Cortical degeneration detected by neurite orientation dispersion and density imaging in chronic lacunar infarcts
Introduction
Lacunar infarcts (LI) are defined as small (diameter <15 mm) subcortical lesions that usually occur in the deep cerebral white matter, basal ganglia region, and pons (1). They are focal lesions (2); however, they may have more widespread effects, resulting in damage to the connected white matter microstructure (3,4), which in turn promotes the degeneration of remote cortical regions via a retrograde degeneration process (5-9). Several previous studies using structural magnetic resonance imaging (MRI) methods have found atrophy in remote cortical regions connected with LI, supporting the theory of retrograde degeneration (8-10).
However, the microstructural alterations underpinning the reduction of cortical thickness are not yet clear. The reduction of cortical volume or thickness might be caused by accelerated neural death (11) or the loss of neuronal complexity, such as morphological changes to neuronal dendrites (12,13) or the reduction of synapses (14). The loss of neuronal cells is irreversible in most cases; however, compromised dendrites for neuronal connectivity and synaptic integration could be reversed by rehabilitative training and environmental enrichment (15). Therefore, understanding the specific microstructural changes might help to evaluate potential therapeutic strategies. Although several histological studies have found that retrograde degeneration could contribute to cortical neuronal morphological changes and the neuronal cells were relatively preserved (16,17), few studies have demonstrated LI-related cortical microstructural changes using in vivo neuroimaging methods.
Neurite orientation dispersion and density imaging (NODDI) is a biophysical diffusion modeling approach (18-21) used to study gray matter microstructural changes. It separates the signals arising from water diffusion in three microstructure environments: intra-cellular, extra-cellular, and cerebrospinal fluid compartments (22). The intracellular component models dendrites and axons as a set of sticks with restricted diffusion perpendicular to the neurite axes and unhindered diffusion. NODDI adopts the Watson distribution to model the distribution of these sticks (neurites), which permits modeling highly dispersed neuritic structures (such as dendritic trees) in the gray matter tissue. The distribution can be reflected by the orientation dispersion index (ODI) in the NODDI model (23). Several clinical studies on normal aging, schizophrenia, Parkinson’s disease (PD), and Alzheimer’s disease (AD) have demonstrated the sensitivity of the NODDI approach in detecting changes to the gray matter neurites (24-26).
Therefore, we aimed to investigate the cortical microstructural changes in patients with chronic LI using the NODDI approach. We hypothesized that the gray matter microstructure would alter after LI, and the loss of neurites might contribute to cortical atrophy.
Methods
Subjects
We retrospectively reviewed our prospectively collected database for patients with sporadic cerebral small vessel disease (CSVD) defined by neuroimaging characteristics, including white matter hyperintensities and lacunes, between January 2014 and December 2018. The inclusion criteria were as follows: (I) patients with previous definite LI history, including imaging evidence (hyperintensity in diffusion-weighted imaging) and corresponding lacunar stroke symptoms; (II) no evidence of cerebral hemorrhage, cerebral trauma, and brain tumors; and (III) patients with magnetic resonance (MR) sequences, including three-dimensional T1 weighted imaging (3D-T1WI), T2 fluid-attenuated inversion recovery (T2-FLAIR), single-shell (used for tractography and routine diffusion tensor reconstruction) and two-shell (used for NODDI processing) diffusion MRI (dMRI). Patients were excluded based on the following criteria: (I) those with multiple (≥2) LI lesions: and (II) poor image quality caused by head motion. Demographic data and vascular risk factors were recorded. All subjects signed informed consent before enrollment. The medical ethics committee approved this research of the Second Affiliated Hospital, Zhejiang University School of Medicine. A total of 37 patients with single chronic LI were included. The study cohort’s demographics, risk factors, and imaging characteristics (n=37) are provided in Table 1.
