Establishment and verification of a prediction model based on clinical characteristics and positron emission tomography/computed tomography (PET/CT) parameters for distinguishing malignant from benign ground-glass nodules
Introduction
In recent years, with the popularity of low-dose computed tomography (CT) in lung cancer screening, the detection rate of ground-glass nodules (GGNs) has gradually increased. GGNs are divided into mixed GGN (mGGN) and pure GGN (pGGN) according to the presence of solid ingredients in the nodules. They are markedly different from solid lung nodules in terms of their biological behavior, and have a higher probability of malignancy than solid nodules (34% vs. 7%) (1,2). GGNs are common in early-stage lung adenocarcinoma but can also be caused by inflammation, interstitial fibrosis, or local hemorrhage (3,4). Due to the small number of cells within a GGN and the difficulty of obtaining material, bronchoscopy or percutaneous lung biopsy present significant limitations for the diagnosis of GGN. Furthermore, they are invasive examinations, and are often accompanied by complications such as pneumothorax and hemorrhage (5). Therefore, imaging remains the primary method to characterize GGNs.
The Fleischner Association Guidelines 2017 (6) recommend that GGN detection should be planned during CT follow-up to determine whether GGNs persist based on the dynamic changes of the nodules. Nodules that are infectious or inflammatory lesions typically reduce in volume or disappear during follow-up. Long-term CT follow-up can cause severe anxiety and repeated radiation exposure for patients, so it is difficult for some patients to accept. Therefore, more efficient imaging techniques are needed to distinguish malignant from benign GGNs. GGNs have different morphologies and diverse imaging manifestations. Recently, it was found that early-stage lung adenocarcinoma with GGNs has a higher incidence in female and non-smoking populations (7), which poses a significant challenge for clinical and imaging physicians.
Several studies (8) have reported that a single CT morphological feature or quantitative parameter is not sufficient for the accurate differential diagnosis of GGNs, and thus a multivariate prediction model is likely to improve diagnostic performance. Previous prediction models for distinguishing benign from malignant lung nodules include the Mayo (9), Veterans Affairs (VA) (10), Brock (11), Herder (12), and Bayesian Inference Malignancy Calculator (BIMC) (13) models. The Mayo and VA models, which were developed based on solid nodules, suggest that age and smoking history are independent risk factors that increase the probability of malignant pulmonary tumors. However, this conclusion is contradicted by the epidemiological characteristics of GGNs. Considering the uniqueness of GGNs, the Brock model adjusted the correlation coefficient according to the nodule type. However, this model is complicated to use, and its derivation data are obtained from primary screening patients with a low malignancy rate (5.5%). Currently, few models are dedicated to GGN prediction.
Our previous study found that early lung adenocarcinoma showed low fluorodeoxyglucose (FDG) uptake on positron emission tomography (PET)/CT imaging (14,15), which is consistent with the results from other groups (4). Moreover, benign GGNs can also lead to false-positive findings due to their high FDG uptake. Scott et al. (16) and Chun et al. (17) indicated that the FDG uptake of benign GGN was significantly higher than that of malignant GGNs. Therefore, it is likely that the glucose metabolism of GGNs is different from that of solid lung nodules.
In this study, we aimed to build a prediction model based on 18F-FDG PET/CT imaging that took both functional metabolism and CT imaging characteristics into account in order to distinguish malignant from benign GGNs.
Methods
Clinical data
In this single-center retrospective study, we screened a total of 228 patients with GGNs who underwent 18F-FDG PET/CT and high-resolution CT (HRCT) examination in our hospital from November 2011 to December 2019. This study was approved by the institutional ethics committee for retrospective analysis {no. [2018] KD 013}, and the requirement for written informed consent was waived. The inclusion criteria for patients were as follows: (I) underwent PET/CT and HRCT examination; (II) definitive diagnosis through surgery within 1 month after PET/CT examination or by volume reduction during CT follow-up for benign GGNs; and (III) a maximum GGN diameter of ≤30 mm. Patients were excluded based on the following criteria: (I) presence of malignant lesions (stage IB or higher) based on the 8th version of lung cancer tumor node metastasis (TNM) staging pathology standard; (II) poor image quality or GGNs that were difficult to measure; (III) a history of malignant tumors in the previous 5 years; and (IV) severe liver disease or diabetes. In total, 170 patients met the eligibility criteria, and 58 patients were excluded. The data of the included patients were collected by reviewing the cases or follow-up via phone calls. The patient selection process is shown in Figure 1.
