Reduction of missed thoracic findings in emergency whole-body computed tomography using artificial intelligence assistance
IntroductionOther Section
Major trauma can cause prolonged disability or death and remains the leading cause of death under the age of 45 (1,2). The interval from the time of injury until computed tomography (CT) is an established key performance indicator that evaluates the clinical care of major trauma patients (3). A minimum standard of 60 minutes (ideally 30 minutes) for image acquisition as well as radiological reporting is recommended (3). In an acute trauma setting, there are many patients receiving a (whole-body) CT scan for the first time. Therefore, there is a high potential to detect relevant secondary image findings that have been unknown so far and might need follow-up control or treatment. Since radiologists must report time-critically in an emergency setting, there is a relevant risk of under-detection with regard to secondary findings. Indeed, other studies have demonstrated relevant discrepancies between initial reports and supplementary senior reviews of emergency CTs (4,5). To our knowledge, the added value of any software-based automatic review for the detection of secondary findings in emergency CTs has not been reported so far.
Advances in artificial intelligence (AI) techniques raise the question whether the additional semi-automated image analysis (AI-assisted reporting) might reduce the number of missed secondary findings in a time-efficient fashion. Recent studies demonstrated the general ability of AI to perform image analysis on the level of healthcare specialists (6-9). Nevertheless, available AI algorithms usually have a very narrow clinical focus and are often solely evaluated by quantifying the achieved diagnostic metrics compared with those of radiologists. In the emergency setting, promising results could exemplarily be shown for the CT detection of intracranial hemorrhage (10,11), ischemic strokes (12) or vertebral fractures (13).
Here, we clinically evaluate a software platform (including several prototype AI algorithms not yet commercially available and limited to chest CT analysis) that aims to detect the following secondary thoracic findings: lung lesions, cardiomegaly, coronary plaques, aortic aneurysms and vertebral fractures. We hypothesize a relevant number of initially missed secondary findings that would have been detected in an AI-assisted reading setting.
MethodsOther Section
The study was approved by institutional ethics board of LMU University Hospital (approval number 18-399); individual consent was waived due to retrospective observational study character and re-analysis of anyway performed and clinically indicated CT imaging.
Patient population
We retrospectively identified 105 patients who received a whole-body CT scan in our emergency department (shock-room) from January to November 2019. Patients have been consecutively included. No exclusion criteria have been applied. Siemens SOMATOM Force CT scanner was used; the protocol included a native skull CT, a whole-body CT (skull base until proximal thigh) with arterial contrast media enhancement (90 mL Imeron® 400 MCT, flow 5 mL/s, 5 s delay after the detection of 100 Hounsfield units (HU) in the ascending aorta, detailed scanning parameters, see Table 1) and an additional abdominal scan with venous contrast media enhancement (additional delay of 40 s after the previous scan had been finished). The raw DICOMs (one DICOM per slice) of the thoracic arterial contrast medium phase (slice thickness 0.75 mm, soft tissue kernel Br36d) were analyzed by a set of AI algorithms (abdominal slices have been cropped to reduce the processing time, no other preprocessing was performed). Graphically illustrated results have been returned to our Picture Archiving and Communication System (PACS) (see Figure 1). The separately acquired native skull and venous phase abdominal scans (included in our standard emergency whole-body CT protocol) were not included in our analysis, which is solely focused on thoracic pathologies.
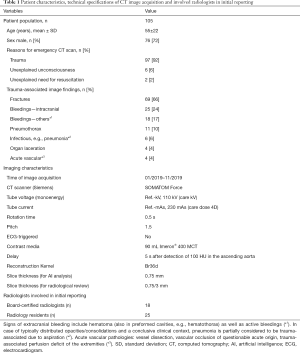
Full table
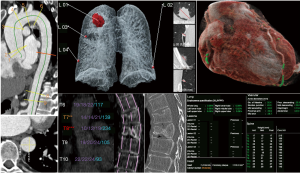
Age (55±22 years), gender (72% male), reasons for emergency CT scan (93% trauma) and major image findings were recorded (see Table 1). As consecutively extracted from clinical routine, image data also includes examinations of limited quality [e.g., pulsation artefacts in non-electrocardiogram (ECG)-gated CTs or respiratory artifacts]. Eighteen out of 105 CT scans (17%) have been originally reported by a board-certified radiologist alone, the other 87 CT scans (83%) have been commonly reported by a radiology resident and a board-certified radiologist. Twenty-five different radiology residents and 18 different board-certified radiologists have been involved, see Table 1.
