Deep transfer learning based on magnetic resonance imaging can improve the diagnosis of lymph node metastasis in patients with rectal cancer
Introduction
In patients with rectal cancer, lymph nodes (LNs) are one of the main areas of metastasis, and LN metastasis is the main cause of postoperative local recurrence and death (1). However, current diagnostic methods and criteria used for N staging of rectal carcinoma are unsatisfactory, and LN status has not effectively selected patients for preoperative chemoradiation therapy (CRT) (2,3). Therefore, accurate N staging in rectal cancer patients before treatment is important for determining the clinical stage, treatment strategy, and prognosis (4,5). Previous studies have revealed that the accuracy of endorectal ultrasounds (EUS) and computed tomography (CT) in detecting nodal metastases varied greatly (62–83% and 22–73%, respectively) (6,7). Magnetic resonance imaging (MRI) evaluates LN status by measuring the short axial (SA) diameter and can achieve 58–70% sensitivity and 75–85% specificity in identifying malignant nodes (8). Its use is limited by the size overlap between benign and malignant LNs (9) despite its popularity. Therefore, none of these current predictive methods are satisfactory.
Radiomics is a combined medical and industrial approach that uses advanced artificial intelligence (AI) to solve specific clinical problems. In recent years, radiomics has been used to evaluate multiple kinds of tumors and is increasingly being applied in the clinical setting (10,11). MRI-based radiomics models have been used to distinguish cancer from benign tissues and to reflect the histological characteristics of rectal cancer (12,13). AI can change diagnosis and management through its ability to make classifications that are difficult for human experts and its ability to review a large number of images (14) rapidly. Since it is difficult to acquire large amounts of data from medical images, transfer learning was adopted (15). Transfer learning is a type of deep learning that uses a pretrained model and requires fewer medical images. This method begins with initializing the network using pretrained weights from a similar architecture network and then fine-tunes the parameters to fit the target application. According to the class number in the new classification task, the last fully connected layer is usually replaced with as many neurons as the new class number (16).
To our knowledge, this is the first study to identify LN status using the deep transfer learning method on a node-by-node basis in patients with rectal cancer. This will provide clinicians with more reliable and accurate preoperative N staging diagnosis and assist with clinical treatments.
Methods
Patients
This prospective study was conducted between April 2018 and March 2019 and was approved by the Institutional Review Board at Harbin Medical University Cancer Hospital. Inclusion criteria were as follows: (I) patients diagnosed with rectal cancer by endoscopic biopsy and scheduled to undergo surgery within 2 weeks after MRI; (II) no history of treatment before the MRI; (III) no contraindications and can undergo high-resolution MRI; (IV) patients with at least one mesorectal (peritumoral) or superior mesenteric LNs on MRI; and (V) maximum SA diameter of LNs ≥3 mm. The following exclusion criteria were applied: (I) patients who received radiotherapy or chemotherapy before surgery; (II) patients with poor tolerance of MRI; (III) patients with no satisfactory MRI scans; and (IV) patients in whom the target LN could not be detected during surgery. Finally, a total of 129 patients with definite rectal cancer were recruited (Figure 1).
High-resolution rectal MRI parameters
All patients underwent rectal MRI before surgery using a Philips Achieva 3.0T MR scanner with a 16-channel torso array coil. An MR sagittal T2-weighted (T2W) scan sequence was obtained with the following parameters: TR/TE =3,000 ms/100 ms; number of signal frequency (NSA) =2; layer thickness =4.0 mm; and layer spacing =0.4 mm; FOV =240×240 mm. The rectal lesions’ position was determined in the sagittal position, which was perpendicular to the intestinal canal lesions, with a transverse T2W scan: TR =3,824 ms; TE =110 ms; NSA =3; layer thickness =3.5 mm; and interval =0.2 mm. According to the sagittal lesion position, patients with parallel pathological changes received a coronal T2W scan: TR =3,824 ms; TE =110 ms; NSA =3; layer thickness =3.0 mm; and layer spacing =0.2 mm. The LNs were then located in the sagittal, transverse, and coronal images.
Imaging assessment
LN location and image acquisition
The MR images (original images) were reviewed based on the consensus of one abdominal radiologist (R1) with 6-years’ experience in rectal MRIs. This radiologist determined the largest separable LN in the mesorectal or rectal superior artery region on the T2W images. The location and SA diameter of the LN was recorded.