Image collection
A Biograph mCT [64] type PET/CT scanner (Siemens, Erlangen, Germany) was used for whole-body imaging. The patients fasted for 4–6 hours before imaging, with blood glucose ≤11 mmol/L. 18F-FDG was then injected intravenously at approximately 4.44 MBq/kg for about 60 minutes prior to PET/CT imaging. A low-dose localized CT scan was performed first, followed by a whole-body PET acquisition, with 2 min/bed. The reconstruction method was performed as follows: TrueX + time-of-flight (TOF) (ultraHD-PET) with two iterations and 21 subsets, and Gaussian filtering with a full-width at a half maximum of 2.0 mm; matrix (pixels) 200×200, zoom 1.00, and a 3D image acquisition mode. Respiratory gating was not used. The image was evaluated using TrueD software (Siemens, Germany).
Following the PET/CT scan, we immediately performed a breath-holding HRCT scan of the lung nodule with the following settings: tube voltage 140 kV, tube current automatically adjusted according to human anatomy, tissue density using the automatic exposure control (CARE Dose 4D, Siemens Healthcare, Germany), rotation time 0.5 s, thread pitch 0.6, layer thickness 1.0 mm, layer interval 0.5 mm, matrix 512×512, lung window (window width: 1,200 HU, window level: −600 HU), and mediastinum window (window width: 350 HU, window level: 40 HU).
Image analysis
Imaging features were collected and reviewed separately by two experienced nuclear medicine physicians with more than 7 years of experience (R Niu, X Shao). Cases of inconsistent opinions were resolved by discussion and consensus. PET image parameters included following: the maximum standardized uptake value (SUVmax) of the pulmonary nodule, liver SUVmean, and the SUV index (the ratio of nodule SUVmax to liver SUVmean). A more detailed description of the measurement methods can be found in our previous study (14).
CT image parameters included nodule number (solitary, multifocal), nodule type (pGGN, mGGN), location (peripheral, center), shape (round/oval, irregular), margin (smooth, lobulated), bronchial sign, vacuole sign, pleural indentation, vessel convergence sign, DGGN (maximum diameter of the nodules), Dsolid (maximum diameter of the solid components), CTR (ratio of Dsolid to DGGN), CTGGO (average attenuation value of the ground glass components), CTLP (average attenuation value of the normal lung parenchyma around the nodule), and ΔCTGGO-LP (difference between CTGGO and CTLP). The above parameters were measured under 3–8 times magnification, and all of the data were averaged from the measurements of the two physicians.
Statistical analysis
Continuous variables are expressed as mean ± standard deviation (SD) when they were normally distributed; otherwise they are expressed as P50 (P25–P75). Categorical variables are expressed as a frequency (%). For comparison between groups, continuous variables were analyzed using the unpaired Student’s t-test or the Mann-Whitney U nonparametric test, and categorical variables were tested using the Pearson chi-square test or Fisher’s exact test. The intraclass correlation coefficient (ICC) was used to analyze the consistency of the measurements between the two observers.
The splitSample function automatically divided the original dataset into a derivation set and validation set at a ratio of 1:1. Multivariate logistic regression was used to establish the prediction model in the derivation set. The variable introduction standard was P<0.3. The independent variable was screened by collinearity (variation inflation factor, VIF), and the elimination criterion was VIF >10. The minimum Akaike’s information criterion (AIC) was used to select the optimal model parameters and calculate the odds ratio (OR) and 95% confidence interval (CI). We also plotted the nomogram of the prediction model, which could visually display the prediction results of each GGN, and a calibration curve was also plotted to show the prediction accuracy of the nomogram. A receiver operating characteristic (ROC) curve of the prediction model was plotted, and the area under the curve (AUC) and its 95% CI were calculated. The AUCs of the derivation and validation sets were compared using z-statistics and Hanley and McNeil programs (18). All of the statistical analyses were performed using R3.4.3 (http://www.R-project.org; software packages: glmnet, pROC, rms). All statistical tests were two-sided, and P value <0.05 was considered statistically significant.
Results
Study populations
This study included 170 patients with GGNs, including 56 males and 114 females, with an average age of 60.7±9.2 years (ranging from 31 to 81 years). Among these patients, 157 (92.4%) underwent video-assisted thoracic surgery (VATS), 11 (6.5%) underwent thoracotomy, and 2 (1.2%) were confirmed to have benign GGNs during CT follow-up. The patients were divided into a benign group (21 cases) and an adenocarcinoma group (149 cases).
