL/kg for 30–50 kg. The flow rate was adjusted according to a fixed injection time of 15 s and the contrast-enhanced scan started 17 s after the start of contrast injection.
The raw data was reconstructed to 0.625 mm thin slice images using 3 reconstruction methods: ASIR-V with the standard 50% weight (50% ASIR-V), 100% weight (100% ASIR-V), and DLIR with a high setting (DLIR-H). All reconstructions used a standard kernel. The patient and scan information are listed in Table 1.
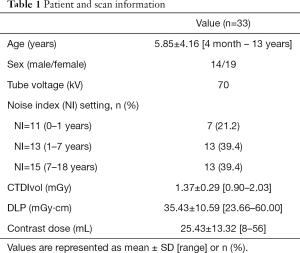
Full table
Image quality evaluation
All images were transferred to an advantage workstation (AW4.7, GE Healthcare, USA). Two observers with 15 and 8 years of experience in reviewing pediatric chest CT images evaluated the qualitative image quality using a 5-point scoring system. All patient and scanning-related information were hidden from observers. The observers could freely adjust the window width and position for observation and used multiplanar reconstructions (MPR) and three-dimensional images for the evaluation. If the scores given by the 2 observers were not the same, a third senior doctor with 20 years of experience in reviewing pediatric chest CT images evaluated the images and gave the final score. Qualitative image quality evaluation included the overall image noise, vascular structure contrast, and vascular structure edge clarity. Scores with at least 3 points were accepted for diagnosis, with 5 points being the best. The specific evaluation criteria are listed in Table 2.

Full table
After the qualitative evaluation, the 2 observers conducted quantitative measurements on the AW workstation together. They selected the largest section of the heart and set a circular region of interest (ROI) on the descending aorta (Ao) with a diameter half that of the Ao and on the back muscle (Mu) at the same imaging level to measure their CT attenuation value (in HU) and standard deviation (SD) value. The signal-to-noise ratio (SNR) and the contrast-to-noise ratio (CNR) of the descending aorta were calculated using the following formula:
Statistical analysis
All the data were expressed as mean ± standard deviation. The differences among the 3 image groups were analyzed. Continuous variables following the normal distribution were analyzed using the repeated measures analysis of variance with Bonferroni correction. The ordinal scales or variables that failed to follow the normal distribution were analyzed using Friedman’s test. P<0.05 was considered to indicate a statistically significant difference.
Results
The results of the qualitative scores and quantitative measurements are shown in Table 3.
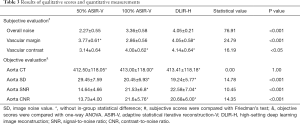
Full table
Exemplary images are shown in Figure 1. Figure 1A,B,C are the MPR images, and 1D,E,F are axial images. Figure 1G,H,I are three-dimensional images with 50% ASIR-V, 100% ASIR-V, and DLIR-H, respectively. The qualitative scoring results showed that for the overall image noise, the 50% ASIR-V image (Figure 1A) and the 100% ASIR-V image (Figure 1B) had lower qualitative scores than DLIR-H (Figure 1C), which was a statistically significant difference (P<0.001). At a 0.625 mm thin slice thickness, the 50% ASIR-V images had higher than desired image noise for the soft tissue to meet the diagnostic requirements, even though they were acceptable for analyzing the vessels. The vascular contrast of all 3 reconstruction groups met the diagnostic requirements. The 100% ASIR-V and DLIR-H images had no statistically significant difference (P>0.05), while both were statistically better than the 50% ASIR-V images (P<0.001) (Figure 1D,E,F). For blood vessel margins, DLIR-H outperformed the ASIR-V images (P<0.05), and those of the 100% ASIR-V images were judged to be too smooth to meet the diagnostic requirements (Figure 1G,H,I). The quantitative measurement results showed that the CT values of the 3 reconstruction groups were not significantly different and that image noise, SNR, and CNR of DLIR-H images were similar to those of 100% ASIR-V images, which were both significantly better than the 50% ASIR-V images.
