Value of total lesion glycolysis and cell-of-origin subtypes for prognostic stratification of diffuse large B-cell lymphoma patients
Introduction
Diffuse large B-cell lymphoma (DLBCL), as the most common subtype of lymphoma, is a heterogeneous clinicopathologic entity accounting for 30% of non-Hodgkin’s lymphomas (1). Although the introduction of rituximab (R) to cyclophosphamide, doxorubicin, vincristine, and prednisone (CHOP) has significantly improved survival outcomes for DLBCL patients (2), approximately 30–40% of patients still experience a relapse or are refractory to therapy (3).
Designed in 1993, the International Prognostic Index (IPI) was the first widely accepted prognostic score to predict patients’ survival outcomes with DLBCL and other aggressive lymphomas (4). However, the power of IPI in risk stratification has been questioned in the R era, especially because of its limited capacity for identifying patients with a higher risk (5,6). To improve risk stratification, the National Comprehensive Cancer Network International Prognostic Index (NCCN-IPI), established in patients treated with R-CHOP, was proposed by Zhou et al. in 2014 (7). Nonetheless, a large number of patients with an unfavorable outcome remain insufficiently identified (8). The prominent challenge for accurate outcomes prediction by these traditional models comes from the clinically and biologically heterogeneous nature of DLBCL; these models are exclusively based on clinical variables and therefore fail to capture information on biological and molecular patterns (9). Therefore, there is a pressing need for newer strategies to subcategorize DLBCL patients better.
Fluorine-18-fluorodeoxyglucose (18F-FDG) positron emission tomography/computed tomography (PET/CT) has become a routine tool for prognostic assessment and management in DLBCL. Baseline volume-based PET parameters, such as total metabolic tumor volume (TMTV) and total lesion glycolysis (TLG), reflecting the tumor burden and metabolism, have been proposed as promising prognostic biomarkers in DLBCL. A growing body of literature shows that baseline TMTV or TLG can be used to risk-stratify patients with DLBCL (10-14). Furthermore, a recent study reported that TMTV could even allow for identifying a small group of high-risk NCCN-IPI of ≥4 patients with significantly different outcomes (15).
Cell-of-origin subtypes determined by immunohistochemical (IHC) algorithms has identified 2 distinct molecular subtypes of DLBCL: the germinal center B-cell (GCB) and the non-GCB (16). Multiple studies have demonstrated that non-GCB is associated with more aggressive behavior and worse outcomes than GCB patients when treated with R-CHOP (16-18). Therefore, we also assessed the cell-of-origin subtypes as a prognostic marker in this study.
The purpose of the present study was to explore the potential additional predictive value of PET biomarkers and cell-of-origin subtypes in patients with DLBCL.
Methods
Participant cohort
The retrospective study was conducted with de novo nodal DLBCL patients between September 2012 and July 2017, who underwent baseline whole-body 18F-FDG PET/CT. The inclusion criteria were as follows: histological confirmation of de novo nodal DLBCL, treatment with R-CHOP or R-CHOP-like regimens with curative intent, and 18F-FDG PET/CT before treatment. The exclusion criteria were as follows: previous malignancy, chemotherapy, radiotherapy history, or incomplete follow-up or therapy (Figure 1). Clinical parameters [gender, age, B symptoms, Eastern Cooperative Oncology Group (ECOG) performance status, lactic acid dehydrogenase (LDH) level, and bone marrow involvement status] were collected from their medical records. Bone marrow involvement was determined by ilium puncture. The bulky disease was defined as a mass ≥100 mm in diameter (19). Participants were grouped into those with a low-risk NCCN-IPI of <4 and those with a high-risk NCCN-IPI of ≥4 (7).
