Clinical prediction models of fractional flow reserve: an exploration of the current evidence and appraisal of model performance
Introduction
Since its introduction, fractional flow reserve (FFR) is a standard indicator of blood flow and has great value in assessing coronary lesions’ severity (1). Robust evidence has shown that FFR-guided percutaneous coronary intervention (PCI) provides improved outcomes for coronary artery disease (CAD) patients compared to strategies guided by invasive coronary angiography (2). However, interventional cardiologists are still largely dependent on visual estimations of lumen narrowing rather than physiological measurements in deciding whether to undertake revascularization (3). The underutilization of a physiological measurement may be related to the high costs of pressure wires, the discomfort induced by vasodilation, and the prolonged procedure time (4). However, given that PCI can be deferred for nearly 75% of FFR-interrogated lesions in a large registry (5), a risk-stratification tool is needed to facilitate lesion selection for invasive functional assessments.
This issue could be resolved by predicting FFR from clinical characteristics and angiographic parameters. Clinical prediction models (CPMs) are tools that combine multiple variables, such as history, routine diagnostic tests, or laboratory results, to predict a probability related to diagnosis or prognosis (6). Compared with other types of models based on geometric reconstruction and computational fluid dynamics, CPMs cannot give a specific value of FFR but can help stratify patients or lesions that are more likely to have an abnormal FFR (see Figure 1). These CPMs may diminish the need for pressure wires and thus reduce both medical expenditure and potential complications (7). Despite an increasing number of published FFR CPMs in recent years, there has been no attempt to evaluate recent advances on this topic comprehensively. Thus, clinicians may find it difficult to determine which model is ideal for practical use. This study sought to characterize available FFR CPMs, compare their included predictors and development methods, and assess their performance and methodological quality.
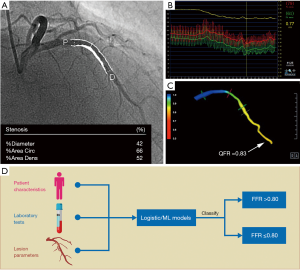
Methods
This review was conducted following the preferred reporting items for systemic reviews and meta-analyses (PRISMA) statement (8) and another recent guideline (9). It was prospectively registered at the International Prospective Register of Systematic Reviews (PROSPERO; URL: www.crd.york.ac.uk/PROSPERO; registration number: CRD42019125011). The population, intervention, comparison, outcome, timing, and setting (PICOTS) system (9) was used to formulate the review questions (see Table 1).
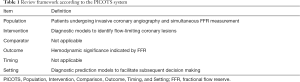
Full table
Search strategy and study selection
A comprehensive search for relevant publications was conducted in three databases [i.e., PubMed, EMBASE, and the Cochrane Central Register of Controlled Trials (CENTRAL)] from inception to April 14, 2019. A combination of MeSH/Emtree and keywords comprising FFR and CPMs were used with no language restrictions. Details of the search strategies are provided in the https://cdn.amegroups.cn/static/public/qims-20-1274-1.pdf. After searching for citations in the electronic databases, two authors (Drs. Zuo and Zhang) separately screened the titles and abstracts to compile a preliminary list. They resolved any disagreements by consensus. Studies were deemed relevant if they examined any FFR CPM regardless of whether it had been subject to external validation. Studies investigating the prognostic impact of CPMs were not included in this review. Only original articles were considered for inclusion. Studies were also excluded if they were based on physics (e.g., computational fluid dynamics) or did not develop a multivariable model. The references of the included studies were checked to identify any additional publications related to FFR CPMs.
Definitions
A prediction model study was defined as a study that developed a new CPM (10), evaluated the performance of a CPM (which was usually referred to as “validation”) (11), or did both. The purpose of validation is to assess a model’s performance by using development cohorts processed by resampling techniques, including bootstrapping and cross-validation (“internal validation”) (12), or by using other independent datasets as validation cohorts (“external validation”) (13). In a prediction model study, “random splitting” (i.e., an approach whereby a whole dataset is randomly divided into development and validation cohorts) may be undertaken (14); however, this approach cannot provide a reasonable estimate of a model’s performance due to the similarity between the two cohorts (15,16) and should be considered a form of “internal” rather than “external” validation (14). Conversely, “sample splitting” by time (or “temporal validation”) represents a better alternative to random splitting and can be considered an intermediate form of internal and external validation (14).
