Aberrant cerebral perfusion pattern in amnestic mild cognitive impairment and Parkinson’s disease with mild cognitive impairment: a comparative arterial spin labeling study
Introduction
Mild cognitive impairment (MCI), the transitional phase between aging process and dementia, has been recognized as the potential forerunner of Alzheimer’s disease (AD) and Parkinson’s disease with dementia (PDD), and its progression is variable (1-3). Approximately 76% of MCI patients who progress to AD have amnestic MCI (aMCI), which is featured with selective memory impairment (4). Although cognitive dysfunctions in PD are heterogeneous, up to 80% of PD-MCI patients subsequently develop PDD in the advanced stages of the disease (5,6). Despite having distinct pathological hallmarks, PDD and AD share some pronounced features, including genetic commonalities (7), extrapyramidal signs (8), epigenetic alterations (9), cholinergic deficits (10), amyloid-β (Aβ) deposition (11), and the same typical pattern of cortical atrophy (12) or functional alteration (13). However, there are clear differences between AD and PDD, which may be related to the earlier stage before these overlapping neurodegenerative changes begin (14). Given these underlying pathologies, neuroimaging is essential for the early detection of structural or functional alterations in vivo to refine disease diagnosis and clarify the underlying neural mechanisms.
When Kunst et al. (15) compared cortical atrophy in groups with aMCI and PD-MCI, they found no specific differences, probably due to the shared pattern of cortical atrophy. As functional changes precede structural impairments, it is desirable to monitor aberrant neural activity in the MCI stage. Cerebral blood flow (CBF), which is coupled with neuronal activity or metabolism, has been shown to be a biomarker of brain function (16). Evidence of extensive hypoperfusion in the MCI stage with different pathologies was revealed by several radiotracer (positron emission tomography, PET; single-photon emission computed tomography, SPECT) literatures (17-19). As an alternative, arterial spin labeling (ASL), a noninvasive MRI technique for quantitative cerebral perfusion measurement, is a promising proxy that requires no radiotracer and has yielded convincing results in AD and PD (20,21). In addition to providing an absolute quantitative measurement of CBF, ASL can also directly reflect neural connectivity with lower inter-subject variability than PET or SPECT (22). Havsteen et al. (23) has demonstrated the network’s function that is achieved by the synchronously altered CBF-connectivity from the same functional brain regions.
ASL studies of perfusion changes have demonstrated that progressive hypoperfusion is associated with disease severity and progression in both AD and PD (20,24,25). Le Heron et al. (14) compared cerebral perfusion abnormalities in AD and PDD, reporting that the pattern of hypoperfusion was very similar between these dementia syndromes. However, the earlier difference in perfusion patterns in the prodrome of these two conditions remains unclear. Additionally, Ma et al. (26) identified an analogous PD-related pattern (PDRP) with normalized CBF derived from ASL, claiming that normalized CBF would be the more sensitive measure with which to visualize perfusion changes. Therefore, in this study, we conducted a comparative analysis of regional normalized CBF in aMCI and PD-MCI by using ASL. Furthermore, we focused on the differences in CBF-connectivity between MCI groups under different pathologies. We hypothesized that there exist different patterns of regional perfusion that are detectable by ASL, and CBF-associated changes could serve as noninvasive diagnostic biomarkers in discriminating MCI subtypes and enhancing the understanding of related neurovascular mechanisms.
Methods
Participants
A total of 94 non-demented patients (all right-handed Chinese individuals) were recruited from January 2018 to February 2020 via healthy examination for community, newspaper advertisements, or cognitive clinic. Among all participants, 5 patients (2 aMCI, 3 PD-MCI) were excluded due to insufficient image quality (severe susceptibility artifacts or uncomplete coverage), 3 subjects (1 aMCI, 2 PD-MCI) were also excluded from further analyses because the motion parameters (provided in data preprocessing) exceeded the 3 mm and 3° criteria (27). The final sample consisted of 44 (26 male and 18 female) patients with aMCI and 42 (18 male and 24 female) patients with PD-MCI. We also enrolled 50 (25 male and 25 female) healthy controls (HCs) matched with both MCI groups for age, sex, and years of education. Ethics permission was granted by the Institutional Review Board of Clinical Medical College, Yangzhou University (2018KY-074), and written consent was obtained from each participant after a detailed explanation of the procedures 24 hours prior to the examinations.
Patients with aMCI were diagnosed based on criteria modified from those proposed by Petersen (28): (I) subjective memory deficit admitted by the subject and an informant; (II) objectively impaired memory function documented by delayed recall in Hopkins Verbal Learning Test-Revised (HVLT-R); (III) preservation of independence in activities of daily living; (IV) Clinical Dementia Rating (CDR) is scored 0.5, and (V) absence of dementia. Clinical diagnoses of PD-MCI met the following criteria: (I) the United Kingdom Parkinson’s Disease Society Brain Bank Clinical Diagnostic Criteria (29) and Movement Disorders Society Task Force criteria (30); (II) disease stage ≤ Hoehn & Yahr (H&Y) stage II; (III) cognitive impairment with a recommended Montreal Cognitive Assessment (MoCA) score <26; (IV) cognitive dysfunction (at least two test scores >1.5 standard deviations below the standardized mean score); (V) preservation of independence in activities of daily living; (VI) CDR of 0.5, and (VII) absence of dementia. Before the study entry, the MCI participants were required to have a controlled and optimized routine dosage of drugs (psychotropic or antiparkinsonian) ≥4 weeks, and in the OFF medication state for at least 12 hours.
