Quantitative assessment of structural and functional changes in temporal lobe epilepsy with hippocampal sclerosis
Introduction
Mesial temporal lobe epilepsy (mTLE) with hippocampus sclerosis (HS) is one of the most common causes of drug-resistant focal epilepsy and can be treated surgically (1). However, only approximately 30% of mTLE patients are thought to have normal MRI images (2). Identifying HS using MRI strengthens the mTLE diagnosis and is crucial for pre-surgical management, as it is strongly associated with epileptogenicity and surgical outcome (3). Seizure freedom after amygdala-hippocampectomy or temporal lobe resection ranges from 60% to 80% for MRI-positive TLE, when compared to MRI-negative TLE patients, who range between 36% and 76% (4,5). The presurgical evaluation of MRI-negative TLE patients remains challenging, necessitating improved quantitative analyses of HS to facilitate pre-surgical evaluations, leading to more favorable surgical outcomes.
In clinical practice, the two main manifestations used to identify HS are hippocampal atrophy seen in coronal T1-weighted images and increased signal intensity of the hippocampus on T2-weighted fluid-attenuated inversion recovery (T2-FLAIR) images (6). Additionally, temporal horn enlargement (7) and blurring of the gray mater-white matter (GM/WM) margin in the temporal pole (6,7) may accompany HS to varying degrees. Structural abnormalities in other limbic structures (6) and the neocortex have also been reported (8). The amygdala has a key role in temporal lobe epilepsy. It is usually involved in the epileptic network (9) and in certain patients it is regarded as an independent epileptogenic focus (10). How the amygdala manifests itself in TLE is disputed. Some studies reported amygdala atrophy (11,12). Others had reported enlargement of the amygdala (AE) in TLE patients with normal hippocampi, considering it a distinct subtype of TLE (13,14). Even in large-scale neuroimaging studies undertaken by the ENIGMA-epilepsy consortium with over 700 mTLE patients, no amygdala atrophy or enlargement was evident (8). Thus, it remains unclear whether quantitative amygdala structural analysis would prove useful in the presurgical evaluation.
Structural covariance and functional connectivity (FC) can also be used to understand the interactions between brain regions (15). TLE can be conceptualized as a network disorder involving brain regions far beyond the hippocampus (16,17). In TLE patients, voxel-based studies investigating the relationship between hippocampal and extrahippocampal volume loss have shown limbic network pathology that depends on the amount of mesiotemporal damage (18,19). Similarly, a surface-based study using the entorhinal cortex as a seed for cortical thickness covariance analysis showed alterations in limbic networks (20). FC inferred from stereo-electroencephalography (SEEG) offers a direct approach to investigating the functional coupling between the hippocampus and amygdala in TLE (21). Increased high-frequency broadband (HFB, 70–170 Hz) power amplitude in SEEG recordings is an effective index of local neural spiking and is associated with evoked or spontaneous brain activity (22). Such a metric can be used to measure quantitatively the FC properties of brain regions in the interictal state (23). As part of the limbic structure, involvement of the amygdala-hippocampus complex in the initiation and propagation of mTLE seizures has been demonstrated (9). By measuring both structural and FC between the hippocampus and amygdala, we may gain important insights into how these regions interact in TLE and their potential uses in diagnosis.
In this study, our aims were: (I) to quantify structural changes in the hippocampus and amygdala in mTLE; and (II) to understand the interactions between the hippocampus and amygdala using structural and FC analysis.
Methods
Participant selection
From a dataset of patients referred to Beijing Tiantan Hospital and Beijing Fengtai Hospital for the investigation of drug-resistant mTLE between 2015 and 2019, patients were retrospectively selected, using these inclusion criteria: (I) underwent anterior temporal lobectomy (ATL) or selective amygdalohippocampectomy (SAH); (II) resected hippocampal specimen suitable for histological analysis defined using the International League Against Epilepsy (ILAE) HS classification scheme (24); (III) not type III FCD defined using histopathology (e.g., HS with FCD in temporal lobe); (IV) high quality imaging data without motion artefacts, aliasing, or rippling related to eye movement and SEEG recordings without noise where available; (V) no history of dystocia hypoxia, encephalitis, or severe traumatic brain injury; (VI) no intracranial lesions, such as malformations of cortical development, epidermoid cyst, tumor, or vascular malformations; (VII) no encephalomalacia and severe or diffuse brain atrophy; and (VIII) non-reoperation.
All patients underwent preoperative 3.0 T clinical MRI, including at least T1-weighted magnetization prepared rapid acquisition gradient echo (T1-MPGAGE) and T2-FLAIR. All the patients underwent surgery after presurgical evaluation. This evaluation included a comprehensive history, evaluation of electroclinical and neuroimaging data evaluation and, where appropriate, invasive pre-surgical SEEG. Certain clinical information was extracted from patient medical records: age; sex; lateralization of affected hippocampus; age at epilepsy onset; duration of epilepsy; radiological report; and postoperative histology. For subgroup analysis, patient MRI scans were classified as “MRI negative” (no visible abnormalities) or “MRI positive” according to the radiology report. MRI images were typically reviewed by two or more radiologists. The diagnostic criteria included marked hippocampus atrophy, hyperintensity on T2-FLAIR images, and partial loss of internal architecture.