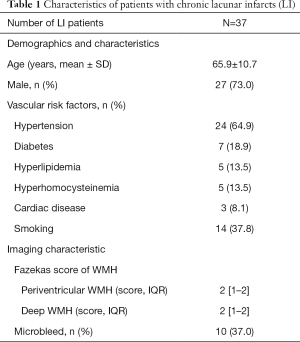
Full table
MRI acquisition
All subjects underwent a MRI using a 3.0-T MR scanner (Discovery MR 750; GE Healthcare, Milwaukee, WI) with an eight-channel phased array head coil. The parameters of the MR sequences were as follows: (I) T2-FLAIR: repetition time (TR) =8,400 ms; echo time (TE) =150 ms; inversion time (TI) =2,100 ms; flip angle =90°; matrix =256×256; field of view (FOV) =24 cm; voxel size =2 mm ×2 mm ×4 mm; (II) 3D-T1WI was acquired using a fast spoiled gradient echo sequence (FSPGR) with TR =7.28 ms; TE =3 ms; TI =450 ms; flip angle =8°; matrix =250×250; voxel size =1 mm ×1 mm ×1 mm; FOV =25 cm; (III) single-shell dMRI was performed using an echo planar imaging (EPI) sequence with TR =8,000 ms; TE =80 ms; 30 directions with b =1,000 s/mm2, three non-diffusion-weighted (b=0) volumes; matrix =128×128; voxel size =2 mm ×2 mm ×2 mm; FOV =25 cm; (IV) two-shell dMRI data was acquired using an EPI sequence with TR =5,000 ms; TE =95 ms; 30 directions with b =1,000 s/mm2 and 30 directions with b=2,000 s/mm2, three non-diffusion-weighted (b=0) volumes ; matrix =128×128; voxel size =2 mm ×2 mm × 4 mm; FOV =24 cm.
Image processing
Diffusion data preprocessing
Diffusion images (both single-shell and two-shell dMRI data) were preprocessed using Functional MRI of the Brain (FMRIB) software library (FSL) version 5.0.7 (FMRIB Center, Department of Clinical Neurology, University of Oxford, Oxford, England; http://www.fmrib.ox.ac.uk/fsl/). Brain tissue was extracted using the Brain Extraction Tool in FSL. Diffusion-weighted images for each of the 30 directions were corrected for eddy currents and head motion (27).
Identifying the ipsilesional cortex connecting with the LI and the corresponding contralesional cortex
LI segmentation and deterministic tractography
Firstly, chronic LI was manually segmented on 3D-T1WI images by an experienced neuroradiologist (XY) using MRIcron (http://www.mricro.com; University of South Carolina, Columbia, SC, USA), as 3D-T1WI has been reported to be sensitive in detecting chronic LI (28). Since many fibers passing through the chronic LI might be disrupted, the segmented masks were enlarged by two voxels in each direction to enable fiber tracking. The LI masks were then registered to b0 images using Advanced Normalization Tools (ANTs) (http://stnava.github.io/ANTs) via the non-rigid transformation method and were used as the seeds for deterministic tractography. Deterministic tractography was performed in the native diffusion space based on the Euler Delta Crossings algorithm using the open-source Diffusion Imaging in Python software (http://dipy.org) (29). Fiber-tracking was restricted by combining the generalized fractional anisotropy (GFA) and maximum turning angle. The GFA was set at >0.2, the maximum turning angle was set at 45°, and the step size was 0.5 mm.
Determining the ipsilesional and contralesional cortical region of interest (ROI)
Individual 3D-T1WI was automatically parcellated based on the Desikan-Killiany Atlas, which contains 68 cortical regions (34 per hemisphere) using FreeSurfer (version 6.0 https://surfer.nmr.mgh.harvard.edu/). Individual segmentation results were then registered to the individual diffusion space using ANTs. The ipsilesional cortical ROI was determined by two neuroradiologists (HH, XY) and was defined as the cortical region with the most fiber connections with LI on the coronal view of 3D-T1WI. Discrepancies were resolved by discussion until a consensus was reached. The inter-observer agreement between the two neuroradiologists was 0.854 (kappa value). The contralesional cortical ROI was defined as the mirror cortical region in the contralesional hemisphere. Finally, ROIs from both hemispheres were used to analyze cortical thickness and microstructural changes. The detailed processing steps are shown in Figure 1.