AI algorithm modules
AI-Rad Companion Chest CT (Siemens Healthineers, Erlangen, Germany) is a software platform including AI-algorithm modules for the detection of different abnormalities in the thorax. An on-premise prototype not yet commercially available has been used in this work. The prototype was trained with hundreds (heart segmentation, thoracic vertebrae analysis) up to several thousands (lung lesion analysis, lung lobe segmentation) datasets, for each algorithm of at least five different clinical sites, up to 50% public data and at least three vendors. Training data was native as well as contrast enhanced (coronary plaque detection and heart segmentation: native only) and reconstructed using filtered backprojection and iterative reconstruction using soft to hard reconstruction kernels. The training data had no particular focus on emergency CTs.
The processing time was measured as 5–10 minutes per CT, including the analysis of the following modules (corresponding network architectures are provided in a Supplemental file).
Heart segmentation and coronary plaque detection
Heart segmentation is performed using a deep U-shaped network (14) consisting of four convolutions and down-sampling steps, followed by four similar up-sampling layers. The heart segmentation mask is used to compute the heart volume and to define a region of interest (ROI) for the coronary calcium detection. Within the ROI an initial set of voxels as candidates for potentially calcified regions is identified by HU-thresholding. For each candidate voxel an image patch surrounding the voxel is fed into a deep learning-based classification algorithm with two components: A convolutional neural network which takes the image patch and a precomputed coronary territory map as inputs, and a dense neural network which operates on the coordinates of the voxel. Combining the features from both components allows a final prediction as to whether the voxel belongs to the coronary arteries. The total volume of the detected coronary calcium is reported.
Aorta analysis
Aortic landmarks (aortic root/aortic arch center/brachiocephalic artery bifurcation/left common carotid artery/left subclavian artery/celiac trunk) are detected automatically based on deep reinforcement learning (15). Within the ROI the segmentation is performed using an adversarial deep image-to-image network (DI2IN) in a symmetric convolutional encoder-decoder architecture (16). Based on the aortic mask, a centerline model is used to generate the aortic centerline which is used in combination with aortic landmarks to identify nine measurement planes according to the guidelines of the American Heart Association (AHA) (17). In each measurement plane, multiple diameters are generated by computing intersections of rays starting from the centerline. Based on these diameters, the maximum in-plane diameter and its perpendicular equivalent are reported. The maximum diameters are used for threshold-based categorization for different anatomic landmark positions derived from the AHA guidelines (threshold #1 for diameters exceeding the population’s mean by more than two standard deviations, threshold #2 for diameters exceeding the population’s mean by more than 50%, definition of landmark positions including population’s mean as described in the following subsection “aorta analysis”).
Lung lobe segmentation (for heart/lung volume ratio quantification)
This algorithm computes segmentation masks of the five lung lobes by taking the entire 3D CT volumes as input and, based on thereon, indicates probability maps indicating how likely voxels belongs to each lung lobe. It uses a DI2IN in a symmetric convolutional encoder-decoder architecture (16).
Lung lesions analysis
Lung nodule detection is performed in a two-step approach: nodule candidate generation (NCG) and false positive (FP) reduction. The NCG is a 3D region proposal network based on faster-R-CNN (18) that indicates a few suspicious regions called “nodule candidates” and assigns probability scores. The FP reduction module consisting of several Res-Net (19) units further evaluates the likelihood for the nodule candidate to be a true nodule or a FP one by updating the scores generated by the NCG module. The final decision is made by considering the weighted sum of the scores generated by NCG and FP reduction modules. After detection, nodules are algorithm-based segmented and 2D diameter and 3D volume are provided. The algorithm does not provide a confidence assessment regarding possible malignancy.
Thoracic vertebrae analysis
The 12 thoracic vertebrae are localized and labeled using an algorithm based on wavelet features, AdaBoost, and local geometry constraints (20). The vertebra centers are used to determine regions of interest for the subsequent vertebral segmentation. Segmentation is performed within the ROI using a DI2IN in a symmetric convolutional encoder-decoder architecture (16). The sagittal midplane is extracted from the segmentation masks and within this plane height measurements are performed at anterior, medial and posterior location. Afterwards, the heights are compared with the values of the neighboring vertebras using the Genant severity grading method (21). Although originally developed on chest radiographs, the Genant method is also widely used in CT imaging (22). Height reductions exceeding 20% of the adjacent vertebra’s heights are considered as suspicious for fracture. The algorithm does not distinguish between preexisting and new fractures.