To analyze the LN images blindly, avoiding influence from the primary tumor, radiologist R1 manually segmented the selected LNs on the maximum cross-sectional slices of the original images (including axis, sagittal, and coronal T2W images) using free, open-source software (Scrtopic1.0). All the LN screenshots underwent a minimal rectangular segmentation along the margin of the LNs. Each LN screenshot of T2W images in different directions in JPEG form was obtained for further analysis as a separate sample (Figure 2).
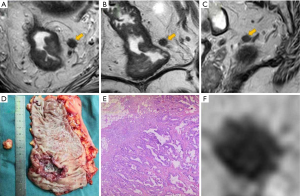
Qualitative evaluation of LN images
A second radiologist (R2) with 5-years’ experience and a third (R3) with 10-years’ experience reviewed the LN screenshots of the T2W images without SA diameter (Cohort 1) and identified their status independently. The criteria were based on irregular borders, heterogeneous signal intensity, and round shape. LNs with two or more of these criteria were considered suspicious positive. Radiologists R2 and R3 then reviewed the LN screenshots of the T2W images with SA diameter measurements (Cohort 2) and identified their status independently. The criteria were LNs with irregular borders, heterogeneous signal intensity, and round shape. For LNs with SA diameter <5 mm, 3 criteria were considered suspicious positive. For LNs with SA diameter between 5 and 9 mm, two criteria had to be suspicious, and for LNs with SA diameter >9 mm, all criteria had to be suspicious positive (17).
Ex vivo LN localization for node-by-node matching
Total mesorectal excision (TME) was performed within 2 weeks after MRI by a specialized colorectal surgeon. After surgery, the pathologist and radiologist R1 cooperatively matched the postoperative specimen with preoperative examination findings and located the target LN, which was then harvested for pathological examination (Figure 2). The LN status (benign and malignant) were depended on postoperative pathological results and classified as positive or negative.
Transfer learning: fine-tuning the convolutional neural network
Deep transfer learning is an AI method that is used for pre-training on large public imaging databases of networks and for extracting characteristics, such as edge, texture, and grayscale (18). These textures are applied to the target domain that contains small samples. Therefore, this method is suitable for medical imaging analysis. This study’s transfer learning method was executed on a pretrained Inception-v3 model, which had been trained for ImageNet Visual Recognition Challenge. As each LN’s size varied, the screenshots obtained were different (20×21 to 102×111 pixels). The Inception_v3 pre-trained model requires an input image dimension with 299×299 pixels. However, the cropped regions of interest (ROIs) were smaller than this size. Therefore, the samples were padded to match the dimensions of the model. All uniformed LN screenshots of T2W images were then inputted into the model. The first step was data set preparation. To maximize the training data volume and reduce the differences in neural network recognition performance, the medical imaging data was randomly divided into a training group and a validation group based on the images (each LN was considered a subject rather than each patient). The training dataset was 80% of the T2WI images, while the validation dataset was 20% of T2WI images, and these were distinct from the training datasets. For each image, the training set image was subtracted, and the input image was resized to match the input layer dimension of Inception-v3. Data augmentation was used to increase samples because it can expand the training dataset’s size, avoid overfitting, and help improve network performance (19). The data argument methods included horizontal flip, random Gaussian, random rotation, and vertical flip (15).
The pre-trained model weights were loaded into the Inception-v3 architecture. After data preparation, augmented data were used to train Inception-v3. Various model parameter values were adjusted. In training, parameters of the deep pretrained model were set as follows: a different number of layers were frozen at the beginning of the experiment [no freeze and fine-tuning to all transferred layers; freezing layers from 1 to n (n=1, 2, 3, 5, 7, and 9)]. Freezing the first three layers had the best diagnostic performance in our samples. Therefore, the weights of the first three layers were frozen, and the other parameters were fine-tuned. The optimizer was stochastic gradient descent; batch size was 64; learning-rate was 10-4; decay was 10-6; momentum was 0.95; epoch was 200; the loss function was binary cross-entropy. The training was performed on the graphics processing unit (GPU; NVIDIA, GTX1080Ti). A nonlinear operation was added in each convolution layer with the following activation function (20):
where φ denotes the feature map of the convolution layer, n is the number of convolution filters, x denotes input, w denotes filter, and b denotes bias. This design improved the computing power of the network and increased the depth and nonlinearity of the network. The feature map matrix was flattened into a column vector by a full connection layer. Finally, an activation function, such as sigmoid, was used to classify the output as the final result (Figure 3).
