Associations between brain volumetry and relaxometry signatures and the Edmonton Frail Scale in frailty
Introduction
Frailty is a geriatric condition characterized by decreased reserve leading to a diminished capacity to respond to stressors (1,2). Frailty is closely associated with poor health outcomes, including falls, disability, and mortality (3-5). The frailty status can be evaluated by various assessment tools, such as the Fried frailty phenotype (6), the FRAIL scale (7), the clinical frailty scale (8), and the Edmonton frailty scale (EFS) (9). The EFS, developed by Rolfson et al., has been used widely in practice and is a valid and reliable tool to evaluate frailty that does not require any specialized training (9). It includes nine domains: cognition, general health status, functional independence, social support, medication use, nutrition, mood, continence, and functional performance. Despite multiple studies evaluating the EFS in frailty, none have focused on the brain relaxometry signatures that are correlated to this scale, which may help to reveal the underlying pathophysiological changes of the brain in frailty.
Relaxometry is sensitive to the brain’s composition and microstructure by reflecting the magnetization relaxation characteristics of brain tissue (10). Therefore, relaxometry characteristics have been investigated in several brain imaging studies (11-14). Relaxometry studies have also been conducted on other organs, such as the cartilage (15), kidney (16), etc. However, the application of relaxometry in clinical practice is limited by several factors. Firstly, conventional sequences for the acquisition of relaxometry are time-consuming (17). Secondly, acquisition of different relaxometry through different sequences usually leads to misregistration among images due to patient motion, resulting in difficulties in obtaining an accurate overall evaluation with multiple relaxometry parameters.
The emergence of synthetic magnetic resonance imaging (MRI) overcomes the limitations above and allows for the clinical application of relaxometry evaluation. Synthetic MRI is based on the quantification of relaxation times and proton density (PD) by the multi-echo acquisition of a saturation-recovery using the turbo spin-echo readout (QRAPMASTER) method (18). This technique enables simultaneous quantification of T1, T2, and PD values, as well as the synthesis of multiple contrast-weighted images from one scan in several minutes (19,20). Moreover, synthetic MRI offers volumetry information of different types of brain tissues through an automatic segmentation of brain tissue based on the relaxation values (20), including volumes of gray matter (GM), white matter (WM), cerebral spinal fluid (CSF), and myelin content. Thus, synthetic MRI can provide volumetry and relaxometry information within one scan, making a quantitative evaluation of the central nervous system feasible in clinics.
The accumulation of common brain pathologies helps to bring about the progression of physical frailty in the elderly (21). Since relaxometry can reflect the composition changes, we can infer that relaxometry changes may exist in frailty. Also, reduced global and regional brain volume has been identified in frailty in some previous studies (22,23), which used three-dimensional T1-weighted images for volume analysis and the Fried frailty phenotype for frailty assessment. No previous reports have explored whether synthetic MRI signatures, including volumetry and relaxometry signatures, are correlated with the EFS in frailty to the best of our knowledge. The present study aimed to investigate the following: (I) the association between brain volumetry and relaxometry signatures and the EFS in frailty; and (II) the association between brain volumetry and relaxometry signatures and each domain score of the EFS.
Methods
Subjects
The local institutional review board approved this prospective study (No. 2018BJYYEC-121-02). From September 2018 to February 2019, 109 consecutive inpatients in the neurology ward (aged ≥65) were enrolled in this study. The exclusion criteria were as follows: (I) patients that were unable to provide informed consent (n=10); (II) patients who could not cooperate with the assessment procedure (such as deafness, unstable vital signs, etc.) (n=4); (III) patients with Parkinson’s disease (n=12); (IV) patients that had experienced acute ischemic stroke (n=21); (V) those without EFS evaluation (n=6); (VI) patients with severe stage of Alzheimer’s disease (Mini-Mental State Examination <15) or other dementia (n=4); (VII) those with contraindications for MRI examination (n=5); (VIII) patients with severe cerebral pathologies on MRI (n=3); and (IX) patients with incomplete MRI scans or unsatisfactory image quality (n=4). Finally, 40 subjects were included in this study. Written informed consent was obtained from each subject before participation in this study.
Frailty assessment
Trained researchers performed frailty assessment according to the EFS. The EFS assesses nine domains related to frailty, including cognition, general health status, functional independence, social support, medication usage, nutrition, mood, continence, and functional performance, with scores ranging from 0 to 17. Subjects with a score ≥8 were classified as frail (9).