Data extraction and quality assessment
We extracted data regarding study characteristics and modeling methods, including predictors, model performance, and methodological factors. Predictors were defined as statistically independent variables or risk indicators that were combined to develop a CPM (14,17). Predictors can range from demographic variables, medical history, and physical signs to imaging results, laboratory tests, and other useful information for prediction. In the present study, the following three types of predictors were identified in the FFR CPMs: (I) patient characteristics (e.g., sex and age); (II) angiographic features; and (III) lesion-specific parameters. Lesion-specific parameters were defined as the target lesion’s quantitative variables (e.g., diameter stenosis), while angiographic features referred to other descriptions used in imaging, such as calcification and tortuosity. In our setting, model discrimination reflected the ability to distinguish ischemic lesions (FFR ≤0.80) from non-ischemic lesions (FFR >0.80). Discrimination was measured using the receiver operating characteristic curve; a higher area under the curve (AUC) indicated better discrimination. Generally, an AUC value of 0.50 indicates that the discrimination is no better than chance, a value of 0.60–0.75 indicates that the discrimination is helpful, and a value greater than 0.75 indicates that the discrimination is strong (18). Model calibration (or goodness of fit) is also an important indicator for evaluating a model and reflects the concordance between the expected risk and observed risk (i.e., whether a model can correctly estimate the actual risk) (18). A calibration plot or Hosmer-Lemeshow test usually assesses it; a small P value suggests poor calibration. A poorly calibrated model will underestimate or overestimate the occurrence of an event.
The methodological quality of the included studies was determined using the prediction model risk of the bias assessment tool (PROBAST) (19), which assessed predictive studies across the following four domains: (I) participants; (II) predictors; (III) outcome; and (IV) analysis. Studies can be classified as low, unclear, and have a high risk of bias or applicability. Bias was defined as any systematic error that was present in a prediction model study that would lead to distorted results and hamper validity (20). Applicability refers to the degree of agreement between the included studies and the review question regarding population, predictors, or outcomes (20). For example, concerns regarding applicability may arise if the participants in a study (e.g., patients in hospital settings) differ from those characterized in the review question (e.g., individuals in primary care settings). Two independent reviewers (Drs. Zuo and Zhang) were involved in the data extraction and critical appraisal of the studies, and a third investigator (Dr. Ma) was consulted if a discrepancy arose.
Data synthesis
Due to the inadequate number of validation studies on the same model (n≤3), a meta-analysis was not performed; rather, the published literature was qualitatively summarized to provide insights into FFR CPMs.
Results
A total of 1,296 citations were identified in the electronic databases based on our search strategy (see Figure 2). After removing duplicates, all the titles and abstracts were screened to exclude non-relevant publications, after which 34 full-text articles were assessed for eligibility. Subsequently, 18 articles were excluded because they constructed physics-based models (n=13), did not establish a CPM (n=3), or used coronary computed tomographic angiography (CCTA)-derived FFR (CT-FFR) or functionally significant CAD as a reference standard (n=2). Overall, 16 studies (7,21-35) were identified that described 11 different FFR CPMs.
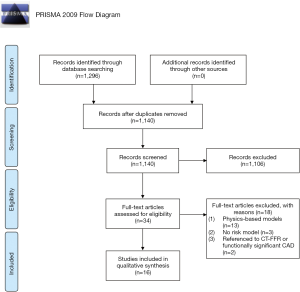
General characteristics and predictors
Most reports were conducted in Australia (23,25,32) (n=3), China (33,34) (n=2), South Korea (n=2) (31,35), and the United States (27,29) (n=2) (see Figure 3A). All CPMs were designed to identify lesion-specific ischemia assessed by FFR, including 5,625 and 1,495 lesions for derivation and validation, respectively (see Table 2). Nine studies focused exclusively on intermediate angiographic coronary stenosis. The mean age of the included participants ranged from 59 to 67 years, and the proportion of males varied from 60.6% to 88.0%. Three quarters of the studies were single-centered. The overall event (FFR ≤0.80) rate had a median of 37.1% (ranging from 20.7% to 68.0%). Details of the participant characteristics of all the studies are provided in the https://cdn.amegroups.cn/static/public/qims-20-1274-1.pdf.