A group of healthy controls with no history of neurological disease also underwent the same MRI procedure. For SEEG FC analysis, the disease control group needed to these criteria: (I) no recorded ictal SEEG onset discharges in mesial temporal lobe structures; (II) lesions detected by presurgical evaluation were not located in the temporal lobe; and (III) intact brain structures. The study was approved by the Ethics Committee of the Beijing Tiantan Hospital and informed consent was obtained in writing from all participants.
MRI acquisition and image processing
MRIs were acquired for all participants using a 3T Siemens Verio scanner with a T1-MPRAGE sequence [repetition time (TR) =2,300 ms, echo time (TE) =2.53 ms, flip angle =12°, slice thickness =1 mm, no gap, voxel size =1 mm × 1 mm × 1 mm] and an axial T2-FLAIR sequence (TR =7,000 ms, TE =80 ms, flip angle =12°, slice thickness =1 mm, no gap, voxel size =1.5 mm × 1.5 mm × 1.5 mm).
The overall analysis pipeline, including structural analysis, FC analysis, and automated detection is shown in Figure 1.
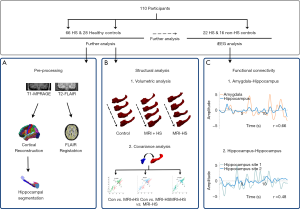
FreeSurfer version 6.0 (https://surfer.nmr.mgh.harvard.edu) was used to create cortical reconstructions (25-27). In brief, the processing involves (I) segmentation of white matter; (II) tessellation of the gray/white matter boundary; (III) inflation of the folded surface tessellation; (IV) automatic correction of topological defects; and (V) FLAIR data was used to improve pial surfaces. These steps have been described in detail elsewhere (6,28). For FLAIR image processing, initially an anatomical segmentation was created for the geometric transfer matrix (GTM), and then linear registration was undertaken to register FLAIR images with the anatomical images. Partial volume correction (PVC) with Muller-Gartner analysis (29) was used to address the partial volume effect and quantify intensity information accurately. Intensity values were normalized by scaling using the intensity in pons, and finally smoothed using a 5-mm FWHM Gaussian diffusion kernel. Automated segmentation of the amygdala and hippocampus was performed with the segmentaHA_T1 command in FreeSurfer (30,31). Preprocessing results of each subject were assessed visually, and any inaccuracies were corrected manually (Figure 1A).
Morphological/intensity features
Normalized hippocampus volume
After automated segmentation of the hippocampus, quality control, and any necessary manual edits, the hippocampal volumes from all participants were obtained using the intrinsic module segmentHA_T1. In addition, to correct for individual variability in intracranial size, the value of the hippocampus volume was normalized using the estimated total intracranial volume (eTIV), obtained from the individual’s intracranial volume and the scaling factor (32).
FLAIR signal intensity of hippocampus
After co-registration of T1 images, intensity normalization, and smoothing, as described above, the value of the normalized FLAIR signal intensity was recorded.
Normalized volume of temporal horn of lateral ventricle
Similar to the normalized hippocampus volume, the volume of the temporal horn of the lateral ventricle was corrected for the eTIV.
Gray-white matter (GM/WM) boundary blurring in the temporal pole
The contrast in intensity between GM and WM was calculated, as blurring of GM/WM boundary in temporal pole is commonly identified in HS (6). The GM/WM signal intensity ratio was computed with the pctsurfcon command in FreeSurfer to the following equation:
[1]
where pct is the mean surface-wise GM/WM contrast, and G is the GM signal intensity sampled at 50% of the cortical thickness above the GM/WM boundary. W is the WM signal intensity sampled 1 mm below the white surface. The more blurred the GM/WM boundary, the closer pct is to zero.
Participants’ left/right hemispheric data were sorted relative to the epileptogenic focus (e.g., ipsi- and contralateral to the focus).
Structural analysis
Patients were divided into three groups: control group, MRI-positive and MRI-negative HS patient groups. Structural analysis consisted of two parts. First, an evaluation of the degree of hippocampal atrophy and amygdala enlargement in MRI-positive and MRI-negative HS patients based on a structural volumetric analysis comparing the hippocampal volume, FLAIR signal intensity, volume of the temporal horn of the lateral ventricle, and GW/WM boundary blurring in the temporal pole of the amygdala and hippocampus between the 3 groups. Additional comparisons were made of morphological characteristics between the affected (ipsilateral) and unaffected (contralateral) hippocampi in HS patients.
Second, structural covariance analysis was undertaken by calculating the linear correlation between normalized volumes of the amygdala and hippocampus. To determine the relative atrophy of the amygdala and hippocampus, we calculated the volumetric ratio of the normalized hippocampus volume divided by the normalized amygdala volume (Figure 1B).