PET/CT image acquisition and analysis
All participants underwent whole-body 18F-FDG PET/CT on a combined Gemini GXL PET/CT scanner with a 16-slice CT component (Philips Corp., Amsterdam, the Netherlands). After 6 hours of fasting, 185–370 MBq of 18F-FDG (5.18 MBq/kg) was administered intravenously. Blood glucose was checked before FDG injection, and no participant showed a level >140 mg/dL. Each participant was weighed for standardized uptake value determination before each scan. Whole-body PET/CT scans (from the top of the head to the upper thigh) were started 60 min after radiopharmaceutical injection. Emission data were acquired for 2 min in each bed position. The CT acquisition data were used for attenuation correction and corrected PET images were reconstructed using the ordered subsets expectation maximization (OSEM) method.
Two experienced nuclear medicine physicians read the PET/CT images. These physicians were blinded to all participant information and the participant’s clinical condition. The results were discussed by the two physicians. Images were reviewed using the volume viewer software on a dedicated workstation (CompassView 5.0, Philips Corp., Amsterdam, the Netherlands) to calculate maximal standard uptake value (SUVmax), metabolic tumor volume (MTV), and TLG. Due to the high interobserver reproducibility previously described in lymphoma, voxels’ boundaries were produced automatically with the 41% SUVmax threshold method recommended by the European Association of Nuclear Medicine (20). The TMTV was obtained by summing the MTV of all lesions. The TLG was calculated as the product of TMTV and mean SUV. Liver and bone marrow involvement was calculated only if there was focal uptake. The spleen was considered as involved and calculated if focal uptake or diffuse uptake of >150% of the liver background was observed.
Tissue microarrays and IHC
Tissue microarrays were built by using three representative cores (0.6 mm) of formalin-fixed, paraffin-embedded tissue from each case. We performed IHC staining on 4 µm sections using standard procedures. A decision tree established by Hans et al. with the indicated cut-offs was used to define GCB and non-GCB subtypes (21).
Statistical analysis
All statistical analyses were performed using SPSS 22.0 (IBM Corp., Armonk, NY, USA). Progression-free survival (PFS) and overall survival (OS) were chosen as the endpoints. The definition of PFS was the interval from the time of diagnosis to the date of the first relapse, progression, death from any cause, or last follow-up. The OS was defined as the interval between diagnosis and the date of death from any cause or last follow-up. Survival conditions were evaluated by the Kaplan-Meier method and compared by log-rank tests. The PET and clinical variables and NCCN-IPI, which were significant in the univariate analysis, were entered into a multivariate analysis using the Cox proportional hazards model. Receiver operating characteristic (ROC) curves were used to estimate the accuracy of predicting ideal cut-off values for SUVmax, TMTV, and TLG. A P value less than 0.05 was considered statistically significant.
Results
Participant characteristics and survival results
The clinical characteristics of the 184 participants included in this study are summarized in Table 1. The participant’s median age was 59 years (14–89 years). A total of 76 participants experienced disease relapse or progression, 62 participants died after a median follow-up of 35 months (3–87 months), and the 3-year PFS and OS were 65.2% and 73.0%, respectively.
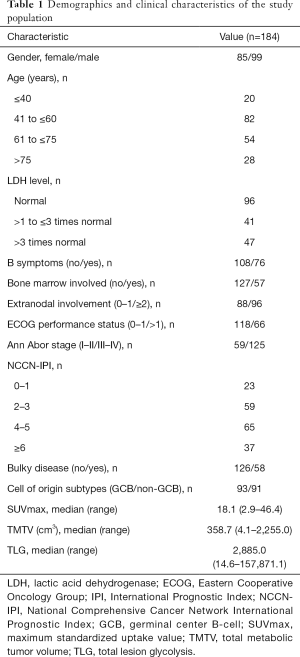
Full table
Clinical characteristics of participants concerning TMTV and TLG
The differences in clinical characteristics between the dichotomized TMTV and TLG groups are shown in Table S1. High LDH level, the presence of B symptoms, bone marrow involvement, extranodal involvement, high ECOG performance status, high Ann Arbor stage, high NCCN-IPI, and the presence of bulky disease were significantly associated with high TMTV and high TLG.