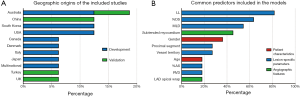
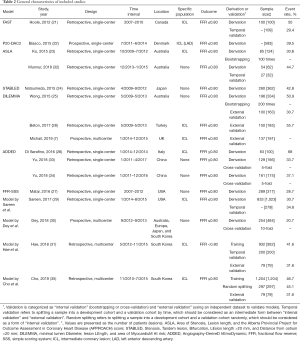
Full table
Among the 11 unique CPMs, 7 were developed by logistic regression analyses (21-25,27,29), 3 by machine-learning (ML) algorithms (30,31,35), and 1 by division (26). The number of final variables in each CPM varied greatly from 2 to 34. Quantitative angiography was applied in all 11 models, suggesting its essential role in predicting hemodynamic significance. Of these imaging-based models, 8 used invasive coronary angiography (21,22,24-27,29,35), 2 used non-invasive CCTA (23,30), and 1 used both (31). Lesion-specific parameters were used in all models, and >90% of models contained angiographic features. Conversely, patient characteristics (e.g., age and sex) were only considered in 27% of the models (27,30,35). The most commonly used predictors in the FFR CPMs were lesion length (82%), percent diameter stenosis (64%), and minimal lumen diameter (55%) (see Figure 3B). A complete list of the included variables is provided in the https://cdn.amegroups.cn/static/public/qims-20-1274-1.pdf
Model performance
Table 3 shows the performance metrics of the included models, including their discrimination and calibration. As Figure 4 shows, 15 studies reported a median AUC of 0.85 (ranging from 0.735 to 0.94), and 9 models (82%) showed strong discrimination as indicated by an AUC of ≥0.75. Only 3 models measured calibration using the Hosmer-Lemeshow test (25,26,29), calibration plots (25), or tables (29). There was no evidence that the performances of the FFR CPMs had been compared based on discrimination or calibration.
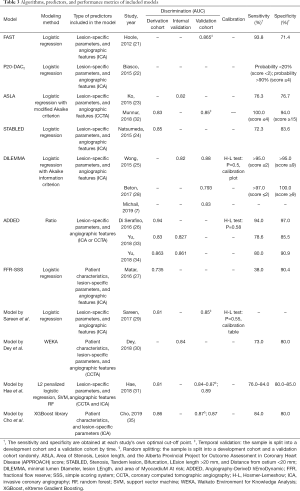
Full table
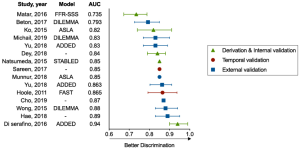
Among the five models undergoing external validation, a ML model developed by Hae et al. (31) had the highest discrimination (AUC: 0.89). The model incorporated a wide range of 34 predictors into its algorithm, including angiographic parameters, CCTA-based subtended myocardial volume, and demographic features. Compared with conventional logistic regression models, the three ML models (30,31,35) integrated more determinants (>10 for all) and required less time. The processing time of one ML model (30) was <30 seconds, whereas its logistic regression model counterpart (23) had a processing time of 102.6±37.5 seconds. The DILEMMA score (25) was the most tested score across all models and had a good degree of discrimination and calibration. Its reported AUCs ranged from 0.793 to 0.88 across three external cohorts from different races and the score was well calibrated as assessed by a calibration plot.
Quality assessment of study methods
In total, 13 studies were retrospective, and 9 models were derived from retrospective cohorts. There were three models (22,24,27) that had not been subject to any validation type, and three models (21,29,30) were only internally validated, limiting their generalizability. External validation occurred in five models, three of which were validated in different studies from their original reports (23,25,26). However, only one study (31) was conducted in compliance with the transparent reporting of a multivariable prediction model for individual prognosis or diagnosis (TRIPOD) statement (14). Notably, only two studies (25,29) presented the predicted probabilities across subgroups using a calibration plot or table, and none of the studies mentioned missing data.
Table 4 summarizes the results of our methodological evaluation, including the risk of bias and applicability. According to PROBAST, the risk of bias in participants’ domains, predictors, and outcomes was low in 14, 11, and 16 studies, respectively. However, all of the studies showed a high risk of bias in the domain of analysis, which was mainly driven by an inadequate number of events per variable, an inappropriate conversion of continuous variables, a univariable selection of predictors, and a lack of calibration plots (https://cdn.amegroups.cn/static/public/qims-20-1274-1.pdf). Conversely, all of the studies ranked “low concern for applicability”, suggesting a good match between the review question and included studies.