Participants were excluded as follows: (I) family history of PD, secondary parkinsonism, or parkinsonism syndrome; (II) additional intracranial lesions, including cerebrovascular diseases, head trauma with fracture or hemorrhage, intracranial mass lesions, history of intracranial surgery, or any disease involved the nervous system, such as diabetes, alcoholism; (III) neurological or psychiatric diseases, including epilepsy, depression, schizophrenia, dysthymic disorder; (IV) other therapies on cognitive function, including additional psychotropic or anticholinergic medications; (V) any MRI contraindications, such as electronic or metal implants, and claustrophobia; (VI) uncooperative state, including severe visual or hearing impairment; and (VII) severe head motion parameters. Additionally, HC participants were excluded with scores of MoCA and Mini-Mental State Examination (MMSE) <26. Two neurological experts (Hengzhong Zhang, with 35 years working seniority, and Yao Xu, with 31 years working seniority) conducted the criteria and evaluation by executing a structured inquiry to participants and their caregivers.
Clinical performance evaluation
For PD-MCI patients, Movement Disorder Society-Unified Parkinson’s Disease Rating Scale-III (MDS-UPDRS-III) was taken for the disease severity assessment, and the disease stage were evaluated by H&Y scale. Referring to Tomlinson et al. (31), calculation of the levodopa equivalent daily dose (LEDD) in PD-MCI individuals was performed. The CDR was scored for exclusion of dementia. The global cognitive function of all subjects was scored using the MMSE and MoCA. In addition, the HVLT-R, Digit Symbol Substitution Test (DSST), Trail Making Test (TMT)-A and TMT-B, Verbal Fluency Test (VFT), and Clock Drawing Test (CDT) were executed in a fixed order to assess detailed cognitive domains (memory, attention, executive function, language, and visuospatial function) as previously introduced (32).
Data acquisition
The enrolled subjects received MRI scanning with a 3.0-tesla (Discovery MR750, GE Medical Systems, Milwaukee, WI, USA) scanner equipped with a commercial 8-channel phased array head coil. Before scanning, subjects were instructed to fast for at least 4 hours and abstained from any vasoactive drugs at least 12 hours. During the acquisition, all the subjects lie in a supine position and wore headphones, they were asked to keep closing eyes and awake without thinking about things. Three-dimensional (3D) pseudo-continuous ASL (pCASL) sequence with a background suppression was as follows: repetition time (TR) 10.5 ms, echo time (TE) 4.9 ms, flip angle 111°, slice thickness 4 mm without gap, field of view (FOV) 240 mm × 240 mm, matrix size 128×128, labeling duration 1,500 ms, postlabeling delay (PLD) 2,025 ms, number of excitations 3, number of slices 36, total scan time 4 min and 44 s, units: mL/100 g/min. This sequence also included a fluid-suppressed proton density acquisition with the same image dimensions as the pCASL but without radio frequency labeling for CBF quantitation and image registration. Structural data (high-resolution T1-weighted images) were obtained using a 3D whole-brain brain volume imaging sequence. The sequence parameters were as follows: TR 12 ms, TE 5.1 ms, inversion time 450 ms, flip angle 15°, slice thickness 1 mm, no gaps, FOV 240 mm × 240 mm, matrix size 256×256, voxel size 1 mm × 1 mm × 1 mm; number of slices 172, total scan time 5 min and 20 s.
MRI data preprocessing and analysis
FuncTool software (version 4.6, GE Medical Systems, Milwaukee, WI, USA) with a general kinetic model for ASL was utilized to generate the CBF images (33). The generated CBF maps were preprocessed by Statistical Parametric Mapping (SPM, version 12, https://www.fil.ion.ucl.ac.uk/spm) based on MATLAB R2016b software (MathWorks Inc., Natick, MA, USA). Okonkwo et al. (34) has introduced ASL data preprocessing detailedly. Briefly, motion and magnetic field B0 inhomogeneities correction for CBF images of individuals were first conducted. We removed participants who translated and rotated higher than 3 mm and 3° (27) respectively, referring to the head movement parameters calculated by SPM; each participant’s CBF images were co-registered to that person’s T1 anatomic maps, and T1 maps were normalized to Montreal Neurological Institute (MNI) space; the CBF maps were then brought to the MNI template by spatial transforms concatenation, with a resampling voxel size of 2×2×2 mm3; the non-brain tissues were removed from each co-registered CBF map. By subtracting the global mean and dividing by the standard deviation (z-scored), the preprocessed CBF maps were normalized as previously introduced (35-37), which is more sensitive for detection of subtle alterations in regional perfusion. Finally, the z-scored CBF (z-CBF) maps were smoothed with an 8×8×8 mm3 full width at half maximum (FWHM) Gaussian kernel. A relative increase in perfusion with respect to other groups was interpreted as preserved perfusion.
Gray matter volume (GMV) calculation
The GMV of each voxel was calculated and used as one of covariates for the statistical analysis, for the correction of atrophy or partial volume effects. SPM12 was used for GMV calculation. Segmentation was performed for the T1 anatomic maps, by dividing white matter, gray matter (GM) and cerebrospinal fluid with the standard uniform segmentation model. The GM concentration maps were initial affine registered to MNI space, nonlinear deformation of GM concentration maps were then processed using the diffeomorphic anatomical registration through exponentiated Lie algebra technique, and were resampled to a voxel size of 1.5×1.5×1.5 mm3. The GMV of each voxel was obtained by multiplying the GM concentration graph by the nonlinear determinant obtained by the spatial normalization step. Then, the GMV maps were smoothed with a 6×6×6 mm3 FWHM Gaussian kernel. Finally, the mean GMV of each individual were extracted.
Disease-related specific region analysis
Referring to previous ASL studies, specific AD-related regions (25,38), including the bilateral posterior cingulate cortex (PCC), precuneus, and hippocampus, along with specific PD-related regions (39,40), including the bilateral caudate, putamen, globus pallidus, and thalamus, were selected. Theses seed regions of interest (ROIs) were extracted from the automated anatomical labeling (AAL) atlas (41). Within each participant, the extraction of z-CBF values for each seed ROI from individual z-CBF maps was performed for further analysis of ROI-based group differences.