Statistical analysis
All statistical analyses were performed using SPSS for Windows version 24.0 (SPSS Inc., Chicago, IL, USA). Quantitative data were summarized on our dataset as the mean ± standard deviation (SD). Qualitative data were summarized as the total number of cases on our dataset and percentages. The independent sample t-test and chi-square test were used for different features. Interobserver agreement was assessed using Cohen’s kappa statistic (21). The receiver operating characteristic (ROC) curves were constructed to determine the best diagnostic accuracy based on the Youden index. The sensitivity, specificity, positive predictive value (PPV), and negative predictive value (NPV) were calculated for each method in the different groups. P<0.05 was considered to be statistically significant.
Results
A total of 129 patients were enrolled in the study, including 83 males and 46 females aged between 33 and 80 years of age. In this cohort, 21 patients were in the T2 stage (16.3%), 105 in the T3 stage (81.4%), and 3 patients were in the T4a stage (2.30%) (Table 1). Preoperative MRI revealed 227 targeted LNs that were isolated and the biggest in the LN drainage area of each patient, which could seek in the TME operation. After the operation, the pathologist and radiologist R1 cooperatively sought the targeted LNs corresponding to the MR images node by node. The postoperative pathological examination confirmed that 99 LNs were positive (43.6%), as shown in 319 T2W images (including axis, sagittal and coronal images), and 128 LNs were negative (56.4%), as shown in 325 T2W images (including axis, sagittal and coronal images). The SA diameters of positive and negative LNs were 4–22 and 3–9 mm, respectively.
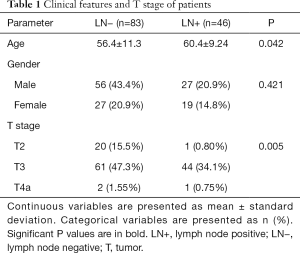
Full table
Radiologist R2 found that PPV, NPV, sensitivity, and specificity in Cohort 1 were 64.7%, 61.6%, 54.7%, and 70.9%, respectively, while the AUC and accuracy were 0.626 and 62.7%, respectively. In Cohort 2, PPV, NPV, sensitivity, and specificity were 62.7%, 66.1%, 68.2%, and 60.4%, respectively, while the AUC and accuracy were 0.643 and 64.3%, respectively. Radiologist R3 found that PPV, NPV, sensitivity, and specificity in Cohort 1 were 65.5%, 68.5%, 69.8%, and 64.1%, respectively, with an AUC and accuracy of 0.671 and 67.1%, respectively. In Cohort 2, PPV, NPV, sensitivity, and specificity were 64.6%, 69.4%, 72.3%, and 61.3%, respectively, with an AUC and accuracy of 0.670 and 66.9%, respectively. In the deep transfer learning method, PPV, NPV, sensitivity, and specificity were 95.2%, 95.3%, 95.3%, and 95.2%, respectively, and the AUC and accuracy were 0.994 and 95.7%, respectively (Table 2).
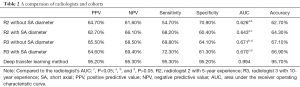
Full table
When the same radiologist analyzed data, the AUC showed no significant difference between the cohort with SA diameter measurements and the cohort without SA diameter measurements (P>0.05). However, a significant difference in the AUC was detected between the results of radiologists R2 and R3 when the SA diameter of LNs was known (P<0.05), but no significant difference was found when the SA diameter was unknown (P>0.05) (Figures 3,4).
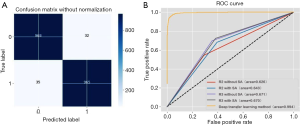
In Cohort 1, Cohen’s kappa coefficient value between the two radiologists was 0.359 [95% confidence interval (CI) 0.228 to 0.430], indicating fair agreement (P<0.01). In Cohort 2, Cohen’s kappa coefficient value between the two radiologists was 0.465 (95% CI, 0.396 to 0.534), indicating moderate agreement (P<0.01).
Discussion
Accurately determining the status of LNs in rectal cancer, including number and location, can guide treatment planning and provide reference indicators for patients’ prognosis (4,5). The existing diagnostic rates of LN metastasis in rectal cancer using multimodal examination methods such as CT/MRI/positron emission tomography–CT/EUS are not satisfactory (22–85%) (6-8).