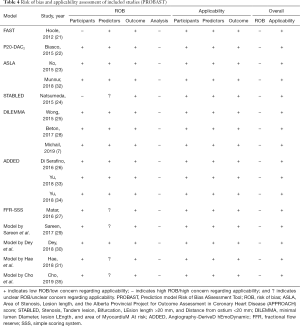
Full table
Discussion
To the best of our knowledge, this systematic review was the first to synthesize and appraise FFR CPMs; it is also the first study to provide current evidence that practitioners can use to assess the validity and quality of FFR CPMs. Of 16 studies, 11 models were identified, 5 of which had been externally validated. Discrimination ability was strong in the majority of models; however, calibration performance was not well documented. An application of PROBAST revealed that very few studies met the statistical analysis requirements but sound results were acquired in other domains. Thus, it may be premature to support a CPM for identifying hemodynamically significant coronary stenoses in clinical practice until further investigations with standardized methodologies and performance reports are conducted.
Under routine angiographic evaluation, coronary lesions may be misclassified and inappropriately managed, as a high frequency of discrepancy between angiography and FFR has been found (36). CPMs could be used to integrate the anatomical and physiological assessments of coronary stenosis (without the need for a pressure wire), reducing the overall expense and minimizing the potential complications associated with invasive procedures. Additionally, several virtual indexes have also been proposed to detect flow-limiting lesions based on computational fluid dynamics, including the quantitative flow ratio (QFR) and CT-FFR (37,38).
Compared with other emerging techniques, CPMs have several advantages. First, risk scores are easy to calculate. Consequently, CPMs are suitable for use in centers where pressure wires or virtual indexes are unavailable and will optimize which patients are referred for further evaluations and shorten the consultations’ length. Additionally, CPMs could be used as initial screening tools, which would enable unnecessary FFR measurements to be avoided. Indeed, a recent study showed that nearly half of intermediate lesions with a DILEMMA score of ≤2 or ≥9 could potentially have been deferred for invasive functional assessments (7). Second, CPMs can integrate more ischemia-related information than a three-dimensional vessel reconstruction, including demographic characteristics, physical examinations, laboratory tests, and imaging. Dynamics and other factors influence the physiological severity of coronary stenosis; thus, a combination of virtual indexes and CPMs would provide a more accurate and comprehensive assessment of lesions than a single-method approach (33). Despite the advantages mentioned above, current CPMs still have some limitations. They can only distinguish ischemic from non-ischemic lesions, and they cannot simulate the FFR value. Further, the included studies rarely reported the time needed to complete the prediction, which raises another concern that should be addressed before they are used in practice.
In the future, a simultaneous risk calculation with coronary angiography in the catheterization laboratory could be developed to determine whether an intervention should proceed. Compared to conventional logistic regression, ML has been recognized as a promising approach for predictor identification and big-data processing, and thus will likely play an important role in the improvement of CPMs (39). Notably, Dey et al. (30) conducted a study in which ML integrated feature selection, model building, and cross-validation. This algorithm can be executed on a standard personal computer within 30 seconds and exhibited a higher AUC than logistic regression (0.84 vs. 0.78). Deep neural networks, an objective and automated method for feature extraction, could help to simplify the process of image identification and reduce the potential biases that arise from manual quantifications (40), which are non-inferior to experienced observers in the analysis of cardiovascular magnetic resonance (41). Similarly, the amount of subtended myocardial volume, which is a significant predictor of flow-limiting lesions, can be roughly estimated by angiographic indexes (23,25,26); however, ML provides a feasible approach to measure its value accurately (31).
Notably, uncertainty remains about models’ performance, especially when used for distinct populations based on derivation cohorts. External validation is necessary, as internal validation insufficiently reflects models’ actual performance in a relatively small cohort (42). However, only a small proportion of the FFR CPMs underwent external validation, which raises concerns about their generalizability. Despite satisfactory discrimination, most FFR CPMs were originally designed for intermediate-grade lesions, limiting the scope of their application. After the primary study, further efforts should be undertaken to investigate their prognostic effects and analyze their cost-effectiveness. Apart from discrimination, the net clinical benefits could help us identify the models that best support decision making and lead to better outcomes (43).