CBF-connectivity analyses
We selected the survived clusters (seed ROIs) with group differences in the z-CBF maps. For each participant, extraction of z-CBF values for each seed ROI from individual z-CBF maps was conducted. Referring to previous studies (42,43), for each group, a multiple regression model was used for the calculation of correlation coefficient (CBF-connectivity) between each seed ROI and all other voxels of the whole brain across individuals. Confounding covariates (sex, age, education, and mean GMV) were applied. Familywise error (FWE) method (P<0.05) was used for multiple comparisons correction. For each group, correlation (positive or negative) between voxels and each seed ROI was therefore identified. A spatial mask, where the z-CBF of each voxel was correlated with the z-CBF of the ROI in any of the three groups, was generated by merging the corrected CBF-connectivity maps of each group. For any pair of voxels, the z-CBF correlation among the three groups may have different slopes, reflecting the difference in CBF-connectivity. By using the merged spatial mask, we performed analysis of covariance (ANCOVA) with multiple regression models. The significant differences among groups were analyzed, representing a statistically altered z-CBF correlation with each seed ROI.
Statistical analysis
The normality distribution of clinical and demographic data was tested by Kolmogorov-Smirnov methods. One-way analysis of variance was utilized for the comparisons of age among groups. Education, disease duration, and cognitive scores were the continuous variables that not normally distributed. Kruskal-Wallis test was conducted for the analysis (for education and cognitive scores) among groups. The comparison between the aMCI and PD-MCI groups (for disease duration) was conducted by Mann-Whitney U test. Chi-squared (χ2) test was performed for the comparisons of sex among groups. SPSS software (version 19.0, SPSS Inc., Chicago, IL, United States) was utilized for the above-mentioned statistical analyses. Statistically significant was defined as a P value <0.05.
For the z-CBF and CBF-connectivity analyses, comparisons among groups were performed in a voxel-wise manner using ANCOVA within spatial mask (z-CBF, whole brain grey matter mask; CBF-connectivity, the merged mask as above described). We further analyzed the difference between each group within the significant regions by Post hoc. SPM 12 software was utilized for these statistical analyses. Multiple comparisons correction for ANCOVA and post hoc t-tests was performed using the nonstationary cluster-level FWE method, with a cluster-defining threshold of P=0.001 and a corrected cluster significance of P<0.05 by the FWE. We also utilized the Permutation testing for more strict correction. Spearman correlation analysis was used to analysis the correlations between z-CBF value of significant regions and scores of neuropsychological performance and disease severity (UPDRS-III) separately. SPSS 19.0 software was utilized for the correlation analysis, and statistically significant was defined as a P value <0.05. ROI-based group differences analysis was performed using ANCOVA followed by post hoc Bonferroni tests. Furthermore, receiver operating characteristic (ROC) analysis was generated for the aMCI group and PD-MCI group to determine the ability of the z-CBF in the regions with significant group differences. For the above analyses, sex, age, education, and individual mean GMV were taken as covariates.
Results
Demographic and clinical characteristics
Participant demographic and clinical characteristics are shown in Table 1. We did not found statistical differences in age, sex, or years of education among the three groups (P>0.05). Both aMCI and PD-MCI patients suffered from poor performance of general cognition and all five cognitive domains in comparison with the HC group (P<0.05). Patients with aMCI demonstrated more severe impairment of episodic memory (HVLT-R delayed, P=0.028; HVLT-R recognition P=0.042) and less severe deficits in executive function (TMT-B, P=0.031) than PD-MCI subjects. Although the MMSE scores were not significantly different between the MCI groups (P>0.05), the MoCA scale of the PD-MCI patients was scored significantly lower than that of the aMCI patients (P=0.001).
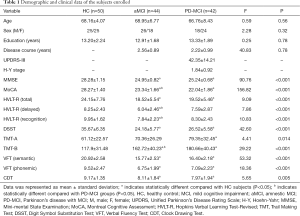
Full table
Group-level differences in normalized CBF
Although there was no cluster survived when applying Permutation testing, we found significant differences with FWE correction. In the voxel-based analysis, the survived clusters in z-CBF maps among the three groups are demonstrated in Figure 1 and Table 2. The survived clusters among the HC, aMCI, and PD-MCI groups, which were analyzed by one-way ANCOVA analysis, were in the left postcentral gyrus, right putamen, bilateral middle frontal gyrus (MFG), right angular gyrus, left superior medial frontal gyrus, and right supplementary motor area (SMA). As compared with the HC subjects, aMCI group showed increased z-CBF in the left postcentral gyrus, bilateral putamen, right anterior cingulate gyrus (ACG), right SMA, and left middle cingulate gyrus (MCG), while higher z-CBF in the left postcentral gyrus, bilateral putamen, and right ACG was found in PD-MCI patients. Relative to the HC subjects, decreased z-CBF in the right MFG, left superior frontal gyrus (SFG), left superior medial frontal gyrus (SMFG), and right angular gyrus was observed in the aMCI group; additionally, z-CBF was decreased in the right SFG, left SMFG, right angular gyrus, and right MFG of the PD-MCI group. Moreover, post hoc analysis also demonstrated that, compared to the aMCI group, PD-MCI patients had relatively lower z-CBF in the bilateral putamen, left precentral gyrus, left MCG, and right MFG.
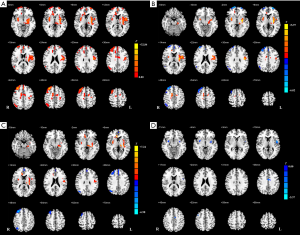
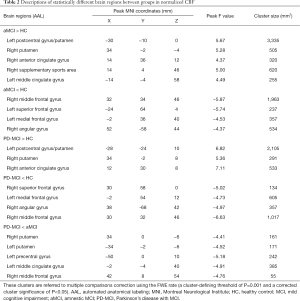
Full table
Group-level differences of ROI-based analyses
The results of ROI analyses are shown in Figure 2. As expected, all of the specific ROIs reached significant differences after ANOVA contrast analysis (P<0.05). Except for the precuneus (found only in the aMCI group), the z-CBFs of the remaining ROIs were significantly different in both MCI patients relative to the HC group (P<0.05). We found that only putamen z-CBF values were significantly different between any two groups (P<0.05).