MRI protocol
All MRI data were acquired using a 3.0 T MR scanner (SIGNA Pioneer, GE Healthcare, Milwaukee, WI, USA) equipped with a 32-channel head-neck receiver coil. Routine MR sequences, including axial T2-weighted imaging, T1-weighted imaging, T2 fluid-attenuated inversion recovery, diffusion-weighted imaging, and sagittal T1-weighted imaging, were performed initially to exclude subjects with severe cerebral pathologies. Synthetic MRI (MAGnetic resonance image Compilation, MAGiC) was acquired through the whole head with an oblique axial prescription parallel to the anterior commissure-posterior commissure line. The acquisition parameters of MAGiC were as follows: field of view =240 mm × 240 mm; matrix =320×256; repetition time =4,906 ms; echo time 1/echo time 2 =22.0/87.9 ms; echo-train length =12; bandwidth =22.73 kHz; number of excitation =1; slice thickness/gaP =4/0.5 mm; slice number =32. The scan time for MAGiC was 4 mins 55 sec. T1-weighted structural images were also acquired using a three-dimensional fast spoiled gradient-recalled echo (3D-FSPGR) sequence, with the following acquisition parameters: field of view =256 mm × 256 mm; matrix =256×256; repetition time =6.0 ms; echo time =1.9 ms; flip angle =11°; bandwidth =31.25 kHz; number of excitation=1; slice thickness/gaP=1/0 mm; slice number =200. The scan time for 3D-FSPGR was 4 min 19 sec.
Image processing and analysis
Relaxation maps and brain segmentation results were generated from the MAGiC raw data using SyMRI 8.0 software (SyntheticMR AB, Linköping, Sweden). Global volumetry including gray matter volume (GMV), white matter volume (WMV), cerebral spinal fluid volume (CSFV), myelin volume (MYV), brain parenchymal volume (BPV), intracranial volume (ICV), gray matter fraction (GMF = GMV/BPV), white matter fraction (WMF = WMV/BPV), myelin fraction (MYF = MYV/BPV), GMV/ICV, WMV/ICV, CSF/ICV, and MYV/ICV were obtained. The processing steps for acquiring the global relaxometry, regional relaxometry, and volumetry were as follows. Firstly, a rigid registration between the relaxation maps acquired from the MAGiC, and the images of 3D-FSPGR were performed using SPM12 software (http://www.fil.ion.ucl.ac.uk/spm/). Secondly, segmentation and normalization [Montreal Neuroscience Institute (MNI) space] were performed using the CAT12 toolbox (http://www.neuro.uni-jena.de/cat/) implemented in SPM12 to obtain the tissue probability maps and normalized relaxation maps. The global relaxometry in GM, WM, and CSF was calculated by averaging the relaxation values from voxels with partial volume exceeding 95% of the corresponding tissue. Finally, the Anatomical Automatic Labeling (AAL) atlas (http://www.gin.cnrs.fr/en/tools/aal/) was used to extract the brain regional relaxometry and volumetry without distinguishing between the left and right hemisphere (33 regions).
Statistical analysis
All data were analyzed using the SPSS 13.0 statistical package (IBM, Armonk, NY, USA) and MATLAB (MathWorks, Natick, MA, USA). Considering the association between the brain's volumetry and relaxometry with age, the associations between the total EFS (and the score of each domain) and the volumetry and relaxometry of the brain were investigated by partial correlation eliminating the effects of age. Multiple comparisons of regional GM volumetry and relaxometry analyses were controlled using false discovery rate (FDR) correction according to 33 selected brain regions. The results of correlations between the social support score of the EFS and volumetry or relaxometry could not be computed, as the social support score of the subjects was constant (0). The level of significance was set at P<0.05.
Results
The demographic and clinical data of the 40 subjects are shown in Table 1.
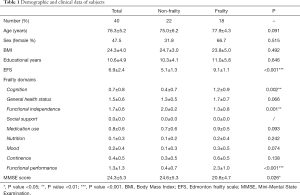
Full table
Global volumetry, regional GM volumetry, and global relaxometry showed strong correlations with the EFS
Results of correlations between the EFS and global volumetry are shown in Table 2. Results of correlations between the EFS and regional GM volumetry, which had significant correlations, are shown in Table 2 and Figure 1. Multiple regional GM volumetry showed significant correlations with the EFS. Results of correlations between the EFS and global relaxometry, which had significant correlations, are shown in Table 3. No significant correlation was found between the EFS and regional GM relaxometry.