There were four major causes of risk of bias in the included studies: (I) an inadequate number of participants with the outcome; (II) the univariate selection of predictors; (III) the inappropriate categorization of continuous predictors; (IV) an absence of calibration. The first two limitations may exacerbate concerns about optimistic model performance, particularly those that did not undergo external validation. Despite no consensus on the reasonable number of events per variable, we adopted a cut-off point of >20 and >200 for logistic regression and ML, respectively (20,44). The number of outcomes should be at least 100 for validation studies (45), but some studies failed to meet the minimum criterion. We also noted that univariate analyses for screening candidate predictors were used in more than half of the included development studies. This could lead to biases, as confounding factors might hide some significant predictors in the derivation dataset (46). Our review systematically summarized the most commonly used predictors in FFR CPMs. Consequently, future studies may benefit from selecting these directly rather than by univariate screening. Further, statistical power is impaired when a continuous predictor is categorized without using predefined or widely accepted cut-off points (47), which are often seen in risk scores for simplicity. This problem could be solved with automatic calculation tools, such as web calculators and mobile apps. The final shortcoming deserves more attention in subsequent validations. Some studies measured calibration based on goodness-of-fit tests; however, a P value alone does not provide any useful information regarding the extent of miscalibration (20). This issue arose because some of the early work was conducted before the publication of the TRIPOD statement and because less attention was paid to calibration than discrimination.
Limitations
This work had several limitations. First, 81% of the studies were retrospective and may be inherently influenced by confounding factors. Second, heterogeneity across the studies and insufficient validation meant that model performance could not be quantitatively synthesized; thus, a narrative description of FFR CPMs was produced that was largely based on their characteristics and methodological quality. Third, caution should be adopted in interpreting the comparative performance of models, as no existing study has validated them within the same cohort. Fourth, most of the included studies only focused on discrimination and did not combine it with calibration in evaluating overall model performance. Thus, the current evidence on FFR CPMs is still too weak to draw strong inferences about their actual effects.
Conclusions
In conclusion, published FFR CPMs showed good diagnostic performance in detecting hemodynamically significant coronary stenoses and may reduce the need for pressure wires. Many FFR CPMs have been developed; however, less than half of these have been externally validated, and their calibration has rarely been reported. ML techniques may have advantages in terms of accuracy and speed that could prove particularly important in clinical use. Future studies should seek to examine the external validity of existing models and optimize model performance rather than developing new models.
Acknowledgments
Funding: This work was supported by the Jiangsu Provincial Key Research and Development Program (BE2016785), the Jiangsu Provincial Key Medical Discipline (ZDXKA2016023), and the National Natural Science Foundation of China (81870213).
Footnote
Conflicts of Interest: All of the authors have completed the ICMJE uniform disclosure form (available at http://dx.doi.org/10.21037/qims-20-1274). The authors have no conflicts of interest to declare.
Ethical Statement: This article did not include any information about patients; thus, ethical approval and informed consent were not needed.