This study used a node-by-node examination method, providing a gold standard for data analysis with high credibility. To our knowledge, LN involvement is a predominant factor in poor prognosis, but preoperative radiological LN staging is currently not satisfactory (2,3,6). There could be two reasons for this. First, it is not easy to match the imaging nodes with the histopathological nodes, with each bringing about unreliable results. Second, the LN size may be too small to distinguish internal details, leading to inconsistency and inaccuracy.
There are many AI methods for the diagnosis of LN status in rectal cancer. Tse et al. used an improved computer algorithm to quantitatively analyze MRI morphological features (including chemical shift artifact, relative mean signal intensity, signal heterogeneity, and nodal size) to predict LN status in rectal cancer. The predicting accuracy using combinations of these quantified features were 67–86% (22). Huang et al. used radiomics nomogram to improve the accuracy by 23% compared with traditional CT in the preoperative evaluation of LN status (5). However, these results were all lower than the deep transfer learning method used in this current study to identify LNs metastasis from rectal cancer. Deep learning has been widely recognized in various fields and has achieved good results in studying medical images. Kai et al. used a multiparametric deep learning model on MR images and achieved accurate automated detection and segmentation of meningioma tissue despite diverse scanners (23). Wang et al. engineered and trained a convolutional neural network to establish a deep learning model on MRI for liver tumor diagnosis (24). Given the small sample size of most medical imaging data, the method of deep transfer learning may be beneficial. This method was very effective in predicting LN status in colorectal cancer (25). We further applied this method of deep transfer learning to predicting LN metastasis in rectal cancer by optimizing the algorithm. In this study, good outcomes were achieved with deep transfer learning by freezing the first three layers. The PPV, NPV, sensitivity, and specificity were 95.2%, 95.3%, 95.3%, and 95.2%, respectively, and the AUC and accuracy were 0.994 and 95.7%, respectively. This was higher than the accuracy achieved by the radiologists (62.7–67.1%), meanwhile it also avoided the diagnosis inconsistency from different radiologists (kappa =0.359–0465). Therefore, using the deep transfer learning method can improve the accuracy of rectal cancer N staging and provide more reliable treatment guidance and prognosis.
The senior radiologist R3 compared with the junior radiologist R2 had better diagnostic performance, although there was no significant difference. This illustrates that although experience is important for clinical diagnosis, uniform standards can narrow the experience gap. Another notable result is that, although SA diameter enhanced consistency, the cohort with LN SA diameter measurements did not significantly improve AUC and accuracy compared to the cohort without SA diameter measurements. However, the sensitivity improved in Cohort 2, while the specificity declined for both R2 and R3. These results suggested that LN SA diameter helps positive LN diagnosis but may simultaneously increase false-negative results. Therefore, SA diameter is not a decisive factor in evaluating LN status and may lead to over-staging. In our research, the LN T2W screenshot images were analyzed alone without the SA diameter, and thus any influence from node size was avoided, and good results were obtained. Therefore, this study suggests that in rectal LN diagnosis, the internal details of the LNs, such as border, signal intensity, and morphology, should be used as the main criteria in MRI.
This study had some limitations. First, only the largest visible LN in one region on the MRI were enrolled, while LNs with SA diameter <3 mm were excluded. Second, all the LNs were from the mesorectal and rectal superior arterial regions, while pelvic sidewall LNs were not considered. Third, the data used for deep transfer learning analysis included only T2W images and T1- and diffusion-weighted images were not considered. Lastly, the study was conducted in a single-center study. Future studies should address these limitations to achieve improved results.
In conclusion, the deep transfer learning method is suitable for medical image analysis, especially in small samples. Most importantly, based on the algorithms used, deep transfer learning showed an encouraging performance in classifying rectal LNs, using detailed internal features alone without SA diameter. This method can influence the preoperative clinical staging and treatment decisions for patients with rectal cancer.
Acknowledgments
Funding: This study received funding from the National Natural Science Foundation of China (No. 61773134 and No. 81301297); the Applied Technology Research, the Development Foundation of Harbin City (No. 2016RAQXJ043); and the Harbin Medical University Cancer Hospital HaiYan Funds (No. JJZD2020-17).