Among the 170 patients, 113 had a solitary GGN, and 57 had multifocal GGNs (with a median number of 4, ranging from 2 to 50). A total of 507 GGNs were detected, and 197 GGNs were confirmed via postoperative pathological examination or clinical follow-up. Owing to the stepwise protocol for the treatment of multifocal GGNs (6,19), 310 GGNs without a definite diagnosis by pathological examination or follow-up were excluded from the analysis because of the lack of information on the radiological-pathological correlation. There were 27 GGNs in the benign group, including 4 fungal infections, 3 granulomatous inflammations, 2 organizing pneumonias, 1 sclerosing alveolar cell tumor, 1 specific interstitial pneumonia, 1 pulmonary alveoli epithelium bronchial metaplasia, and 15 other inflammatory lesions. There were 170 GGNs in the adenocarcinoma group, including 5 atypical adenomatous hyperplasia (AAH), 5 in situ carcinomas (AIS), 17 microinvasive adenocarcinomas (MIA), and 143 invasive adenocarcinomas (IAC). The pathological classification of the adenocarcinoma group followed the latest classification standards for lung adenocarcinoma from 2011, which were amended by the International Association for Oncology/American Thoracic Society/European Respiratory Society (20). Patients with multiple nodules had the same etiology.
The comparison of clinical characteristics between the two groups is shown in Table 1. The proportion of females in the adenocarcinoma group was significantly higher than that in the benign group (P=0.012). There were no significant differences in age, smoking history, fasting blood glucose, ratio of multifocal GGNs, GGN number, and tumor markers [carcinoembryonic antigen (CEA), cytokeratin 19 fragments (CYFRA21-1), carbohydrate antigen (CA) 199, neonatal status epilepticus (NSE), squamous cell carcinoma antigen (SCCAg)] between the two groups (all P>0.05).
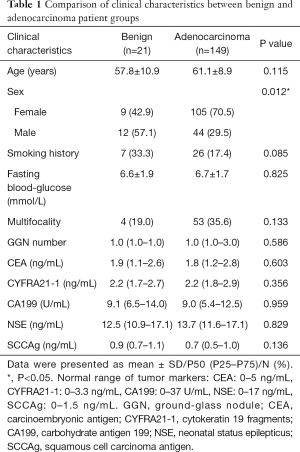
Full table
Comparison of baseline data between derivation and validation sets
The 197 GGNs were randomly divided into a derivation set (n=97) and a verification set (n=100) at a 1: 1 ratio by statistical software. The comparison of baseline data between the two sets is shown in Table 2. The clinical data and PET/CT image features were not significantly different between the derivation and verification sets (all P>0.05). The two observers exhibited perfect consistency in the results of PET/CT and HRCT parameter measurement (ICC: 0.908–0.999, all P<0.001).
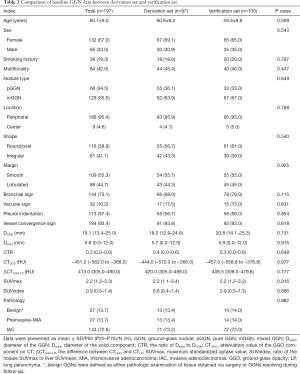
Full table
Univariate analysis of benign and malignant GGNs in the derivation set
We performed univariate logistic analysis on benign and malignant GGNs in the derivation set. As shown in Table 3, there were notable differences in sex, nodule type, SUVmax, and SUV index between benign and malignant GGNs (all P<0.05). In contrast, no significant differences were observed in age, smoking history, multifocal, location, shape, margin, bronchial sign, vacuole sign, pleural indentation, vessel convergence sign, DGGN, Dsolid, CTR, CTGGO, and ΔCTGGO-LP between benign and malignant GGNs (all P>0.05).