Image analysis, results quantification & statistics
Initial radiologists’ reports and AI algorithm results have been screened for discrepancies focusing on the above-mentioned secondary findings. AI algorithm findings have been reviewed by an experienced radiology resident (3 years of experience in thoracic imaging with >5,500 reported CT scans; ambiguous cases were discussed with a board-certified radiologist). AI-detected heart and coronary plaque volumes of subgroups differing in whether coronary plaques or cardiomegaly was manually suspected by radiologists have been statistically compared by Student’s t-test. The discriminative power of the corresponding algorithms has been analyzed by receiver operating curve (ROC) analysis with radiologists’ evaluation serving as reference standard. ROC operating points have been approximated to the maximum sum of sensitivity and specificity (Youden’s statistics) and corresponding metrics [e.g., sensitivity, specificity, FPs, positive predictive value (PPV)] have been quantified (23). The software GraphPad Prism was used for graphic illustrations and statistical analysis.
Heart volume
Heart volume was classified according to the initial radiologists’ reports as follows: “cardiomegaly” vs. “borderline” vs. “initially not reported”. Initially not reported cardiac sizes have been re-classified by the above-mentioned radiology resident. Correlation and ROC analysis was performed based on the AI-calculated total heart volume and the radiologists’ assessment as a reference standard (“borderline” and “cardiomegaly” have been pooled and considered as pathologic yielding in a necessary binary reference standard).
Coronary plaques
Coronary plaques were classified according to the initial radiologists’ reports as follows: “reported” vs. “not reported”. AI-detected plaques have been reviewed for plausibility. Correlation and ROC analysis were based on the AI-detected plaque volume.
Aorta analysis
Aorta analysis was based on diameter quantification at nine landmark positions according to the AHA guidelines with the corresponding population characteristics (mean ± standard variation) (20): #1—sinuses of Vasalva (37.7±3.8 mm); #2—sinotubular junction (33.2±3.7 mm); #3—mid ascending aorta (28.6±3.6 mm); #4—proximal aortic arch (28.2±3.5 mm); #5—mic aortic arch (27.7±3.3 mm); #6—proximal descending aorta (27.3±3.2 mm); #7—mid descending aorta (26.9±3.1 mm), #8—aorta at diaphragm (25.6±3.4 mm), #9—aorta at celiac origin (26.1±3 mm). AI-detected diameters exceeding the population’s mean by more than two standard variations have been radiologically reviewed for plausibility and discrepancies compared with the original reports have been assessed.
Lung lesions
Lung lesions were classified as follows: “solely detected by radiologists” vs. “detected by radiologists and AI” vs. “solely detected by AI”. Hereby, only the three largest lesions detected by the AI algorithm were considered. Lesions that have been only detected by the AI have been radiologically reviewed for plausibility and subclassified as follows: “granuloma” vs. “perifissural lymph nodes (PFNL)” vs. “trauma-associated” (including locally restricted ventilation, lung contusion, infiltrates e.g., after aspiration) vs. “scarred/post-inflammatory” vs. “unclear/in recommendation of control” (>6 mm according to Fleischner’s criteria assuming a low-risk profile of the patients without any history of malignoma).
Vertebral fractures
Vertebral fractures were classified as follows: “solely detected by radiologists” vs. “detected by radiologists and AI” vs. “solely detected by AI”. Fractures that have been only detected by the AI have been radiologically reviewed for plausibility including a visual assessment regarding fracture age.