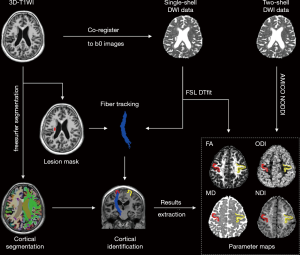
Cortical thickness analysis
Estimations of cortical thickness were performed using FreeSurfer by calculating the mean distance between grey matter/white matter and grey matter/cerebrospinal fluid surfaces at each vertex across the cortical mantle. The procedures have been described in detail in previous studies (30). For each ROI, the percentage change of cortical thickness was calculated by: ΔCTh = (CThipsi − CThcon)/CThcon, where CTh is the cortical thickness.
Diffusion parameters analysis
FA and MD were calculated using DTIFit within FSL based on the single-shell dMRI data. NODDI parameters, including (neurite density index) NDI and ODI, were computed using the Accelerated Microstructure Imaging via Convex Optimization (AMICO) toolbox (31), based on the two-shell dMRI data.
All cortical ROIs were registered to b0 maps in the single-shell and two-shell diffusion imaging space, respectively, using ANTs via the non-rigid transformation method, and the results were then manually corrected by a neuroradiologist (RZ). The corrected results were re-checked by another experienced radiologist (YJ). The mean NDI, ODI, FA, and MD values in the ipsilesional and contralesional cortices’ ROIs were measured (Figure 1).NDI’s percentage changes were calculated by: ΔNDI = (NDI_ipsilesional – NDI_contralesional)/(NDI_contralesional), and ΔODI was calculated in the same way.
Statistical analysis
Statistical analysis was performed using SPSS 23.0 (SPSS Inc., Chicago, USA). All normally distributed variables were reported as mean ± standard deviation, and non-normally distributed variables were reported as median (25th–75th percentile). Categorical variables were presented as frequency (percentage). The Kolmogorov-Smirnov test was used for the analysis of normally distributed data.
The cortical thickness and NODDI metrics (including NDI and ODI) were compared between the ipsilesional and contralesional cortices using a paired t-test. Cohen’s d effect size was calculated by the open-source G*power version 3.1.0. The Pearson correlation coefficient was used to analyze the association between cortical thickness and microstructural metrics and the association between the percentage change of cortical thickness and NODDI metrics.
Considering that neuronal organizations are different across brain cortical regions (32), which may influence the microstructural analysis, and given that a large portion of our subjects had precentral gyrus involvement, we further divided patients into two subgroups: (I) those connecting with the precentral cortex and (II) those connecting with the non-precentral cortices.
Results
Identification of corresponding cortex
The identified ipsilesional cortex is listed in Table S1. The precentral cortex was involved in 23 patients (62.2%), and nine patients (24.3%) had superior-frontal cortex involvement. Other cortical regions, including postcentral, posterior cingulate, caudal middle frontal, and pars triangularis cortex, were involved in the remaining five patients. Therefore, 23 patients had LI connecting with the precentral cortex (precentral group), and 14 patients had LI connecting with the non-precentral cortex (non-precentral group).
Comparisons of cortical thickness and microstructure between the ipsilesional and contralesional cortices
Analysis of all patients
Reduced cortical thickness was observed at the ipsilesional cortex compared to the contralesional cortex (2.465 vs. 2.497, P=0.008). The ODI in the ipsilesional cortex was significantly lower than the ODI in the contralesional cortex (2.451 vs. 2.456, P=0.035). The effect size of cortical thickness and ODI were 0.463 and 0.361, respectively. No significant differences were observed in NDI, FA, and MD between the ipsilesional and contralesional cortices (0.379 vs. 0.379, P=0.925; 0.274 vs. 0.270, P=0.284; 9.38×10−3vs. 9.19×10−3, P=0.128, respectively). The details are shown in Figure 2.
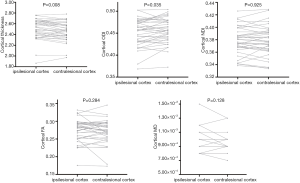
Analysis of the subgroups
The precentral group had reduced cortical thickness at the ipsilesional cortex compared to the contralesional cortex (2.466 vs. 2.502, P=0.015). The ODI in the ipsilesional cortex was significantly lower than the ODI in the contralesional cortex (0.450 vs. 0.458, P=0.004). Marginal significance was found in MD (9.43×10−3vs. 9.13×10−3, P=0.050), while no significant differences were seen in NDI and FA. (0.383 vs. 0.384, P=0.646; 0.291 vs. 0.288, P=0.397; respectively). The details are shown in Figure 3. LI’s effect size to the precentral cortical thickness and ODI were 0.520 and 0.792, respectively, and no significant differences were found in the non-precentral group. The details are shown in Figure S1.