Full table

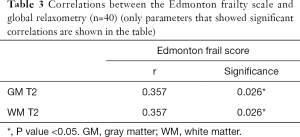
Full table
Both global volumetry and global relaxometry showed strong correlations with some domain scores of the EFS
Results of correlations between domain scores of the EFS and global volumetry, which had significant correlations, are shown in Table 4. Results of correlations between domain scores of the EFS and global relaxometry, which had significant correlations, are shown in Table 5.
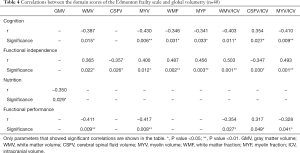
Full table
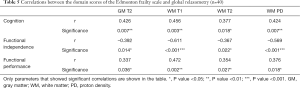
Full table
Some regional GM volumetry showed strong negative correlations with the functional performance score
Results of correlations between the functional performance score and regional GM volumetry are shown in Figure 2. Significant negative correlations were identified between the functional performance score and the GMV of rolandic (r=–0.394, P=0.043), olfactory (r=–0.458, P=0.035), rectus (r=–0.394, P=0.043), cingulate (r=–0.411, P=0.039), hippocampus (r=–0.479, P=0.034), parahippocampal (r=–0.448, P=0.035), and precuneus (r=–0.414, P=0.039). No notable correlations were found between regional GMV and the cognition score, general health status score, functional independence score, medication use score, nutrition score, mood score, or continence score.

Some regional GM relaxometry showed strong correlations with the functional independence score
Results of correlations between the functional independence score and regional GM T1 values are shown in Figure 3. Significant negative correlations were found between the functional independence score and the T1 value of caudate (r=–0.617, P<0.001). No notable correlations were identified between the other scores and regional GM T1 values.

Results of correlations between the functional independence score and regional GM T2 values are shown in Figure 4. Significant negative correlations were identified between the functional independence score and the T2 values of insula (r=–0.510, P=0.015) and caudate (r=–0.633, P<0.001). No notable correlations were found between the other scores and regional GM T2 values. Furthermore, no significant correlations between the domain scores of the EFS and regional GM PD values were identified.

Discussion
This was the first study to explore the association between brain volumetry and relaxometry signatures with the EFS (and each domain score of the EFS) in frailty. We used partial correlation to eliminate the influence of age, as age is closely related to frailty and may also cause changes in brain volumetry and relaxometry. Moreover, we added FDR correction to the P value to control multiple comparisons in regional GM volumetry and relaxometry analyses. We found that both brain volumetry and relaxometry showed strong correlations with the EFS and some EFS domain scores, demonstrating the potential of synthetic MRI to reveal the underlying pathophysiology of frailty.
In the present study, global brain volume and multiple regional GMV showed significant negative correlations with the EFS. This was consistent with the results of several previous studies, which showed that reduced global and regional brain volume changes were found in frailty (22-24), despite differing frailty assessment tools. Such results demonstrate the progressive brain atrophy during the development of frailty. It is important to emphasize that brain atrophy during frailty involves the entire brain, including GM, WM, and myelin, which indicates that the brain's pathophysiological changes during frailty may be global, as opposed to being limited to a specific region of the brain.