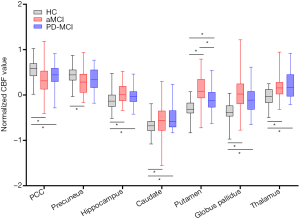
Correlations between z-CBF alterations and clinical performance
The significant correlations between the z-CBF alterations and disease severity (UPDRS-III) or neuropsychological evaluation are demonstrated graphically in Figure 3. In the PD-MCI patients, we explored that z-CBF values in the right MFG were negatively correlated with executive function scored by TMT-B significantly (ρ=−0.33, P=0.031). Additionally, the increased z-CBF values in the left precentral gyrus and bilateral putamen had significant positive correlations with motor impairment as assessed by UPDRS-III scores (ρ=0.43, P=0.004; ρ=0.46, P=0.002; ρ=0.32, P=0.034). In the aMCI group, we found that z-CBF values in the left precentral gyrus were negatively correlated with memory performance as scored by HVLT-R (total) significantly (ρ=−0.31, P=0.043). We also discovered that the increased z-CBF values in the right MFG were had significant positive correlations with memory dysfunction as assessed by HVLT-R (recognition) scores (ρ=0.34, P=0.025). There were no significant correlations between the other significant regions and clinical characteristics.
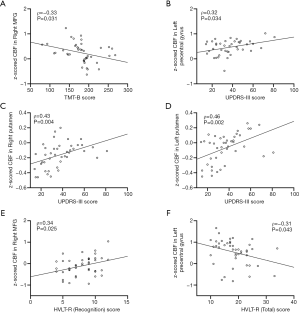
Group-level differences in CBF-connectivity
After FWE correction, we found significant differences among groups. The CBF-connectivity maps of significant clusters among the three groups are demonstrated in Figure 4 and Table 3. Among groups, the significant clusters of CBF-connectivity were as follows: between the left precentral gyrus seed and right postcentral gyrus and right postcentral gyrus; between the left MCG seed and right inferior temporal gyrus and right cuneus; and between the right MFG seed and left precentral gyrus and right angular gyrus.
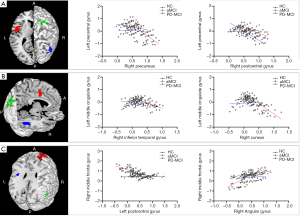
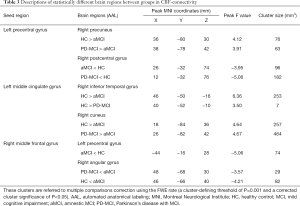
Full table
Compared with both HC and PD-MCI subjects, the aMCI group showed significantly decreased CBF-connectivity between the left precentral gyrus and right precuneus, left MCG and right cuneus and significantly increased CBF-connectivity between the right MFG and right angular gyrus. For the HC group, significantly decreased CBF-connectivity between the left MCG and right inferior temporal gyrus (ITG) and between the left precentral gyrus and right postcentral gyrus were found relative to either aMCI or PD-MCI. Additionally, the aMCI group also demonstrated significantly decreased CBF-connectivity between the right MFG and right precentral gyrus in comparison with the HC group.
ROC analysis for differential diagnosis
All of the significant clusters that differed between the aMCI and PD-MCI groups had good discriminatory power with an area under the curve (AUC) of >0.7. The AUC, sensitivity and specificity of each cluster are summarized in Figure 5. In particular, the right putamen showed the most powerful discriminatory ability: the sensitivity was 83.33%, and the specificity was 72.73% at a cutoff value of mean z-CBF coefficients of −0.04.
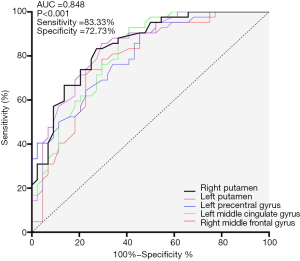
Discussion
In the present study, we focused on the distinct CBF properties of patients with aMCI and PD-MCI using the ASL approach and obtained several major findings. In comparison with HC subjects, the aberrant perfusion in aMCI patients was more extensive than that of PD-MCI patients. Despite the overlapping impaired regions, the pattern of perfusion in the PD-MCI subjects was distinct from that in the aMCI patients, along with characterized pathologic changes itself. The normalized CBF value of significantly different clusters performed well in discriminating diagnosis among the three groups.
Metabolic changes in brain neurons are dynamic and sensitive in the prodromal phase of neurodegenerative diseases. ASL can directly quantify absolute CBF values and has been used to predict altered perfusion during the progression of neurodegenerative diseases. Compared with matched normal controls, a hypoperfusion pattern has been reported in AD, PD, MCI patients, and even elderly subjects (20,21). However, some regional compensatory processes or hyperactivation might be masked by this global reduction. By employing normalized CBF, our study revealed some preserved perfusion in MCI groups, especially in the aMCI subtype, since this relative perfusion was reported with few studies. In comparison with HC subjects, both MCI groups demonstrated preserved perfusion mainly in basal ganglia subregions, limbic system, and sensorimotor cortex, along with decreased perfusion in the associated frontal, parietal and parietotemporal cortex. Such patterns are in line with previous studies using radiotracers (44,45).