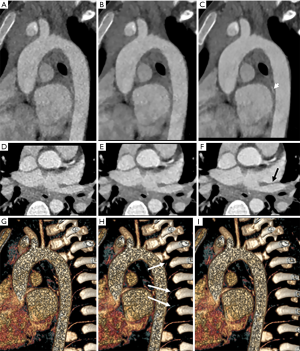
Discussion
In our study, we investigated the feasibility of improving image quality in pediatric chest CTA acquired with low radiation dose and contrast volume by using the newly developed DLIR algorithm compared with the state-of-the-art ASIR-V algorithm. The low radiation dose condition was realized by using a combination of 70 kVp and ATCM with relatively low mAs ranges, which generates increased image noise when conventional FBP reconstruction is used. Our results demonstrated the ability of DLIR to further reduce image noise under the low radiation dose condition without negatively impacting the display of small vessels and edges.
Specifically, our results indicated that DLIR-H further reduced image noise by 35% compared to the 50% ASIR-V (19.24±5.77 vs. 29.45±7.59 HU). We also reconstructed images using ASIR-V with 100% weight (100% ASIR-V) to demonstrate ASIR-V’s noise reduction ability with higher weights. Our results indicated that 100% ASIR-V also further reduced image noise by 30% compared with 50% ASIR-V (20.45±6.93 vs. 29.45±7.59 HU). However, we noticed blurred blood vessel walls (Figure 1E) or some spatial resolution loss for the small blood vessels (Figure 1H) with the 100%ASIR-V algorithm. On the other hand, the DLIR-H algorithm significantly reduced image noise to similar levels as the 100% ASIR-V images, and at the same time, maintaining good spatial resolution for displaying small vessels and vessel walls (Figure 1C,F,I). By optimizing both image noise and spatial resolution, DLIR-H was the only algorithm that provided acceptable scores on all 3 aspects of the qualitative image quality evaluation in our study. Our results were consistent with the findings of Greffier et al. (20). Their phantom study noted that both the DLIR with a high setting and 100% ASIR-V significantly reduced the NPS peak value compared to 50% ASIR-V. However, the task-based transfer function (TTF) of 100% ASIR-V was degraded, and the values were significantly lower than those of DLIR and 50% ASIR-V at every dose level.
Our study results indicated that even at a low radiation dose level, we had enough vessel contrast and relatively clear vessel margins for diagnosing blood vessels using 50%ASIR-V images. However, under the low radiation dose condition, image noise for the soft tissue on the thin slice images was obvious, and the image quality scoring in terms of image noise was sub-optimal for diagnosis. In our routine clinical practice, higher than desired image noise is handled by using images at thicker slice thickness (5 mm) for reviewing soft tissues and organs, which of course requires additional reconstruction in the workflow and sacrifices spatial resolution in the axial direction. However, with DLIR-H, high image noise was significantly reduced to fully satisfy the diagnostic requirement for reviewing soft tissues and organs using thin-slice images, significantly improving clinical workflow and spatial resolution. Furthermore, vessel contrast and vessel clarity were also improved with the DLIR-H algorithm. The improved image contrast and image noise may provide room for future radiation dose and/or contrast volume reduction should we decide to maintain the current image quality.
There were still some shortcomings in our study. First, the number of cases was relatively small. Hence, studies with a larger number of cases should be carried out in the future. We did not subcategorize contrast enhancement based on patient age to study the possible age-dependent variations in contrast enhancement due to the small number of patients. Also, this study was focused on whether DLIR could improve image quality; therefore, only a small part of the stored data was used, and no comprehensive disease types were included. Secondly, this study was a retrospective study to assess the potential of DLIR in image quality improvement at the current low dose scan conditions. Even though image noise reduction can often be indirectly converted to the dose reduction ability, further research is needed using the actual scans acquired at lower radiation doses to evaluate the dose reduction potential directly. Third, due to the limited patient population in our study, we did not evaluate the reconstruction algorithms' dependence on tube voltage and radiation exposure level. Future studies must obtain a complete picture for these reconstruction algorithms and the optimal combination of different tube voltages and DLIR with different strengths. Finally, because the original scan was designed for CTA, no observation of the lungs could be made to evaluate further whether DLIR improved the lungs’ diagnosis.