Brain relaxometry changes were also found in frailty, with significant correlations between the EFS and global relaxometry. As we know, MRI relaxation times are sensitive to changes in brain tissue microstructure integrity and tissue composition (25-29). As for the central nervous system, changes in relaxation times contribute to demyelination, neuronal cell death, axonal loss, and water content changes (14,30-32). Based on these previous studies’ results, we can speculate about the underlying pathophysiology of frailty according to our results. For global relaxometry, positive correlations were identified between the EFS and T2 values of global GM and WM, while T1 and PD values of global GM and WM did not show significant correlations with the EFS. This may be attributable to the fact that T2 is generally more sensitive to pathophysiology than T1 and PD, previously reported (25). It seems that the T2 value of brain tissue increases with the progression of frailty. The influencing factors of T2 mainly include water content, myelin density (33), and the presence of paramagnetic molecules, such as iron (34) at a given field strength. The increase of T2 may be due to increased water content, myelin loss, axonal loss, and gliosis. However, it is difficult to confirm which factor/s plays the dominant part in the changes to T2 value. For regional GM relaxometry, no significant correlations were found with the EFS. Two possible reasons may have contributed to this result. Firstly, the pathophysiological changes in brain GM might be global during frailty instead of being limited to a single region. Secondly, the present study's small sample size may have limited the significance of regional GM relaxometry alteration, especially when the number of analyzed brain regions was relatively large. A larger study in the future can solve this. Furthermore, we did not analyze the regional WM relaxometry in the present study. Whether regional WM relaxometry shows differences in frailty should also be addressed in the future.
We further analyzed the associations between brain volumetry and relaxometry signatures with each EFS domain score in frailty. The results showed that reduced WMV and MYV were mainly correlated with the cognition and functional performance scores for global volumetry, while reduced GMV was mainly correlated with the nutrition score. These results indicated that reduced WM and GM volumes might play different roles in the EFS domains. Specifically, a reduced WM volume plays a dominant role in the cognition and functional performance scores, while reduced GM volume plays a dominant role in the nutrition score. Interestingly, the functional independence score results were positively correlated with WMV and MYV, which seemed to be difficult for giving a reasonable explanation. Generally, a reduced volume of brain tissue should be more reasonable for the progression of frailty, which means that negative correlation between the functional independence score and brain volume might be more reasonable. Such contradictory results require further confirmation in future research.
For global relaxometry, both the cognition and functional performance scores were positively correlated with the T2 of GM, and the T1, T2, and PD of WM, while the functional independence score was negatively correlated with the T2 of GM, and the T1, T2, and PD of WM. These results were similar to those of global volumetry, which showed reverse correlations for the cognition, functional performance (negative), and functional independence (positive) scores. After we noticed these coincidental results, we performed a correlation analysis for the domain scores. Interestingly, the cognition and functional performance scores showed a significantly positive correlation, and both showed notably negative correlations with the functional independence score (Table S1). Two possible reasons may explain these results. Firstly, the cognition and functional performance scores' underlying pathological basis was contrary to that of the functional independence score. Secondly, the correlations between these three domain scores were only occasional in the present study subjects. However, it was difficult for us to confirm which of these explanations was correct, and thus, further studies with larger samples are needed in the future.
The results of the cognition score deserve greater consideration. In the present study, the cognition score was correlated with reduced WMV, MYV, WMF, MYF, WMV/ICV, and MYV/ICV, and increased CSF/ICV. This indicated that the decreased volume of global WM and myelin, as opposed to GM, was mainly correlated with the cognition score. The lack of a significant correlation between the cognition score and regional GMV further confirms this point. However, previous studies reported significant risk factors for dementia, including lower volumes of the hippocampus (35), entorhinal cortex, and surrounding structures (36). Their findings seemed to differ from our results. We focused on the regional GM volume, while previous studies focused on the overall brain region, including both GM and WM, which may have led to disparate results. As for relaxometry, the cognition score was positively correlated with the T2 of GM and with the T1, T2, and PD of WM. One possible pathophysiological change for this may be increased water content in brain tissue. Moreover, myelin loss, axonal loss, and gliosis may also exist in the WM. It has been shown that the volume of WM hyperintensities (WMHs) may have significant neuropathological and clinical associations with cognitive impairment (35,37). Also, Zeng et al. identified a relationship between lower fractional anisotropy (FA) and higher-grade WMHs, which implied an underlying pathology of demyelination and axonal loss (37). This was consistent with our finding and speculation regarding the relaxometry changes in WM. No regions showed increased or decreased relaxometry with the cognition score, even though the classic regions which are related to cognition, such as the hippocampus and parahippocampal regions. It is important to note that, in the present study, we only focused on the GM of these regions. The WM changes in these regions remain unclear. Further study is needed for regional relaxometry evaluation of these regions, including both GM and WM.