Univariate and multivariate analysis
The ideal cut-off values for PFS calculated by the ROC curve analysis for SUVmax, TMTV, and TLG were 18.4 months, 205.3 cm3, and 1,852 cm3, respectively. Due to the strong correlation between TMTV and TLG, only TLG was entered in the Cox regression model. The univariate analyses are shown in Table 2 and Figure 2. The TLG, cell-of-origin subtypes, as well as NCCN-IPI, were both PFS and OS predictors. High TLG (≥1,852) [PFS: hazard ratio (HR) =1.871, P=0.016; OS: HR =1.920, P=0.025], non-GCB (PFS: HR =2.367, P<0.001; OS: HR =2.084, P=0.005) as well as high NCCN-IPI (≥4) (PFS: HR =2.314, P=0.003; OS: HR =2.281, P=0.009) were shown to be independently significantly associated with inferior PFS and OS after multivariate analysis (Table 3).
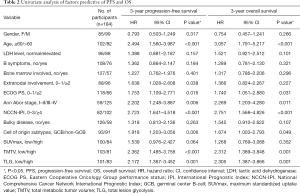
Full table
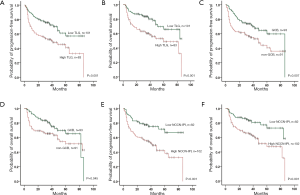
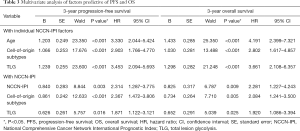
Full table
Revised risk model to predict PFS and OS in DLBCL
Based on the number of risk factors (high TLG, non-GCB, and high NCCN-IPI), a revised risk model was designed, and the participants were divided into four risk groups with very different outcomes (PFS: χ2=30.179, P<0.001; OS: χ2=23.649, P<0.001): low-risk group (none of the 3 predictors, 29 participants)/low-intermediate risk group (1 predictor, 65 participants)/high-intermediate risk group (2 predictors, 60 participants)/high-risk group (all 3 predictors, 30 participants) (Figure 3). Survival curves generated by the revised risk model showed a stronger ability to reveal further discrimination among subgroups compared with the NCCN-IPI alone, especially for participants with very bad survival outcomes (PFS: χ2=9.963, P=0.002; OS: χ2=4.166, P=0.041, respectively) (Figure 3 and Table 4).
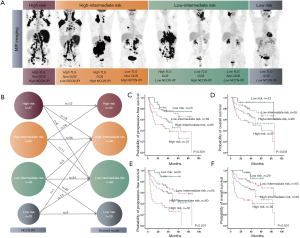
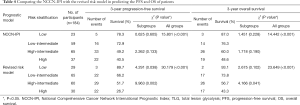
Full table
Due to the high impact of age as a statistically independent predictor of PFS and OS in multivariate analysis, we also evaluated the prognostic value of the revised risk model in age subgroups (≤60 and >60 years old, separately). In both age subgroups, the model presented good discrimination for predicting PFS and OS, especially for the participants who were more than 60 years old (PFS: χ2=8.551, P=0.003; OS: χ2=10.819, P=0.001, respectively). The differences in PFS and OS are shown in Table S2 and Figure 4.
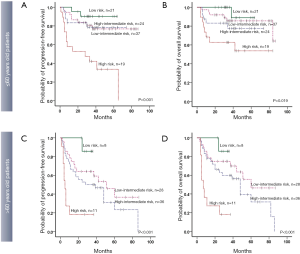
Discussion
Since the introduction of rituximab, the survival of patients with DLBCL has significantly improved. However, the clinical and genetic heterogeneity of DLBCL often presents challenges for accurate risk stratification and prognostic modeling. Herein, we mainly discuss whether the addition of volume-based PET parameters and cell-of-origin subtypes into NCCN-IPI can yield optimal prognostic risk models for DLBCL patients.