SEEG recordings
SEEG was recorded using a clinical long-term monitoring system (Nihon-Kohden Neurofax EEG-1200, 192 or 256 channels, Tokyo, Japan) with a sampling rate of 1,000 or 2,000 Hz and a bandpass filter of 1.6–300 or 0.08–600 Hz. Each depth electrode (Huake-Hengsheng Medical Technology, Beijing, China) had a diameter of 0.8 mm with 8–16 contacts. The center-to-center spacing of contacts was 3.5 mm. The strategy used for placing electrodes was a clinical decision based on pre-surgical noninvasive information which generated hypotheses concerning the localization of epileptogenic zones. Depth electrodes were placed using a CRW frame-based system (Integra Radionics, Burlington, MA, USA). A postoperative computerized tomography (CT) scan was used to confirm the absence of intracranial bleeding and the accuracy of electrode position. Long-term SEEG monitoring was undertaken for twenty-four hours after electrode implantation in order to record two or more habitual seizures. To reduce distortion during recording, SEEG signals were referenced to the most electrographically silent channel outside of the seizure focus, which was typically a white matter channel.
The SEEG based FC analysis
Patients with depth electrodes covering the amygdala and hippocampus were used for the FC analysis. In each case, five 5 min SEEG segments which were at least six hours before or after ictal discharges during sleep were selected randomly. SEEG segments were exported from NeuroWorkbench (Nihon-Kohden Tokyo, Japan) in edf format and preprocessed through a bespoke MATLAB pipeline that used functions for electrophysiological signal processing from SPM12 (33) and Fieldtrip (34).
Signals were first down-sampled to 1,000 Hz. Next, notch filtering was used to remove the 50 Hz line noise and its harmonic. Then, a bipolar re-referencing was undertaken (35). Next, we calculated the envelope signal of HFB (70–170 Hz) oscillations. The envelope signals were bandpass filtered using a fourth-order Butterworth filter with 0.1–1 Hz frequency threshold. Each segment was split into multiple 15 s epochs. Epochs contaminated by artifacts were excluded. Contacts in the amygdala and hippocampus were identified visually using coregistered presurgical MRI and postsurgical CT images.
The bipolar re-referenced channel, which has two corresponding contacts located within the amygdala or hippocampus, with the highest amplitude, was selected as the representative channel for each anatomical site. The Pearson correlation coefficient of two 0.1–1 Hz filtered envelope signals was used as a surrogate for the strength of FC between two anatomical sites. Pearson correlation coefficients of each epoch were then averaged at an individual level (Figure 1C). The distance between two bipolar channels was defined as the Euclidean distance between the two centers of four contacts.
Statistical analysis
Lilliefors test was used to confirm that all data fitted a normal distribution. Analysis of all participant demographic data and all intergroup analysis was undertaken using Student’s t-test or Mann-Whitney U test, as appropriate. The degree of association in the structural covariance analysis was calculated using a Pearson correlation coefficient (r) or the Spearman rank correlation coefficient. All calculations were undertaken using MATLAB 2018b version software (R2018b, The Mathworks, Natick, MA, USA). Statistical comparison was only undertaken for groups comprising six or more patients. A two-sided P value less than 0.05 was considered statistically significant. A false discovery rate (FDR) correction was used for multiple comparisons.
Results
Demographics
A total of 66 patients with HS confirmed by pathology (32 females, 33 left TLE, mean ± SD age =24.9±8.4 years, mean ± SD duration =12.3±8.0 years, mean ± SD ipsilateral amygdala volume =1702.5±264.7 mm3, mean ± SD ipsilateral hippocampus volume =2,617.5±402.8 mm3), 28 healthy controls (10 females, mean age =16.6±9.9 years, mean bilateral amygdala volume =1,776.9±288.1 mm3, mean bilateral hippocampus volume =3,420.8±476.4 mm3) and 16 epilepsy patients without HS but who had undergone SEEG implantation (7 females, 6 lesions were located in the left hemisphere, mean age =24.0±10.1 years, mean duration =10.6±6.8 years) were included in the study (Table 1).
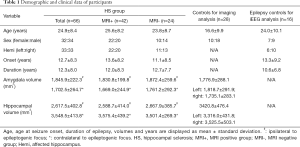
Full table
Twenty-two of the HS patients underwent depth electrode implantation. All such patients had one or more depth electrode implanted in the amygdala and one implanted in the hippocampus. Ten patients had two or more depth electrodes implanted in the hippocampus. Of the 16 disease controls used in the SEEG FC analysis, 10 had one or more electrodes implanted in the amygdala and one in the hippocampus, one had bilateral amygdala and hippocampal implantation, and nine had two or more electrodes implanted in the hippocampus. All the 16 SEEG disease controls had focal resections. Eleven had FCD (2 FCD I, 4 FCD IIa, 5 FCD IIb), one had ganglioglioma (WHO Grade I, GG), and four had gliosis only. Demographic information for these patients is shown in Table 1. The supplementary table 1 (see online: https://cdn.amegroups.cn/static/public/qims-20-624-1.pdf) summarizes the demographic and clinical information for all participants in the cohort.