Correlations were found between the nutrition score and reduced global GMV. However, global relaxometry, regional GM volumetry, or GM relaxometry did not significantly correlate with the nutrition score. The nutrition score of the EFS is mainly focused on weight loss. Drummen et al. reported no relationship between weight loss and global GM volume or cortical thickness and observed changes in cortical thickness in the right and left anterior cingulate gyrus following the weight-loss period (38). However, the participants in their study were aged 25–70 years, with a body mass index (BMI) ≥ of 25 kg/m2, which may have led to the differences between the volumetry results of the present study and those of Drummen et al. To date, there are no studies that have reported the results of brain relaxometry changes in weight loss, and the results remain unclear. As body weight regulation is extremely complex, involving the brain structure and brain activity and hormones, brain volumetry, and relaxometry changes related to the nutrition score still require further confirmation.
As for the mood score, continence score, general health status score, and medication use score, no significant correlations were found between them and global or regional GM parameters. Mood score in the EFS mainly focuses on whether the subject often feels sad or depressed. Regarding depression, several regional GMV changes have been identified in previous studies, including volume increase and reduction in different regions, such as the amygdala, the dorsal frontomedian cortex, and the right paracingulate cortex, etc. (39-41). To date, definite relaxometry changes of brain regions in depression remain unclear. As the evaluation of mood in the EFS is different from the evaluation of depression in previous studies, it is difficult to compare our results with previous research. As for continence, urge incontinence is common in the elderly. Positron emission tomography (PET) and functional MRI (fMRI) have unveiled a network of brain regions involved in the control of urine (42), among which the most firmly established ones were the periaqueductal gray, the insula, and the dorsal anterior cingulate cortex (43). Furthermore, the prefrontal cortex was also mentioned (43). Owing to the differences in techniques between the present study and previous studies, comparisons of the results are not available. As for the general health status score and medication use score, both are overall evaluations of health status and medication use, which may also be influenced by subjective factors and may have led to the present study’s negative results. Moreover, we noticed that the mood score, continence score, general health status score, and medication use score did not exhibit significant differences between the non-frailty and frailty groups in the present study. This point may also influence the correlation analysis results to some extent. Overall, the confirmation of volumetry and relaxometry changes related to the four scores mentioned above still requires further study with larger sample sizes.
To date, the underlying pathology of frailty remains unclear, as are the underlying pathologies of each domain of the EFS. This makes it difficult to explain the exact mechanisms of the volumetry and relaxometry results, especially the relaxometry results. According to our review of previous studies we made some speculations; however, it remains challenging to confirm which factors may drive relaxation upwards or result in the opposite effect in vivo, as the factors underpinning relaxation are extremely complex (13). The possible mechanisms of the EFS and its domain scores still need further investigation. However, the present study results indicated the significant potential of synthetic MRI in revealing the brain’s underlying pathophysiological changes in frailty, including changes in brain volume, brain tissue microstructure integrity, and tissue composition.
There were some limitations to the present study that should be noted. Firstly, the sample size was small. Secondly, this was a cross-sectional study, making it difficult to confirm the order of volumetry and relaxometry changes and frailty development. Thirdly, regional WM parameters were not involved. Fourthly, the subjects’ social support score was constant, resulting in a lack of analysis of correlations between the social support score and brain parameters. A longitudinal follow-up study with larger sample size and more comprehensive analysis for further investigation of the EFS in the future may resolve the above limitations.
In conclusion, brain volumetry and relaxometry signatures showed strong associations with the EFS and some EFS domain scores in frailty. The associations may reveal possible underlying pathophysiology (changes in brain volume, brain tissue microstructure integrity, and tissue composition) of the EFS and may even reveal different EFS domains.
Acknowledgments
The authors thank Dr. Na Lu and Dr. Yuwei Jiang (Beijing Hospital) for MRI data acquisition, and Dr. Qianwei Zheng, Dr. Fang Liu, and Dr. Yaodan Liang (Beijing Hospital) for clinical data acquisition.
Funding: This work was supported in part by grants from the National Natural Science Foundation of China (81771826), the CAMS Innovation Fund for Medical Sciences (2018-I2M-1-002), and the Beijing Municipal Science & Technology Commission (D181100000218003).
Footnote
Conflicts of Interest: All authors have completed the ICMJE uniform disclosure form (available at http://dx.doi.org/10.21037/qims-20-852). Dr. MW reports grants from Beijing Municipal Science & Technology Commission, during the conduct of the study. Dr. MC reports grants from National Natural Science Foundation of China, grants from CAMS Innovation Fund for Medical Sciences, during the conduct of the study. The other authors have no conflicts of interest to declare.