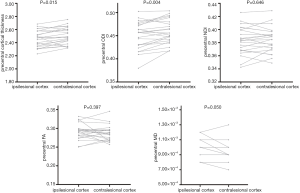
Correlation between CTh and microstructure parameters
Analysis of all patients
In the overall analysis, we did not identify correlations between the cortical thickness and NODDI metrics. The details are shown in Figure S2.
The percentage change of cortical thickness and NODDI metrics were: −1.20%±2.87% for Δ CTh, −1.07%±3.02% for Δ ODI, and 0.09%±2.30% for Δ NDI. We did not observe any correlations between Δ ODI and Δ CTh (r=0.060, P=0.724), and Δ NDI and Δ CTh (r=−0.048, P=0.778).
Analysis of the subgroups
In the precentral group, we found that cortical thickness was correlated with ODI (r=0.437, P=0.037) and NDI (r=0.473, P=0.023) in the ipsilesional cortex. In the contralesional cortex, cortical thickness was correlated with ODI (r=0.440, P=0.036), and the correlation between cortical thickness and NDI was also significant (r=0.622, P=0.002). The details are shown in Figure 4.
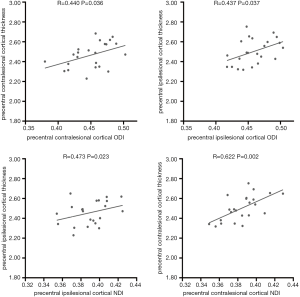
In the precentral group, the percentage changes of cortical thickness and NODDI metrics were: −1.43%±2.72% for Δ CTh, −1.88%±2.84% for Δ ODI, and 0.24%±2.56% for Δ NDI. We did not identify correlations between precentral Δ ODI and Δ CTh (r=0.223, P=0.307), Δ NDI, or Δ CTh (r=−0.118, P=0.592).
Furthermore, we did not observe any correlations between cortical thickness and NODDI metrics in the non-precentral group. The percentage change of cortical thickness and NODDI metrics in the non-precentral group were: −0.83%±3.16% for Δ CTh, 0.25%±2.91% for Δ ODI, and 0.62%±1.76% for Δ NDI. We did not find any correlations between Δ ODI and Δ CTh (r=−0.253, P=0.382), or between Δ NDI and Δ CTh (r=0.032, P=0.915).
Discussion
There were two main findings in this study. Firstly, we observed decreased cortical thickness and reduced ODI in the remote cortex connected with the chronic LI. Secondly, in the precentral group, we found that both ODI in the ipsilesional and contralesional cortices were significantly correlated with cortical thickness.
In this study, individual chronic LI was selected as the seed for tractography to identify the remote cortex connected with the LI. Consistent with previous reports (8,33), reduced cortical thickness was observed in the ipsilesional cortex compared to the contralesional cortex. Retrograde degeneration along white matter tracts connecting with the LI has been proposed as the main secondary damage mechanism in the remote cortex (8). Also, the lack of nutrition supplements, such as newly synthesized protein and lipids, and the termination of communication between the axon and soma contribute to cortical thinning (34,35).
We applied the NODDI model to explore cortical microstructure abnormalities and observed a reduction of ODI in the ipsilesional cortex after LI. The NODDI model (32) proposes that ODI estimating neurite dispersion reflects the degree of complexity of dendritic trees in the context of grey matter tissue, which has been histologically validated in animal models (36) and human multiple sclerosis (MS) disease (37). Therefore, combined with our results, the decreases in ODI could be interpreted as reduced dendritic arborization. Retrograde axonal signals control the growth and patterning of dendrites (38). Previous studies investigating dendritic arborization found that axonal input deprivation would affect the growth of dendritic branches and neurite distribution (39,40). Our findings showed evidence of the retrograde degeneration after LI from the microstructural level with neuroimaging. Nevertheless, further histological analyses will be needed to demonstrate these changes.