Morphological/intensity features
The volumes of the amygdala and hippocampus were compared between HS patients and controls. Ipsilateral and contralateral volumes of corresponding anatomical structures were also compared.
In the ipsilateral hippocampus, both MRI-positive and MRI-negative patients had significantly lower normalized volumes than healthy controls (Mann-Whitney U: 7.61, P<0.01; Mann-Whitney U: 6.51, P<0.01, respectively; Figure 2A). However, only MRI-positive HS patients also had increased FLAIR signal intensity in the ipsilateral hippocampus (Student’s t: −2.05, Puncorrected<0.05; Figure 2A).
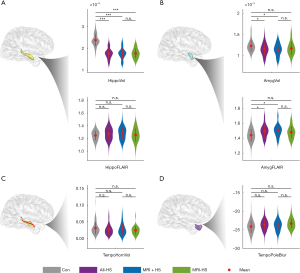
In the ipsilateral amygdala, HS patients had significantly lower normalized volume and higher FLAIR signal intensity (Mann-Whitney U: 2.92, P<0.05; Student’s t: −3.57, P=0.01, respectively; Figure 2B). These changes were mostly observed in MRI-positive HS patients (amygdala volume: Mann-Whitney U: 2.75, P<0.05; FLAIR signal: Student’s t: −3.65, P=0.01, respectively; Figure 2B). There was no significant difference between MRI-negative HS patients and controls (amygdala volume: Mann-Whitney U: 1.93, P>0.05; FLAIR signal: Student’s t: −1.95, P>0.05). There were no inter-group significant differences in normalized volume of the temporal horn of the lateral ventricle and blurring of temporal pole (Figure 2C,D).
Although clear structural differences exist between healthy controls and HS patients, there were no significant quantifiable differences between MRI-positive and MRI-negative patients. In controls, significant differences between the left and right hippocampi were absent for any of the four structural features (Figure S1).
For the hippocampus and amygdala, there were significant differences between the ipsilateral and contralateral sides in HS patients (Figure 3A,B). Ipsilateral hippocampi and the amygdala had decreased normalized volumes (hippocampus: Student’s t: −14.58, P<0.01; amygdala: Student’s t: −4.26, P<0.01) and increased FLAIR signal intensity (hippocampus: Student’s t: −2.24, Puncorrected=0.03; amygdala: Student’s t: 4.44, P<0.01). Whereas, measured values for the contralateral hippocampi and amygdala were similar to those of controls (hippocampal volume: Mann-Whitney U: −0.86, P=0.39; hippocampal FLAIR signal: Student’s t: 0.65, P=0.51; amygdala volume: Mann-Whitney U: −1.16, P=0.24; amygdala FLAIR signal: Student’s t: 0.61, P=0.24, respectively). For the other two features, the normalized volume of the ipsilateral temporal horn was significantly greater when compared to the contralateral side (Student’s t: 2.31, P=0.03) and controls (Mann-Whitney U: 3.32, P<0.01). The temporal pole was significantly less blurred on the contralateral side compared to the ipsilateral side in HS patients (Student’s t: 4.93, P<0.01) and controls (Student’s t: 2.81, P<0.01) (Figure 3C,D). In summary, the hippocampal and amygdala volume and FLAIR intensity were significantly different between ipsilateral and contralateral sides, but the corresponding values of the contralateral side were similar to controls.
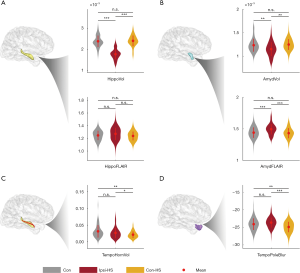
Structural correlation and relative atrophy degree
In the structural covariance analysis, we evaluated the relationship between the normalized hippocampal and amygdala volumes. The Z-score normalized relative amygdala and hippocampal volumes in controls and HS patients were positively correlated: patients with larger hippocampal volumes had larger amygdala volumes (ρSpearman=0.72, P<0.01) (Figure 4A). Volume ratios between hippocampus and amygdala were significantly higher in HS group (Mann-Whitney U: 7.25, P<0.01, Mann-Whitney U: 10.66, P<0.01, respectively; Figure 4A). Subgroup analysis indicated that both MRI-positive and MRI-negative HS groups had a high positive correlation between hippocampal and amygdala volumes. However, their hippocampus/amygdala volumetric ratio did not show a significant difference (Mann-Whitney U: 0.33, P=0.74; Figure 4B). Significant positive correlations were also observed for ipsilateral and contralateral amygdala/hippocampus ratio comparisons in the HS group. Moreover, the volumetric ratio was not different for the contralateral amygdala/hippocampus ratio between contralateral side of HS patients and controls (Mann-Whitney U: 0.23, P=0.82) (Figure 4C).