Open Access Statement: This is an Open Access article distributed in accordance with the Creative Commons Attribution-NonCommercial-NoDerivs 4.0 International License (CC BY-NC-ND 4.0), which permits the non-commercial replication and distribution of the article with the strict proviso that no changes or edits are made and the original work is properly cited (including links to both the formal publication through the relevant DOI and the license). See: https://creativecommons.org/licenses/by-nc-nd/4.0/.
References
- Pijls NH, van Son JA, Kirkeeide RL, De Bruyne B, Gould KL. Experimental basis of determining maximum coronary, myocardial, and collateral blood flow by pressure measurements for assessing functional stenosis severity before and after percutaneous transluminal coronary angioplasty. Circulation 1993;87:1354-67. [Crossref] [PubMed]
- Tonino PAL, De Bruyne B, Pijls NHJ, Siebert U, Ikeno F, van't Veer M, Klauss V, Manoharan G, Engstrøm T, Oldroyd KG, Ver Lee PN, MacCarthy PA, Fearon WFFAME Study Investigators. Fractional flow reserve versus angiography for guiding percutaneous coronary intervention. N Engl J Med 2009;360:213-24. [Crossref] [PubMed]
- Dattilo PB, Prasad A, Honeycutt E, Wang TY, Messenger JC. Contemporary patterns of fractional flow reserve and intravascular ultrasound use among patients undergoing percutaneous coronary intervention in the United States. J Am Coll Cardiol 2012;60:2337-9. [Crossref] [PubMed]
- Götberg M, Cook CM, Sen S, Nijjer S, Escaned J, Davies JE. The evolving future of instantaneous wave-free ratio and fractional flow reserve. J Am Coll Cardiol 2017;70:1379-402. [Crossref] [PubMed]
- Ahn JM, Park DW, Shin ES, Koo BK, Nam CW, Doh JH, Kim JH, Chae IH, Yoon JH, Her SH, Seung KB, Chung WY, Yoo SY, Lee JB, Choi SW, Park K, Hong TJ, Lee SY, Han M, Lee PH, Kang SJ, Lee SW, Kim YH, Lee CW, Park SW, Park SJ. IRIS-FFR Investigators. Fractional flow reserve and cardiac events in coronary artery disease: data from a prospective IRIS-FFR registry (Interventional Cardiology Research Incooperation Society Fractional Flow Reserve). Circulation 2017;135:2241-51. [Crossref] [PubMed]
- Laupacis A, Sekar N, Stiell IG. Clinical prediction rules: a review and suggested modifications of methodological standards. JAMA 1997;277:488-94. [Crossref] [PubMed]
- Michail M, Dehbi HM, Nerlekar N, Davies JE, Sharp ASP, Talwar S, Cameron JD, Brown AJ, Wong DT, Mathur A, Hughes AD, Narayan O. Application of the DILEMMA score to improve lesion selection for invasive physiological assessment. Catheter Cardiovasc Interv 2019;94:E96-103. [Crossref] [PubMed]
- Moher D, Liberati A, Tetzlaff J, Altman DG. PRISMA Group. Preferred reporting items for systematic reviews and meta-analyses: the PRISMA statement. Ann Intern Med 2009;151:264-9. [Crossref] [PubMed]
- Debray TP, Damen JA, Snell KI, Ensor J, Hooft L, Reitsma JB, Riley RD, Moons KG. A guide to systematic review and meta-analysis of prediction model performance. BMJ 2017;356:i6460. [Crossref] [PubMed]
- Royston P, Moons KG, Altman DG, Vergouwe Y. Prognosis and prognostic research: Developing a prognostic model. BMJ 2009;338:b604. [Crossref] [PubMed]
- Altman DG, Vergouwe Y, Royston P, Moons KG. Prognosis and prognostic research: validating a prognostic model. BMJ 2009;338:b605. [Crossref] [PubMed]
- Harrell FE Jr, Lee KL, Mark DB. Multivariable prognostic models: issues in developing models, evaluating assumptions and adequacy, and measuring and reducing errors. Stat Med 1996;15:361-87. [Crossref] [PubMed]
- Moons KG, Kengne AP, Grobbee DE, Royston P, Vergouwe Y, Altman DG, Woodward M. Risk prediction models: II. External validation, model updating, and impact assessment. Heart 2012;98:691-8. [Crossref] [PubMed]
- Moons KG, Altman DG, Reitsma JB, Ioannidis JP, Macaskill P, Steyerberg EW, Vickers AJ, Ransohoff DF, Collins GS. Transparent Reporting of a multivariable prediction model for Individual Prognosis or Diagnosis (TRIPOD): explanation and elaboration. Ann Intern Med 2015;162:W1-73. [Crossref] [PubMed]
- Steyerberg EW, Bleeker SE, Moll HA, Grobbee DE, Moons KG. Internal and external validation of predictive models: a simulation study of bias and precision in small samples. J Clin Epidemiol 2003;56:441-7. [Crossref] [PubMed]
- Steyerberg EW, Harrell FE Jr, Borsboom GJ, Eijkemans MJ, Vergouwe Y, Habbema JD. Internal validation of predictive models: efficiency of some procedures for logistic regression analysis. J Clin Epidemiol 2001;54:774-81. [Crossref] [PubMed]