ResultsOther Section
Heart size
Heart size was initially reported for 54 out of 105 patients (51%); among them 29 patients with “normal heart size” (53.7%), 15 patients with “cardiomegaly” (27.8%) and 10 patients having been classified as “borderline” (18.5%) (see Figure 2, A1). AI analysis revealed significantly elevated total heart volumes for those patients radiologically classified as “borderline” or “cardiomegaly” compared with those radiologically classified as “normal heart size” (see Figure 2, A1). For 51 out of 105 patients (49%) without a mentioned cardiac size assessment in the original report, heart size was visually reviewed by an experienced radiology resident: here again, AI-detected total heart volume significantly correlates with the resident’s re-assessment (see Figure 2, A1). The similar effect could be shown by considering the ratios between total lung and total cardiac volume (see Figure 2, B1) (three patients were excluded because of non-functional lung volumetry, e.g., due relevant pneumothorax). ROC analysis with the radiologists’ heart size assessment as a reference standard (“cardiomegaly” and “borderline” have been pooled and considered as an enlarged heart to obtain the necessary binary reference standard) revealed an AUC of 0.754 for the AI-detected total heart size (see Figure 2, A2). In the same way, an AUC of 0.775 could be reached by considering the AI-detected heart-lung-volume-ratios (see Figure 2, B2). The definition of a total heart volume threshold (threshold optimization according to Youden Statistics) at 902.5 mL for an AI-based “yes-or-no-call” achieved a sensitivity/specificity of 77.3%/67.3%, 17 FPs and a resulting PPV of 70.7% (for “borderline” and “cardiomegaly”). Similarly, the definition of a heart-lung-volume-ratio threshold at 0.245 for an AI-based decision yields a sensitivity/specificity of 88.5%/60.0%, 19 FPs and a resulting PPV of 69.7%. By applying the AI threshold for total/relative heart assessment, the AI would have revealed 25 out of 32 patients with an enlarged heart size that was not mentioned in the initial report, among them 21 out of 25 cases with visually confirmed cardiomegaly or borderline heart size.
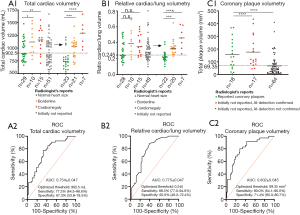
Coronary plaque volumetry
Coronary plaque volumetry revealed plaques for 99 out of 105 patients with a high false positive rate (FPR) supposedly caused by motion artefacts (observed for approx. 90% of scans) and applied contrast media: AI-based plaque suspicion was radiologically confirmed for only 35 out of 99 patients (35%), among them 17 patients with plaques that had not been mentioned in the initial report (see Figure 2, C1). Despite of the high FPR, detected plaque volume quantification significantly correlates with radiologists’ binary “yes-or-no-decision” and the corresponding ROC analysis revealed an AUC of 0.802 (see Figure 2, C2). The definition of a threshold at 69.35 mm3 for an AI-based “yes-or-no-call” (threshold optimization, Youden statistics) achieved a sensitivity/specificity of 80%/71.4%, 20 FPs with a PPV of 58%. Fifteen out of 17 patients with initially non-reported coronary plaques would have been identified by AI-based plaque volume detection exceeding this threshold.
Aorta analysis
Aorta analysis revealed 35 out of 105 patients (33%) with initially non-reported but AI-detected aortic diameters exceeding the population’s mean by at least two standard deviations for at least one landmark position, which was radiologically confirmed for 34 out of 35 cases. The non-confirmed case showed relevant motion artefacts of the ascending aorta. Eighteen out of the 34 patients with aortic ectasias showed enlarged diameters at more than one landmark position; the ascending aorta was involved in 33 out of 34 cases. Three out of 34 patients had an ascending aorta diameter exceeding the population’s mean by more than 50% (see Figure 3).
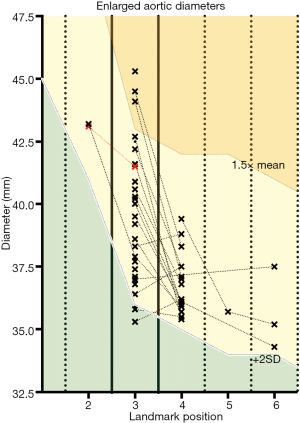
Lung lesions
Lung lesions were detected for 66 out of 105 patients: detection solely by radiologists for one patient (1.5%), detection by radiologists as well as AI for 11 patients (16.7%) and detection solely by the AI algorithm for 54 patients (81.8%) (see Figure 4, A1). In a single-lesion-focused illustration limited to the three largest AI-detected lesions for each patient, we identified 81 out of 108 (75%) lesions that have been solely detected by the AI algorithm (see Figure 4, A2). Sixty-four out these 81 lesions (79%) were radiologically confirmed for plausibility and classified as follows: scarred or post-inflammatory (n=30, 37.0%), trauma-associated including pathologies as previously described in the methodology section (n=21, 25.9%), granulomas (n=5, 6.2%), PFNLs (n=5, 6.2%) and unclear in recommendation of control according to Fleischner’s criteria (n=3, 3.7%) (see Figure 4, A3).