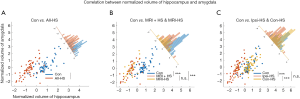
FC analysis between the amygdala and hippocampus
In total, 38 epilepsy patients were included in the SEEG FC analysis. The SEEG cohort comprised 11 MRI-positive HS patients, 11 MRI-negative HS patients, and 16 non-HS disease controls. All patients had depth electrode coverage of the amygdala and hippocampus. SEEG data comprised 5 randomly selected segments (mean ± SD duration time =5.2±1.1 minutes) from a hippocampal and an amygdala contact for each patient during sleep.
The process of intracranial SEEG electrode localization and visualization was undertaken using an established protocol (36) (Figure 5A). For the FC calculation, the mean ± SD distances between channel pairs were comparable between groups. For the electrode pair within the hippocampus, the Euclidean distances of control, MRI + HS, and MRI- HS were 15.9±2.8, 14.9±3.2, 14.4±2.0 mm respectively (one-way ANOVA, P=0.39). For amygdala-hippocampus distances, the distances between groups were 19.1±3.8, 18.8±4.1, 21.0±5.2 mm (one-way ANOVA, P=0.41). In the interictal state, amygdala-hippocampus connectivity was significantly reduced in HS patients (Student’s t: 2.58, P=0.03) and particularly in MRI-negative HS patients (Student’s t: 3.33, P=0.01). There was no statistically significant difference between MRI-positive and MRI-negative subgroups (Student’s t: 1.55, P=0.14). However, connectivity between electrodes within the hippocampus of both MRI-positive and MRI-negative HS patient groups was significantly increased when compared to controls (Student’s t: −2.49, P=0.02) (Figure 5B).
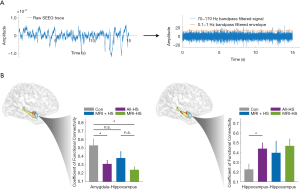
Discussion
Patients with drug-resistant focal epilepsy but without structural abnormalities in MRI are challenging in presurgical evaluation, which is often longer, with a poorer prognosis, an increased risk of neurological complications, and a reduced likelihood of seizure freedom post-operatively (37). HS is among the most common pathologies found in this MRI-negative cohort (38). We sought to improve the presurgical evaluation of HS patients by quantifying structural and connectivity changes characteristic of the condition. We found HS patients, including MRI-negative cases, had structural and functional abnormalities in the ipsilateral mesiotemporal lobe structures. These features might be useful for developing future work developing automatic frameworks to detect and lateralise HS.
The affected hippocampus in TLE patients had significant structural abnormalities when compared to controls, with decreased normalized volume and increased normalized FLAIR signal in both MRI-positive and MRI-negative patients. Only MRI-positive HS patients exhibited temporal pole blurring, while the volume of the temporal horn of the ventricles was the same between groups. In the structural covariance analysis, volumes of the hippocampus and amygdala on the affected side were tightly coupled in HS patients. However, the atrophy was more pronounced in the hippocampus than the amygdala in both MRI-positive and MRI-negative patients. These results demonstrated that at a group level, distinct structural abnormalities existed in both MRI-positive and MRI-negative HS patients. Nevertheless, it is important to recognize that group level differences do not necessarily mean that all individuals have quantifiable hippocampal atrophy. Previous work by Coan et al. found quantifiable hippocampal atrophy in 13% of MRI negative patients (39).
SEEG based FC revealed hypersynchronous electrical activity within the hippocampus and hypoconnectivity between the amygdala and hippocampus in both MRI-positive and MRI-negative HS patients. The amygdala and hippocampus are both allocortical regions that are highly interconnected and often form part of the epileptogenic network (40). Seizures originating from the hippocampus may result in progressive and secondary atrophy of the amygdala. SEEG assesses the FC between amygdala and hippocampus. In this study, amygdala-hippocampal connectivity was decreased, in accordance with previous fMRI hippocampal seed-based analysis (41). This may be due to dysfunction of the epileptogenic hippocampus causing less functional connections to other brain regions, including the amygdala. However, increased intra-hippocampus FC was also evident. This may be as a result of epileptiform activity, such as interictal discharge, within the affected hippocampus (16).
In clinical practice, identification of HS typically relies on hippocampal atrophy in coronal T1 imaging, hyperintensity in T2 or FLAIR imaging, and loss of internal architecture. However, such diagnosis requires experienced neuroradiologists and a proper diagnosis can be missed. In our single center, the detection rate of pathologically confirmed HS using radiology was 63.6% (42/66), with the accuracy rising to 87.9% (58/66) after epilepsy surgery evaluation by a multidisciplinary team that in addition to MRI data analysis, semiology, EEG discharge pattern, PET hypometabolism zone and SEEG data where available. Given the difficulty detecting HS in 36.4% of patients, automated quantification of hippocampal structures might assist in the presurgical clinical evaluation of these patients. However, quantifying individual features is unlikely to greatly improve HS detection, as only a limited proportion of MRI-negative patients will have quantifiable hippocampal atrophy. To circumvent such restrictions, we have pursued a multivariate approach.