Our study demonstrated that participants with high TLG (≥1,852 cm3) had inferior survival outcomes compared to those with low TLG and that 41.3% of patients with high TLG eventually experienced disease relapse, and 33.7% died. This finding is consistent with the results reported in previous studies (22,23). Although the SUVmax is an easy-to-calculate index of the 18F-FDG metabolic rate, it represents an only 1-pixel point of the lesion and could not reflect the entire glucose metabolism condition lesion. Compared to SUVmax, TMTV, and TLG, requiring a three‐dimensional delineation of 18F-FDG avid lesions from PET/CT could be a more powerful index in evaluating tumor burden for patient risk stratification. Although it has not reached a consensus (14,24,25), this study strengthened our belief that the volume parameter TLG, derived from the SUVmean and TMTV, may encompass more metabolic activity information of a tumor lesion.
Participants with a non-GCB phenotype were at a higher risk of relapse or progression than those with a GCB phenotype (3-year PFS: χ2=7.168, P=0.007, 3-year OS: χ2=3.972, P=0.045, respectively) in this study. The present study confirmed the excellent performance of cell-of-origin subtypes in predicting survival outcomes in DLBCL patients, as reported in previous studies (18,26). Cell-of-origin subtypes defined by the IHC-based Hans algorithm could even discriminate very high-risk patients (27). Furthermore, in Toledano et al.’s study (28), the combination of TMTV and cell-of-origin subtypes could allow for significantly better risk stratification of patients with DLBCL in terms of both PFS and OS.
The analysis showed that the NCCN-IPI were predictive of PFS (χ2=15.801, P<0.001) and OS (χ2=14.442, P<0.001), respectively. However, consistent with a previous study (8), it failed to identify a very high-risk group (PFS: χ2=2.262, P=0.133; OS: χ2=1.718, P=0.190, respectively) who should be considered as candidates for more intensive treatments. To improve the accuracy of the NCCN-IPI, the inclusion of TLG and cell-of-origin subtypes as new variables were explored, and the revised risk model showed a better ability of discrimination of a very poor survival outcome group with a 3-year PFS and OS of 18.2% (PFS: χ2=9.963, P=0.002; OS: χ2=4.166, P=0.041, respectively). To the best of our knowledge, few attempts have been made to evaluate the possible additional co-predictive value of both the TLG and cell-of-origin subtypes to the NCCN-IPI.
Older age is an adverse prognostic factor that correlates with inferior survival in DLBCL (29,30). Like previous studies, the present study showed that age was an independent prognostic factor of DLBCL patients (PFS: HR =3.330, P<0.001; OS: HR =3.661, P<0.001, respectively). The poor outcome of elderly DLBCL patients might be partly explained by their decreased ability to tolerate standard chemotherapy regimens and physicians’ tendency to administer more dilute treatments that are better tolerated but deemed less effective (31). The revised risk model could help discriminate the majority of patients with inferior survival outcomes in the different age subgroups, especially for the patients over 60 years old deemed to be very high-risk group (PFS: χ2=8.551, P=0.003; OS: χ2=10.819, P=0.001, respectively), which could be used in making therapeutic decisions.
The limitations of this study arose from its retrospective and single-centered nature. Second, the results among studies might be inconsistent due to the lack of standardization of thresholds used for delineating tumors. The European Association of Nuclear Medicine has recommended the 41% SUVmax threshold method chosen in our study because of the better interobserver agreement (20). Third, the IHC classification systems by the Hans algorithm used in this study as a substitute for a gene expression profile (GEP), which is the gold standard for determining the cell-of-origin subtypes of DLBCL. However, GEP techniques are not routinely utilized in clinical settings because they are costly, time-consuming, and inaccessible to many centers (32). Due to its good correlation (86% concordance) with GEP (33), the algorithm is recommended by the WHO classification to differentiate GCB from non-GCB tumor cells via IHC (34). Considering the difference in both treatment strategy and prognosis between extranodal and nodal DLBCL (35,36), extranodal DLBCL participants were not included in this study.
In conclusion, TLG, cell-of-origin subtypes, and NCCN-IPI are independent prognostic factors of survival in DLBCL patients. Moreover, the revised risk model can stratify patients better than the NCCN-IPI, especially for patients at high risk, which suggests its potential for integration into decision making to achieve a more personalized medicine approach.