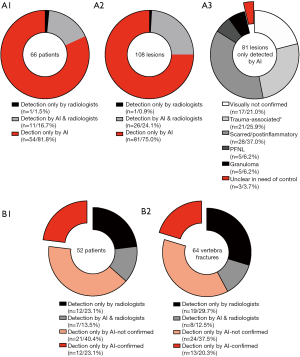
Vertebral fractures
Vertebral fractures were detected by radiologists and/or the AI algorithm for 52 out of 105 patients (49.5%) including 64 different fractures (see Figure 4, B1/B2): 19 out of 64 fractures (29.7%) were solely detected by radiologists, 8 out of 64 fractures (12.5%) by radiologists as well as by the AI and 37 out of 64 vertebra bodies (57.8%) were classified as suspicious for fracture only by the AI algorithm. The algorithm showed a high FPR: only 13 out of 37 fractures (35.1%) that have been exclusively detected by the AI algorithm were radiologically classified as suspicious for fracture (see Figure 4, B2). These 13 detected and initially missed fractures (12 different patients) were slight impressions of the endplates without relevant affections of the posterior edge, two of them (two different patients) radiologically appeared to have an acute traumatic origin.
DiscussionOther Section
Based on 105 “shock-room” emergency CT scans, we demonstrated an AI system that would have decreased the number of missed secondary thoracic findings in an AI-assisted reading setting. The added clinical value could be quantified by the number of additional findings as follows: up to 25 (23.8%) patients with cardiomegaly or borderline heart size, 17 (16.2%) patients with coronary plaques, 34 (32.4%) patients with dilatations of the thoracic aorta, 13 additional vertebral fractures (two of them with an acute traumatic origin) and three lung lesions of two different patients that were radiologically classified as “in recommendation to control”.
Since all the patients have been referred to our emergency department as possibly life-threatening shock-room cases (most of them due to trauma), it can be assumed that they received whole-body imaging for the first time that possibly yields a relevant number of findings that had not been known so far. The patient’s benefit of additionally detected secondary findings depends on the clinical relevance in terms of necessary follow-up or treatment. Detected cardiomegaly or coronary plaques for example should lead to a cardiovascular follow-up, possibly ending up in a primary or secondary prevention that also includes medical treatment (e.g., statins, β-blockers or ASS). Although total cardiac volume estimation in non-ECG-gated CTs cannot be considered as sufficiently accurate for a cardiac diagnostic (no gender- or age-dependent size assessment compared to an appropriate reference population, systolic or diastolic heart phase acquired randomly without ECG-gating), it can yield a recommendation for further diagnostics, e.g., echocardiography. Also, the detection of pre-existing vertebral fractures possibly due to osteoporosis might indicate an anti-osteoporotic treatment. But also, the indication for follow-up-imaging remains important: for malignancy assessment of lung lesions or to optimally balance the risks of aortic surgery with the probability of aneurysm rupture with high mortality in the case of growing aortic aneurysms.
Nevertheless, not only sensitivity but also the PPV of the demonstrated algorithms is crucial to also not overwhelm reporting radiologists with FPs or non-relevant findings. Coronary plaque and vertebral fracture detection especially do not seem to be sufficiently specific in our clinical setting; focusing on coronary plaque detection, limited PPVs of 58% due to a high number of FPs (n=20) are assumed to be caused by the CT protocol (contrast media enhancement, no ECG-gating) yielding pulsation artefacts of the coronary vessels and a limited contrast between contrast-enhanced blood vessels and directly adjacent calcified plaques. Indeed, from the radiologists’ point of view, there were strong limitations for the coronary artery assessment in 95 out of 105 CT scans due to pulsation artefacts. Consequently, we would strongly expect an increasing diagnostic accuracy of the AI algorithm, e.g., by using ECG-gated cardiac CT protocols.
Focusing on vertebral fracture detection, we also observed a relevant number of FNs (n=19) as well as FPs (n=24), here limiting the PPV to 46.7%. This was commonly caused by an inappropriate segmentation of the vertebral bodies, but also due to the FP detection of other pathologies that are prone to mimic vertebral cover plate impressions, e.g., Schmorl’s nodes. Furthermore, fracture detection is limited to vertebral height reduction which does not allow for fracture age assessment and might miss fractures without a secondary vertebral height reduction.