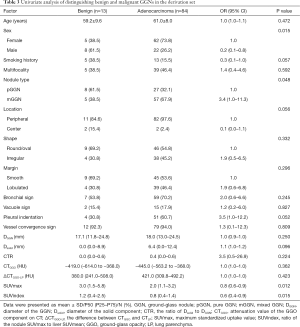
Full table
The ROC curves of the quantitative indicators including DGGN, Dsolid, CTR, CTGGO, ΔCTGGO-LP, SUVmax, and SUV-index were plotted separately, with AUCs of 0.485 (95% CI: 0.289–0.682; P=0.884), 0.654 (95% CI: 0.500–0.809; P=0.051), 0.600 (95% CI: 0.417–0.783; P=0.285), 0.458 (95% CI: 0.261–0.655; P=0.678), 0.549 (95% CI: 0.343–0.754; P=0.644), 0.631 (95% CI: 0.443–0.820; P=0.158), and 0.606 (95% CI: 0.409–0.803; P=0.276), respectively. These results demonstrated that no single quantitative indicator could effectively distinguish between benign and malignant GGNs (all P>0.05).
Development and verification of the PET/CT multivariate model
Using malignant GGNs as the dependent variable, and the 19 aforementioned indices as independent variables, we performed a binary multivariate logistic regression analysis to establish a regression model. With this model, we obtained the independent factors for identifying malignant GGNs, ORs, and 95% CIs (Table 4). From the analysis, we found that sex, nodule location, margin, pleural indentation, and SUV index were all independent factors for predicting malignant GGNs.
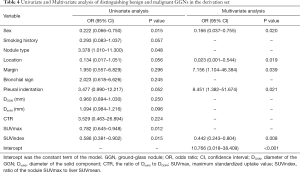
Full table
The formula of the prediction model was as follows: Logit (P) = 2.376 − 1.795 × (female = 0, male = 1) – 3.758 × (peripheral = 0, center = 1) + 1.968 × (smooth = 0, lobulated = 1) + 2.134 × (pleural indentation) – 0.816 × SUV index, where P denotes probability.
The ROC curve of the prediction model in the derivation set was plotted; the AUC was 0.875 (95% CI: 0.773–0.976; P<0.001), with a sensitivity of 0.702 and a specificity of 0.923. The positive likelihood ratio was 9.131, and the negative likelihood ratio was 0.322. The ROC curve of the prediction model in the verification set was also plotted; the AUC was 0.874 (95% CI: 0.770–0.977; P<0.001), with a sensitivity of 0.826, and a specificity of 0.857. The positive likelihood ratio was 5.779, and the negative likelihood ratio was 0.204. The AUC of the derivation set and the validation set were very similar (0.875 vs. 0.874), and the difference between them was not statistically significant (P=0.989) (Figure 2).
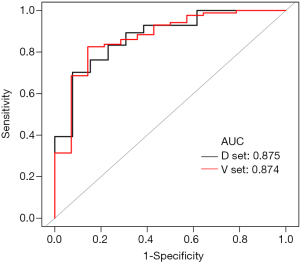
We also plotted the nomogram (Figure 3A) and a calibration curve (Figure 3B,C) of the PET/CT prediction model to identify benign and malignant GGNs. The calibration curve displayed the predicted and observed values of the prediction model in the derivation and verification sets, which had a good consistency.
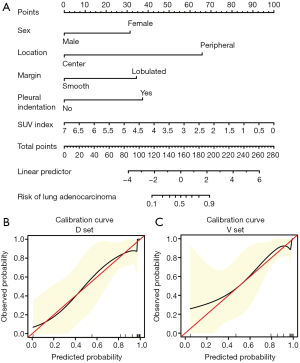
Next, we further divided the adenocarcinoma group into a preinvasive-MIA group and an IAC group based on the degree of invasion. We analyzed the identification power of the prediction model between the benign group and these two adenocarcinoma subgroups. The results showed that, for benign GGN and preinvasive-MIA, the AUC was 0.876 (95% CI: 0.740–1.000; P<0.001), with a sensitivity of 0.923, and a specificity of 0.769. The positive likelihood ratio was 4.000, and the negative likelihood ratio was 0.100. For benign GGNs and IAC, the AUC was 0.874 (95% CI: 0.771–0.978; P<0.001), with a sensitivity of 0.732, and a specificity of 0.923. The positive likelihood ratio was 9.521, and the negative likelihood ratio was 0.290. After ROC analysis and verification (Figure 4A,B), the efficacy of the model in distinguishing benign from preinvasive MIA was not markedly different between the derivation and verification sets (AUC: 0.876 vs. 0.827, z=0.458, P=0.647). Similarly, the efficacy of the model in distinguishing benign GGNs from IAC was also not significantly different between the two sets (AUC: 0.874 vs. 0.883, z=–0.127, P=0.899).