Ethical Statement: The trial was conducted in accordance with the Declaration of Helsinki. The study was approved by the local institution review board of Beijing Hospital (No. 2018BJYYEC-121-02), and informed consent was obtained from all patients.
Open Access Statement: This is an Open Access article distributed in accordance with the Creative Commons Attribution-NonCommercial-NoDerivs 4.0 International License (CC BY-NC-ND 4.0), which permits the non-commercial replication and distribution of the article with the strict proviso that no changes or edits are made and the original work is properly cited (including links to both the formal publication through the relevant DOI and the license). See: https://creativecommons.org/licenses/by-nc-nd/4.0/.
References
- Bergman H, Ferrucci L, Guralnik J, Hogan DB, Hummel S, Karunananthan S, Wolfson C. Frailty: an emerging research and clinical paradigm--issues and controversies. J Gerontol A Biol Sci Med Sci 2007;62:731-7. [Crossref] [PubMed]
- Clegg A, Young J, Iliffe S, Rikkert MO, Rockwood K. Frailty in elderly people. Lancet 2013;381:752-62. [Crossref] [PubMed]
- Chang SF, Lin PL. Frail phenotype and mortality prediction: a systematic review and meta-analysis of prospective cohort studies. Int J Nurs Stud 2015;52:1362-74. [Crossref] [PubMed]
- Rockwood K, Mitnitski A, Song X, Steen B, Skoog I. Long-term risks of death and institutionalization of elderly people in relation to deficit accumulation at age 70. J Am Geriatr Soc 2006;54:975-9. [Crossref] [PubMed]
- Mitnitski A, Song X, Skoog I, Broe GA, Cox JL, Grunfeld E, Rockwood K. Relative fitness and frailty of elderly men and women in developed countries and their relationship with mortality. J Am Geriatr Soc 2005;53:2184-9. [Crossref] [PubMed]
- Fried LP, Tangen CM, Walston J, Newman AB, Hirsch C, Gottdiener J, Seeman T, Tracy R, Kop WJ, Burke G, McBurnie MACardiovascular Health Study Collaborative Research Group. Frailty in older adults: evidence for a phenotype. J Gerontol A Biol Sci Med Sci 2001;56:M146-56. [Crossref] [PubMed]
- Morley JE, Malmstrom TK, Miller DK. A simple frailty questionnaire (FRAIL) predicts outcomes in middle aged African Americans. J Nutr Health Aging 2012;16:601-8. [Crossref] [PubMed]
- Rockwood K, Song X, MacKnight C, Bergman H, Hogan DB, McDowell I, Mitnitski A. A global clinical measure of fitness and frailty in elderly people. CMAJ 2005;173:489-95. [Crossref] [PubMed]
- Rolfson DB, Majumdar SR, Tsuyuki RT, Tahir A, Rockwood K. Validity and reliability of the Edmonton Frail Scale. Age Ageing 2006;35:526-9. [Crossref] [PubMed]
- Does MD. Inferring brain tissue composition and microstructure via MR relaxometry. NeuroImage 2018;182:136-48. [Crossref] [PubMed]
- Chen LW, Wang ST, Huang CC, Tu YF, Tsai YS. T2 Relaxometry MRI Predicts Cerebral Palsy in Preterm Infants. Am J Neuroradiol 2018;39:563-8. [Crossref] [PubMed]
- Hasan KM, Halphen C, Boska MD, Narayana PA. Diffusion tensor metrics, T2 relaxation, and volumetry of the naturally aging human caudate nuclei in healthy young and middle-aged adults: possible implications for the neurobiology of human brain aging and disease. Magn Reson Med 2008;59:7-13. [Crossref] [PubMed]
- Necus J, Smith FE, Thelwall PE, Flowers CJ, Sinha N, Taylor PN, Blamire AM, Wang Y, Cousins DA. Quantification of brain proton longitudinal relaxation (T1) in lithium-treated and lithium-naïve patients with bipolar disorder in comparison to healthy controls. Bipolar Disord 2021;23:41-8. [Crossref] [PubMed]