The AI-based lung lesion detection reveals a high number of additional results from which the majority (64 out of 81, 79%) was visually confirmed. Nevertheless, we would like to mention that harmless findings (such as granulomas, PFNLs or small post-inflammatory nodules) are often noticed by radiologists but not necessarily mentioned in the reports. Ruling out non-relevant AI-findings is time-consuming. For that reason, it will be important to implement not only lesion detection but also malignancy assessment in the ongoing algorithm development. Also, traumatic lung lesions and respiratory artefacts (limited patient condition) overrepresented in patients of critical posttraumatic condition might hamper the AI-based discrimination of preexisting lung lesions. But even the applied algorithm identified two patients with initially missed lung lesions that have been classified as in recommendation to control according to Fleischner’s criteria assuming a low-risk profile of the patients; the largest lesion was measured at 8 mm.
AI-based aorta assessment yielded the most additional results: 34 out of 35 AI-findings were radiologically confirmed with none of these mentioned in the primary radiologists’ reports. In particular, three patients were identified with diameters of the ascending aorta exceeding the population’s mean by more than 50% and one patient had a diameter of the ascending aorta larger than 45 mm, which is considered as a lower threshold for therapeutic interventions for patients with additional risk factors (e.g., family history, history of dissection or aortic regurgitation) according to the 2014 European Society of Cardiology (ESC) guidelines (24).
Limitations of our study refer to the scanning protocol and radiological re-assessment of AI findings. We quantified algorithm performance based on CT images acquired with a suboptimal scanning protocol, e.g., regarding aorta or heart assessment in non-ECG triggered scans and with contrast media hampering coronary plaque detection. Nevertheless, we compared with radiologists who equally have to deal with scanning parameters not optimized for the detection of specified secondary findings and in doing so, we also characterized the algorithm robustness in a clinical representative setting. Furthermore, radiological re-assessment of AI findings for plausibility and classification was solely performed by one radiology resident (3 years of experience in thoracic imaging with >5,500 CT scans, ambiguous cases reviewed by a board-certified radiologist) in a proof-of-concept setting, which does not allow for inter-reader variability analysis. Nevertheless, reported AI-findings have also been correlated with the initial reporting with at least one board-certified radiologist involved. Here, our approach is not able to distinguish between initially missed secondary findings and those findings that have not been mentioned due to the lack of relevance.
In order to finally also address possible future AI applications in radiology: Our results support the idea that AI applications can assist the radiologist especially where detections, measurements and quantitative assessments are involved. The rapid and automatic detection of pathological lesions or pathological measurements is intended to reduce the rate of missed findings, but also enables quick triaging with regard to the radiologists’ reading list. Urgent cases can be presented to the radiologist in a prioritized manner after passing through AI software and thus, urgent medical interventions can be initiated earlier. The accurate assessment of image data facilitated by AI also enables the establishment of quantitative imaging biomarkers. In this way, information that is contained in image data but has not been taken into account so far can be of great use for the patient and the treating physician (for example in the form of “lab-like results”).
ConclusionsOther Section
In conclusion, we demonstrated in a retrospective proof-of-concept setting the high potential of AI approaches to reduce the number of missed secondary findings in clinical emergency settings that require a very time-critical radiological reporting. In particular, the integration of different specialized algorithms in a single software solution is promising to avoid clinically too narrow AI applications. But also with regard to less urgent applications of medical imaging, it should be mentioned that especially non-radiology clinicians might even take more benefit from AI-assisted image analysis compared to anyway well-trained radiologists, e.g., in clinical settings without 24/7 radiology coverage or long turnaround times for radiology reporting. Although algorithms primarily need a high sensitivity to effectively reduce the number of initially missed CT findings, ongoing research should focus on algorithm improvements with regard to specificity to also reduce the number of FPs or non-relevant algorithm findings, which otherwise need to be manually ruled out by radiologists in a time-consuming procedure and also might affect radiologists’ clinical decision making.
AcknowledgmentsOther Section
Funding: LMU Department of Radiology received research funding by Siemens Healthcare AG (grant recepient BO Sabel).
FootnoteOther Section
Conflicts of Interest: All authors have completed the ICMJE uniform disclosure form (available at http://dx.doi.org/10.21037/qims-20-1037). Dr. JIS reports personal fees from Siemens Healthcare GmbH, outside the submitted work and during the conduct of the study (employment); Drs. JR and BOS report compensation by Siemens Healthineers for speaker’s activity at conferences. All authors affiliated to LMU Department of Radiology report grants from Siemens Healthcare GmbH, during the conduct of the study (see acknowledgments above). The other authors have no conflicts of interest to declare.
Ethical Statement: The study was approved by institutional ethics board of LMU University Hospital (approval number 18-399); individual consent was waived due to retrospective observational study character and re-analysis of anyway performed and clinically indicated CT imaging.