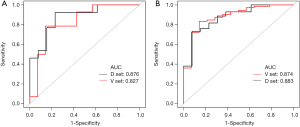
Moreover, in the derivation set, the AUC of distinguishing benign from preinvasive MIA was similar to the AUC of distinguishing benign from IAC (0.876 vs. 0.874, z=0.023, P=0.981), and these two AUCs were similar to the AUC of distinguishing the benign group from the whole adenocarcinoma group (z was 0.012 and –0.014, respectively; P was 0.991 and 0.989, respectively).
Discussion
The identification of benign and malignant GGNs has garnered increase attention in clinical practice in recent years, yet there is still no unified and recognized effective imaging method (21). This study retrospectively analyzed the clinical and imaging data of 197 GGNs and developed a prediction model for distinguishing benign from malignant GGNs based on 18F-FDG PET/CT. This model was verified to be feasible and straightforward, and the diagnostic efficacy was relatively high (AUC =0.875, 95% CI: 0.773–0.976).
The univariate analysis showed that the proportion of female patients in the adenocarcinoma group was higher than that in the benign group, and sex (female) was also found to be an independent risk factor for malignant GGNs in the multivariate analysis. The probability of malignant GGNs in females was six times higher than that in males, which is consistent with the results reported by Zheng et al. (22). We also found that the proportion of mGGN in the adenocarcinoma group was significantly higher than that in the benign group. However, this parameter was not included in the prediction model, which may be attributable to the overlap in the imaging manifestation of solid components between benign and malignant GGNs, and thus mGGN is not unique to IAC. It is more likely that mGGN correlates with another feature with stronger discriminatory power and so it was not retained. In this study, we also found that the proportion of smoking patients in the benign group was higher than that in the adenocarcinoma group, which may be related to the higher proportion of males in the benign group; however, no significant effect of smoking status was observed. Moreover, there was no notable difference in the tumor markers (serum) between the benign and adenocarcinoma groups, which resulted in low sensitivity, suggesting that these markers have limited identification value for GGNs. This result might be attributable to the fact that patients with malignant GGNs were mostly in the early stage of lung cancer, when tumor markers had not yet been released into peripheral blood, or were at low concentrations (23). The above results further confirmed that GGN-based lung tumors are different from traditional lung cancer and have different epidemiological, clinical, and biological characteristics.
Also, nodule location was included in the model as an independent factor, suggesting that a GGN located peripherally or subpleurally is more likely to be malignant than one located in the central lung parenchyma, which is consistent with previous reports (24). Lobulation and pleural indentation were also common features of malignant GGNs (25,26) and were included in the model as CT morphological parameters, making the model more reliable. The PET metabolic parameter, SUV index, is also a helpful factor in the model, with higher SUV index values indicating a greater likelihood that the GGN is benign. This may be due to the fact that the benign group in this study mainly consisted of inflammatory lesions, and 18F-FDG, as a glucose analog imaging agent, accumulates both in tumor and inflammatory tissue. When inflammation occurs, vascular permeability increases and inflammatory cells accumulate, with an increase in glucose utilization by cells. Numerous lung diseases, such as tuberculosis, cryptococcal infection, and organizing pneumonia can show abnormally high imaging agent uptake (27,28). On the other hand, GGN lung adenocarcinoma has a lower degree of nuclear abnormality, fewer tumor cells, a longer doubling time, a lower metabolism rate, and less 18F FDG uptake, which is consistent with previous reports by Scott et al. and Chang et al. (16,29). In our study, the SUVmax was not included in the model, likely because the SUV index (SUVmax of lesion/SUVmean of liver) is strongly correlated with SUVmax. Thus, the information contained within the SUVmax was incorporated into the model in its normalized form (normalized by liver uptake), and not in its raw form.