Furthermore, in the subgroup analysis, we found that ODI reduction and cortical thinning were more significant in the precentral group. This finding is consistent with previous total brain analysis of cortical degeneration after LI. Both voxel-based morphological (VBM) analysis and surfaced-based thickness studies consistently found degeneration of the precentral cortex in the affected hemisphere (5-7). Two previous studies investigating the retrograde degeneration mechanism after LI also limited the cortical analysis in motor-related regions (8,9). One possible reason might be that the precentral cortex is more sensitive to the loss of retrograde axonal input. The precentral cortex is comprised of substantial pyramidal neurons that intensively communicate with axonal input signals. Furthermore, a recent study demonstrated that cortical thinning, which occurred extensively in the cortical layer, consisted of pyramidal neurons (41).
Although we did not find any correlations between cortical thickness and NODDI metrics in our analyses of all patients, we found that cortical thickness was correlated with ODI in the ipsilesional cortex in the precentral group. The microstructural changes behind the cortical thinning were complicated. The most widely accepted microstructural change underlying cortical degeneration is the loss of neuronal cells. Nevertheless, recent studies have found that the loss of cortical volume or thickness could be caused by the destruction of neurite complexity, while neuron cell bodies were relatively preserved. For example, in a study of a preterm cerebral ischemic animal model, disturbances of cerebral neuronal arborization were the main reason for the loss of cortical volume (13). One AD study found a substantial reduction in cortical thickness in the trans-entorhinal cortex. However, no changes were observed in the volume occupied by neuronal cell bodies (14). Therefore, our findings suggest that retrograde degeneration initiated by LI might initially damage the neurite arborization represented by ODI, and then, the reduction of ODI contributes to the cortical thinning. It has been reported that compromised dendrites for neuronal connectivity and synaptic integration could be reversed by rehabilitative training and environmental enrichment (15). Therefore, the ODI value could be a potential early biomarker for cortical degeneration after LI, guiding clinical therapies for preserving and recovering cortical functions.
Significant differences were exhibited in the NODDI parameters but not in the diffusion tensor imaging (DTI) parameters in our study, suggesting that the NODDI metrics were more sensitive than FA or MD in detecting cortical microstructural changes, which was also demonstrated in previous studies (42-44). Accordingly, the NODDI approach is an appropriate method for the analysis of cortical microstructural changes.
There are some limitations in this study that should be noted. Firstly, the 4 mm-thick slices used in the two-shell diffusion-weighted imaging may have caused inaccurate registration between the NODDI maps and the 3D-T1WI. To control the quality of registration, the registered NODDI maps were carefully corrected by a senior neuroradiologist. Secondly, the connected cortex was obtained from an anatomical atlas, which may have overestimated the regions connected with the subcortical LI. However, it is difficult to identify the specific cortex connecting with the LI without a reference atlas.
Furthermore, the heterogeneity of the cortical area identified from an individual subject may be a source of potential bias in our analysis. Lastly, neurological functions, such as motor functions and cognition were not analyzed in our study. These should be investigated in future studies.
Conclusions
NODDI metrics could reflect cortical microstructural changes following LI, and the decreased ODI suggests that loss of dendrites might contribute to cortical thinning.
Acknowledgments
Funding: This work was supported by the 13th Five-year Plan for the National Key Research and Development Program of China (Grant No.: 2016YFC1306600), the Zhejiang Provincial Natural Science Foundation of China (Grant No.: LSZ19H180001 & LQ17H180002), the National Natural Science Foundation of China (Grant No.: 81271530, 81901706 & 81771820), and the Health and Family Planning Commission of Zhejiang Province (Grant No.: 2016KYA099).
Footnote
Conflicts of Interest: All authors have completed the ICMJE uniform disclosure form (available at http://dx.doi.org/10.21037/qims-20-880). The authors have no conflicts of interest to declare.
Ethical Statement: This research was approved by the medical ethics committee of the Second Affiliated Hospital, Zhejiang University School of Medicine. The study was conducted in accordance with the Declaration of Helsinki and the Harmonized Tripartite Guidelines for Good Clinical Practice from the International Conference on Harmonization. Written informed consent was obtained from the patients for publication of this study and any accompanying images. A copy of the written consent is available for review by the Editor-in-Chief of this journal.