- Wasson JH, Sox HC, Neff RK, Goldman L. Clinical prediction rules. Applications and methodological standards. N Engl J Med 1985;313:793-9. [Crossref] [PubMed]
- Alba AC, Agoritsas T, Walsh M, Hanna S, Iorio A, Devereaux PJ, McGinn T, Guyatt G. Discrimination and calibration of clinical prediction models: users' guides to the medical literature. JAMA 2017;318:1377-84. [Crossref] [PubMed]
- Wolff RF, Moons KGM, Riley RD, Whiting PF, Westwood M, Collins GS, Reitsma JB, Kleijnen J, Mallett S. PROBAST Group. PROBAST: a tool to assess the risk of bias and applicability of prediction model studies. Ann Intern Med 2019;170:51-8. [Crossref] [PubMed]
- Moons KGM, Wolff RF, Riley RD, Whiting PF, Westwood M, Collins GS, Reitsma JB, Kleijnen J, Mallett S. PROBAST: A tool to assess risk of bias and applicability of prediction model studies: explanation and elaboration. Ann Intern Med 2019;170:W1-33. [Crossref] [PubMed]
- Hoole SP, Seddon MD, Poulter RS, Starovoytov A, Wood DA, Saw J. Development and validation of the fractional flow reserve (FFR) angiographic scoring tool (FAST) to improve the angiographic grading and selection of intermediate lesions that require FFR assessment. Coron Artery Dis 2012;23:45-50. [Crossref] [PubMed]
- Biasco L, Pedersen F, Lønborg J, Holmvang L, Helqvist S, Saunamäki K, Kelbaek H, Clemmensen P, Olivecrona GK, Jørgensen E, Engstrøm T, De Backer O. Angiographic characteristics of intermediate stenosis of the left anterior descending artery for determination of lesion significance as identified by fractional flow reserve. Am J Cardiol 2015;115:1475-80. [Crossref] [PubMed]
- Ko BS, Wong DT, Cameron JD, Leong DP, Soh S, Nerlekar N, Meredith IT, Seneviratne SK. The ASLA Score: A CT angiographic index to predict functionally significant coronary stenoses in lesions with intermediate severity—diagnostic accuracy. Radiology 2015;276:91-101. [Crossref] [PubMed]
- Natsumeda M, Nakazawa G, Murakami T, Torii S, Ijichi T, Ohno Y, Masuda N, Shinozaki N, Ogata N, Yoshimachi F, Ikari Y. Coronary angiographic characteristics that influence fractional flow reserve. Circ J 2015;79:802-7. [Crossref] [PubMed]
- Wong DT, Narayan O, Ko BS, Leong DP, Seneviratne S, Potter EL, Cameron JD, Meredith IT, Malaiapan Y. A novel coronary angiography index (DILEMMA score) for prediction of functionally significant coronary artery stenoses assessed by fractional flow reserve: a novel coronary angiography index. Am Heart J 2015;169:564-71.e4. [Crossref] [PubMed]
- Di Serafino L, Scognamiglio G, Turturo M, Esposito G, Savastano R, Lanzone S, Trimarco B, D’Agostino C. FFR prediction model based on conventional quantitative coronary angiography and the amount of myocardium subtended by an intermediate coronary artery stenosis. Int J Cardiol 2016;223:340-4. [Crossref] [PubMed]
- Matar FA, Falasiri S, Glover CB, Khaliq A, Leung CC, Mroue J, Ebra G. When should fractional flow reserve be performed to assess the significance of borderline coronary artery lesions: derivation of a simplified scoring system. Int J Cardiol 2016;222:606-10. [Crossref] [PubMed]
- Beton O, Kaya H, Turgut OO, Yılmaz MB. Prediction of fractional flow reserve with angiographic DILEMMA score. Anatol J Cardiol 2017;17:285-92. [PubMed]
- Sareen N, Baber U, Kezbor S, Sayseng S, Aquino M, Mehran R, Sweeny J, Barman N, Kini A, Sharma SK. Clinical and angiographic predictors of haemodynamically significant angiographic lesions: development and validation of a risk score to predict positive fractional flow reserve. EuroIntervention 2017;12:e2228-35. [Crossref] [PubMed]
- Dey D, Gaur S, Ovrehus KA, Slomka PJ, Betancur J, Goeller M, Hell MM, Gransar H, Berman DS, Achenbach S, Botker HE, Jensen JM, Lassen JF, Norgaard BL. Integrated prediction of lesion-specific ischaemia from quantitative coronary CT angiography using machine learning: a multicentre study. Eur Radiol 2018;28:2655-64. [Crossref] [PubMed]
- Hae H, Kang SJ, Kim WJ, Choi SY, Lee JG, Bae Y, Cho H, Yang DH, Kang JW, Lim TH, Lee CH, Kang DY, Lee PH, Ahn JM, Park DW, Lee SW, Kim YH, Lee CW, Park SW, Park SJ. Machine learning assessment of myocardial ischemia using angiography: development and retrospective validation. PLoS Med 2018;15:e1002693 [Crossref] [PubMed]