Despite a modest sample size, and subtle electrode placement differences in the sEEG analysis, we demonstrate structural and functional changes in patients with temporal lobe epilepsy due to hippocampal sclerosis (HS). These findings provide motivation for future work incorporating these structural and functional features in a multi-variate approach to detect and lateralise HS. Moreover, using a multivariate Mahalanobis distance could improve the robustness of our results, as it is less influenced by measurement noise, assuming this is independent, normally distributed, and orthogonal (42).
Conclusions
Quantitative structural changes in the amygdala and hippocampus occur in both MRI-positive and -negative HS patients. Such changes are accompanied by functional changes including increased functional coupling within the affected hippocampus and decreased coupling between the hippocampus and amygdala.
Acknowledgments
Funding: KZ was funded by the National Natural Science Foundation of China (No. 81771399, 81701276, 82071457) and Beijing Municipal Science & Technology Commission (Z171100001017069). SA was funded by the Rosetrees Trust.
Footnote
Conflicts of Interest: All authors have completed the ICMJE uniform disclosure form (available at http://dx.doi.org/10.21037/qims-20-624). The authors have no conflicts of interest to declare.
Ethical Statement: The study was approved by Ethics Committee of the Beijing Tiantan Hospital. Written informed consent was obtained from patients before publication of this study and similarly for any accompanying images. A copy of the written consent is available for review by the Editor-in-Chief of this journal.
Open Access Statement: This is an Open Access article distributed in accordance with the Creative Commons Attribution-NonCommercial-NoDerivs 4.0 International License (CC BY-NC-ND 4.0), which permits the non-commercial replication and distribution of the article with the strict proviso that no changes or edits are made and the original work is properly cited (including links to both the formal publication through the relevant DOI and the license). See: https://creativecommons.org/licenses/by-nc-nd/4.0/.
References
- Bernasconi A, Bernasconi N, Bernhardt B, Schrader D. Advances in MRI for 'cryptogenic' epilepsies. Nat Rev Neurol 2011;7:99-108. [Crossref] [PubMed]
- Engel J. Mesial temporal lobe epilepsy: what have we learned? Neuroscientist 2001;7:340-52. [Crossref] [PubMed]
- Schwartz TH. MRI-negative temporal lobe epilepsy: is there a role for PET? Epilepsy Curr 2005;5:118-9. [Crossref] [PubMed]
- Immonen A, Jutila L, Muraja-Murro A, Mervaala E, Äikiä M, Lamusuo S, Kuikka J, Vanninen E, Alafuzoff I, Ikonen A, Vanninen R, Vapalahti M, Kälviäinen R. Long-term epilepsy surgery outcomes in patients with MRI-negative temporal lobe epilepsy. Epilepsia 2010;51:2260-9. [Crossref] [PubMed]
- Grewal SS, Alvi MA, Perkins WJ, Cascino GD, Britton JW, Burkholder DB, So E, Shin C, Marsh RW, Meyer FB, Worrell GA, Van Gompel JJ. Reassessing the impact of intraoperative electrocorticography on postoperative outcome of patients undergoing standard temporal lobectomy for MRI-negative temporal lobe epilepsy. J Neurosurg 2019;132:605-14. [Crossref] [PubMed]
- Adler S, Blackwood M, Northam G, Gunny R, Hong S, Bernhardt B, Bernasconi A, Bernasconi N, Jacques T, Tisdall M, Carmichael D, Cross J, Baldeweg T. Multimodal computational neocortical anatomy in pediatric hippocampal sclerosis. Ann Clin Transl Neurol 2018;5:1200-10. [Crossref] [PubMed]
- Bernasconi N, Kinay D, Andermann F, Antel S, Bernasconi A. Analysis of shape and positioning of the hippocampal formation: an MRI study in patients with partial epilepsy and healthy controls. Brain 2005;128:2442-52. [Crossref] [PubMed]