Acknowledgments
Funding: None.
Footnote
Conflicts of Interest: All authors have completed the ICMJE uniform disclosure form (available at http://dx.doi.org/10.21037/qims-20-1166). The authors have no conflicts of interest to declare.
Ethical Statement: This study was approved by the Ethics Committee of Nanjing Drum Tower Hospital, the Affiliated Hospital of Nanjing University Medical School and informed consent was waived from all the participants due to the retrospective nature.
Open Access Statement: This is an Open Access article distributed in accordance with the Creative Commons Attribution-NonCommercial-NoDerivs 4.0 International License (CC BY-NC-ND 4.0), which permits the non-commercial replication and distribution of the article with the strict proviso that no changes or edits are made and the original work is properly cited (including links to both the formal publication through the relevant DOI and the license). See: https://creativecommons.org/licenses/by-nc-nd/4.0/.
References
- Lossos IS. Molecular pathogenesis of diffuse large B-cell lymphoma. J Clin Oncol 2005;23:6351-7. [Crossref] [PubMed]
- Coiffier B, Lepage E, Briere J, Herbrecht R, Tilly H, Bouabdallah R, Morel P, Van Den Neste E, Salles G, Gaulard P, Reyes F, Lederlin P, Gisselbrecht C. CHOP chemotherapy plus rituximab compared with CHOP alone in elderly patients with diffuse large-B-cell lymphoma. N Engl J Med 2002;346:235-42. [Crossref] [PubMed]
- Friedberg JW. Relapsed/refractory diffuse large B-cell lymphoma. Hematology Am Soc Hematol Educ Program 2011;2011:498-505. [Crossref] [PubMed]
- International Non-Hodgkin's Lymphoma Prognostic Factors Project. A predictive model for aggressive non-Hodgkin's lymphoma. N Engl J Med 1993;329:987-94. [Crossref] [PubMed]
- Sehn LH, Berry B, Chhanabhai M, Fitzgerald C, Gill K, Hoskins P, Klasa R, Savage KJ, Shenkier T, Sutherland J, Gascoyne RD, Connors JM. The revised International Prognostic Index (R-IPI) is a better predictor of outcome than the standard IPI for patients with diffuse large B-cell lymphoma treated with R-CHOP. Blood 2007;109:1857-61. [Crossref] [PubMed]
- Ziepert M, Hasenclever D, Kuhnt E, Glass B, Schmitz N, Pfreundschuh M, Loeffler M. Standard International prognostic index remains a valid predictor of outcome for patients with aggressive CD20+ B-cell lymphoma in the rituximab era. J Clin Oncol 2010;28:2373-80. [Crossref] [PubMed]
- Zhou Z, Sehn LH, Rademaker AW, Gordon LI, Lacasce AS, Crosby-Thompson A, Vanderplas A, Zelenetz AD, Abel GA, Rodriguez MA, Nademanee A, Kaminski MS, Czuczman MS, Millenson M, Niland J, Gascoyne RD, Connors JM, Friedberg JW, Winter JN. An enhanced International Prognostic Index (NCCN-IPI) for patients with diffuse large B-cell lymphoma treated in the rituximab era. Blood 2014;123:837-42. [Crossref] [PubMed]
- El-Galaly TC, Villa D, Alzahrani M, Hansen JW, Sehn LH, Wilson D, de Nully Brown P, Loft A, Iyer V, Johnsen HE, Savage KJ, Connors JM, Hutchings M. Outcome prediction by extranodal involvement, IPI, R-IPI, and NCCN-IPI in the PET/CT and rituximab era: a Danish-Canadian study of 443 patients with diffuse-large B-cell lymphoma. Am J Hematol 2015;90:1041-6. [Crossref] [PubMed]