- Munnur RK, Cameron JD, McCormick LM, Psaltis PJ, Nerlekar N, Ko BSH, Meredith IT, Seneviratne S, Wong DTL. Diagnostic accuracy of ASLA score (a novel CT angiographic index) and aggregate plaque volume in the assessment of functional significance of coronary stenosis. Int J Cardiol 2018;270:343-8. [Crossref] [PubMed]
- Yu M, Lu Z, Li W, Wei M, Yan J, Zhang J. CT morphological index provides incremental value to machine learning based CT-FFR for predicting hemodynamically significant coronary stenosis. Int J Cardiol 2018;265:256-61. [Crossref] [PubMed]
- Yu M, Zhao Y, Li W, Lu Z, Wei M, Zhou W, Zhang J. Relationship of the Duke jeopardy score combined with minimal lumen diameter as assessed by computed tomography angiography to the hemodynamic relevance of coronary artery stenosis. J Cardiovasc Comput Tomogr 2018;12:247-54. [Crossref] [PubMed]
- Cho H, Lee JG, Kang SJ, Kim WJ, Choi SY, Ko J, Min HS, Choi GH, Kang DY, Lee PH, Ahn JM, Park DW, Lee SW, Kim YH, Lee CW, Park SW, Park SJ. Angiography‐based machine learning for predicting fractional flow reserve in intermediate coronary artery lesions. J Am Heart Assoc 2019;8:e011685 [Crossref] [PubMed]
- Park SJ, Kang SJ, Ahn JM, Shim EB, Kim YT, Yun SC, Song H, Lee JY, Kim WJ, Park DW, Lee SW, Kim YH, Lee CW, Mintz GS, Park SW. Visual-functional mismatch between coronary angiography and fractional flow reserve. JACC Cardiovasc Interv 2012;5:1029-36. [Crossref] [PubMed]
- Tu S, Westra J, Yang J, von Birgelen C, Ferrara A, Pellicano M, Nef H, Tebaldi M, Murasato Y, Lansky A, Barbato E, van der Heijden LC, Reiber JH, Holm NR, Wijns WFAVOR Pilot Trial Study Group. Diagnostic accuracy of fast computational approaches to derive fractional flow reserve from diagnostic coronary angiography: the international multicenter FAVOR pilot study. JACC Cardiovasc Interv 2016;9:2024-35. [Crossref] [PubMed]
- Taylor CA, Fonte TA, Min JK. Computational fluid dynamics applied to cardiac computed tomography for noninvasive quantification of fractional flow reserve: scientific basis. J Am Coll Cardiol 2013;61:2233-41. [Crossref] [PubMed]
- Goldstein BA, Navar AM, Carter RE. Moving beyond regression techniques in cardiovascular risk prediction: applying machine learning to address analytic challenges. Eur Heart J 2017;38:1805-14. [PubMed]
- Henglin M, Stein G, Hushcha P V, Snoek J, Wiltschko AB, Cheng S. Machine learning approaches in cardiovascular imaging. Circ Cardiovasc Imaging 2017;10:e005614 [Crossref] [PubMed]
- Bai W, Sinclair M, Tarroni G, Oktay O, Rajchl M, Vaillant G, Lee AM, Aung N, Lukaschuk E, Sanghvi MM, Zemrak F, Fung K, Paiva JM, Carapella V, Kim YJ, Suzuki H, Kainz B, Matthews PM, Petersen SE, Piechnik SK, Neubauer S, Glocker B, Rueckert D. Automated cardiovascular magnetic resonance image analysis with fully convolutional networks. J Cardiovasc Magn Reson 2018;20:65. [Crossref] [PubMed]
- Bleeker SE, Moll HA, Steyerberg EW, Donders ART, Derksen-Lubsen G, Grobbee DE, Moons KG. External validation is necessary in prediction research: a clinical example. J Clin Epidemiol 2003;56:826-32. [Crossref] [PubMed]
- Van Calster B, Wynants L, Verbeek JFM, Verbakel JY, Christodoulou E, Vickers AJ, Roobol MJ, Steyerberg EW. Reporting and interpreting decision curve analysis: a guide for investigators. Eur Urol 2018;74:796-804. [Crossref] [PubMed]
- van der Ploeg T, Austin PC, Steyerberg EW. Modern modelling techniques are data hungry: a simulation study for predicting dichotomous endpoints. BMC Med Res Methodol 2014;14:137. [Crossref] [PubMed]
- Collins GS, Ogundimu EO, Altman DG. Sample size considerations for the external validation of a multivariable prognostic model: a resampling study. Stat Med 2016;35:214-6. [Crossref] [PubMed]
- Sun GW, Shook TL, Kay GL. Inappropriate use of bivariable analysis to screen risk factors for use in multivariable analysis. J Clin Epidemiol 1996;49:907-16. [Crossref] [PubMed]
- MacCallum RC, Zhang S, Preacher KJ, Rucker DD. On the practice of dichotomization of quantitative variables. Psychol Methods 2002;7:19-40. [Crossref] [PubMed]