- Whelan CD, Altmann A, Botía JA, Jahanshad N, Hibar DP, Absil J, Alhusaini S, Alvim MKM, Auvinen P, Bartolini E, Bergo FPG, Bernardes T, Blackmon K, Braga B, Caligiuri ME, Calvo A, Carr SJ, Chen J, Chen S, Cherubini A, David P, Domin M, Foley S, França W, Haaker G, Isaev D, Keller SS, Kotikalapudi R, Kowalczyk MA, Kuzniecky R, Langner S, Lenge M, Leyden KM, Liu M, Loi RQ, Martin P, Mascalchi M, Morita ME, Pariente JC, Rodríguez-Cruces R, Rummel C, Saavalainen T, Semmelroch MK, Severino M, Thomas RH, Tondelli M, Tortora D, Vaudano AE, Vivash L, von Podewils F, Wagner J, Weber B, Yao Y, Yasuda CL, Zhang G, Bargalló N, Bender B, Bernasconi N, Bernasconi A, Bernhardt BC, Blümcke I, Carlson C, Cavalleri GL, Cendes F, Concha L, Delanty N, Depondt C, Devinsky O, Doherty CP, Focke NK, Gambardella A, Guerrini R, Hamandi K, Jackson GD, Kälviäinen R, Kochunov P, Kwan P, Labate A, McDonald CR, Meletti S, O'Brien TJ, Ourselin S, Richardson MP, Striano P, Thesen T, Wiest R, Zhang J, Vezzani A, Ryten M, Thompson PM, Sisodiya SM. Structural brain abnormalities in the common epilepsies assessed in a worldwide ENIGMA study. Brain 2018;141:391-408. [Crossref] [PubMed]
- Bartolomei F, Wendling F, Bellanger JJ, Régis J, Chauvel P. Neural networks involving the medial temporal structures in temporal lobe epilepsy. Clin Neurophysiol 2001;112:1746-60. [Crossref] [PubMed]
- Kullmann DM. What's wrong with the amygdala in temporal lobe epilepsy? Brain 2011;134:2800-1. [Crossref] [PubMed]
- Cendes F, Andermann F, Gloor P, Gambardella A, Lopes-Cendes I, Watson C, Evans A, Carpenter S, Olivier A. Relationship between atrophy of the amygdala and ictal fear in temporal lobe epilepsy. Brain 1994;117:739-46. [Crossref] [PubMed]
- Salmenperä T, Kalviainen R, Partanen K, Pitkanen A. Hippocampal and amygdaloid damage in partial epilepsy: a cross-sectional MRI study of 241 patients. Epilepsy Res 2001;46:69-82. [Crossref] [PubMed]
- Lv RJ, Sun ZR, Cui T, Guan HZ, Ren HT, Shao XQ. Temporal lobe epilepsy with amygdala enlargement: a subtype of temporal lobe epilepsy. BMC Neurol 2014;14:194. [Crossref] [PubMed]
- Mitsueda-Ono T, Ikeda A, Tomimoto H, Hanakawa T, Sawamoto N, Fukuyama H, Takahashi R. Amygdalar enlargement in patients with temporal lobe epilepsy. J Neurol Neurosurg Psychiatry 2011;82:652. [Crossref] [PubMed]
- Alexander-Bloch A, Giedd JN, Bullmore E. Imaging structural co-variance between human brain regions. Nat Rev Neurosci 2013;14:322-36. [Crossref] [PubMed]
- Tong X, An D, Xiao F, Lei D, Niu R, Li W, Ren J, Liu W, Tang Y, Zhang L, Zhou B, Gong Q, Zhou D. Real-time effects of interictal spikes on hippocampus and amygdala functional connectivity in unilateral temporal lobe epilepsy: An EEG-fMRI study. Epilepsia 2019;60:246-54. [Crossref] [PubMed]
- Zhao B, Yang B, Tan Z, Hu W, Sang L, Zhang C, Wang X, Wang Y, Liu C, Mo J, Shao X, Zhang J, Zhang K. Intrinsic brain activity changes in temporal lobe epilepsy patients revealed by regional homogeneity analysis. Seizure 2020;81:117-22. [Crossref] [PubMed]
- Düzel E, Schiltz K, Solbach T, Peschel T, Baldeweg T, Kaufmann J, Szentkuti A, Heinze HJ. Hippocampal atrophy in temporal lobe epilepsy is correlated with limbic systems atrophy. J Neurol 2006;253:294-300. [Crossref] [PubMed]
- Bonilha L, Molnar C, Horner MD, Anderson B, Forster L, George MS, Nahas Z. Neurocognitive deficits and prefrontal cortical atrophy in patients with schizophrenia. Schizophr Res 2008;101:142-51. [Crossref] [PubMed]
- Bernhardt BC, Worsley KJ, Besson P, Concha L, Lerch JP, Evans AC, Bernasconi N. Mapping limbic network organization in temporal lobe epilepsy using morphometric correlations: insights on the relation between mesiotemporal connectivity and cortical atrophy. Neuroimage 2008;42:515-24. [Crossref] [PubMed]
- Wang Y, Sinha N, Schroeder G, Ramaraju S, McEvoy A, Miserocchi A, de Tisi J, Chowdhury F, Diehl B, Duncan J, Taylor P. Interictal intracranial electroencephalography for predicting surgical success: The importance of space and time. Epilepsia 2020;61:1417-26. [Crossref] [PubMed]
- Kucyi A, Schrouff J, Bickel S, Foster BL, Shine JM, Parvizi J. Intracranial electrophysiology reveals reproducible intrinsic functional connectivity within human brain networks. J Neurosci 2018;38:4230-42. [Crossref] [PubMed]
- Bartolomei F, Lagarde S, Wendling F, Mcgonigal A, Jirsa V, Guye M, Bénar C. Defining epileptogenic networks: Contribution of SEEG and signal analysis. Epilepsia 2017;58:1131. [Crossref] [PubMed]