- Harkins RA, Chang A, Patel SP, Lee MJ, Goldstein JS, Merdan S, Flowers CR, Koff JL. Remaining challenges in predicting patient outcomes for diffuse large B-cell lymphoma. Expert Rev Hematol 2019;12:959-73. [Crossref] [PubMed]
- Xie M, Zhai W, Cheng S, Zhang H, Xie Y, He W. Predictive value of F-18 FDG PET/CT quantization parameters for progression-free survival in patients with diffuse large B-cell lymphoma. Hematology 2016;21:99-105. [Crossref] [PubMed]
- Islam P, Goldstein J, Flowers CR. PET-derived tumor metrics predict DLBCL response and progression-free survival. Leuk Lymphoma 2019;60:1965-71. [Crossref] [PubMed]
- Vercellino L, Cottereau AS, Casasnovas O, Tilly H, Feugier P, Chartier L, Fruchart C, Roulin L, Oberic L, Pica GM, Ribrag V, Abraham J, Simon M, Gonzalez H, Bouabdallah R, Fitoussi O, Sebban C, López-Guillermo A, Sanhes L, Morschhauser F, Trotman J, Corront B, Choufi B, Snauwaert S, Godmer P, Briere J, Salles G, Gaulard P, Meignan M, Thieblemont C. High total metabolic tumor volume at baseline predicts survival independent of response to therapy. Blood 2020;135:1396-405. [Crossref] [PubMed]
- Pontoizeau C, Girard A, Mesbah H, Haumont LA, Devillers A, Tempescul A, Salaün PY, Lamy T, Le Jeune F, Palard-Novello X. Prognostic Value of Baseline Total Metabolic Tumor Volume Measured on FDG PET in Patients with Richter Syndrome. Clin Nucl Med 2020;45:118-22. [Crossref] [PubMed]
- Sasanelli M, Meignan M, Haioun C, Berriolo-Riedinger A, Casasnovas RO, Biggi A, Gallamini A, Siegel BA, Cashen AF, Véra P, Tilly H, Versari A, Itti E. Pretherapy metabolic tumour volume is an independent predictor of outcome in patients with diffuse large B-cell lymphoma. Eur J Nucl Med Mol Imaging 2014;41:2017-22. [Crossref] [PubMed]
- Shagera QA, Cheon GJ, Koh Y, Yoo MY, Kang KW, Lee DS, Kim EE, Yoon SS, Chung JK. Prognostic value of metabolic tumour volume on baseline (18)F-FDG PET/CT in addition to NCCN-IPI in patients with diffuse large B-cell lymphoma: further stratification of the group with a high-risk NCCN-IPI. Eur J Nucl Med Mol Imaging 2019;46:1417-27. [Crossref] [PubMed]
- Rosenwald A, Wright G, Chan WC, Connors JM, Campo E, Fisher RI, Gascoyne RD, Muller-Hermelink HK, Smeland EB, Giltnane JM, Hurt EM, Zhao H, Averett L, Yang L, Wilson WH, Jaffe ES, Simon R, Klausner RD, Powell J, Duffey PL, Longo DL, Greiner TC, Weisenburger DD, Sanger WG, Dave BJ, Lynch JC, Vose J, Armitage JO, Montserrat E, López-Guillermo A, Grogan TM, Miller TP, LeBlanc M, Ott G, Kvaloy S, Delabie J, Holte H, Krajci P, Stokke T, Staudt LMLymphoma/Leukemia Molecular Profiling Project. The use of molecular profiling to predict survival after chemotherapy for diffuse large-B-cell lymphoma. N Engl J Med 2002;346:1937-47. [Crossref] [PubMed]
- Lenz G, Staudt LM. Aggressive lymphomas. N Engl J Med 2010;362:1417-29. [Crossref] [PubMed]
- Alizadeh AA, Gentles AJ, Alencar AJ, Liu CL, Kohrt HE, Houot R, Goldstein MJ, Zhao S, Natkunam Y, Advani RH, Gascoyne RD, Briones J, Tibshirani RJ, Myklebust JH, Plevritis SK, Lossos IS, Levy R. Prediction of survival in diffuse large B-cell lymphoma based on the expression of 2 genes reflecting tumor and microenvironment. Blood 2011;118:1350-8. [Crossref] [PubMed]