- Gracien RM, Reitz SC, Hof SM, Fleischer V, Zimmermann H, Droby A, Steinmetz H, Zipp F, Deichmann R, Klein JC. Changes and variability of proton density and T1 relaxation times in early multiple sclerosis: MRI markers of neuronal damage in the cerebral cortex. Eur Radiol 2016;26:2578-86. [Crossref] [PubMed]
- Wan C, Ge L, Souza RB, Tang SY, Alliston T, Hao Z, Li X. T1rho-based fibril-reinforced poroviscoelastic constitutive relation of human articular cartilage using inverse finite element technology. Quant Imaging Med Surg 2019;9:359-70. [Crossref] [PubMed]
- Wang S, Li J, Zhu D, Hua T, Zhao B. Contrast-enhanced magnetic resonance (MR) T1 mapping with low-dose gadolinium-diethylenetriamine pentaacetic acid (Gd-DTPA) is promising in identifying clear cell renal cell carcinoma histopathological grade and differentiating fat-poor angiomyolipoma. Quant Imaging Med Surg 2020;10:988-98. [Crossref] [PubMed]
- Bojorquez JZ, Bricq S, Acquitter C, Brunotte F, Walker PM, Lalande A. What are normal relaxation times of tissues at 3 T? Magn Reson Imaging 2017;35:69-80. [Crossref] [PubMed]
- Warntjes JB, Leinhard OD, West J, Lundberg P. Rapid magnetic resonance quantification on the brain: Optimization for clinical usage. Magn Reson Med 2008;60:320-9. [Crossref] [PubMed]
- Gonçalves FG, Serai SD, Zuccoli G. Synthetic Brain MRI: Review of Current Concepts and Future Directions. Top Magn Reson Imaging 2018;27:387-93. [Crossref] [PubMed]
- Hagiwara A, Warntjes M, Hori M, Andica C, Nakazawa M, Kumamaru KK, Abe O, Aoki S. SyMRI of the Brain: Rapid Quantification of Relaxation Rates and Proton Density, With Synthetic MRI, Automatic Brain Segmentation, and Myelin Measurement. Invest Radiol 2017;52:647-57. [Crossref] [PubMed]
- Buchman AS, Yu L, Wilson RS, Schneider JA, Bennett DA. Association of brain pathology with the progression of frailty in older adults. Neurology 2013;80:2055-61. [Crossref] [PubMed]
- Chen WT, Chou KH, Liu LK, Lee PL, Lee WJ, Chen LK, Wang PN, Lin CP. Reduced cerebellar gray matter is a neural signature of physical frailty. Hum Brain Mapp 2015;36:3666-76. [Crossref] [PubMed]
- Kant IMJ, de Bresser J, van Montfort SJT, Aarts E, Verlaan JJ, Zacharias N, Winterer G, Spies C, Slooter AJC, Hendrikse J. BioCog C. The association between brain volume, cortical brain infarcts, and physical frailty. Neurobiol Aging 2018;70:247-53. [Crossref] [PubMed]
- Nishita Y, Nakamura A, Kato T, Otsuka R, Iwata K, Tange C, Ando F, Ito K, Shimokata H, Arai H. Links Between Physical Frailty and Regional Gray Matter Volumes in Older Adults: A Voxel-Based Morphometry Study. J Am Med Dir Assoc 2019;20:1587-92.e7. [Crossref] [PubMed]
- Tang X, Cai F, Ding DX, Zhang LL, Cai XY, Fang Q. Magnetic resonance imaging relaxation time in Alzheimer's disease. Brain Res Bull 2018;140:176-89. [Crossref] [PubMed]
- Callaghan MF, Freund P, Draganski B, Anderson E, Cappelletti M, Chowdhury R, Diedrichsen J, Fitzgerald TH, Smittenaar P, Helms G, Lutti A, Weiskopf N. Widespread age-related differences in the human brain microstructure revealed by quantitative magnetic resonance imaging. Neurobiol Aging 2014;35:1862-72. [Crossref] [PubMed]
- Callaghan MF, Helms G, Lutti A, Mohammadi S, Weiskopf N. A general linear relaxometry model of R1 using imaging data. Magn Reson Med 2015;73:1309-14. [Crossref] [PubMed]