Open Access Statement: This is an Open Access article distributed in accordance with the Creative Commons Attribution-NonCommercial-NoDerivs 4.0 International License (CC BY-NC-ND 4.0), which permits the non-commercial replication and distribution of the article with the strict proviso that no changes or edits are made and the original work is properly cited (including links to both the formal publication through the relevant DOI and the license). See: https://creativecommons.org/licenses/by-nc-nd/4.0/.
References
- Norrving B. Long-term prognosis after lacunar infarction. Lancet Neurol 2003;2:238-45. [Crossref] [PubMed]
- Ter Telgte A, van Leijsen EMC, Wiegertjes K, Klijn CJM, Tuladhar AM, de Leeuw FE. Cerebral small vessel disease: from a focal to a global perspective. Nat Rev Neurol 2018;14:387-98. [Crossref] [PubMed]
- Reijmer YD, Freeze WM, Leemans A, Biessels GJ. The effect of lacunar infarcts on white matter tract integrity. Stroke 2013;44:2019-21. [Crossref] [PubMed]
- Auriel E, Edlow BL, Reijmer YD, Fotiadis P, Ramirez-Martinez S, Ni J, Reed AK, Vashkevich A, Schwab K, Rosand J. Microinfarct disruption of white matter structure: a longitudinal diffusion tensor analysis. Neurology 2014;83:182-8. [Crossref] [PubMed]
- Jones PW, Borich MR, Vavsour I, Mackay A, Boyd LA. Cortical thickness and metabolite concentration in chronic stroke and the relationship with motor function. Restor Neurol Neurosci 2016;34:733-46. [Crossref] [PubMed]
- Jiang L, Liu J, Wang C, Guo J, Cheng J, Han T, Miao P, Cao C, Yu C. Structural alterations in chronic capsular versus pontine stroke. Radiology 2017;285:214-22. [Crossref] [PubMed]
- Yu X, Yang L, Song R, Jiaerken Y, Yang J, Lou M, Jiang Q, Zhang M. Changes in structure and perfusion of grey matter tissues during recovery from Ischaemic subcortical stroke: a longitudinal MRI study. Eur J Neurosci 2017;46:2308-14. [Crossref] [PubMed]
- Duering M, Righart R, Wollenweber FA, Zietemann V, Gesierich B, Dichgans M. Acute infarcts cause focal thinning in remote cortex via degeneration of connecting fiber tracts. Neurology 2015;84:1685-92. [Crossref] [PubMed]
- Cheng B, Schulz R, Bönstrup M, Hummel FC, Sedlacik J, Fiehler J, Gerloff C, Thomalla G. Structural plasticity of remote cortical brain regions is determined by connectivity to the primary lesion in subcortical stroke. J Cereb Blood Flow Metab 2015;35:1507-14. [Crossref] [PubMed]
- Cheng B, Dietzmann P, Schulz R, Boenstrup M, Krawinkel L, Fiehler J, Gerloff C, Thomalla G. Cortical atrophy and transcallosal diaschisis following isolated subcortical stroke. J Cereb Blood Flow Metab 2020;40:611-21. [Crossref] [PubMed]
- Carassiti D, Altmann D, Petrova N, Pakkenberg B, Scaravilli F, Schmierer K. Neuronal loss, demyelination and volume change in the multiple sclerosis neocortex. Neuropathol Appl Neurobiol 2018;44:377-90. [Crossref] [PubMed]
- Pannese E. Morphological changes in nerve cells during normal aging. Brain Struct Funct 2011;216:85-9. [Crossref] [PubMed]
- Dean JM, McClendon E, Hansen K, Azimi-Zonooz A, Chen K, Riddle A, Gong X, Sharifnia E, Hagen M, Ahmad T. Prenatal cerebral ischemia disrupts MRI-defined cortical microstructure through disturbances in neuronal arborization. Sci Transl Med 2013;5:168ra7. [Crossref] [PubMed]