Models for identifying benign and malignant solitary lung nodules are not uncommon, yet only the Herder and BIMC models are widely accepted and include 18F-FDG metabolic factors. The morphological parameters in the Herder model come from chest radiographs or thick-slice CT, and some cases lack pathological results. The image parameters of our model came from HRCT, which had a more accurate analysis of nodular features, and 99% of the GGNs had pathological diagnosis. Meanwhile, the BIMC model includes factors such as the degree of tumor enhancement; however, for GGNs with limited or no solid component, enhanced CT offers no advantages. The above models are all based on solid nodules and on an increased probability of malignancy with increasing SUV values. However, in GGNs, we observed the opposite. A prospective study by Nomori et al. (30) showed that the biological metabolic activity of GGNs were significantly different from that of solid nodules. They found that 90% of malignant GGNs were false negative on FDG PET images and 80% of benign nodules (focal pneumonia) were false positive. The sensitivity and specificity for GGNs were 10% and 20%, respectively, and the sensitivity and specificity for solid nodules were 90% and 71%, respectively (P<0.001), which confirmed that the solid nodule prediction model did not work effectively. Another study showed that glucose transporters, which facilitate the diffusion of 18F-FDG into cancer cells, are not overexpressed in early-stage lung adenocarcinoma. However, sodium glucose transporter 2, which does not transport 18F-FDG, is present in early-stage carcinoma (31). This may be the molecular cause for the poor performance, and even the reverse association (higher SUVmax; lower probability of malignancy) of 18F-FDG in early-stage lung adenocarcinoma.
Also, the present study plotted a nomogram of the prediction model that combined clinical, PET, and CT information, which may be superior to using advanced CT alone. The calibration curve showed that the model had good prediction accuracy in both the derivation and verification sets. Following stratification of the adenocarcinoma group according to the degree of invasiveness, the model was still robust. Moreover, as the degree of invasiveness (malignancy) increased, the robustness of the model was also increased, which may be related to the more typical malignant characteristics of IAC.
This study also has some limitations that should be noted: (I) the included cases were patients with clinically suspected malignant GGNs, so the proportion and sample size of the benign group was small, which may have resulted in selection bias; (II) although we conducted preliminary verification of the model, this study was a single-center retrospective study, and thus it is still necessary to conduct a multicenter study to expand the sample size further, optimize the prediction model, and perform external verification; (III) this model may be suitable for the differential diagnosis of patients with clinically suspected malignant GGNs, but it is not suitable for screening; and (IV) this study preliminarily demonstrated the potential of the radiomics model; in the future, machine learning or deep learning models should be established in order to improve the predictive performance.
Conclusions
In summary, this study developed and validated a prediction model based on 18F-FDG PET/CT imaging for distinguishing malignant from benign GGNs. Sex, nodule location, margin, pleural indentation, and SUV index were independent factors for predicting malignant GGNs. This model considered the clinical characteristics of patients, CT morphological parameters, and PET metabolic parameters. It showed good diagnostic efficacy and high specificity, which can reduce the misdiagnosis of benign GGNs, avoid unnecessary surgery, and improve the preoperative diagnosis of high-risk GGNs.
Acknowledgments
Funding: Young Talent Development Plan of Changzhou Health Commission (CZQM2020012); Key Laboratory of Changzhou High-tech Research Project (Grant No. CM20193010); Changzhou Sci&Tech Program (Grant No. CJ20180022).
Footnote
Conflicts of Interest: All authors have completed the ICMJE uniform disclosure form (available at http://dx.doi.org/10.21037/qims-20-840). The authors have no conflicts of interest to declare.
Ethical Statement: This study was approved by the institutional ethics committee for retrospective analysis {[2018] KD 013} and did not require informed consent.
Open Access Statement: This is an Open Access article distributed in accordance with the Creative Commons Attribution-NonCommercial-NoDerivs 4.0 International License (CC BY-NC-ND 4.0), which permits the non-commercial replication and distribution of the article with the strict proviso that no changes or edits are made and the original work is properly cited (including links to both the formal publication through the relevant DOI and the license). See: https://creativecommons.org/licenses/by-nc-nd/4.0/.