In an ASL-related comparative study (14), no significant distinct pattern of abnormal perfusion was observed in either AD or PDD. The present study also demonstrated that the impaired pattern of regional hypoperfusion in PD-MCI overlapped with that of aMCI. The decreased z-CBF in the parietal and parietotemporal cortex was in accordance with the hypothesis (46) that cognitive impairment in PD was mostly attributed to synergistic combination of cholinergic deficit in posterior cortex and AD-related pathologic proteins. However, after post hoc analysis, we observed more severe hypoperfusion in the prefrontal cortex of the PD-MCI group, which was negatively related to executive performance, and we also detected a negative relation between z-CBF values of the prefrontal cortex and memory function in aMCI patients. Moreover, relative to the HC and PD-MCI groups, the aMCI group showed increased CBF-connectivity between the right MFG and right angular gyrus, which plays crucial roles in visuospatial processing. This higher connectivity likely indicates a compensatory mechanism along with impaired perfusion in MFG, whereas none of this compensation was found in PD-MCI. Previous studies (47,48) support our finding by confirming that frontal executive and temporal visuospatial dysfunction was more evident in patients with PD than in those with AD.
The present study identified preserved perfusion in both categories of MCI subjects. The basal ganglia subregions modulate cortex-related higher-order behavior and cognition along with association fibers in the limbic system (49). Although the patterns of preserved perfusion of each MCI subtype in our study partially overlapped, it is worth emphasizing that these patterns are associated with distinct characterized physiological processes. Chen et al. (50) indicated that regional CBF could not distinguish excitatory from inhibitory input, although CBF is regarded as the direct reflection of synaptic activity due to neurovascular coupling. In addition, we further revealed some surviving regions (bilateral putamen, left precentral gyrus, and left MCG) with relatively decreased perfusion in the PD-MCI group after post hoc analysis with the aMCI group. These impaired regions are mostly associated with motor symptom disturbance due to the striato-thalamo-cortical circuit in PD, as previously reported (51-54). We also detected that z-CBF values in the bilateral putamen and left precentral gyrus were both positively related to motor deficits. Therefore, we deduced that the regions with increased z-CBF in PD-MCI are more likely to be a downstream effect, following dopaminergic impairment, by receiving the increased inhibitory output via the known direct or indirect pathway. Contrary to the impaired mechanism in PD-MCI, the preserved pattern of perfusion in the aMCI state probably reflect excitatory input as a compensatory process in response to cognitive dysfunction with associated hypoperfusion regions, since we discovered a negative relation between z-CBF values of the left precentral gyrus and memory function. In addition, as compared with HC group, the aMCI group showed consistently decreased CBF-connectivity to cortex that involved in memory dysfunction, despite of preserved perfusion in the left MCG and left precentral gyrus, whereas altered CBF-connectivity in PD-MCI was inconsistent. Our findings support that PD-MCI is heterogeneous with complex movement disturbances and cognitive decline (55), differing from aMCI, which features selective memory deficits (4).
Aberrant absolute CBF values in the PCC, hippocampus, and precuneus are defined as specific predictive biomarkers for cognitive decline in aMCI compared with matched HCs (25). However, these specific regions failed to discriminate aMCI from PD-MCI in the present study, resulting from the shared regional metabolic reductions. In a radiotracer study, Mattis et al. (45) discovered that PD patients showed only slight ADRP expression due to topographic overlap with the network underlying PDRP. By using normalized CBF derived from ASL, Ma et al. (26) validated an analogous PDRP in PD patients. Thus, we further applied the normalized CBF to aMCI patients in a comparative study with PD-MCI. Relative to the negative founding reported by Le Heron et al. (14) when compared AD with PDD by using absolute CBF, our results intriguingly indicate that the normalized CBF (z-CBF) values based on significant clusters within group differences are capable of distinguishing aMCI from PD-MCI with optimal sensitivity and specificity. Normalized CBF (z-CBF) values based on significant clusters within group differences are capable of distinguishing aMCI from PD-MCI with optimal sensitivity and specificity, even though the perfusion alterations were slight in the MCI stage. Previous functional study (27) reported that that functional connectivity derived from BOLD signals could discriminate AD from PDD with the sensitivity of 73.7% and the specificity of 75.4%. z-CBF in the putamen could discriminate aMCI from PD-MCI, the potential forerunner of AD and PDD, with higher sensitivity (83.33%) and similar specificity (72.73%). Therefore, z-CBF in the putamen, which is regarded as a crucial hub involved in motor deficits in PD, could potentially serve as a better perfusion biomarker for differential diagnosis among groups.
There are several limitations that need to be noted. First, the relative small sample size may cause a statistical bias, and larger numbers of patients are needed in further investigations. Second, PD with normal cognition and other MCI subtypes (non-amnestic, vascular, etc.) should be taken into account in future studies, although we focused on the preclinical stage of AD and PDD. Additionally, the ROC analysis in our study was used to determine the ability of the z-CBF in the regions with significant group differences, whereas it would be more valid if this analysis was obtained from a separate sample. Third, the MCI patients in our study were clinically diagnosed, additional biomarkers (such as choline or Aβ deposition reflected by cerebrospinal fluid or PET) would be better for the understanding of the underlying pathologies. Fourth, we only preliminarily used one scale for the evaluation of each cognitive domain. Combined assessments for the detailed cognitive profiles are warranted, and will be more comprehensive for exploring the underlying mechanisms in cognitive impairment. Fifth, we could not investigate the correlation between CBF-connectivity and clinical performance, due to the interregional CBF correlation analysis at the group level. The advanced processed method and developed ASL sequence in the future could settle this restriction. Finally, a multi-PLD ASL approach is necessary in future studies to minimize the transit time differences in individuals, although we used a recommended age-adjusted PLD of 2,025 ms (56).