- Blümcke I, Thom M, Aronica E, Armstrong DD, Bartolomei F, Bernasconi A, Bernasconi N, Bien CG, Cendes F, Coras R, Cross JH, Jacques TS, Kahane P, Mathern GW, Miyata H, Moshé SL, Oz B, Özkara Ç, Perucca E, Sisodiya S, Wiebe S, Spreafico R. International consensus classification of hippocampal sclerosis in temporal lobe epilepsy: a Task Force report from the ILAE Commission on Diagnostic Methods. Epilepsia 2013;54:1315-29. [Crossref] [PubMed]
- Dale AM, Fischl B, Sereno MI. Cortical Surface-Based Analysis: I. Segmentation and Surface Reconstruction. Neuroimage 1999;9:179. [Crossref] [PubMed]
- Zheng F, Li C, Zhang D, Cui D, Wang Z, Qiu J. Study on the sub-regions volume of hippocampus and amygdala in schizophrenia. Quant Imaging Med Surg 2019;9:1025-36. [Crossref] [PubMed]
- Xu R, Hu X, Jiang X, Zhang Y, Wang J, Zeng X. Longitudinal volume changes of hippocampal subfields and cognitive decline in Parkinson's disease. Quant Imaging Med Surg 2020;10:220-32. [Crossref] [PubMed]
- Viviani R, Stöcker T, Stingl JC. Multimodal FLAIR/MPRAGE segmentation of cerebral cortex and cortical myelin. Neuroimage 2017;152:130-41. [Crossref] [PubMed]
- Greve DN, Salat DH, Bowen SL, Izquierdo-Garcia D, Schultz AP, Catana C, Becker JA, Svarer C, Knudsen GM, Sperling RA. Different partial volume correction methods lead to different conclusions: An (18)F-FDG-PET study of aging. Neuroimage 2016;132:334-43. [Crossref] [PubMed]
- Iglesias JE, Augustinack JC, Nguyen K, Player CM, Player A, Wright M, Roy N, Frosch MP, Mckee AC, Wald LL. A computational atlas of the hippocampal formation using ex vivo, ultra-high resolution MRI: Application to adaptive segmentation of in vivo MRI. Neuroimage 2015;115:117-37. [Crossref] [PubMed]
- Saygin ZM, Kliemann D, Iglesias JE, Ajw VDK, Boyd E, Reuter M, Stevens A, Van LK, Mckee A, Frosch MP. High-resolution magnetic resonance imaging reveals nuclei of the human amygdala: manual segmentation to automatic atlas. Neuroimage 2017;155:370. [Crossref] [PubMed]
- Buckner RL, Head D, Parker J, Fotenos AF, Marcus D, Morris JC, Snyder AZ. A unified approach for morphometric and functional data analysis in young, old, and demented adults using automated atlas-based head size normalization: reliability and validation against manual measurement of total intracranial volume. Neuroimage 2004;23:724-38. [Crossref] [PubMed]
- Ashburner J. SPM: A history. Neuroimage 2012;62-248:791-800.
- Oostenveld R, Fries P, Maris E, Schoffelen JM. FieldTrip: Open Source Software for Advanced Analysis of MEG, EEG, and Invasive Electrophysiological Data. Comput Intell Neurosci 2011;2011:156869. [Crossref] [PubMed]
- David O, Blauwblomme T, Job AS, Chabardès S, Hoffmann D, Minotti L, Kahane P. Imaging the seizure onset zone with stereo-electroencephalography. Brain 2011;134:2898-911. [Crossref] [PubMed]
- Hamilton LS, Chang DL, Lee MB, Chang EF. Semi-automated Anatomical Labeling and Inter-subject Warping of High-Density Intracranial Recording Electrodes in Electrocorticography. Front Neuroinform 2017;11:62. [Crossref] [PubMed]
- Malmgren K, Krysl D. Epilepsy: Long-term outcomes in MRI-negative patients with epilepsy. Nat Rev Neurol 2017;13:132-3. [Crossref] [PubMed]
- Wang ZI, Alexopoulos AV, Jones SE, Jaisani Z, Najm IM, Prayson RA. The pathology of magnetic-resonance-imaging-negative epilepsy. Mod Pathol 2013;26:1051-8. [Crossref] [PubMed]
- Coan AC, Kubota B, Bergo FP, Campos BM, Cendes F. 3T MRI quantification of hippocampal volume and signal in mesial temporal lobe epilepsy improves detection of hippocampal sclerosis. AJNR Am J Neuroradiol 2014;35:77-83. [Crossref] [PubMed]
- Mégevand P, Groppe D, Bickel S, Mercier M, Goldfinger M, Keller C, Entz L, Mehta A. The Hippocampus and Amygdala Are Integrators of Neocortical Influence: A CorticoCortical Evoked Potential Study. Brain Connectivity 2017;7:648-60. [Crossref] [PubMed]
- Laufs H, Rodionov R, Thornton R, Duncan J, Lemieux L, Tagliazucchi E. Altered FMRI connectivity dynamics in temporal lobe epilepsy might explain seizure semiology. Front Neurol 2014;5:175. [Crossref] [PubMed]
- Taylor PN, Moreira da Silva N, Blamire A, Wang Y, Forsyth R. EEarly deviation from normal structural connectivity: A novel intrinsic severity score for mild TBI. Neurology 2020;94:e1021-6.