References
- Henschke CI, Yankelevitz DF, Mirtcheva R, McGuinness G, McCauley D, Miettinen OS. CT screening for lung cancer: frequency and significance of part-solid and nonsolid nodules. AJR Am J Roentgenol 2002;178:1053-7. [Crossref] [PubMed]
- Moon Y, Sung SW, Lee KY, Park JK. Clinicopathological characteristics and prognosis of non-lepidic invasive adenocarcinoma presenting as ground glass opacity nodule. J Thorac Dis 2016;8:2562-70. [Crossref] [PubMed]
- Fan L, Fang M, Li Z, Tu W, Wang S, Chen W, Tian J, Dong D, Liu S. Radiomics signature: a biomarker for the preoperative discrimination of lung invasive adenocarcinoma manifesting as a ground-glass nodule. Eur Radiol 2019;29:889-97. [Crossref] [PubMed]
- Cha MJ, Lee KS, Kim HS, Lee SW, Jeong CJ, Kim EY, Lee HY. Improvement in imaging diagnosis technique and modalities for solitary pulmonary nodules: from ground-glass opacity nodules to part-solid and solid nodules. Expert Rev Respir Med 2016;10:261-78. [Crossref] [PubMed]
- Zhu J, Qu Y, Wang X, Jiang C, Mo J, Xi J, Wen Z. Risk factors associated with pulmonary hemorrhage and hemoptysis following percutaneous CT-guided transthoracic lung core needle biopsy: a retrospective study of 1,090 cases. Quant Imaging Med Surg 2020;10:1008-20. [Crossref] [PubMed]
- MacMahon H, Naidich DP, Goo JM, Lee KS, Leung ANC, Mayo JR, Mehta AC, Ohno Y, Powell CA, Prokop M, Rubin GD, Schaefer-Prokop CM, Travis WD, Van Schil PE, Bankier AA. Guidelines for Management of Incidental Pulmonary Nodules Detected on CT Images: From the Fleischner Society 2017. Radiology 2017;284:228-43. [Crossref] [PubMed]
- Kim TJ, Goo JM, Lee KW, Park CM, Lee HJ. Clinical, pathological and thin-section CT features of persistent multiple ground-glass opacity nodules: comparison with solitary ground-glass opacity nodule. Lung Cancer 2009;64:171-8. [Crossref] [PubMed]
- Dai J, Yu G, Yu J. Can CT imaging features of ground-glass opacity predict invasiveness? A meta-analysis. Thorac Cancer 2018;9:452-8. [Crossref] [PubMed]
- Swensen SJ, Silverstein MD, Ilstrup DM, Schleck CD, Edell ES. The probability of malignancy in solitary pulmonary nodules. Application to small radiologically indeterminate nodules. Arch Intern Med 1997;157:849-55. [Crossref] [PubMed]
- Gould MK, Ananth L, Barnett PG. A clinical model to estimate the pretest probability of lung cancer in patients with solitary pulmonary nodules. Chest 2007;131:383-8. [Crossref] [PubMed]
- Baldwin DR. Management of pulmonary nodules according to the 2015 British Thoracic Society guidelines. Key messages for clinical practice. Pol Arch Med Wewn 2016;126:262-74. [Crossref] [PubMed]
- Herder GJ, van Tinteren H, Golding RP, Kostense PJ, Comans EF, Smit EF, Hoekstra OS. Clinical prediction model to characterize pulmonary nodules: validation and added value of 18F-fluorodeoxyglucose positron emission tomography. Chest 2005;128:2490-6. [Crossref] [PubMed]
- Soardi GA, Perandini S, Motton M, Montemezzi S. Assessing probability of malignancy in solid solitary pulmonary nodules with a new Bayesian calculator: improving diagnostic accuracy by means of expanded and updated features. Eur Radiol 2015;25:155-62. [Crossref] [PubMed]
- Niu R, Shao X, Shao X, Wang J, Jiang Z, Wang Y. Lung Adenocarcinoma Manifesting as Ground-Glass Opacity Nodules 3 cm or Smaller: Evaluation With Combined High-Resolution CT and PET/CT Modality. AJR Am J Roentgenol 2019;213:W236-W245. [Crossref] [PubMed]
- Shao X, Niu R, Jiang Z, Shao X, Wang Y. Role of PET/CT in Management of Early Lung Adenocarcinoma. AJR Am J Roentgenol 2020;214:437-45. [Crossref] [PubMed]