- Pfreundschuh M, Ho AD, Cavallin-Stahl E, Wolf M, Pettengell R, Vasova I, Belch A, Walewski J, Zinzani PL, Mingrone W, Kvaloy S, Shpilberg O, Jaeger U, Hansen M, Corrado C, Scheliga A, Loeffler M, Kuhnt E. MabThera International Trial (MInT) Group.Prognostic significance of maximum tumour (bulk) diameter in young patients with good-prognosis diffuse large-B-cell lymphoma treated with CHOP-like chemotherapy with or without rituximab: an exploratory analysis of the MabThera International Trial Group (MInT) study. Lancet Oncol 2008;9:435-44. [Crossref] [PubMed]
- Meignan M, Sasanelli M, Casasnovas RO, Luminari S, Fioroni F, Coriani C, Masset H, Itti E, Gobbi PG, Merli F, Versari A. Metabolic tumour volumes measured at staging in lymphoma: methodological evaluation on phantom experiments and patients. Eur J Nucl Med Mol Imaging 2014;41:1113-22. [Crossref] [PubMed]
- Hans CP, Weisenburger DD, Greiner TC, Gascoyne RD, Delabie J, Ott G, Müller-Hermelink HK, Campo E, Braziel RM, Jaffe ES, Pan Z, Farinha P, Smith LM, Falini B, Banham AH, Rosenwald A, Staudt LM, Connors JM, Armitage JO, Chan WC. Confirmation of the molecular classification of diffuse large B-cell lymphoma by immunohistochemistry using a tissue microarray. Blood 2004;103:275-82. [Crossref] [PubMed]
- Zhang YY, Song L, Zhao MX, Hu K. A better prediction of progression-free survival in diffuse large B-cell lymphoma by a prognostic model consisting of baseline TLG and %ΔSUV(max). Cancer Med 2019;8:5137-47. [Crossref] [PubMed]
- Mesguich C, Roch A, Hindié E, Milpied N, Bordenave L, Tlili G, Bouabdallah K. Prognostic utility of pre-transplantation [(18) F] fluorodeoxyglucose positron emission tomography/computed tomography in patients with diffuse large B-cell lymphoma who underwent rituximab, dexamethasone, high-dose cytarabine, carboplatin salvage chemotherapy. Br J Haematol 2020;188:268-71. [Crossref] [PubMed]
- Ceriani L, Martelli M, Zinzani PL, Ferreri AJ, Botto B, Stelitano C, Gotti M, Cabras MG, Rigacci L, Gargantini L, Merli F, Pinotti G, Mannina D, Luminari S, Stathis A, Russo E, Cavalli F, Giovanella L, Johnson PW, Zucca E. Utility of baseline 18FDG-PET/CT functional parameters in defining prognosis of primary mediastinal (thymic) large B-cell lymphoma. Blood 2015;126:950-6. [Crossref] [PubMed]
- Mikhaeel NG, Smith D, Dunn JT, Phillips M, Møller H, Fields PA, Wrench D, Barrington SF. Combination of baseline metabolic tumour volume and early response on PET/CT improves progression-free survival prediction in DLBCL. Eur J Nucl Med Mol Imaging 2016;43:1209-19. [Crossref] [PubMed]
- Lenz G, Wright GW, Emre NC, Kohlhammer H, Dave SS, Davis RE, Carty S, Lam LT, Shaffer AL, Xiao W, Powell J, Rosenwald A, Ott G, Muller-Hermelink HK, Gascoyne RD, Connors JM, Campo E, Jaffe ES, Delabie J, Smeland EB, Rimsza LM, Fisher RI, Weisenburger DD, Chan WC, Staudt LM. Molecular subtypes of diffuse large B-cell lymphoma arise by distinct genetic pathways. Proc Natl Acad Sci U S A 2008;105:13520-5. [Crossref] [PubMed]