Open Access Statement: This is an Open Access article distributed in accordance with the Creative Commons Attribution-NonCommercial-NoDerivs 4.0 International License (CC BY-NC-ND 4.0), which permits the non-commercial replication and distribution of the article with the strict proviso that no changes or edits are made and the original work is properly cited (including links to both the formal publication through the relevant DOI and the license). See: https://creativecommons.org/licenses/by-nc-nd/4.0/.
ReferencesOther Section
- Trauma Facts. The American Association for the Surgery of Trauma [Internet]. [cited 2020 Mar 23]. Available online: http://www.aast.org/trauma-facts
- Motta-Ramírez GA. The radiologist physician in major trauma evaluation. Gac Med Mex 2016;152:534-46. [PubMed]
- McKechnie PS, Kerslake DA, Parks RW. Time to CT and Surgery for HPB Trauma in Scotland Prior to the Introduction of Major Trauma Centres. World J Surg 2017;41:1796-800. [Crossref] [PubMed]
- Howlett DC, Drinkwater K, Frost C, Higginson A, Ball C, Maskell G. The accuracy of interpretation of emergency abdominal CT in adult patients who present with non-traumatic abdominal pain: results of a UK national audit. Clin Radiol 2017;72:41-51. [Crossref] [PubMed]
- Guven R, Akca AH, Caltili C, Sasmaz MI, Kaykisiz EK, Baran S, Sahin L, Ari A, Eyupoglu G, Kirpat V. Comparing the interpretation of emergency department computed tomography between emergency physicians and attending radiologists: A multicenter study. Niger J Clin Pract 2018;21:1323-9. [PubMed]
- Lakhani P, Sundaram B. Deep Learning at Chest Radiography: Automated Classification of Pulmonary Tuberculosis by Using Convolutional Neural Networks. Radiology. 2017;284:574-82. [Crossref] [PubMed]
- Gulshan V, Peng L, Coram M, Stumpe MC, Wu D, Narayanaswamy A, Venugopalan S, Widner K, Madams T, Cuadros J, Kim R, Raman R, Nelson PC, Mega JL, Webster DR. Development and Validation of a Deep Learning Algorithm for Detection of Diabetic Retinopathy in Retinal Fundus Photographs. JAMA 2016;316:2402-10. [Crossref] [PubMed]
- Esteva A, Kuprel B, Novoa RA, Ko J, Swetter SM, Blau HM, Thrun S. Dermatologist-level classification of skin cancer with deep neural networks. Nature 2017;542:115-8. Erratum in: Nature 2017 Jun 28;546(7660):686. doi: 10.1038/nature22985. [Crossref] [PubMed]
- McKinney SM, Sieniek M, Godbole V, Godwin J, Antropova N, Ashrafian H, Back T, Chesus M, Corrado GS, Darzi A, Etemadi M, Garcia-Vicente F, Gilbert FJ, Halling-Brown M, Hassabis D, Jansen S, Karthikesalingam A, Kelly CJ, King D, Ledsam JR, Melnick D, Mostofi H, Peng L, Reicher JJ, Romera-Paredes B, Sidebottom R, Suleyman M, Tse D, Young KC, De Fauw J, Shetty S. International evaluation of an AI system for breast cancer screening. Nature 2020;577:89-94. [Crossref] [PubMed]
- Ginat DT. Analysis of head CT scans flagged by deep learning software for acute intracranial hemorrhage. Neuroradiology 2020;62:335-40. [Crossref] [PubMed]
- Rao B, Zohrabian V, Cedeno P, Saha A, Pahade J, Davis MA. Utility of Artificial Intelligence Tool as a Prospective Radiology Peer Reviewer - Detection of Unreported Intracranial Hemorrhage. Acad Radiol 2021;28:85-93. [Crossref] [PubMed]
- Murray NM, Unberath M, Hager GD, Hui FK. Artificial intelligence to diagnose ischemic stroke and identify large vessel occlusions: a systematic review. J Neurointerv Surg 2020;12:156-64. [Crossref] [PubMed]
- Tomita N, Cheung YY, Hassanpour S. Deep neural networks for automatic detection of osteoporotic vertebral fractures on CT scans. Comput Biol Med 2018;98:8-15. [Crossref] [PubMed]
- Ronneberger O, Fischer P, Brox T. U-Net: Convolutional Networks for Biomedical Image Segmentation. In: Navab N, Hornegger J, Wells WM, Frangi AF. editors. Medical Image Computing and Computer-Assisted Intervention – MICCAI 2015. Cham: Springer International Publishing, 2015:234-41.