- Scott JA, McDermott S, Kilcoyne A, Wang Y, Halpern EF, Ackman JB. Comparison of (18)F-FDG avidity at PET of benign and malignant pure ground-glass opacities: a paradox? Part II: artificial neural network integration of the PET/CT characteristics of ground-glass opacities to predict their likelihood of malignancy. Clin Radiol 2019;74:692-6. [Crossref] [PubMed]
- Chun EJ, Lee HJ, Kang WJ, Kim KG, Goo JM, Park CM, Lee CH. Differentiation between malignancy and inflammation in pulmonary ground-glass nodules: The feasibility of integrated (18)F-FDG PET/CT. Lung Cancer 2009;65:180-6. [Crossref] [PubMed]
- Hanley JA, McNeil BJ. The meaning and use of the area under a receiver operating characteristic (ROC) curve. Radiology 1982;143:29-36. [Crossref] [PubMed]
- Suzuki K. Whack-a-mole strategy for multifocal ground glass opacities of the lung. J Thorac Dis 2017;9:S201-S207. [Crossref] [PubMed]
- Travis WD, Brambilla E, Noguchi M, Nicholson AG, Geisinger KR, Yatabe Y, Beer DG, Powell CA, Riely GJ, Van Schil PE, Garg K, Austin JH, Asamura H, Rusch VW, Hirsch FR, Scagliotti G, Mitsudomi T, Huber RM, Ishikawa Y, Jett J, Sanchez-Cespedes M, Sculier JP, Takahashi T, Tsuboi M, Vansteenkiste J, Wistuba I, Yang PC, Aberle D, Brambilla C, Flieder D, Franklin W, Gazdar A, Gould M, Hasleton P, Henderson D, Johnson B, Johnson D, Kerr K, Kuriyama K, Lee JS, Miller VA, Petersen I, Roggli V, Rosell R, Saijo N, Thunnissen E, Tsao M, Yankelewitz D. International association for the study of lung cancer/american thoracic society/european respiratory society international multidisciplinary classification of lung adenocarcinoma. J Thorac Oncol 2011;6:244-85. [Crossref] [PubMed]
- Wilson DO, de Torres JP. Lung cancer screening: how do we make it better? Quant Imaging Med Surg 2020;10:533-6. [Crossref] [PubMed]
- Zheng B, Zhou X, Chen J, Zheng W, Duan Q, Chen C. A Modified Model for Preoperatively Predicting Malignancy of Solitary Pulmonary Nodules: An Asia Cohort Study. Ann Thorac Surg 2015;100:288-94. [Crossref] [PubMed]
- Cho JY, Sung HJ. Proteomic approaches in lung cancer biomarker development. Expert Rev Proteomics 2009;6:27-42. [Crossref] [PubMed]
- Miyoshi T, Aokage K, Katsumata S, Tane K, Ishii G, Tsuboi M. Ground-Glass Opacity Is a Strong Prognosticator for Pathologic Stage IA Lung Adenocarcinoma. Ann Thorac Surg 2019;108:249-55. [Crossref] [PubMed]
- Yang J, Wang H, Geng C, Dai Y, Ji J. Advances in intelligent diagnosis methods for pulmonary ground-glass opacity nodules. Biomed Eng Online 2018;17:20. [Crossref] [PubMed]
- Hu H, Wang Q, Tang H, Xiong L, Lin Q. Multi-slice computed tomography characteristics of solitary pulmonary ground-glass nodules: Differences between malignant and benign. Thorac Cancer 2016;7:80-7. [Crossref] [PubMed]
- Prabhu M, Raju S, Chakraborty D, Arora S, Kumar R. Spectrum of 18F-FDG Uptake in Bilateral Lung Parenchymal Diseases on PET/CT. Clin Nucl Med 2020;45:e15-9. [Crossref] [PubMed]
- Capitanio S, Nordin AJ, Noraini AR, Rossetti C. PET/CT in nononcological lung diseases: current applications and future perspectives. Eur Respir Rev 2016;25:247-58. [Crossref] [PubMed]
- Chang JM, Lee HJ, Goo JM, Lee HY, Lee JJ, Chung JK, Im JG. False positive and false negative FDG-PET scans in various thoracic diseases. Korean J Radiol 2006;7:57-69. [Crossref] [PubMed]
- Nomori H, Watanabe K, Ohtsuka T, Naruke T, Suemasu K, Uno K. Evaluation of F-18 fluorodeoxyglucose (FDG) PET scanning for pulmonary nodules less than 3 cm in diameter, with special reference to the CT images. Lung Cancer 2004;45:19-27. [Crossref] [PubMed]
- Scafoglio CR, Villegas B, Abdelhady G, Bailey ST, Liu J, Shirali AS, Wallace WD, Magyar CE, Grogan TR, Elashoff D, Walser T, Yanagawa J, Aberle DR, Barrio JR, Dubinett SM, Shackelford DB. Sodium-glucose transporter 2 is a diagnostic and therapeutic target for early-stage lung adenocarcinoma. Sci Transl Med 2018;10:eaat5933.