Conclusions
The use of DLIR-H can significantly improve image quality and further, reduce image noise by 35% compared to 50% ASIR-V in thin slice pediatric chest CTA acquired at low radiation and contrast medium doses. The improved image quality can then be converted into further radiation and/or contrast volume reduction.
Acknowledgments
Funding: None.
Footnote
Conflicts of Interest: All authors have completed the ICMJE uniform disclosure form (available at http://dx.doi.org/10.21037/qims-20-1158). JL is an employee of GE Healthcare, the manufacturer of the CT system used in this study. The other authors have no conflicts of interest to declare.
Ethical Statement: This was a retrospective study approved by our hospital's ethics committee (Ethics reference number: 2020-Z-161), and informed consent from patients was waived.
Open Access Statement: This is an Open Access article distributed in accordance with the Creative Commons Attribution-NonCommercial-NoDerivs 4.0 International License (CC BY-NC-ND 4.0), which permits the non-commercial replication and distribution of the article with the strict proviso that no changes or edits are made and the original work is properly cited (including links to both the formal publication through the relevant DOI and the license). See: https://creativecommons.org/licenses/by-nc-nd/4.0/.
References
- Tola H, Ozturk E, Yildiz O, Erek E, Haydin S, Turkvatan A, Ergul Y, Guzeltas A, Bakir I. Assessment of Children with Vascular Ring. Pediatr Int 2017;59:134-40. [Crossref] [PubMed]
- Backer CL, Mongé MC, Popescu AR, Eltayeb OM, Rastatter JC, Rigsby CK. Vascular Rings. Semin Pediatr Surg 2016;25:165-75. [Crossref] [PubMed]
- Direskeneli H, Aydin SZ, Merkel PA. Assessment of Disease Activity and Progression in Takayasu's Arteritis. Clin Exp Rheumatol 2011;29:S86-91. [PubMed]
- Hermelijn SM, Elders BB, Ciet P, Wijnen RM, Tiddens HA, Schnater JM. A Clinical Guideline for Structured Assessment of CT-imaging in Congenital Lung Abnormalities. Paediatr Respir Rev 2021;37:80-8. [Crossref] [PubMed]
- Kim HJ, Shin KE, Park JS, Lee H, Lee JW, Chin S, Shin HK. Intralobar Pulmonary Sequestration with Cystic Degeneration Mimicking a Bronchogenic Cyst in an Elderly Patient: A Case Report and Literature Review. Medicine (Baltimore) 2020;99:e19347 [Crossref] [PubMed]
- Zhang N, Zeng Q, Chen C, Yu J, Zhang X. Distribution, Diagnosis, and Treatment of Pulmonary Sequestration: Report of 208 Cases. J Pediatr Surg 2019;54:1286-92. [Crossref] [PubMed]
- Tashtoush B, Memarpour R, Gonzalez J, Gleason JB, Hadeh A. Pulmonary Sequestration: A 29 Patient Case Series and Review. J Clin Diagn Res 2015;9:AC05-08. [Crossref] [PubMed]
- Trotman-Dickenson B. Congenital lung disease in the adult: guide to the evaluation and management. J Thorac Imaging 2015;30:46-59. [Crossref] [PubMed]
- Walker CM, Rosado-de-Christenson ML, Martínez-Jiménez S, Kunin JR, Wible BC. Bronchial arteries: anatomy, function, hypertrophy, and anomalies. Radiographics 2015;35:32-49. [Crossref] [PubMed]
- . The ALARA (as low as reasonably achievable) concept in pediatric CT intelligent dose reduction. Multidisciplinary conference organized by the Society of Pediatric Radiology. August 18-19, 2001. Pediatr Radiol 2002;32:217-313. [PubMed]