- Stüber C, Morawski M, Schäfer A, Labadie C, Wähnert M, Leuze C, Streicher M, Barapatre N, Reimann K, Geyer S, Spemann D, Turner R. Myelin and iron concentration in the human brain: a quantitative study of MRI contrast. NeuroImage 2014;93:95-106. [Crossref] [PubMed]
- Suzuki S, Sakai O, Jara H. Combined volumetric T1, T2 and secular-T2 quantitative MRI of the brain: age-related global changes (preliminary results). Magn Reson Imaging 2006;24:877-87. [Crossref] [PubMed]
- Tang X, Wu D, Yao Y, Gu L, Chen X, Ren Q, Zhang Z. Dorsal hippocampal changes in T2 relaxation times are associated with early spatial cognitive deficits in 5XFAD mice. Brain Res Bull 2019;153:150-61. [Crossref] [PubMed]
- Alshelh Z, Di Pietro F, Mills EP, Vickers ER, Peck CC, Murray GM, Henderson LA. Altered regional brain T2 relaxation times in individuals with chronic orofacial neuropathic pain. NeuroImage Clin 2018;19:167-73. [Crossref] [PubMed]
- Baudrexel S, Nurnberger L, Rub U, Seifried C, Klein JC, Deller T, Steinmetz H, Deichmann R, Hilker R. Quantitative mapping of T1 and T2* discloses nigral and brainstem pathology in early Parkinson's disease. NeuroImage 2010;51:512-20. [Crossref] [PubMed]
- Paus T, Collins DL, Evans AC, Leonard G, Pike B, Zijdenbos A. Maturation of white matter in the human brain: a review of magnetic resonance studies. Brain Res Bull 2001;54:255-66. [Crossref] [PubMed]
- Jara H, Sakai O, Mankal P, Irving RP, Norbash AM. Multispectral quantitative magnetic resonance imaging of brain iron stores: a theoretical perspective. Top Magn Reson Imaging 2006;17:19-30. [Crossref] [PubMed]
- Wu CC, Mungas D, Petkov CI, Eberling JL, Zrelak PA, Buonocore MH, Brunberg JA, Haan MN, Jagust WJ. Brain structure and cognition in a community sample of elderly Latinos. Neurology 2002;59:383-91. [Crossref] [PubMed]
- Wu A, Sharrett AR, Gottesman RF, Power MC, Mosley TH Jr, Jack CR Jr, Knopman DS, Windham BG, Gross AL, Coresh J. Association of Brain Magnetic Resonance Imaging Signs With Cognitive Outcomes in Persons With Nonimpaired Cognition and Mild Cognitive Impairment. JAMA Netw Open 2019;2:e193359 [Crossref] [PubMed]
- Zeng W, Chen Y, Zhu Z, Gao S, Xia J, Chen X, Jia J, Zhang Z. Severity of white matter hyperintensities: Lesion patterns, cognition, and microstructural changes. J Cereb Blood Flow Metab 2020;40:2454-63. [Crossref] [PubMed]
- Drummen M, Heinecke A, Dorenbos E, Vreugdenhil A, Raben A, Westerterp-Plantenga MS, Adam TC. Reductions in body weight and insulin resistance are not associated with changes in grey matter volume or cortical thickness during the PREVIEW study. J Neurol Sci 2019;403:106-11. [Crossref] [PubMed]
- Li H, Wei D, Sun J, Chen Q, Zhang Q, Qiu J. Brain structural alterations associated with young women with subthreshold depression. Sci Rep 2015;5:9707. [Crossref] [PubMed]
- Zhang FF, Peng W, Sweeney JA, Jia ZY, Gong QY. Brain structure alterations in depression: Psychoradiological evidence. CNS Neurosci Ther 2018;24:994-1003. [Crossref] [PubMed]
- Sacher J, Neumann J, Funfstuck T, Soliman A, Villringer A, Schroeter ML. Mapping the depressed brain: a meta-analysis of structural and functional alterations in major depressive disorder. J Affect Disorders 2012;140:142-8. [Crossref] [PubMed]
- Fowler CJ, Griffiths D, de Groat WC. The neural control of micturition. Nat Rev Neurosci 2008;9:453-66. [Crossref] [PubMed]
- Griffiths DJ, Tadic SD, Schaefer W, Resnick NM. Cerebral control of the lower urinary tract: how age-related changes might predispose to urge incontinence. Neuroimage 2009;47:981-6. [Crossref] [PubMed]