- Ghesu FC, Georgescu B, Zheng Y, Grbic S, Maier A, Hornegger J, Comaniciu D. Multi-Scale Deep Reinforcement Learning for Real-Time 3D-Landmark Detection in CT Scans. IEEE Trans Pattern Anal Mach Intell 2019;41:176-89. [Crossref] [PubMed]
- Yang D, Xu D, Zhou SK, Georgescu B, Chen M, Grbic S, Metaxas D, Comaniciu D. Automatic liver segmentation using an adversarial image-to-image network. In: Medical Image Computing and Computer Assisted Intervention − MICCAI 2017 - 20th International Conference, Proceedings. Springer Verlag, 2017:507-15.
- Hiratzka LF, Bakris GL, Beckman JA, Bersin RM, Carr VF, Casey DE Jr, Eagle KA, Hermann LK, Isselbacher EM, Kazerooni EA, Kouchoukos NT, Lytle BW, Milewicz DM, Reich DL, Sen S, Shinn JA, Svensson LG, Williams DMAmerican College of Cardiology Foundation/American Heart Association Task Force on Practice Guidelines. American Association for Thoracic Surgery; American College of Radiology; American Stroke Association; Society of Cardiovascular Anesthesiologists; Society for Cardiovascular Angiography and Interventions; Society of Interventional Radiology; Society of Thoracic Surgeons; Society for Vascular Medicine. 2010 ACCF/AHA/AATS/ACR/ASA/SCA/SCAI/SIR/STS/SVM guidelines for the diagnosis and management of patients with Thoracic Aortic Disease: a report of the American College of Cardiology Foundation/American Heart Association Task Force on Practice Guidelines, American Association for Thoracic Surgery, American College of Radiology, American Stroke Association, Society of Cardiovascular Anesthesiologists, Society for Cardiovascular Angiography and Interventions, Society of Interventional Radiology, Society of Thoracic Surgeons, and Society for Vascular Medicine. Circulation 2010;121:e266-369. Erratum in: Circulation 2010 Jul 27;122(4):e410. [PubMed]
- Ren S, He K, Girshick R, Sun J. Faster R-CNN: Towards Real-Time Object Detection with Region Proposal Networks. IEEE Transactions on Pattern Analysis and Machine Intelligence 2017;39:1137-49. [Crossref] [PubMed]
- He K, Zhang X, Ren S, Sun J. Deep Residual Learning for Image Recognition. 2016 IEEE Conference on Computer Vision and Pattern Recognition (CVPR), Las Vegas, NV, USA, 2016:770-8. doi:
10.1109/CVPR.2016.90 . - Zhan Y, Jian B, Maneesh D, Zhou X. Cross-Modality Vertebrae Localization and Labeling Using Learning-Based Approaches. In: Lecture Notes in Computational Vision and Biomechanics, 2015:301-22.
- Genant HK, Wu CY, van Kuijk C, Nevitt MC. Vertebral fracture assessment using a semiquantitative technique. J Bone Miner Res 1993;8:1137-48. [Crossref] [PubMed]
- Adams JE, Lenchik L, Roux C, Genant HK. Radiological Assessment of Vertebral Fracture. International Osteoporosis Foundation Vertebral Fracture Initiative Resource Document Part II, 2010.
- Youden WJ. Index for rating diagnostic tests. Cancer 1950;3:32-5. [Crossref] [PubMed]
- Erbel R, Aboyans V, Boileau C, Bossone E, Bartolomeo RD, Eggebrecht H, Evangelista A, Falk V, Frank H, Gaemperli O, Grabenwöger M, Haverich A, Iung B, Manolis AJ, Meijboom F, Nienaber CA, Roffi M, Rousseau H, Sechtem U, Sirnes PA, Allmen RS, Vrints CJESC Committee for Practice Guidelines. 2014 ESC Guidelines on the diagnosis and treatment of aortic diseases: Document covering acute and chronic aortic diseases of the thoracic and abdominal aorta of the adult. The Task Force for the Diagnosis and Treatment of Aortic Diseases of the European Society of Cardiology (ESC). Eur Heart J 2014;35:2873-926. Erratum in: Eur Heart J 2015 Nov 1;36(41):2779. [Crossref] [PubMed]