- Domínguez-Álvaro M, Montero-Crespo M, Blazquez-Llorca L, Insausti R, DeFelipe J, Alonso-Nanclares L. Three-dimensional analysis of synapses in the transentorhinal cortex of Alzheimer’s disease patients. Acta Neuropathol Commun 2018;6:20. [Crossref] [PubMed]
- Zhang QW, Deng XX, Sun X, Xu JX, Sun FY. Exercise promotes axon regeneration of newborn striatonigral and corticonigral projection neurons in rats after ischemic stroke. PLoS One 2013;8:e80139. [Crossref] [PubMed]
- Kalil K, Schneider GE. Retrograde cortical and axonal changes following lesions of the pyramidal tract. Brain Res 1975;89:15-27. [Crossref] [PubMed]
- Wannier T, Schmidlin E, Bloch J, Rouiller EM. A unilateral section of the corticospinal tract at cervical level in primate does not lead to measurable cell loss in motor cortex. J Neurotrauma 2005;22:703-17. [Crossref] [PubMed]
- Li CX, Patel S, Zhang X. Evaluation of multi-shell diffusion MRI acquisition strategy on quantitative analysis using multi-compartment models. Quant Imaging Med Surg 2020;10:824-34. [Crossref] [PubMed]
- Mustafi SM, Harezlak J, Kodiweera C, Randolph JS, Ford JC, Wishart HA, Wu YC. Detecting white matter alterations in multiple sclerosis using advanced diffusion magnetic resonance imaging. Neural Regen Res 2019;14:114. [Crossref] [PubMed]
- Gatto RG, Amin M, Finkielsztein A, Weissmann C, Barrett T, Lamoutte C, Uchitel O, Sumagin R, Mareci TH, Magin RL. Unveiling early cortical and subcortical neuronal degeneration in ALS mice by ultra-high field diffusion MRI. Amyotroph Lateral Scler Frontotemporal Degener 2019;20:549-61. [Crossref] [PubMed]
- Gatto RG, Mustafi SM, Amin MY, Mareci TH, Wu YC, Magin RL. Neurite orientation dispersion and density imaging can detect presymptomatic axonal degeneration in the spinal cord of ALS mice. Funct Neurol 2018;33:155. [PubMed]
- Zhang H, Schneider T, Wheeler-Kingshott CA, Alexander DC. NODDI: practical in vivo neurite orientation dispersion and density imaging of the human brain. Neuroimage 2012;61:1000-16. [Crossref] [PubMed]
- Sotiropoulos SN, Behrens TE, Jbabdi S. Ball and rackets: inferring fiber fanning from diffusion-weighted MRI. Neuroimage 2012;60:1412-25. [Crossref] [PubMed]
- Nazeri A, Mulsant BH, Rajji TK, Levesque ML, Pipitone J, Stefanik L, Shahab S, Roostaei T, Wheeler AL, Chavez S. Gray matter neuritic microstructure deficits in schizophrenia and bipolar disorder. Biol Psychiatry 2017;82:726-36. [Crossref] [PubMed]
- Andica C, Kamagata K, Hatano T, Okuzumi A, Saito A, Nakazawa M, Ueda R, Motoi Y, Kamiya K, Suzuki M. Neurite orientation dispersion and density imaging of the nigrostriatal pathway in Parkinson’s disease: Retrograde degeneration observed by tract-profile analysis. Parkinsonism Relat Disord 2018;51:55-60. [Crossref] [PubMed]
- Kamagata K, Zalesky A, Hatano T, Ueda R, Di Biase MA, Okuzumi A, Shimoji K, Hori M, Caeyenberghs K, Pantelis C. Gray Matter Abnormalities in Idiopathic P arkinson’s Disease: Evaluation by Diffusional Kurtosis Imaging and Neurite Orientation Dispersion and Density Imaging. Hum Brain Mapp 2017;38:3704-22. [PubMed]
- Andersson JL, Sotiropoulos SN. An integrated approach to correction for off-resonance effects and subject movement in diffusion MR imaging. Neuroimage 2016;125:1063-78. [Crossref] [PubMed]
- Moreau F, Patel S, Lauzon ML, McCreary CR, Goyal M, Frayne R, Demchuk AM, Coutts SB, Smith EE. Cavitation after acute symptomatic lacunar stroke depends on time, location, and MRI sequence. Stroke 2012;43:1837-42. [Crossref] [PubMed]