- Ren Z, Zhang X, Hu Z, Li D, Liu Z, Wei D, Jia Y, Yu N, Yu Y, Lei Y, Chen X, Guo C, Ren Z, He T. Application of Adaptive Statistical Iterative Reconstruction-V with Combination of 80 kV for Reducing Radiation Dose and Improving Image Quality in Renal Computed Tomography Angiography for Slim Patients. Acad Radiol 2019;26:e324-32. [Crossref] [PubMed]
- Meyer M, Haubenreisser H, Schoepf UJ, Vliegenthart R, Leidecker C, Allmendinger T, Lehmann R, Sudarski S, Borggrefe M, Schoenberg SO, Henzler T. Closing in on the K Edge: Coronary CT Angiography at 100, 80, and 70 kV-initial Comparison of a Second- Versus a Third-Generation Dual-Source CT System. Radiology 2014;273:373-82. [Crossref] [PubMed]
- MacDougall RD, Kleinman PL, Yu L, Lee EY, et al. Pediatric thoracic CT angiography at 70 kV: a phantom study to investigate the effects on image quality and radiation dose. Pediatr Radiol 2016;46:1114-9. [Crossref] [PubMed]
- Shimoyama S, Nishii T, Watanabe Y, Kono AK, Kagawa K, Takahashi S, Sugimura K. Advantages of 70-kV CT Angiography for the Visualization of the Adamkiewicz Artery: Comparison with 120-kV Imaging. AJNR Am J Neuroradiol 2017;38:2399-405. [Crossref] [PubMed]
- Zhu Z, Zhao Y, Zhao X, Wang X, Yu W, Hu M, Zhang X, Zhou C. Impact of preset and postset adaptive statistical iterative reconstruction-V on image quality in nonenhanced abdominal-pelvic CT on wide-detector revolution CT. Quant Imaging Med Surg 2021;11:264-75. [Crossref] [PubMed]
- Goenka AH, Herts BR, Dong F, Obuchowski NA, Primak AN, Karim W, Baker ME. Image Noise, CNR, and Detectability of Low-Contrast, Low-Attenuation Liver Lesions in a Phantom: Effects of Radiation Exposure, Phantom Size, Integrated Circuit Detector, and Iterative Reconstruction. Radiology 2016;280:475-82. [Crossref] [PubMed]
- Yan C, Xu J, Liang C, Wei Q, Wu Y, Xiong W, Zheng H, Xu X. Radiation Dose Reduction by Using CT with Iterative Model Reconstruction in Patients with Pulmonary Invasive Fungal Infection. Radiology 2018;288:285-92. [Crossref] [PubMed]
- Verdun FR, Racine D, Ott JG, Tapiovaara MJ, Toroi P, Bochud FO, Veldkamp WJ, Schegerer A, Bouwman RW, Giron IH, Marshall NW, Edyvean S. Image quality in CT: from physical measurements to model observers. Phys Med 2015;31:823-43. [Crossref] [PubMed]
- Geyer LL, Schoepf UJ, Meinel FG, Nance JW Jr, Bastarrika G, Leipsic JA, Paul NS, Rengo M, Laghi A, De Cecco CN. State of the art: iterative CT reconstruction techniques. Radiology 2015;276:339-57. [Crossref] [PubMed]
- Greffier J, Hamard A, Pereira F, Barrau C, Pasquier H, Paul Beregi J, Frandon J. Image quality and dose reduction opportunity of deep learning image reconstruction algorithm for CT: a phantom study. Eur Radiol 2020;30:3951-9. [Crossref] [PubMed]
- Benz DC, Benetos G, Rampidis G, von Felten E, Bakula A, Sustar A, Kudura K, Messerli M, Fuchs TA, Gebhard C, Pazhenkottil AP, Kaufmann PA, Buechel RB. Validation of Deep-Learning Image Reconstruction for Coronary Computed Tomography Angiography: Impact on Noise, Image Quality and Diagnostic Accuracy. J Cardiovasc Comput Tomogr 2020;14:444-51. [Crossref] [PubMed]
- Park C, Choo KS, Jung Y, Jeong HS, Hwang J, Yun MS. CT iterative vs deep learning reconstruction: comparison of noise and sharpness. Eur Radiol 2021;31:3156-64. [Crossref] [PubMed]
- Bernard A, Comby P, Lemogne B, Haioun K, Ricolfi F, Chevallier O, Loffroy R. Deep learning reconstruction versus iterative reconstruction for cardiac CT angiography in a stroke imaging protocol: reduced radiation dose and improved image quality. Quant Imaging Med Surg 2021;11:392-401. [Crossref] [PubMed]