Footnote
Conflicts of Interest: All authors have completed the ICMJE uniform disclosure form (available at http://dx.doi.org/10.21037/qims-20-525). The authors have no conflicts of interest to declare.
Ethical Statement: Ethics approval was obtained from the Harbin Medical University Cancer Hospital and written informed consent was obtained from each participant.
Open Access Statement: This is an Open Access article distributed in accordance with the Creative Commons Attribution-NonCommercial-NoDerivs 4.0 International License (CC BY-NC-ND 4.0), which permits the non-commercial replication and distribution of the article with the strict proviso that no changes or edits are made and the original work is properly cited (including links to both the formal publication through the relevant DOI and the license). See: https://creativecommons.org/licenses/by-nc-nd/4.0/.
References
- Siegel RL, Miller KD, Jemal A. Cancer statistics, 2018. CA Cancer J Clin 2018;68:7-30. [Crossref] [PubMed]
- Taylor FG, Quirke P, Heald RJ, Moran B, Blomqvist L, Swift I, Sebag-Montefiore DJ, Tekkis P, Brown G. group Ms. Preoperative high-resolution magnetic resonance imaging can identify good prognosis stage I, II, and III rectal cancer best managed by surgery alone: a prospective, multicenter, European study. Ann Surg 2011;253:711-9. [Crossref] [PubMed]
- Baek SJ, Kim SH, Kwak JM, Cho JS, Shin JW, Amar AH, Kim J. Selective use of preoperative chemoradiotherapy for T3 rectal cancer can be justified: analysis of local recurrence. World J Surg 2013;37:220-6. [Crossref] [PubMed]
- Matsuoka H, Nakamura A, Sugiyama M, Hachiya J, Atomi Y, Masaki T. MRI diagnosis of mesorectal lymph node metastasis in patients with rectal carcinoma. what is the optimal criterion? Anticancer Res 2004;24:4097-101. [PubMed]
- Huang YQ, Liang CH, He L, Tian J, Liang CS, Chen X, Ma ZL, Liu ZY. Development and Validation of a Radiomics Nomogram for Preoperative Prediction of Lymph Node Metastasis in Colorectal Cancer. J Clin Oncol 2016;34:2157-64. [Crossref] [PubMed]
- Lambregts DM, Beets GL, Maas M, Kessels AG, Bakers FC, Cappendijk VC, Engelen SM, Lahaye MJ, de Bruine AP, Lammering G, Leiner T, Verwoerd JL, Wildberger JE, Beets-Tan RG. Accuracy of gadofosveset-enhanced MRI for nodal staging and restaging in rectal cancer. Ann Surg 2011;253:539-45. [Crossref] [PubMed]
- Beets-Tan RG, Beets GL. Rectal cancer: review with emphasis on MR imaging. Radiology 2004;232:335-46. [Crossref] [PubMed]
- Park JS, Jang YJ, Choi GS, Park SY, Kim HJ, Kang H, Cho SH. Accuracy of preoperative MRI in predicting pathology stage in rectal cancers: node-for-node matched histopathology validation of MRI features. Dis Colon Rectum 2014;57:32-8. [Crossref] [PubMed]
- Kim JH, Beets GL, Kim MJ, Kessels AG, Beets-Tan RG. High-resolution MR imaging for nodal staging in rectal cancer: are there any criteria in addition to the size? Eur J Radiol 2004;52:78-83. [Crossref] [PubMed]
- Zhang R, Xu L, Wen X, Zhang J, Yang P, Zhang L, Xue X, Wang X, Huang Q, Guo C, Shi Y, Niu T, Chen F. A nomogram based on bi-regional radiomics features from multimodal magnetic resonance imaging for preoperative prediction of microvascular invasion in hepatocellular carcinoma. Quant Imaging Med Surg 2019;9:1503-15. [Crossref] [PubMed]
- Attanasio S, Forte SM, Restante G, Gabelloni M, Guglielmi G, Neri E. Artificial intelligence, radiomics and other horizons in body composition assessment. Quant Imaging Med Surg 2020;10:1650-60. [Crossref] [PubMed]