- Garyfallidis E, Brett M, Amirbekian B, Rokem A, van der Walt S, Descoteaux M, Nimmo-Smith I. Dipy, a library for the analysis of diffusion MRI data. Front Neuroinform 2014;8:8. [Crossref] [PubMed]
- Fischl B, Dale AM. Measuring the thickness of the human cerebral cortex from magnetic resonance images. Proc Natl Acad Sci U S A 2000;97:11050-5. [Crossref] [PubMed]
- Daducci A, Canales-Rodriguez EJ, Zhang H, Dyrby TB, Alexander DC, Thiran JP. Accelerated Microstructure Imaging via Convex Optimization (AMICO) from diffusion MRI data. Neuroimage 2015;105:32-44. [Crossref] [PubMed]
- Fukutomi H, Glasser MF, Zhang H, Autio JA, Coalson TS, Okada T, Togashi K, Van Essen DC, Hayashi T. Neurite imaging reveals microstructural variations in human cerebral cortical gray matter. Neuroimage 2018;182:488-99. [Crossref] [PubMed]
- Wei XE, Shang K, Zhou J, Zhou YJ, Li YH. Acute Subcortical Infarcts Cause Secondary Degeneration in the Remote Non-involved Cortex and Connecting Fiber Tracts. Front Neurol 2019;10:860. [Crossref] [PubMed]
- Perlson E, Maday S, Fu MM, Moughamian AJ, Holzbaur EL. Retrograde axonal transport: pathways to cell death? Trends Neurosci 2010;33:335-44. [Crossref] [PubMed]
- Duering M, Righart R, Csanadi E, Jouvent E, Herve D, Chabriat H, Dichgans M. Incident subcortical infarcts induce focal thinning in connected cortical regions. Neurology 2012;79:2025-8. [Crossref] [PubMed]
- Wang N, Zhang J, Cofer G, Qi Y, Anderson RJ, White LE, Allan Johnson G. Neurite orientation dispersion and density imaging of mouse brain microstructure. Brain Struct Funct 2019;224:1797-813. [Crossref] [PubMed]
- Grussu F, Schneider T, Tur C, Yates RL, Tachrount M, Ianus A, Yiannakas MC, Newcombe J, Zhang H, Alexander DC, DeLuca GC, Gandini Wheeler-Kingshott CAM. Neurite dispersion: a new marker of multiple sclerosis spinal cord pathology? Ann Clin Transl Neurol 2017;4:663-79. [Crossref] [PubMed]
- da Silva S, Wang F. Retrograde neural circuit specification by target-derived neurotrophins and growth factors. Curr Opin Neurobiol 2011;21:61-7. [Crossref] [PubMed]
- Zweifel LS, Kuruvilla R, Ginty DD. Functions and mechanisms of retrograde neurotrophin signalling. Nat Rev Neurosci 2005;6:615-25. [Crossref] [PubMed]
- Vrieseling E, Arber S. Target-induced transcriptional control of dendritic patterning and connectivity in motor neurons by the ETS gene Pea3. Cell 2006;127:1439-52. [Crossref] [PubMed]
- Lotan E, Tavor I, Barazany D, Ben-Amitay S, Hoffmann C, Tsarfaty G, Assaf Y, Tanne D. Selective atrophy of the connected deepest cortical layers following small subcortical infarct. Neurology 2019;92:e567-e575. [Crossref] [PubMed]
- Ohki A, Saito S, Hata J, Okano HJ, Higuchi T, Fukuchi K. Neurite orientation dispersion and density imaging for evaluating the severity of neonatal hypoxic-ischemic encephalopathy in rats. Magn Reson Imaging 2019;62:214-9. [Crossref] [PubMed]
- Winston GP, Vos SB, Caldairou B, Hong SJ, Czech M, Wood TC, Wastling SJ, Barker GJ, Bernhardt BC, Bernasconi N. Microstructural imaging in temporal lobe epilepsy: Diffusion imaging changes relate to reduced neurite density. Neuroimage Clin 2020;26:102231. [Crossref] [PubMed]
- Andica C, Kamagata K, Hatano T, Saito Y, Ogaki K, Hattori N, Aoki S. MR biomarkers of degenerative brain disorders derived from diffusion imaging. J Magn Reson Imaging 2020;52:1620-36. [Crossref] [PubMed]