Conclusions
In conclusion, we observed impaired perfusion patterns in both MCI groups in comparison with HC subjects. Although the aberrant perfusion pattern in the PD-MCI group mostly overlapped with that of the aMCI group, the preserved perfusion regions revealed by normalized CBF are probably linked to distinct pathological mechanisms. The hypoperfusion in the prefrontal cortex might be a specific feature of interest for further studies on cognitive decline. By combining z-CBF values from several significant regions, ASL is capable of differentiating aMCI, PD-MCI, and healthy subjects. Further investigations are required to explore the validation of ASL in monitoring disease progression underlying different pathologies.
Acknowledgments
Funding: This work was supported by the National Natural Science Foundation of China (NSFC81571652); Natural Science Foundation of Jiangsu Province (No. BK20201118); “333 Project” of Jiangsu Province (BRA2017154); Science and technology project of Yangzhou (YZ2018059).
Footnote
Conflicts of Interest: All authors have completed the ICMJE uniform disclosure form (available at http://dx.doi.org/10.21037/qims-20-1259). The authors have no conflicts of interest to declare.
Ethical Statement: Ethics permission from the Institutional Review Board of Clinical Medical College, Yangzhou University (2018KY-074), and written consent was obtained from each participant.
Open Access Statement: This is an Open Access article distributed in accordance with the Creative Commons Attribution-NonCommercial-NoDerivs 4.0 International License (CC BY-NC-ND 4.0), which permits the non-commercial replication and distribution of the article with the strict proviso that no changes or edits are made and the original work is properly cited (including links to both the formal publication through the relevant DOI and the license). See: https://creativecommons.org/licenses/by-nc-nd/4.0/.
References
- Petersen RC, Negash S. Mild cognitive impairment: an overview. CNS Spectr 2008;13:45-53. [Crossref] [PubMed]
- Anang JB, Gagnon JF, Bertrand JA, Romenets SR, Latreille V, Panisset M, Montplaisir J, Postuma RB. Predictors of dementia in Parkinson disease: a prospective cohort study. Neurology 2014;83:1253-60. [Crossref] [PubMed]
- Guo S, Xiao B, Wu C. Identifying subtypes of mild cognitive impairment from healthy aging based on multiple cortical features combined with volumetric measurements of the hippocampal subfields. Quant Imaging Med Surg 2020;10:1477-89. [Crossref] [PubMed]
- Yaffe K, Petersen RC, Lindquist K, Kramer J, Miller B. Subtype of mild cognitive impairment and progression to dementia and death. Dement Geriatr Cogn Disord 2006;22:312-9. [Crossref] [PubMed]
- Svenningsson P, Westman E, Ballard C, Aarsland D. Cognitive impairment in patients with Parkinson's disease: diagnosis, biomarkers, and treatment. Lancet Neurol 2012;11:697-707. [Crossref] [PubMed]
- Xu R, Hu X, Jiang X, Zhang Y, Wang J, Zeng X. Longitudinal volume changes of hippocampal subfields and cognitive decline in Parkinson's disease. Quant Imaging Med Surg 2020;10:220-32. [Crossref] [PubMed]
- Grünblatt E. Commonalities in the genetics of Alzheimer's disease and Parkinson's disease. Expert Rev Neurother 2008;8:1865-77. [Crossref] [PubMed]
- Ditter SM, Mirra SS. Neuropathologic and clinical features of Parkinson's disease in Alzheimer's disease patients. Neurology 1987;37:754-60. [Crossref] [PubMed]
- Marques SC, Oliveira CR, Pereira CM, Outeiro TF. Epigenetics in neurodegeneration: a new layer of complexity. Prog Neuropsychopharmacol Biol Psychiatry 2011;35:348-55. [Crossref] [PubMed]
- Olde Dubbelink KT, Schoonheim MM, Deijen JB, Twisk JW, Barkhof F, Berendse HW. Functional connectivity and cognitive decline over 3 years in Parkinson disease. Neurology 2014;83:2046-53. [Crossref] [PubMed]
- Hepp DH, Vergoossen DL, Huisman E, Lemstra AW, Berendse HW, Rozemuller AJ, Foncke EM, van de Berg WD. Distribution and Load of Amyloid-beta Pathology in Parkinson Disease and Dementia with Lewy Bodies. J Neuropathol Exp Neurol 2016;75:936-45. [Crossref] [PubMed]
- Rektorova I, Biundo R, Marecek R, Weis L, Aarsland D, Antonini A. Grey matter changes in cognitively impaired Parkinson's disease patients. PLoS One 2014;9:e85595 [Crossref] [PubMed]
- Rane S, Donahue MJ, Claassen DO. Amnestic mild cognitive impairment individuals with dissimilar pathologic origins show common regional vulnerability in the default mode network. Alzheimers Dement (Amst) 2018;10:717-25. [Crossref] [PubMed]
- Le Heron CJ, Wright SL, Melzer TR, Myall DJ, MacAskill MR, Livingston L, Keenan RJ, Watts R, Dalrymple-Alford JC, Anderson TJ. Comparing cerebral perfusion in Alzheimer's disease and Parkinson's disease dementia: an ASL-MRI study. J Cereb Blood Flow Metab 2014;34:964-70. [Crossref] [PubMed]