- Ma X, Shen F, Jia Y, Xia Y, Li Q, Lu J. MRI-based radiomics of rectal cancer: preoperative assessment of the pathological features. BMC Med Imaging 2019;19:86. [Crossref] [PubMed]
- Gröne J, Loch FN, Taupitz M, Schmidt C, Kreis ME. Accuracy of Various Lymph Node Staging Criteria in Rectal Cancer with Magnetic Resonance Imaging. J Gastrointest Surg 2018;22:146-53. [Crossref] [PubMed]
- MERCURY Study Group , Shihab OC, Taylor F, Bees N, Blake H, Jeyadevan N, Bleehen R, Blomqvist L, Creagh M, George C, Guthrie A, Massouh H, Peppercorn D, Moran BJ, Heald RJ, Quirke P, Tekkis P, Brown G. Relevance of magnetic resonance imaging-detected pelvic sidewall lymph node involvement in rectal cancer. Br J Surg 2011;98:1798-804. [Crossref] [PubMed]
- Kermany DS, Goldbaum M, Cai W, Valentim CCS, Liang H, Baxter SL, McKeown A, Yang G, Wu X, Yan F, Dong J, Prasadha MK, Pei J, Ting MYL, Zhu J, Li C, Hewett S, Dong J, Ziyar I, Shi A, Zhang R, Zheng L, Hou R, Shi W, Fu X, Duan Y, Huu VAN, Wen C, Zhang ED, Zhang CL, Li O, Wang X, Singer MA, Sun X, Xu J, Tafreshi A, Lewis MA, Xia H, Zhang K. Identifying Medical Diagnoses and Treatable Diseases by Image-Based Deep Learning. Cell 2018;172:1122-31.e9. [Crossref] [PubMed]
- Abidin AZ, Deng B. AM DS, Nagarajan MB, Coan P, Wismuller A. Deep transfer learning for characterizing chondrocyte patterns in phase contrast X-Ray computed tomography images of the human patellar cartilage. Comput Biol Med 2018;95:24-33. [Crossref] [PubMed]
- Brown G, Richards CJ, Bourne MW, Newcombe RG, Radcliffe AG, Dallimore NS, Williams GT. Morphologic predictors of lymph node status in rectal cancer with use of high-spatial-resolution MR imaging with histopathologic comparison. Radiology 2003;227:371-7. [Crossref] [PubMed]
- LeCun Y, Bengio Y, Hinton G. Deep learning. Nature 2015;521:436-44. [Crossref] [PubMed]
- Zhen X, Chen J, Zhong Z, Hrycushko B, Zhou L, Jiang S, Albuquerque K, Gu X. Deep convolutional neural network with transfer learning for rectum toxicity prediction in cervical cancer radiotherapy: a feasibility study. Phys Med Biol 2017;62:8246-63. [Crossref] [PubMed]
- Ferlay J, Soerjomataram I, Dikshit R, Eser S, Mathers C, Rebelo M, Parkin DM, Forman D, Bray F. Cancer incidence and mortality worldwide: sources, methods and major patterns in GLOBOCAN 2012. Int J Cancer 2015;136:E359-86. [Crossref] [PubMed]
- Litjens G, Kooi T, Bejnordi BE, Setio AAA, Ciompi F, Ghafoorian M, van der Laak J, van Ginneken B, Sanchez CI. A survey on deep learning in medical image analysis. Med Image Anal 2017;42:60-88. [Crossref] [PubMed]
- Tse DM, Joshi N, Anderson EM, Brady M, Gleeson FV. A computer-aided algorithm to quantitatively predict lymph node status on MRI in rectal cancer. Br J Radiol 2012;85:1272-8. [Crossref] [PubMed]
- Laukamp KR, Thiele F, Shakirin G, Zopfs D, Faymonville A, Timmer M, Maintz D, Perkuhn M, Borggrefe J. Fully automated detection and segmentation of meningiomas using deep learning on routine multiparametric MRI. Eur Radiol 2019;29:124-32. [Crossref] [PubMed]
- Wang CJ, Hamm CA, Savic LJ, Ferrante M, Schobert I, Schlachter T, Lin M, Weinreb JC, Duncan JS, Chapiro J, Letzen B. Deep learning for liver tumor diagnosis part II: convolutional neural network interpretation using radiologic imaging features. Eur Radiol 2019;29:3348-57. [Crossref] [PubMed]
- Li J, Wang P, Li YZ, Zhou Y, Liu XL, Luan K. Transfer Learning of Pre-Trained Inception-V3 Model for Colorectal Cancer Lymph Node Metastasis Classification. Proceedings of 2018 IEEE International Conference on Mechatronics and Automation August 5-8, Changchun, China.