- Montalbán C, Díaz-López A, Martín A, Baile M, Sanchez JM, Sancho JM, García O, Novelli S, Monter-Rovira A, Salar A, Bastos M, Gutiérrez A, Bento L, Córdoba R, Arquero T, González de Villambrosia S, Barranco G, De Oña R, López Guillermo A, Rodriguez Salazar MJ, Domínguez JF, Fernández R, Queizan JA, Rodríguez J, Abraira V, García JFGELTAMO-IPI Project Investigators. Differential prognostic impact of GELTAMO-IPI in cell of origin subtypes of Diffuse Large B Cell Lymphoma as defined by the Hans algorithm. Br J Haematol 2018;182:534-41. [Crossref] [PubMed]
- Toledano MN, Desbordes P, Banjar A, Gardin I, Vera P, Ruminy P, Jardin F, Tilly H, Becker S. Combination of baseline FDG PET/CT total metabolic tumour volume and gene expression profile have a robust predictive value in patients with diffuse large B-cell lymphoma. Eur J Nucl Med Mol Imaging 2018;45:680-8. [Crossref] [PubMed]
- Dixon DO, Neilan B, Jones SE, Lipschitz DA, Miller TP, Grozea PN, Wilson HE. Effect of age on therapeutic outcome in advanced diffuse histiocytic lymphoma: the southwest oncology group experience. J Clin Oncol 1986;4:295-305. [Crossref] [PubMed]
- Shi Z, Tang X, Shen Q, et al. Clinical characteristics, treatment patterns and outcomes of patients older than 80 years diagnosed with DLBCL in China over a 10-year period. Cancer Chemother Pharmacol 2019;84:127-37. [Crossref] [PubMed]
- Sarkozy C, Coiffier B. Diffuse large B-cell lymphoma in the elderly: a review of potential difficulties. Clin Cancer Res 2013;19:1660-9. [Crossref] [PubMed]
- Barton S, Hawkes EA, Wotherspoon A, Cunningham D. Are we ready to stratify treatment for diffuse large B-cell lymphoma using molecular hallmarks? Oncologist 2012;17:1562-73. [Crossref] [PubMed]
- Meyer PN, Fu K, Greiner TC, Smith LM, Delabie J, Gascoyne RD, Ott G, Rosenwald A, Braziel RM, Campo E, Vose JM, Lenz G, Staudt LM, Chan WC, Weisenburger DD. Immunohistochemical methods for predicting cell of origin and survival in patients with diffuse large B-cell lymphoma treated with rituximab. J Clin Oncol 2011;29:200-7. [Crossref] [PubMed]
- Perroca MG, Jericó MC, Paschoal JV. Identification of care needs of patients with and without the use of a classification instrument. Rev Esc Enferm USP 2014;48:625-31. [Crossref] [PubMed]
- Shi Y, Han Y, Yang J, Liu P, He X, Zhang C, Zhou S, Zhou L, Qin Y, Song Y, Liu Y, Wang S, Jin J, Gui L, Sun Y. Clinical features and outcomes of diffuse large B-cell lymphoma based on nodal or extranodal primary sites of origin: Analysis of 1,085 WHO classified cases in a single institution in China. Chin J Cancer Res 2019;31:152-61. [Crossref] [PubMed]
- Zelenetz AD, Gordon LI, Abramson JS, Advani RH, Bartlett NL, Caimi PF, Chang JE, Chavez JC, Christian B, Fayad LE, Glenn MJ, Habermann TM, Lee Harris N, Hernandez-Ilizaliturri F, Kaminski MS, Kelsey CR, Khan N, Krivacic S, LaCasce AS, Mehta A, Nademanee A, Rabinovitch R, Reddy N, Reid E, Roberts KB, Smith SD, Snyder ED, Swinnen LJ, Vose JM, Dwyer MA, Sundar H. NCCN Guidelines Insights: B-Cell Lymphomas, Version 3.2019. J Natl Compr Canc Netw 2019;17:650-61. [Crossref] [PubMed]