- Kunst J, Marecek R, Klobusiakova P, Balazova Z, Anderkova L, Nemcova-Elfmarkova N, Rektorova I. Patterns of Grey Matter Atrophy at Different Stages of Parkinson's and Alzheimer's Diseases and Relation to Cognition. Brain Topogr 2019;32:142-60. [Crossref] [PubMed]
- Zhang N, Gordon ML, Goldberg TE. Cerebral blood flow measured by arterial spin labeling MRI at resting state in normal aging and Alzheimer's disease. Neurosci Biobehav Rev 2017;72:168-75. [Crossref] [PubMed]
- Drzezga A. The Network Degeneration Hypothesis: Spread of Neurodegenerative Patterns Along Neuronal Brain Networks. J Nucl Med 2018;59:1645-8. [Crossref] [PubMed]
- Albrecht F, Ballarini T, Neumann J, Schroeter ML. FDG-PET hypometabolism is more sensitive than MRI atrophy in Parkinson's disease: A whole-brain multimodal imaging meta-analysis. Neuroimage Clin 2019;21:101594 [Crossref] [PubMed]
- Riederer I, Bohn KP, Preibisch C, Wiedemann E, Zimmer C, Alexopoulos P, Forster S. Alzheimer Disease and Mild Cognitive Impairment: Integrated Pulsed Arterial Spin-Labeling MRI and (18)F-FDG PET. Radiology 2018;288:198-206. [Crossref] [PubMed]
- Barzgari A, Sojkova J, Maritza Dowling N, Pozorski V, Okonkwo OC, Starks EJ, Oh J, Thiesen F, Wey A, Nicholas CR, Johnson S, Gallagher CL. Arterial spin labeling reveals relationships between resting cerebral perfusion and motor learning in Parkinson's disease. Brain Imaging Behav 2019;13:577-87. [Crossref] [PubMed]
- Fazlollahi A, Calamante F, Liang X, Bourgeat P, Raniga P, Dore V, Fripp J, Ames D, Masters CL, Rowe CC, Connelly A, Villemagne VL, Salvado O. Increased cerebral blood flow with increased amyloid burden in the preclinical phase of alzheimer's disease. J Magn Reson Imaging 2020;51:505-13. [Crossref] [PubMed]
- Puig O, Henriksen OM, Vestergaard MB, Hansen AE, Andersen FL, Ladefoged CN, Rostrup E, Larsson HB, Lindberg U, Law I. Comparison of simultaneous arterial spin labeling MRI and (15)O-H2O PET measurements of regional cerebral blood flow in rest and altered perfusion states. J Cereb Blood Flow Metab 2020;40:1621-33. [Crossref] [PubMed]
- Havsteen I, Damm Nybing J, Christensen H, Christensen AF. Arterial spin labeling: a technical overview. Acta Radiol 2018;59:1232-8. [Crossref] [PubMed]
- Kübel S, Stegmayer K, Vanbellingen T, Walther S, Bohlhalter S. Deficient supplementary motor area at rest: Neural basis of limb kinetic deficits in Parkinson's disease. Hum Brain Mapp 2018;39:3691-700. [Crossref] [PubMed]
- Dolui S, Li Z, Nasrallah IM, Detre JA, Wolk DA. Arterial spin labeling versus (18)F-FDG-PET to identify mild cognitive impairment. Neuroimage Clin 2020;25:102146 [Crossref] [PubMed]
- Ma Y, Huang C, Dyke JP, Pan H, Alsop D, Feigin A, Eidelberg D. Parkinson's disease spatial covariance pattern: noninvasive quantification with perfusion MRI. J Cereb Blood Flow Metab 2010;30:505-9. [Crossref] [PubMed]
- Lee YH, Bak Y, Park CH, Chung SJ, Yoo HS, Baik K, Jung JH, Sohn YH, Shin NY, Lee PH. Patterns of olfactory functional networks in Parkinson's disease dementia and Alzheimer's dementia. Neurobiol Aging 2020;89:63-70. [Crossref] [PubMed]
- Petersen RC. Mild cognitive impairment as a diagnostic entity. J Intern Med 2004;256:183-94. [Crossref] [PubMed]
- Hughes AJ, Daniel SE, Kilford L, Lees AJ. Accuracy of clinical diagnosis of idiopathic Parkinson's disease: a clinico-pathological study of 100 cases. J Neurol Neurosurg Psychiatry 1992;55:181-4. [Crossref] [PubMed]
- Litvan I, Goldman JG, Troster AI, Schmand BA, Weintraub D, Petersen RC, Mollenhauer B, Adler CH, Marder K, Williams-Gray CH, Aarsland D, Kulisevsky J, Rodriguez-Oroz MC, Burn DJ, Barker RA, Emre M. Diagnostic criteria for mild cognitive impairment in Parkinson's disease: Movement Disorder Society Task Force guidelines. Mov Disord 2012;27:349-56. [Crossref] [PubMed]
- Tomlinson CL, Stowe R, Patel S, Rick C, Gray R, Clarke CE. Systematic review of levodopa dose equivalency reporting in Parkinson's disease. Mov Disord 2010;25:2649-53. [Crossref] [PubMed]
- Bian R, Zhang Y, Yang Y, Yin Y, Zhao X, Chen H, Yuan Y. White Matter Integrity Disruptions Correlate With Cognitive Impairments in Asthma. J Magn Reson Imaging 2018; Epub ahead of print. [Crossref] [PubMed]
- Ambarki K, Wahlin A, Zarrinkoob L, Wirestam R, Petr J, Malm J, Eklund A. Accuracy of Parenchymal Cerebral Blood Flow Measurements Using Pseudocontinuous Arterial Spin-Labeling in Healthy Volunteers. AJNR Am J Neuroradiol 2015;36:1816-21. [Crossref] [PubMed]
- Okonkwo OC, Xu G, Oh JM, Dowling NM, Carlsson CM, Gallagher CL, Birdsill AC, Palotti M, Wharton W, Hermann BP, LaRue A, Bendlin BB, Rowley HA, Asthana S, Sager MA, Johnson SC. Cerebral blood flow is diminished in asymptomatic middle-aged adults with maternal history of Alzheimer's disease. Cereb Cortex 2014;24:978-88. [Crossref] [PubMed]
- Aslan S, Lu H. On the sensitivity of ASL MRI in detecting regional differences in cerebral blood flow. Magn Reson Imaging 2010;28:928-35. [Crossref] [PubMed]
- Arslan DB, Gurvit H, Genc O, Kicik A, Eryurek K, Cengiz S, Erdogdu E, Yildirim Z, Tufekcioglu Z, Ulug AM, Bilgic B, Hanagasi H, Tuzun E, Demiralp T, Ozturk-Isik E. The cerebral blood flow deficits in Parkinson's disease with mild cognitive impairment using arterial spin labeling MRI. J Neural Transm (Vienna) 2020;127:1285-94. [Crossref] [PubMed]
- Rane S, Koh N, Oakley J, Caso C, Zabetian CP, Cholerton B, Montine TJ, Grabowski T. Arterial spin labeling detects perfusion patterns related to motor symptoms in Parkinson's disease. Parkinsonism Relat Disord 2020;76:21-8. [Crossref] [PubMed]
- Xie L, Das SR, Pilania A, Daffner M, Stockbower GE, Dolui S, Yushkevich PA, Detre JA, Wolk DA. Task-enhanced arterial spin labeled perfusion MRI predicts longitudinal neurodegeneration in mild cognitive impairment. Hippocampus 2019;29:26-36. [Crossref] [PubMed]
- Pelizzari L, Lagana MM, Di Tella S, Rossetto F, Bergsland N, Nemni R, Clerici M, Baglio F. Combined Assessment of Diffusion Parameters and Cerebral Blood Flow Within Basal Ganglia in Early Parkinson's Disease. Front Aging Neurosci 2019;11:134. [Crossref] [PubMed]
- Melzer TR, Watts R, MacAskill MR, Pearson JF, Rueger S, Pitcher TL, Livingston L, Graham C, Keenan R, Shankaranarayanan A, Alsop DC, Dalrymple-Alford JC, Anderson TJ. Arterial spin labelling reveals an abnormal cerebral perfusion pattern in Parkinson's disease. Brain 2011;134:845-55. [Crossref] [PubMed]
- Tzourio-Mazoyer N, Landeau B, Papathanassiou D, Crivello F, Etard O, Delcroix N, Mazoyer B, Joliot M. Automated anatomical labeling of activations in SPM using a macroscopic anatomical parcellation of the MNI MRI single-subject brain. Neuroimage 2002;15:273-89. [Crossref] [PubMed]
- Zhu J, Zhuo C, Qin W, Xu Y, Xu L, Liu X, Yu C. Altered resting-state cerebral blood flow and its connectivity in schizophrenia. J Psychiatr Res 2015;63:28-35. [Crossref] [PubMed]
- Zhang YN, Huang YR, Liu JL, Zhang FQ, Zhang BY, Wu JC, Ma Y, Xia J, Hao Y, Huo JW. Aberrant resting-state cerebral blood flow and its connectivity in primary dysmenorrhea on arterial spin labeling MRI. Magn Reson Imaging 2020;73:84-90. [Crossref] [PubMed]
- Eidelberg D. Metabolic brain networks in neurodegenerative disorders: a functional imaging approach. Trends Neurosci 2009;32:548-57. [Crossref] [PubMed]
- Mattis PJ, Niethammer M, Sako W, Tang CC, Nazem A, Gordon ML, Brandt V, Dhawan V, Eidelberg D. Distinct brain networks underlie cognitive dysfunction in Parkinson and Alzheimer diseases. Neurology 2016;87:1925-33. [Crossref] [PubMed]
- Anderkova L, Barton M, Rektorova I. Striato-cortical connections in Parkinson's and Alzheimer's diseases: Relation to cognition. Mov Disord 2017;32:917-22. [Crossref] [PubMed]
- Ekman U, Eriksson J, Forsgren L, Domellof ME, Elgh E, Lundquist A, Nyberg L. Longitudinal changes in task-evoked brain responses in Parkinson's disease patients with and without mild cognitive impairment. Front Neurosci 2014;8:207. [Crossref] [PubMed]
- Aarsland D. Cognitive impairment in Parkinson's disease and dementia with Lewy bodies. Parkinsonism Relat Disord 2016;22:S144-8. [Crossref] [PubMed]
- Brooks DJ, Piccini P. Imaging in Parkinson's disease: the role of monoamines in behavior. Biol Psychiatry 2006;59:908-18. [Crossref] [PubMed]
- Chen Y, Pressman P, Simuni T, Parrish TB, Gitelman DR. Effects of acute levodopa challenge on resting cerebral blood flow in Parkinson's Disease patients assessed using pseudo-continuous arterial spin labeling. PeerJ 2015;3:e1381 [Crossref] [PubMed]
- Wu T, Wang L, Hallett M, Li K, Chan P. Neural correlates of bimanual anti-phase and in-phase movements in Parkinson's disease. Brain 2010;133:2394-409. [Crossref] [PubMed]
- Ekman U, Eriksson J, Forsgren L, Mo SJ, Riklund K, Nyberg L. Functional brain activity and presynaptic dopamine uptake in patients with Parkinson's disease and mild cognitive impairment: a cross-sectional study. Lancet Neurol 2012;11:679-87. [Crossref] [PubMed]
- Luo C, Song W, Chen Q, Zheng Z, Chen K, Cao B, Yang J, Li J, Huang X, Gong Q, Shang HF. Reduced functional connectivity in early-stage drug-naive Parkinson's disease: a resting-state fMRI study. Neurobiol Aging 2014;35:431-41. [Crossref] [PubMed]
- Manza P, Zhang S, Li CS, Leung HC. Resting-state functional connectivity of the striatum in early-stage Parkinson's disease: Cognitive decline and motor symptomatology. Hum Brain Mapp 2016;37:648-62. [Crossref] [PubMed]
- Kalia LV, Lang AE. Parkinson's disease. Lancet 2015;386:896-912. [Crossref] [PubMed]
- Benedictus MR, Leeuwis AE, Binnewijzend MA, Kuijer JP, Scheltens P, Barkhof F, van der Flier WM, Prins ND. Lower cerebral blood flow is associated with faster cognitive decline in Alzheimer's disease. Eur Radiol 2017;27:1169-75. [Crossref] [PubMed]